A Rutgers analysis shows that artificial intelligence approaches to precision medicine are advancing rapidly, but many suffer from disorganization and gaps.
Health IT Analytics
By Shania Kennedy
August 22, 2022
Site Editor:
Joaquim Cardoso MSc.
health transformation — institute for research, strategy and advisory
October 26, 2022
Researchers at Rutgers have concluded that …
… artificial intelligence (AI)-based approaches to precision medicine are limited or slowed by various structural issues, such as disorganization, data standardization, and gaps in approaches used.
The analysis evaluated and compared 32 of the most prevalent AI approaches to study preventive treatments for multiple diseases, including obesity, Alzheimer’s, inflammatory bowel disease, breast cancer and major depressive disorder.
The researchers found that out of all the approaches evaluated, none could be used for all treatments, and programs that could evaluate multiple treatments were limited.
These findings, the authors note, indicate that the field of AI-based precision medicine is advancing rapidly, but suffers from significant disorganization, which has the potential to hamper future progress.
Because AI and precision medicine both incorporate a wealth of different data and approaches, many different computing approaches have cropped up.
These findings, the authors note, indicate that the field of AI-based precision medicine is advancing rapidly, but suffers from significant disorganization, which has the potential to hamper future progress.
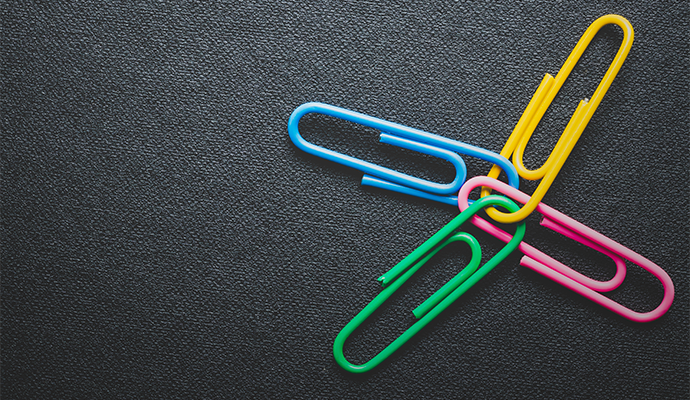
“Precision medicine is one of the most trending subjects in basic and medical science today,” …
… said Zeeshan Ahmed, PhD, lead author on the study and an assistant professor of medicine at Rutgers Robert Wood Johnson Medical School, in the press release.
“Major reasons include its potential to provide predictive diagnostics and personalized treatment to variable known and rare disorders.
However, until now, there has been very little effort exerted in organizing and understanding the many computing approaches to this field.
We want to pave the way for a new data-centric era of discovery in health care.”
The research team also argues that genetics is “arguably the most data-rich and complex component of precision medicine,” …
… underscoring the importance of evaluating the objectives, methodologies, data sources, ethics, and gaps in approaches used for any precision medicine research or models.
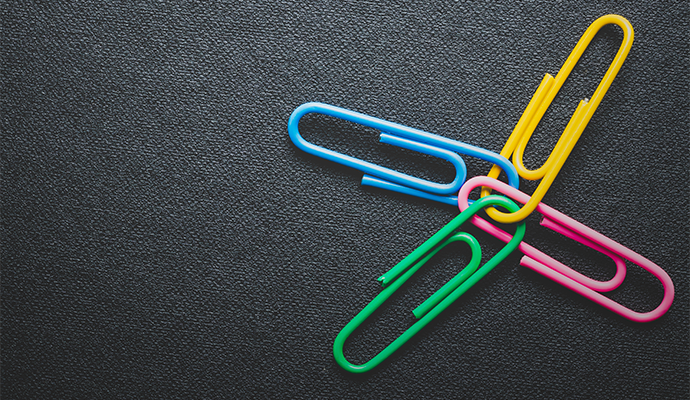
To address the issues presented in the analysis, the researchers suggest that the scientific community embrace the major challenges, …
… like improved data standardization and enhanced protection of personal identifying information, while also being vigilant for technical issues, such as needing to correct errors in genomic and clinical data.
“AI has the potential to play a vital role to achieve significant improvements in providing better individualized and population healthcare at lower costs,” Ahmed said.
“We need to strive to address possible challenges that continue to slow the advancements of this breakthrough treatment approach.”
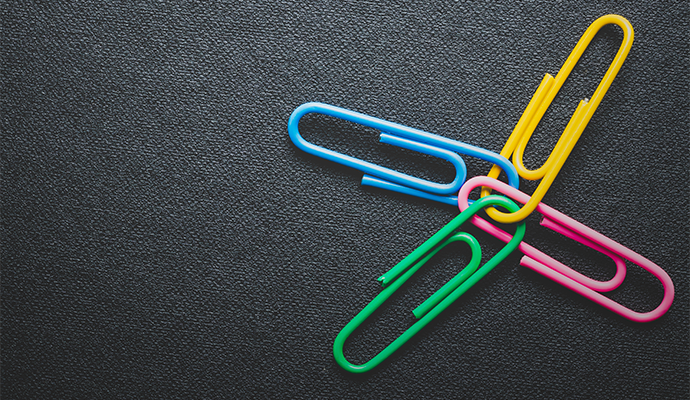
Limitations and challenges in AI approaches also limit advances in other areas.
In a recent interview with HealthITAnalytics, Rosalind Picard, founder and director of the Affective Computing Research Group at the Massachusetts Institute of Technology (MIT) Media Lab and principal investigator at MIT’s Jameel Clinic, outlined …
… how limitations in AI and wearables negatively impact depression research.
Bias in AI is also a major hurdle slowing its uptake in healthcare, …
… but strategies for avoiding algorithmic bias and research-based equitable healthcare algorithm frameworks are part of a growing effort to address these problems and promote health equity.
Originally published at https://healthitanalytics.com
ORIGINAL PUBLICATION (excerpt)
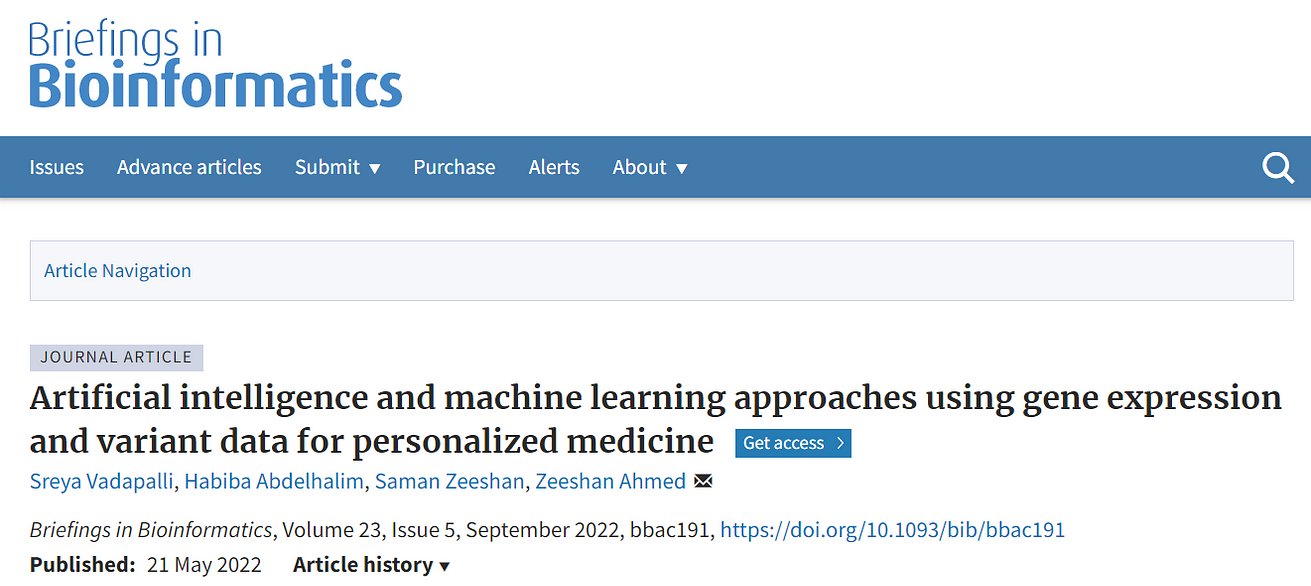
Artificial intelligence and machine learning approaches using gene expression and variant data for personalized medicine
Briefings in Bioinformatics
Sreya Vadapalli, Habiba Abdelhalim, Saman Zeeshan, Zeeshan Ahmed
21 May 2022
Abstract
What is the opportunity?
- Precision medicine uses genetic, environmental and lifestyle factors to more accurately diagnose and treat disease in specific groups of patients, and it is considered one of the most promising medical efforts of our time.
- The use of genetics is arguably the most data-rich and complex components of precision medicine.
What are the challenges?
- The grand challenge today is the successful assimilation of genetics into precision medicine that translates across different ancestries, diverse diseases and other distinct populations,
which will require clever use of artificial intelligence (AI) and machine learning (ML) methods.
What is the scope of the paper?
- Our goal here was to review and compare scientific objectives, methodologies, datasets, data sources, ethics and gaps of AI/ML approaches used in genomics and precision medicine.
- We selected high-quality literature published within the last 5 years that were indexed and available through PubMed Central.
- Our scope was narrowed to articles that reported application of AI/ML algorithms for statistical and predictive analyses using whole genome and/or whole exome sequencing for gene variants, and RNA-seq and microarrays for gene expression.
- We did not limit our search to specific diseases or data sources.
What are the findings?
- Based on the scope of our review and comparative analysis criteria, we identified 32 different AI/ML approaches applied in variable genomics studies and report widely adapted AI/ML algorithms for predictive diagnostics across several diseases.
REFERENCE PUBLICATION
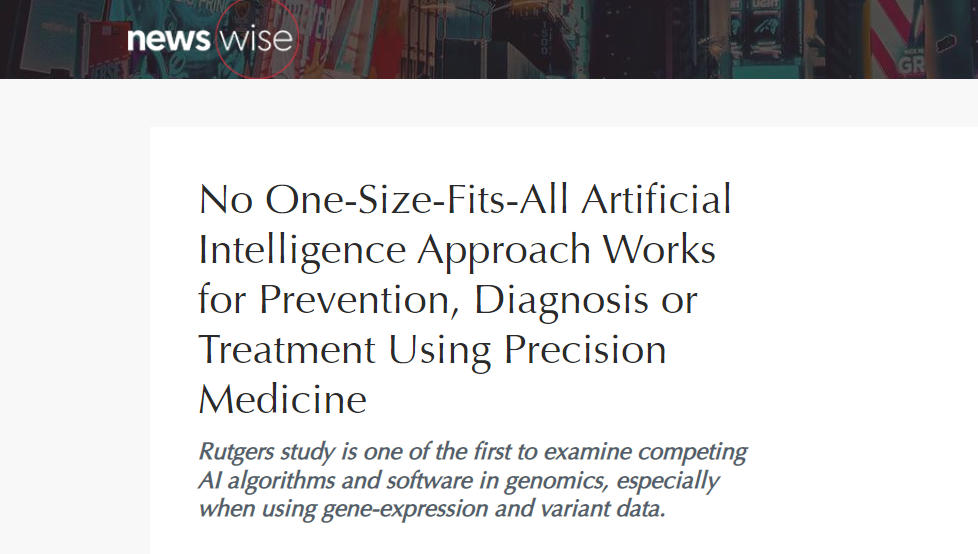
No One-Size-Fits-All Artificial Intelligence Approach Works for Prevention, Diagnosis or Treatment Using Precision Medicine
Rutgers study is one of the first to examine competing AI algorithms and software in genomics, especially when using gene-expression and variant data.
News Wise
by Rutgers University-New Brunswick
18-Aug-2022
A Rutgers analysis of dozens of artificial intelligence (AI) software programs used in precision, or personalized, medicine to prevent, diagnose and treat disease found that no program exists that can be used for all treatments.
“Precision medicine is one of the most trending subjects in basic and medical science today,” said Zeeshan Ahmed, an assistant professor of medicine at Rutgers Robert Wood Johnson Medical School who led the study, published in Briefings in Bioinformatics.
“Major reasons include its potential to provide predictive diagnostics and personalized treatment to variable known and rare disorders.
However, until now, there has been very little effort exerted in organizing and understanding the many computing approaches to this field.
We want to pave the way for a new data-centric era of discovery in health care.”
Precision medicine, a technology still in its infancy, is an approach to treatment that uses information about an individual’s medical history and genetic profile and relates it to the information of many others to find patterns that can help prevent, diagnose or treat a disease.
Precision medicine, a technology still in its infancy, is an approach to treatment that uses information about an individual’s medical history and genetic profile and relates it to the information of many others to find patterns that can help prevent, diagnose or treat a disease.
The AI-based approach rests on a high level of both computing power and machine-learning intelligence because of the enormous scope of medical and genetic information scoured and analyzed for patterns.
The comparative and systematic review, believed by the authors to be one of the first of its kind, identified 32 of the most prevalent precision medicine AI approaches used to study preventive treatments for a range of diseases, including obesity, Alzheimer’s, inflammatory bowel disease, breast cancer and major depressive disorder.
The bevy of AI approaches analyzed in the study — the researchers combed through five years of high-quality medical literature — suggest the field is advancing rapidly but is suffering from disorganization, Ahmed said.
In AI, software programs simulate human intelligence processes. In machine learning, a subcategory of AI, programs are designed to “learn” as they process more and more data, becoming ever more accurate at predicting outcomes.
The effort rests on algorithms, step-by-step procedures for solving a problem or performing a computation.
Researchers such as Ahmed, who conducts studies on cardiovascular genomics at the Rutgers Institute for Health, Health Care Policy and Aging Research (IFH), are racing to collect and analyze complex biological data while also developing the computational systems that undergird the endeavor.
Researchers … are racing to collect and analyze complex biological data while also developing the computational systems that undergird the endeavor.
Because the use of genetics is “arguably the most data-rich and complex component of precision medicine,” Ahmed said, the team focused especially on reviewing and comparing scientific objectives, methodologies, data sources, ethics and gaps in approaches used.
Those interested in precision medicine, he said, can look to the paper for guidance as to which AI programs may be best suited for their research.
Those interested in precision medicine, he said, can look to the paper for guidance as to which AI programs may be best suited for their research.
To aid the advent of precision medicine, the study concluded that the scientific community needs to embrace several “grand challenges,” from addressing general issues such as improved data standardization and enhanced protection of personal identifying information to more technical issues such as correcting for errors in genomic and clinical data.
To aid the advent of precision medicine, the study concluded that the scientific community needs to embrace several “grand challenges,” : (1) from addressing general issues such as improved data standardization and (2) enhanced protection of personal identifying information (3) to more technical issues such as correcting for errors in genomic and clinical data.
“AI has the potential to play a vital role to achieve significant improvements in providing better individualized and population healthcare at lower costs,” Ahmed said.
“We need to strive to address possible challenges that continue to slow the advancements of this breakthrough treatment approach.”
Other Rutgers researchers involved in the study included Sreya Vadapalli and Habiba Abdelhalim, research assistants at the IFH, and Saman Zeeshan, a bioinformatics research scientist and former postdoctoral research associate at the Rutgers Cancer Institute of New Jersey.
Names mentioned:
Zeeshan Ahmed, PhD, assistant professor of medicine at
Rutgers Robert Wood Johnson Medical School
Rosalind Picard, founder and director of the Affective Computing Research Group at the Massachusetts Institute of Technology (MIT) Media Lab and principal investigator at MIT’s Jameel Clinic,
Other Rutgers researchers involved in the study included
Sreya Vadapalli and
Habiba Abdelhalim, research assistants at the IFH, and
Saman Zeeshan, a bioinformatics research scientist and former postdoctoral research associate at the
Rutgers Cancer Institute of New Jersey.