2020: Expanding AI’s Impact With Organizational Learning
MIT Sloan Management Review, in collaboration with
BCG — Boston Consulting Group
By Sam Ransbotham, Shervin Khodabandeh, David Kiron, François Candelon, Michael Chu, and Burt LaFountain
Findings from the 2020 Artificial Intelligence Global Executive Study and Research project
Excerpt by
Joaquim Cardoso MSc.
Digital and Health Strategy (DHS)
Institute for better health, care, cost and universal health
July 1, 2022
Better Together: How Humans and AI Interact
Matching the right mode of human-machine interaction to the situation affects whether feedback advances or limits organizational learning with AI.
In some processes, managers design AI solutions to largely work alone. For example, at toolmaker Stanley Black & Decker, image-processing algorithms monitor the quality of its tape-measure manufacturing.
Cameras capture images as tape measures pass through various points of manufacture and flag defects in real time before the company wastes additional resources on defective tapes.
These AI systems work independently in real time because waiting for human input would slow the process.
But humans still have a role, because as Carl March, Industry 4.0 director of analytics at Stanley Black & Decker, says, “Defects, at times, still warrant some actions to give additional validation, as there are sometimes gray areas.”
The process still involves human effort in exceptional cases, but it doesn’t have to slow the main process flow.
FIGURE 5: LEADERS SUCCESSFULLY DEPLOY MULTIPLE INTERACTION MODES
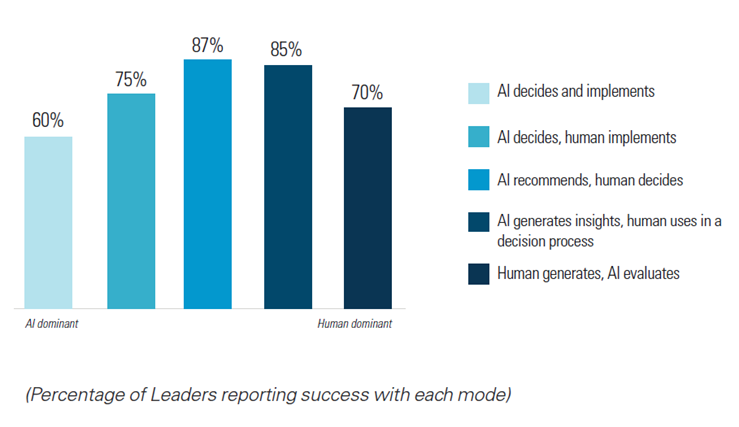
Leaders successfully integrate humans and AI.
FIGURE 6: MORE INTERACTION MODES LEAD TO BETTER RESULTS
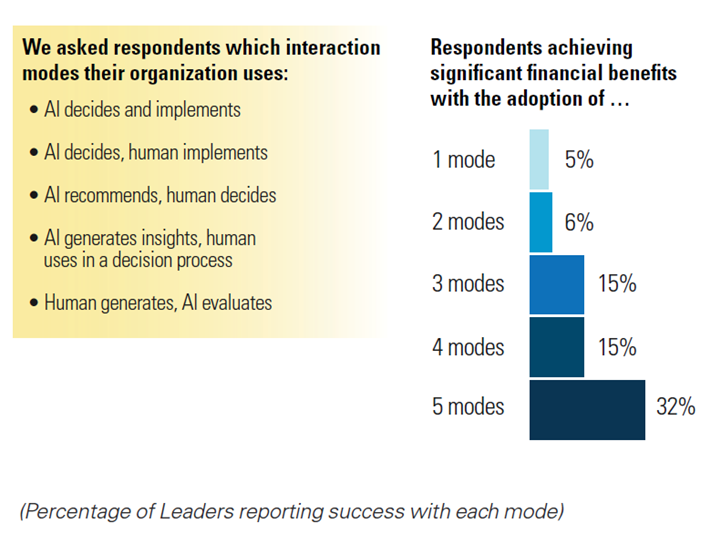
Organizations adopting multiple modes of human-AI interaction are more likely to achieve significant financial benefits with AI.
Other processes may require a much greater role for human discernment and input.
At Walmart, employees not only have extensive operational experience managing in-store product assortment but also an ability to understand context that even extensive historical data lacks — such as sudden, radical shifts due to the COVID-19 pandemic.
Machine learning depends on historical data being relevant to current and future states. “But when faced with COVID,” Walmart vice president of machine learning Prakhar Mehrotra says, “the world completely changed and we could no longer predict the future from the past.”
For assortment management, Walmart designs AI solutions to present recommendations to managers.
Managers can agree, disagree, and comment to improve both the current recommendation and future recommendations. In a COVID-19 world, management disagreement with AI solutions was a critical source of new machine learning.
Walmart ensures that decisions reflect the entirety of knowledge available — in databases as well as in people’s heads. Well-designed roles support mutual learning.
Our survey results reveal five ways humans and AI work together. Leaders use each mode well but report particular proficiency integrating human and AI roles. (See Figure 5, page 10.)
Our survey results reveal five ways humans and AI work together. Leaders use each mode well but report particular proficiency integrating human and AI roles.
Leaders haven’t found just one way to structure and refine human-AI interactions.
Instead, they deploy multiple modes of human-AI interaction. Organizations that successfully use all five modes are six times more likely to attain significant financial benefits than those able to use just one or two.
Furthermore, companies gain the most when they increase their expertise from four to five modes. (See Figure 6, page 10.)
Broader competencies allow organizations to fit a wider variety of interaction modes to a wider variety of situations.
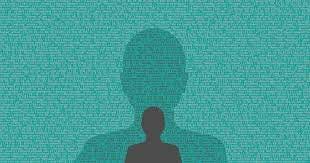
Repsol illustrates how a single organization can use these five distinct modes of human-AI interaction, deploying each as situations require.
Al decides and implements: In this mode, AI has nearly all the context and can quickly make decisions. Human involvement would only slow down an otherwise fast process. Repsol embeds AI in its customer relationship management system to deliver real-time personalized offers, like discounts and free car washes, to consumers at its 5,000 retail service stations, with humans providing only a light layer of oversight and supervision and maintaining compliance with local regulations. (See the sidebar “Responsible AI.”)
AI decides, human implements: AI can capture the context well and make decisions, but humans — rather than software or robotics, for instance — implement the solutions. Repsol uses this mode for AI predictive maintenance in offshore production facilities. AI identifies parts at risk of failure and schedules a maintenance review. Post-review, human operators then schedule the replacement, taking into account part availability and scheduled maintenance.
BOX: RESPONSIBLE AI
The term responsible AI doesn’t yet have a universally accepted definition. For our survey, we defined responsible AI in terms of improving fairness in algorithms and reducing biases in decision-making; promoting inclusivity and a diversity of perspectives; providing model interpretability and explainability to AI end users; ensuring data privacy and security in AI; complying with legal requirements; and monitoring the social impact and ethics of AI. Among Leaders, 90% say they are developing or already have developed responsible AI strategies. But action lags intent — only 57% of Leaders have specific roles and processes to enforce responsible AI. A smaller percentage have an appointed leader for responsible AI (52%), and an even smaller percentage offer training on responsible AI processes and practices (43%).
That doesn’t surprise Alice Xiang, head of fairness, transparency, and accountability research at the Partnership on AI, a research institute focused on the intersection of AI and society. “There is a constant struggle,” she says. “Organizations often don’t have a business incentive to engage and invest in this space unless they are subject to legal or reputational risk.”
Compliance and risk management need not be the only motivations for adopting responsible AI practices. Of the organizations that already have in place responsible AI strategies, 72% find that these strategies actually increase the financial benefits of AI, and 62% report that they decrease operational risk. If organizations view responsible AI practices only from a compliance perspective, they may miss out on financial benefits. Responsible AI doesn’t have to be altruistic; it can make financial sense.
Mutual learning is an ongoing process that improves both human and machine decision-making.
AI recommends, human decides: This mode is appropriate when organizations must make a large number of decisions repeatedly and the AI can incorporate most but not all of the business context. Repsol’s AI for crude-oil blending integrates and analyzes millions of factors, including the type of crude and the operating conditions of the refinery, to recommend blending schedules for the next 30 days. Humans then decide which blending schedule to use, depending on expected global market conditions.
AI generates insights, human uses them in a decision process: In this mode, inherently creative work requires human thought, but AI insights can inform the process. For workforce planning, algorithms at Repsol combine forecasts from machine learning models with human experience and insight to determine future workforce needs. Human resource managers use the result as input to hiring and training plans.
“Algorithms don’t know an org chart … They cut across the organization.” –Prakhar Mehrotra, vice president, machine learning, Walmart
Human generates, AI evaluates: Humans generate many hypothetical situations but rely on AI to tediously assess many complex dependencies. For example, Repsol uses digital twins of physical assets, such as wells, to simulate the consequences of possible operational changes and validate hypotheses. Using multiple engineering and operational efficiency models, managers can simulate consequences before actually changing a physical well.
Furthermore, organizations not only need to use these multiple modes of human-machine interaction but also must be able to switch between these interaction modes as changing contexts demand. For example, the sudden and massive changes in behaviors driven by 2020 COVID-19 pandemic lockdowns showed that certain algorithms required more human oversight. At Walmart, for example, Mehrotra describes the early days of the pandemic as “a type of cold-start problem” and says the question was, “How quickly can AI agents learn what has recently happened?” Initially, processes required a larger human role, but as data accrued, the AI system could make better recommendations. Even well-designed processes may change as situations demand, and Leaders are prepared to change defined roles as required.
Our research shows that organizational learning with AI depends on success with three aspects of human-machine interactions:
- breadth (using as many of the five modes as possible),
- fit (selecting the appropriate mode for each context), and
- agility (switching between modes as needed).[1]
[1] Our survey results indicate that smaller businesses — in contrast with larger organizations — capture most of their AI-related benefits from learning with the most autonomous mode (AI decides and implements). One reason may be that smaller companies typically have less organizational complexity, which can make it easier to realize significant financial benefits from automation than at larger companies.
About the authors:
SAM RANSBOTHAM, is a professor in the information systems department at the Carroll School of Management at Boston College, as well as guest editor for MIT Sloan Management Review’s Artificial Intelligence and Business Strategy Big Ideas initiative.
SHERVIN KHODABANDEH, is a senior partner and managing director at BCG, and the co-leader of BCG GAMMA (BCG’s AI practice) in North America. He can be contacted at shervin@bcg.com.
DAVID KIRON, is the editorial director of MIT Sloan Management Review, where he directs the publication’s Big Ideas program. He can be contacted at dkiron@mit.edu.
FRANÇOIS CANDELON, is a senior partner and managing director at BCG, and the global director of the BCG Henderson Institute.
MICHAEL CHU, is a partner and associate director at BCG, and a core member of BCG GAMMA.
BURT LAFOUNTAIN is a partner and managing director at BCG, and a core member of BCG GAMMA.
SPECIAL CONTRIBUTORS
Rodolphe Charme di Carlo and Allison Ryder
To cite this report, please use:
Ransbotham, S. Khodabandeh, D. Kiron, F. Candelon, M. Chu, and B. LaFountain, “Expanding AI’s Impact With Organizational Learning,” MIT Sloan Management Review and Boston Consulting Group, October 2020.
Originally published at: https://sloanreview.mit.edu