This is a republication of an excerpt of the paper “Computational pathology in 2030 [and the future role of “Pathologists”] : a Delphi study forecasting the role of AI in pathology within the next decade”, with the title above, focusing on the topic in question.
Computational pathology in 2030 [and the future role of “Pathologists”] : a Delphi study forecasting the role of AI in pathology within the next decade
institute for health transformation (InHealth)
Joaquim Cardoso MSc
Founder, Chief Researcher & Editor
and CSO
January 25, 2023
EXECUTIVE SUMMARY
Key Message(s)
- Experts agreed that AI would be routinely and impactfully used within AP laboratory and pathologist clinical workflows by 2030.
- High consensus was reached on 100 items across nine categories encompassing the impact of AI on
- (1) pathology key performance indicators (KPIs) and
- (2) the pathology workforce and specific tasks performed by
- (3) pathologists and
- (4) AP lab technicians, as well as
- (5) specific AI applications and their likelihood of routine use by 2030,
- (6) AI’s role in integrated diagnostics,
- (7) pathology tasks likely to be fully automated using AI, and
- (8) regulatory/legal and
- (9) ethical aspects of AI integration in pathology.
Background
- Artificial intelligence (AI) is rapidly fuelling a fundamental transformation in the practice of pathology.
- However, clinical integration remains challenging, with no AI algorithms to date in routine adoption within typical anatomic pathology (AP) laboratories.
- This survey gathered current expert perspectives and expectations regarding the role of AI in AP from those with first-hand computational pathology and AI experience.
Methods
- Perspectives were solicited using the Delphi method from 24 subject matter experts between December 2020 and February 2021 regarding the anticipated role of AI in pathology by the year 2030.
- The study consisted of three consecutive rounds:
- 1) an open-ended, free response questionnaire generating a list of survey items;
- 2) a Likert-scale survey scored by experts and analysed for consensus; and
- 3) a repeat survey of items not reaching consensus to obtain further expert consensus.
Findings
- Consensus opinions were reached on 141 of 180 survey items (78.3%).
- Experts agreed that AI would be routinely and impactfully used within AP laboratory and pathologist clinical workflows by 2030.
- High consensus was reached on 100 items across nine categories encompassing the impact of AI on
(1) pathology key performance indicators (KPIs) and
(2) the pathology workforce and specific tasks performed by
(3) pathologists and
(4) AP lab technicians, as well as
(5) specific AI applications and their likelihood of routine use by 2030,
(6) AI’s role in integrated diagnostics,
(7) pathology tasks likely to be fully automated using AI, and
(8) regulatory/legal and
(9) ethical aspects of AI integration in pathology.
Interpretation
- This systematic consensus study details the expected short-to-mid-term impact of AI on pathology practice.
- These findings provide timely and relevant information regarding future care delivery in pathology and raise key practical, ethical, and legal challenges that must be addressed prior to AI’s successful clinical implementation.
INFOGRAPHIC
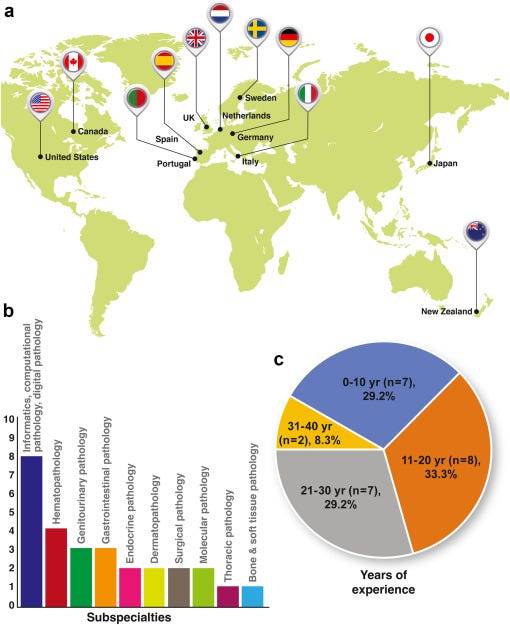
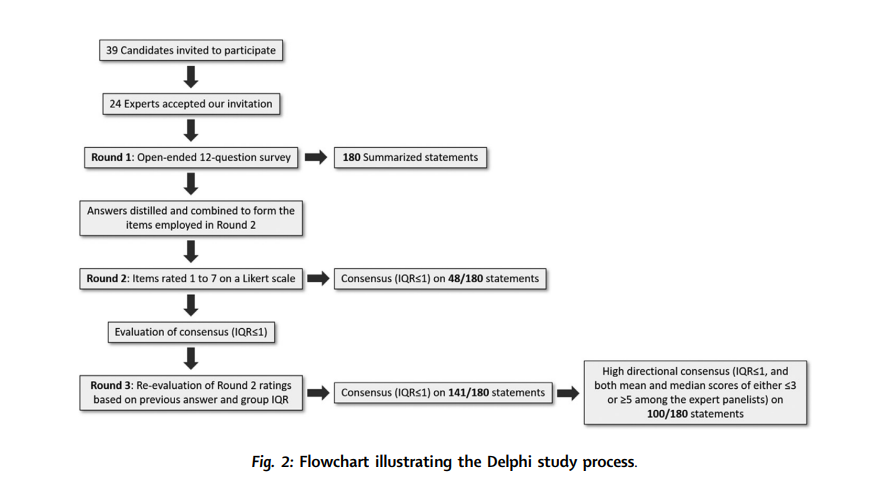
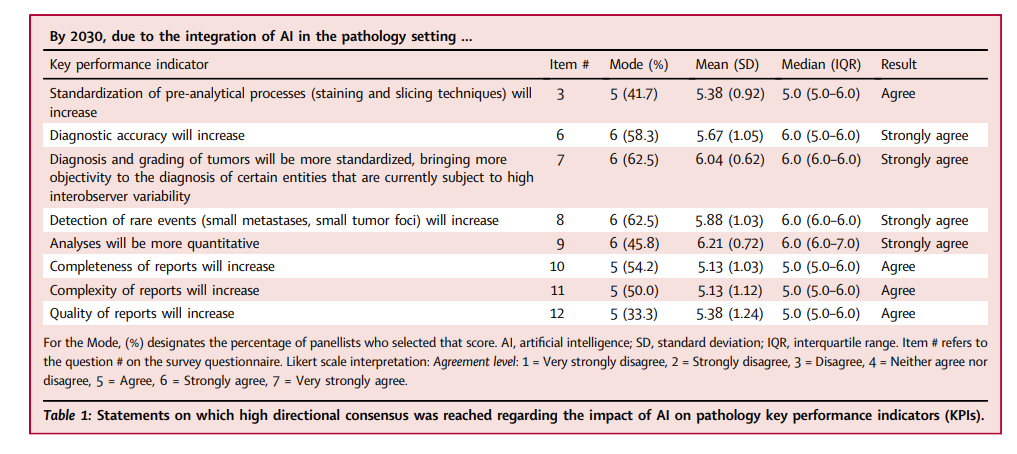
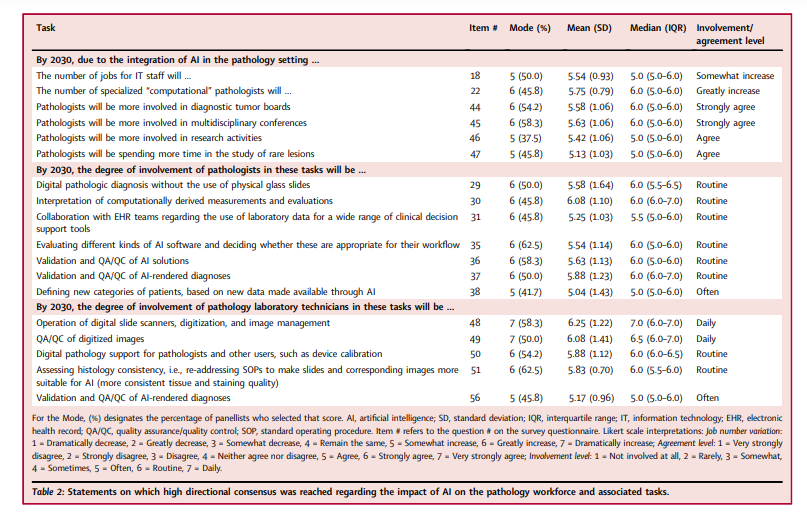
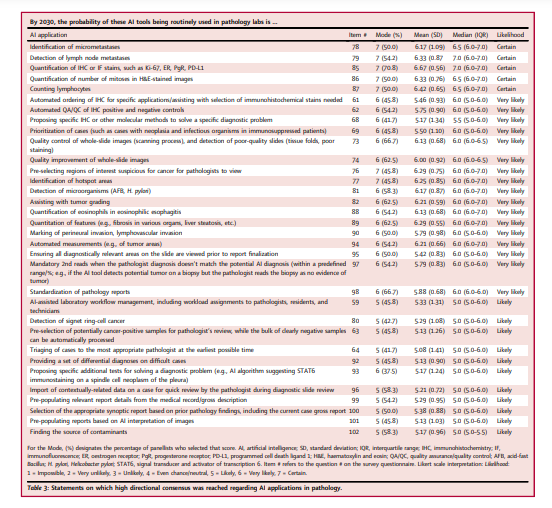
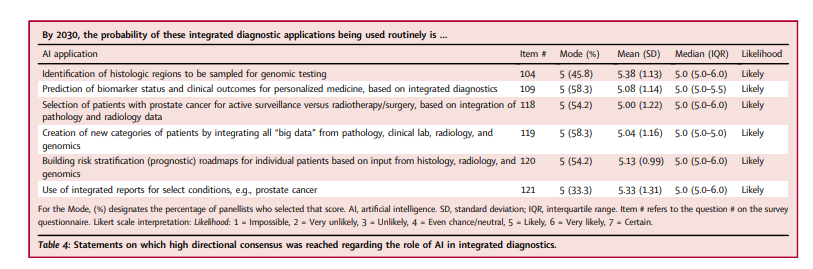
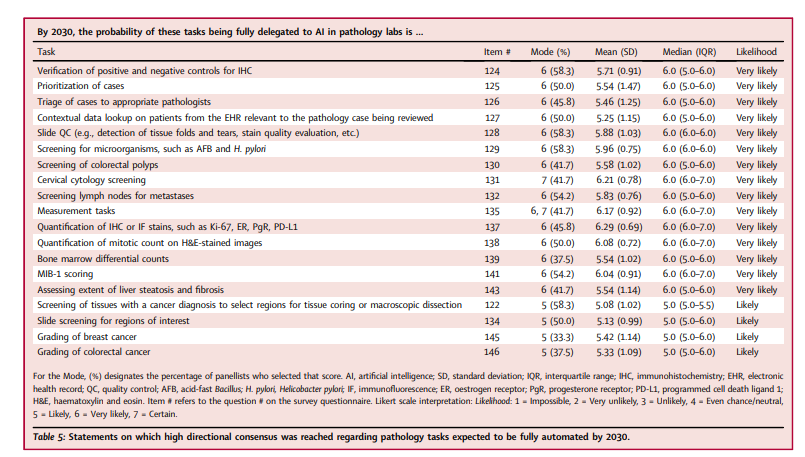
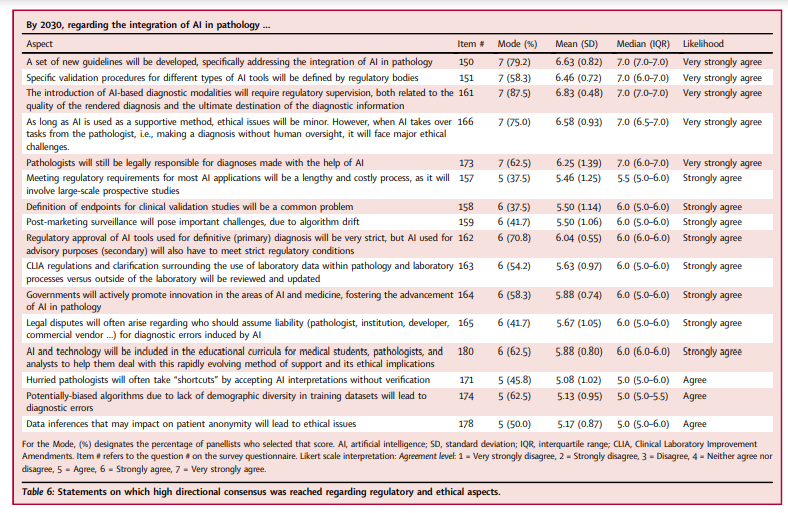
ORIGINAL PUBLICATION (excerpt)
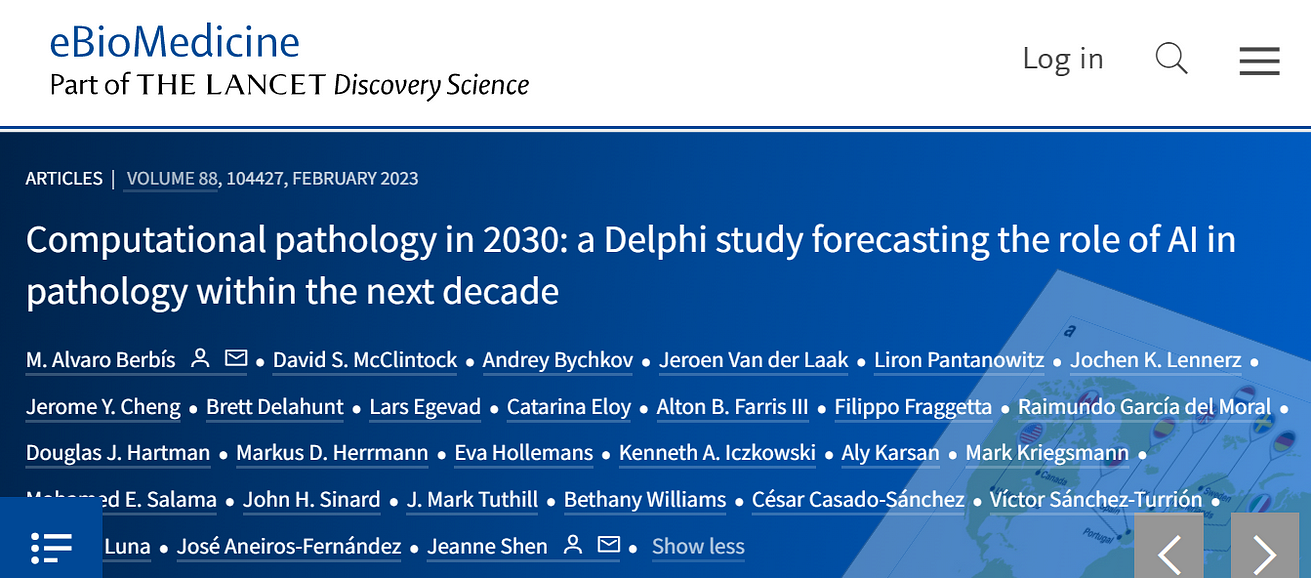
Computational pathology in 2030: a Delphi study forecasting the role of AI in pathology within the next decade
The Lancet — eBiomedicine
M. Alvaro Berbís ,a,b, * David S. McClintock,c Andrey Bychkov,d Jeroen Van der Laak,e Liron Pantanowitz,f Jochen K. Lennerz,g Jerome Y. Cheng,f Brett Delahunt,h Lars Egevad,i Catarina Eloy,j Alton B. Farris III,k Filippo Fraggetta,l Raimundo García del Moral,m Douglas J. Hartman,n Markus D. Herrmann,o Eva Hollemans,p Kenneth A. Iczkowski,q Aly Karsan,r Mark Kriegsmann,s Mohamed E. Salama,t John H. Sinard,u J. Mark Tuthill,v Bethany Williams,w César Casado-Sánchez,x Víctor Sánchez-Turrión,y Antonio Luna,z José Aneiros-Fernández,a,l and Jeanne Shenaa, **
January 03, 2023
Introduction
Artificial intelligence (AI) is set to fuel an unprecedented transformation in healthcare by contributing to more accurate diagnoses, more agile, cost-effective, and standardized clinical workflows, and more effective and personalized treatments.
Excitement and expectations regarding its potential have continued to build, as evidenced by the growing list of medical AI publications (from just 203 articles in 2005 to 12,563 in 2019).
Pathology has attracted attention as an image-rich specialty likely to be strongly impacted by advances in AI and was recently the most-published specialty among 17 specialties engaged in medical AI research.
The development of machine learning-based tools for image analysis has led to a surge in AI applications promising to revolutionize pathology workflows, and the advent of a new field, computational pathology (CPath).
Key examples of AI application in anatomic pathology (AP) include
- automated assessment of prognostic biomarkers such as Ki-67 in breast cancer,
- tumour grading in prostate cancer,
- diagnosis of metastatic breast cancer in lymph nodes,
- and optimization of clinical laboratory workflows, such as automated quality control (QC).
However, few algorithms are currently in routine clinical use, and there is a dearth of studies evaluating their impact in clinical settings.
Simultaneously, ethical concerns have been raised regarding potential data privacy breaches, systemic algorithmic bias, harm related to erroneous AI-generated outputs, and exacerbation of healthcare disparities.
Along with hurdles related to regulatory approval and reimbursement for AI products, these have contributed to a significant AI “translation gap” in pathology, which we define as the failure to prospectively validate and successfully integrate AI models into real-world clinical workflows.
Although various opportunities and challenges surrounding AI in pathology have been extensively discussed in the literature, to date, there has been no systematic survey regarding this topic from the short-to-medium term perspective of digital and CPath experts.
To address this gap, we conducted a consensus survey to gain detailed insight into the current challenges, expectations, and perspectives surrounding the role of AI in pathology, from the standpoint of an international panel of “early adopters”, most of them pathologists in active clinical practice with first-hand experience developing and evaluating the clinical utility of AI algorithms.
For this survey, we applied the Delphi method, a robust, widely accepted tool for building consensus among experts which has outperformed standard statistical methods.
Our goals were to:
- 1) investigate the expected impact of AI on pathology;
- 2) forecast the extent of clinical AI implementation by 2030; and
- 3) provide specific insights into which technical, legal, regulatory, and ethical aspects of AI integration will require the most attention in the coming years.
We expect the results of this study to be of broad interest to a wide range of digital health professionals.
Methods
See the original publication (this is an excerpt version only)
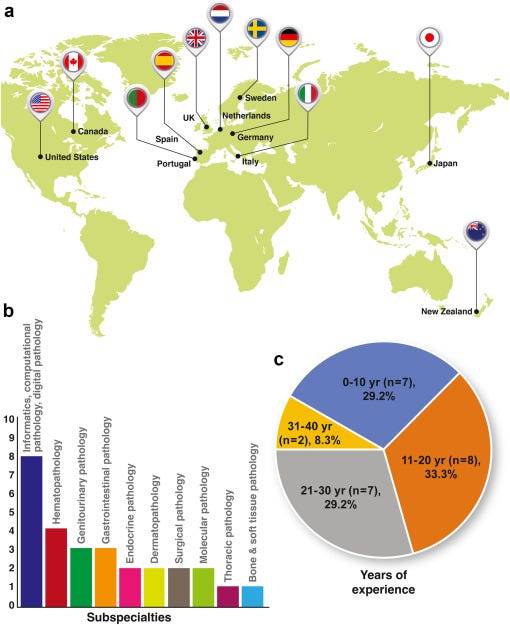
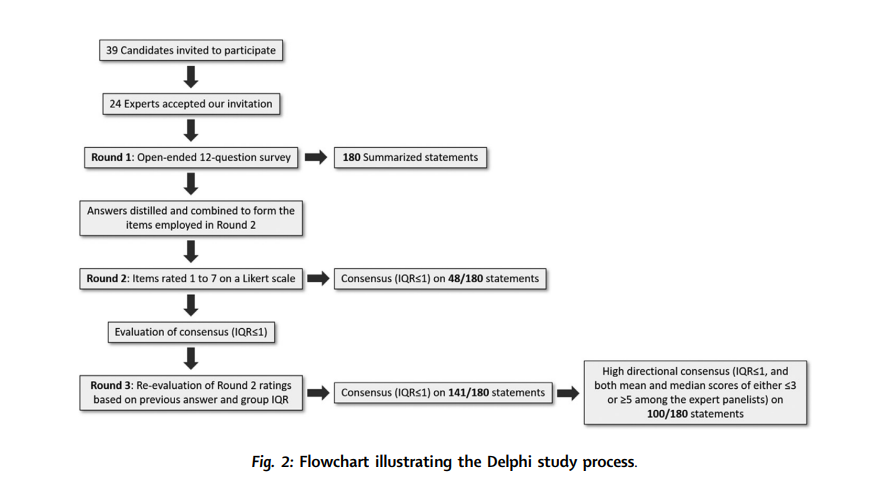
The remaining statements are further discussed in the sections below, which summarize the most significant survey results across the nine domains (Table S4).
- 1.Impact of AI on pathology KPIs
- 2.AI’s impact on the pathology workforce and tasks
- 3.Applications of AI to pathology and integrated diagnostics
- 4.Regulatory and ethical aspects of AI integration in pathology
1.Impact of AI on pathology KPIs
There was agreement that AI would improve multiple laboratory KPIs (Table 1 and Table S4), but that histopathologic analyses would become more quantitative and diagnostic reports more complex.
There was also agreement that AI would lead to greater standardization of diagnostic and pre-analytical processes; as a result, an increase in satisfaction of referring physicians was expected. Statements on the likelihood of cost-per-case and number of second-opinion consultations decreasing with AI use failed to reach consensus, although most predicted that cost-per-case would not decrease, at least within the next 8–10 years. Table 1 indicates that, by 2030, there will be growth in CPath as a subspecialty, with AI applications assisting pathologists in making more accurate, standardized, objective, quantitative, and complete diagnoses.
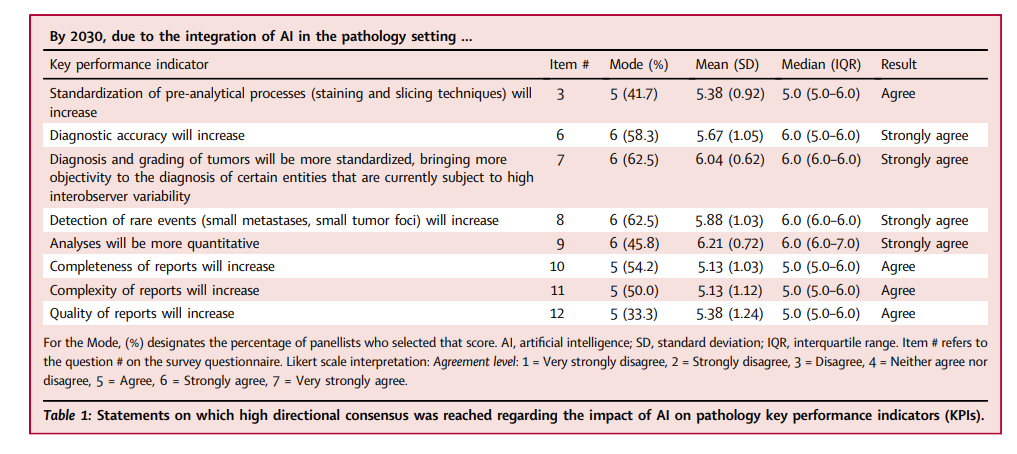
2.AI’s impact on the pathology workforce and tasks
There was agreement that AI adoption would not greatly affect the size of the overall pathology job market; however, the types and frequencies of tasks performed by pathologists and laboratory technicians were expected to change significantly.
It was also agreed that AI would facilitate subspecialisation, with the number of CPathologists greatly increasing (Table 2), and that pathologists would be routinely involved in new tasks related to AI incorporation into their workflows, participating in the development of AI solutions and contributing to the definition of new patient categories. AI adoption was expected to increase pathologist involvement in ancillary activities (research, multidisciplinary conferences, etc.).
Compared to panellists who had been in practice ≥11 years, those in practice ≤10 years more strongly agreed that digital pathologic diagnosis without physical glass slides would be routine by 2030 (p = 0.041, Wilcoxon rank-sum test), with median = 7.0 [IQR 6.0–7.0] (compared to 6.0 [IQR 5.0–6.0], for those in practice ≥11 years) and mean = 6.43 [SD (standard deviation) = 0.79], compared to 5.23 [SD = 1.79] for those in practice ≥11 years.
The same group also more strongly agreed that interpretation of computationally-derived measurements and evaluations would be routine (p = 0.048, Wilcoxon rank-sum test), with median = 7.0 [IQR 6.0–7.0] (compared to 6.0 [6.0–6.0], for those in practice ≥11 years) and mean = 6.71 [SD = 0.49] (compared to 5.82 [SD = 1.19], for those in practice ≥11 years).
The work of pathology technicians was also expected to undergo major changes due to AI adoption (Table 2).
Technicians would routinely be involved in digital and computational workflows by operating scanners, calibrating devices, and QA/QC’ing digitized images. A slight majority of panellists thought that technicians might directly participate in AI-assisted diagnosis, although consensus was not reached.
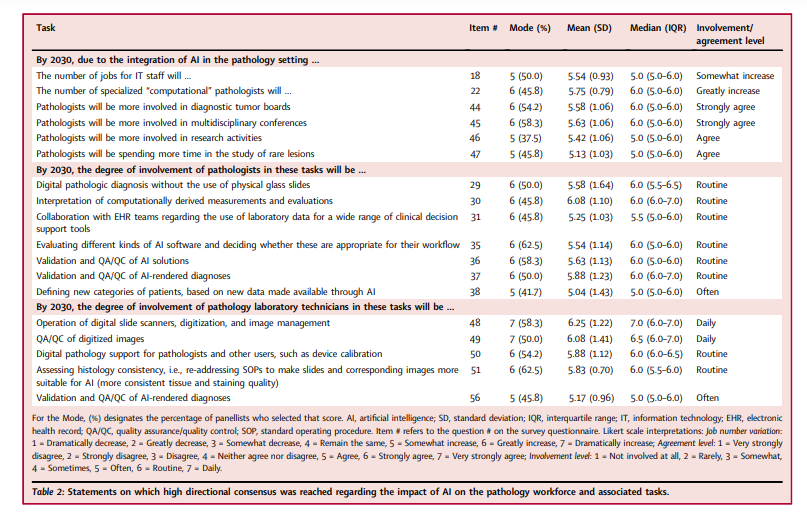
Panellists subspecializing in informatics/digital/CPath more strongly felt that technicians would routinely be providing digital pathology support by performing device calibration and other tasks) (p = 0.0050, Wilcoxon rank-sum test), with median = 7.0 [IQR 6.0–7.0] and mean = 6.63 [SD = 0.52] (compared to median = 6.0 [IQR 5.0–6.0] and mean = 5.50 [SD = 1.15] for those not subspecializing in informatics/digital/CPath).
Similarly, this group felt more strongly that technicians would routinely be involved in assessing and improving the consistency of histologic preparation to make images more suitable for AI (p = 0.049, Wilcoxon rank-sum test), with mean = 6.25 [SD = 0.46] (compared to mean = 5.63 [SD = 0.72], with the same medians of 6.0 [IQR 6.0–6.5 vs 5.0–6.0], for those not subspecializing in informatics/digital/CPath).
3.Applications of AI to pathology and integrated diagnostics
AI was expected to positively impact many aspects of the pathology workflow, with several applications expected to be in routine use by 2030 (Table 3).
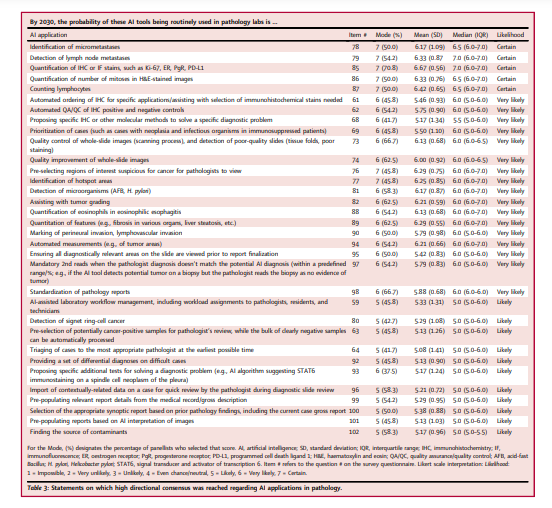
For the analysis and interpretation of histologic images, these included
- algorithms for identifying hotspots (i.e., during mitotic counts),
- microorganisms (acid-fast bacilli and Helicobacter pylori) and cancer,
- and tumour grading.
- There was also certainty that AI would be in routine use for automated quantification of immunohistochemical (IHC) and immunofluorescent (IF) biomarkers,
- counting of mitotic figures and lymphocytes, and lymph node metastasis identification.
Manual tasks expected to be replaced by AI included size measurement and perineural and lymphovascular invasion detection in malignancies.
In addition, it was expected that AI-based computational/virtual staining would replace the need for multiplex IHC/IF.
AI was expected to
- increase diagnostic efficiency by prioritizing regions of interest (ROIs) suspicious for cancer involvement for pathologist review,
- and by pre-populating diagnostic reports using medical records, gross descriptions,
- and AI-generated image interpretations.
In addition, it was expected to facilitate more accurate diagnoses by importing contextually relevant clinical data for pathologist review,
- providing a set of differential diagnoses, and
- prompting second reads on cases with discrepancies between pathologist and AI-rendered diagnoses.
AI was expected to significantly impact laboratory workflows through automated case prioritization and ancillary stain recommendation or ordering (Table 3).
Regarding differences in opinion between the panellist subgroups, those subspecializing in informatics/digital/CPath less strongly believed that AI would routinely be used for eosinophil quantification in eosinophilic esophagitis (p = 0.049 (Wilcoxon rank-sum test), mean = 5.75 [SD = 0.46]) compared to the other subspecialists (mean = 6.31 [SD = 0.70], with the same medians of 6.0 [IQRs 5.5–6.0 vs 6.0–7.0]).
AI was expected to foster the integration of pathology with other diagnostic modalities (Table 4), …
… with multimodal-AI enabling the combination of diverse data types (gross/macroscopic, microscopic, radiologic, and genomic) in a single interface and facilitating integrated diagnostic reporting for diseases such as prostate cancer.
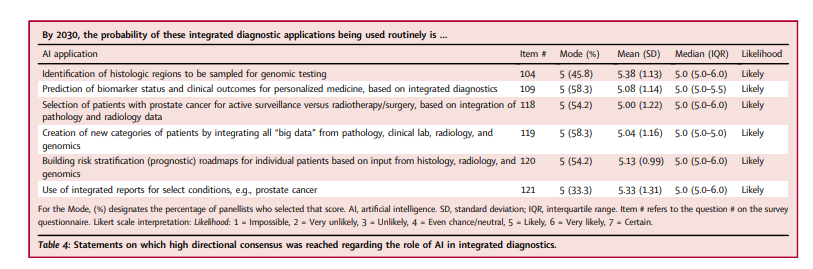
Consequently, it was expected that AI-powered integrated diagnostics would lead to significant advances in personalized healthcare …
… by categorizing patients based on differential risk-stratification (prognostic) roadmaps and clinical outcome predictions.
- Panellists with longer practice experience more strongly believed that the integration of pathologic and radiologic data would routinely be used to select patients for active surveillance versus radiotherapy/surgery in prostate cancer (p = 0.044 (Wilcoxon rank-sum test), equal medians of 5.0 [IQRs 3.0–5.0 vs 5.0–6.0], mean = 5.29 [SD = 1.10] for ≥11 years’ and mean = 4.29 [SD = 1.25] for ≤10 years’ practice experience, respectively).
- They also more strongly believed that AI would routinely be used to build risk stratification roadmaps for patients based on multimodal input data (p = 0.020, Wilcoxon rank-sum test; equal medians of 5.0 [IQR 5.0–6.0 vs 4.0–5.0], mean = 5.41 [SD = 0.94] versus 4.43 [SD = 0.79] for ≥11 years’ and ≤10 years’ practice experience, respectively).
It was thought likely that AI would not simply assist with, but would fully replace, pathologists on several tasks (Table 5), and that work assignment and case triage were likely to be fully AI-automated.
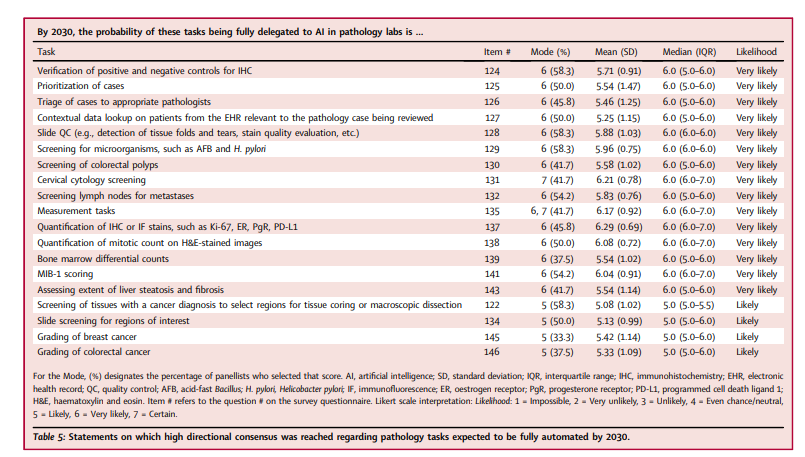
- The panellists practicing outside of North America thought it more likely that colorectal polyp screening would be fully delegated to AI (p = 0.047, Wilcoxon rank-sum test; mean = 6.0 [SD = 0.89] and median = 6 [IQR 6.0–7.0] for non-North American panellists; mean = 5.23 [SD = 1.01] and median = 5.0 [IQR 5.0–6.0] for North American panellists).
- Those in practice longer (≥11 years) thought it more likely that mitotic counts would be fully delegated to AI (p = 0.036, Wilcoxon rank-sum test; equal medians of 6.0, mean = 6.29 [SD = 0.69 vs. 5.57 [0.53] for those with ≥11 and ≤ 10 years’ practice experience, respectively).
4.Regulatory and ethical aspects of AI integration in pathology
The panellists foresaw significant regulatory and ethical challenges posed by AI integration (Table 6) and agreed that both primary diagnostic and secondary (e.g., advisory/assistive) algorithms would have to meet strict regulatory requirements.
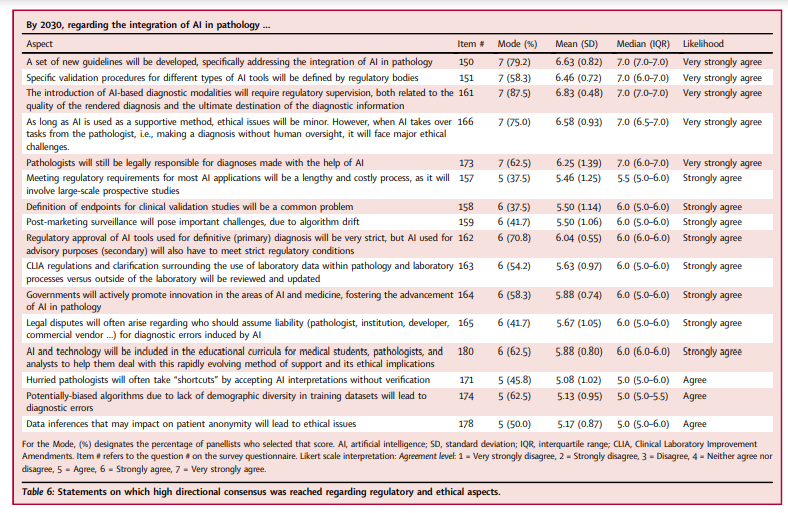
There was agreement that regulatory bodies would create new guidelines addressing AI integration into pathology, providing specific validation procedures, and simplifying regulatory pathways for AI tools, although clearance of AI software would still be a lengthy and costly process.
There was also agreement that the regulatory approval of adaptive algorithms which continuously evolve in response to new input data would be possible, …
… but that algorithm drift would pose important challenges that would need to be addressed through close post-market surveillance.
It was also anticipated that legal disputes would arise regarding liability for diagnostic errors induced by AI, with pathologists still being held legally responsible for AI-assisted diagnoses.
The North American panellists more strongly believed that CLIA regulations and clarification surrounding the use of laboratory data within pathology, as well as laboratory processes, would need to be reviewed and updated (p = 0.031, Wilcoxon rank-sum test; median = 6.0 [IQR 6.0–6.0] vs. median = 5.0 [IQR 5.0–6.0] and mean = 6.0 [SD = 0.82] vs. mean = 5.18 [SD = 0.98] for North American vs. other panellists, respectively).
Those subspecializing in informatics/digital/CPath less strongly believed that legal disputes would often arise regarding liability for AI-induced diagnostic errors (p = 0.018, Wilcoxon rank-sum test; mean = 4.88 [SD = 1.13], median = 5 [4.0–6.0]), compared to those not subspecializing in those areas (mean = 6.06 SD = 0.77], median = 6 [IQR 5.5–7.0]).
It was acknowledged that there would be major ethical issues arising from the full delegation of tasks to AI, such as the likelihood that hurried pathologists would often accept AI interpretations without sufficient verification.
Conversely, there was disagreement regarding whether the “black box” nature of AI algorithms would cause pathologists to often make diagnoses without enough clinical explainability.
Those in practice fewer years (≤10 years) more strongly believed that ethical issues would result from data inferences which might compromise patient anonymity (p = 0.0044, Wilcoxon rank-sum test; mean = 6.0 [SD = 0.82], median = 6.0 [IQR 5.0–7.0]), compared to those in practice ≥11 years (mean = 4.82 [SD = 0.64], median = 5.0 [IQR 4.0–5.0]).
Finally, there was consensus that ethical issues would arise due to:
- 1) risk for diagnostic error from potentially biased algorithms trained on insufficiently diverse datasets; and
- 2) lack of proper informed consent when using patient data (which the panel agreed would become a common practice).
However, it was expected that regulatory bodies would address the preceding ethical and legal challenges, and that funding bodies would actively promote innovation in AI and medicine, thereby fostering the advancement of AI in pathology.
It was also anticipated that AI would be integrated into medical school and continuing medical education curricula in order to help pathologists adapt to this rapidly evolving area and its associated legal and ethical implications.
It was also anticipated that AI would be integrated into medical school and continuing medical education curricula in order to help pathologists adapt to this rapidly evolving area and its associated legal and ethical implications.
Discussion
From this consensus study of 24 experts with first-hand CPath/AI experience, we obtained specific insight into consistently agreed-upon opportunities and challenges, as well as perspectives and predictions, regarding the expected role of AI in pathology over the next decade.
Despite the diversity of nationalities, subspecialties, and years of professional experience represented (with all panellists holding attending pathologist and/or faculty positions), the panellists were able to reach consensus agreement on 140 (78.3%) of the 180 items surveyed.
There was particularly strong consensus that AI would improve the KPI of diagnostic accuracy, …
… at least partially by assisting with the detection of rare events (such as small tumour foci and metastases), standardizing the diagnosis and grading of tumours, and making histopathologic analyses more quantitative.
There was also particularly strong consensus that the number of specialized CPathologists would greatly increase, …
… as would pathologist involvement in multidisciplinary conferences, and that the types of tasks routinely performed by pathology technicians would change significantly.
It was felt to be almost certain that specific pathology AI applications would be routinely used by 2030 (i.e., algorithms for lymph node metastasis identification and mitosis, lymphocyte, and IHC/IF stain quantification).
It was also thought very likely that algorithms would be routinely used for some specific pre-analytical , analytical and post-analytical tasks
It was also thought very likely that algorithms would be routinely used for specific pre-analytical (automated QA/QC, suggestion/ordering of ancillary studies, and case prioritization), analytical (microorganism detection and tumour grading/measurement) and post-analytical tasks (enforcement of mandatory second reads upon significant discrepancies between pathologist and AI-rendered diagnoses).
It was felt to be very likely that many of these tasks, along with colorectal polyp and cervical cytology screening, case triage/assignment, and contextual electronic health record data lookup, would be fully delegated to AI.
These predictions are consistent with existing applications in the pathology AI literature.
Many applications projected to be routinely used by 2030 address basic tasks currently performed by pathologists (in which the ground truth label is typically defined by the pathologist), rather than “aspirational” tasks …
- … such as prediction of molecular biomarker status (including gene expression profiles,
- microsatellite instability,
- mutational status and copy number alterations),
- treatment response, survival,
- and other clinical outcomes directly from morphologic features,
in contrast to the attention paid to these categories by academic researchers and industry stakeholders.
In a recent survey …
… asking 75 computational pathology experts (with medical and non-medical backgrounds) to rank the degree of interest, importance, and/or promise of 12 solid tumour-specific pathology AI applications revealed that …
… the “aspirational” applications were consistently rated most highly.
The somewhat discrepant findings between this and our survey suggest that those with non-medical backgrounds are more optimistic about the near-term role of “aspirational” AI applications.
In a 2018 online survey of pathologists, trainees, and other respondents regarding AI integration into diagnostic pathology,
- 81% of respondents predicted AI integration within 5–10 years,
- 38% felt it would have no impact on pathologist employability,
- only 42% felt it would create new positions and improve employment prospects, and
- 20% were concerned or extremely concerned that AI would displace them from their jobs.
In a 2018 online survey of pathologists, trainees, and other respondents regarding AI integration into diagnostic pathology, … 81% of respondents predicted AI integration within 5–10 years, …
Approximately 28% were unsure of AI’s impact on efficiency or believed that AI would have no or a negative impact on efficiency.
In contrast, our panelists were more optimistic regarding the impact of AI on the pathologist workforce, although there was similar reservation regarding whether AI would truly lead to increased efficiency.
Finally, it is worth noting that our panellists could not reach consensus on 39 of 180 statements (Table S4), such as whether AI would reduce the cost-per-case or number of cases requiring pathologist review or increase patient satisfaction.
- They were uncertain whether AI outputs for clinical decision-making would always need to be reviewed by a pathologist,
- whether their “black box” nature would cause pathologists to make diagnoses without enough clinical explainability,
- whether pathologists would make diagnoses contrary to their own judgment because of AI software recommendations, and
- whether other healthcare professionals could use AI tools to diagnose cases without pathologists.
There was also no consensus on whether AI would lead to de-skilling of pathologists or whether it would be possible to ensure that pathologists took full responsibility for double-checking and confirming AI-rendered diagnoses.
Due to the current AI “translation gap” in pathology, there have been a limited number of studies evaluating the impact of AI tools on pathologist behaviour, laboratory expenditures, medicolegal liability, and patient satisfaction.
The lack of consensus regarding these is expected to be resolved as more AI tools are evaluated in prospective clinical settings and more consideration is directed toward ensuring that tools are integrated into workflows in ways that maximize safety, efficiency, and positive patient outcomes.
Similarly, processes for obtaining regulatory approval for AI tools are expected to evolve as the number of vendors seeking to market Cpath/AI algorithms increases.
In the United States, the centralized Food and Drug Administration (FDA) is responsible for clearing medical devices (including Cpath/AI algorithms) through one of three pathways:
- the premarket approval (PMA),
- the de-novo premarket review,
- or the 510(k) pathway, depending on the risk level of the device and the availability of a previously-approved predicate device).
As of October 5, 2022, only eight unique AI/ML-enabled medical devices have been cleared by the FDA, of which two are for AP (one for identification of prostate cancer in prostate needle biopsies and the other for Pap smear screening); the remaining 6 tools are all for Hematology (peripheral blood cell counting/analysis).
In contrast to the United States, the regulatory approval process in Europe is decentralized, with Conformité Européenne (CE) mark approval being performed by accredited private Notified Bodies.
The number of AI/ML-enabled medical devices with Conformité Européenne (CE) mark approval is greater; from a comprehensive review of devices approved between August 2014 and August 2020, we identified eight additional AP devices (predominantly in the areas of breast and prostate cancer diagnosis, lymph node metastasis detection, breast immunohistochemistry interpretation, and Ki67 hotspot scoring).
In addition to the preceding devices, we were able to identify through an online search 17 more AP devices which had received CE mark approval as of September 2022.
We expect the regulatory landscape to evolve as the list of devices and algorithms grows in the coming decade.
We expect the regulatory landscape to evolve as the list of devices and algorithms grows in the coming decade.
This systematic consensus study was subject to a few limitations.
Given the voluntary nature and substantial time commitment required to complete all rounds, not all invitees agreed to participate, which could have introduced non-response bias.
The inclusion criteria and selection procedure for potential panellists, including use of the PubMed database (which tends to index a larger proportion of English-language publications), could also have led to unintentional geographic bias in our panel, whereby respondents from outside of North America and Europe, such as Asia, Africa, and Latin America, were relatively underrepresented.
As a consequence of the location of practice of most of the participants in North America and Europe, our results may or may not be generalizable to other parts of the world.
We also note the relative underrepresentation of women within the initial candidate (9 of 39) and final panellist (4 of 24) lists, which likely reflects the general underrepresentation of women in the field of AI.
Both the underrepresentation of women and non-North American/European panelists are important limitations of the current study which we hope will be addressed by future more geographically- and gender-diverse surveys that could be more specifically targeted toward demographics not well-represented in the current one.
Lastly, due to the focus of the study on soliciting the opinions of attending pathologist/faculty-level individuals with specific experience in AI/CPath, other potential stakeholders such as pathologists without AI/CPath experience, pathology trainees and technicians, non-pathologist physicians, and patients, were not represented in our panel.
It will be important to take into consideration the opinions of these (and other additional) stakeholder groups, as the field of Cpath/AI moves forward.
In conclusion,
the results of this systematic consensus study have provided a detailed vision of what pathology might look like in 2030, from the standpoint of those with frontline experience developing and evaluating pathology AI tools
AI is expected to have a deep impact on pathology, and our study provides detailed insight into the current challenges and expectations surrounding its role in pathology, including
- timely and relevant information regarding how pathology care might be delivered in the future,
- assuming all regulatory and ethical questions are addressed.
While we expect that our findings will be of great interest to a wide variety of stakeholders, we also hope that the preceding limitations will be sufficiently addressed in forthcoming studies, with our survey and its freely available data collection forms serving as a model for independent validation and extension.
While we expect that our findings will be of great interest to a wide variety of stakeholders, we also hope that the preceding limitations will be sufficiently addressed in forthcoming studies,
Originally published at https://www.thelancet.com
About the authors & affiliations
M. Alvaro Berbís ,a,b, * David S. McClintock,c Andrey Bychkov,d Jeroen Van der Laak,e Liron Pantanowitz,f Jochen K. Lennerz,g Jerome Y. Cheng,f Brett Delahunt,h Lars Egevad,i Catarina Eloy,j Alton B. Farris III,k Filippo Fraggetta,l Raimundo García del Moral,m Douglas J. Hartman,n Markus D. Herrmann,o Eva Hollemans,p Kenneth A. Iczkowski,q Aly Karsan,r Mark Kriegsmann,s Mohamed E. Salama,t John H. Sinard,u J. Mark Tuthill,v Bethany Williams,w César Casado-Sánchez,x Víctor Sánchez-Turrión,y Antonio Luna,z José Aneiros-Fernández,a,l and Jeanne Shenaa, **
a Department of R&D, HT Médica, San Juan de Dios Hospital, Córdoba, Spain b Faculty of Medicine, Autonomous University of Madrid, Madrid, Spain c Department of Laboratory Medicine and Pathology, Mayo Clinic, Rochester, MN, USA d Department of Pathology, Kameda Medical Center, Kamogawa, Chiba, Japan e Department of Pathology, Radboud University Medical Center, Nijmegen, the Netherlands f Department of Pathology, University of Michigan, Ann Arbor, MI, USA g Department of Pathology, Center for Integrated Diagnostics, Massachusetts General Hospital/Harvard Medical School, Boston, MA, USA h Wellington School of Medicine and Health Sciences, University of Otago, Wellington, New Zealand i Department of Oncology-Pathology, Karolinska Institute, Stockholm, Sweden j Pathology Laboratory, Institute of Molecular Pathology and Immunology, University of Porto, Porto, Portugal k Department of Pathology and Laboratory Medicine, Emory University, Atlanta, GA, USA l Pathology Unit, Azienda Sanitaria Provinciale Catania, Gravina Hospital, Caltagirone, Italy mDepartment of Pathology, San Cecilio Clinical University Hospital, Granada, Spain n Department of Anatomic Pathology, University of Pittsburgh Medical Center, Pittsburgh, PA, USA o Department of Pathology, Massachusetts General Hospital and Harvard Medical School, Boston, MA, USA p Department of Pathology, Erasmus University Medical Center, Rotterdam, the Netherlands q Department of Pathology, Medical College of Wisconsin, Milwaukee, WI, USA r Department of Pathology & Laboratory Medicine, University of British Columbia, Michael Smith Genome Sciences Centre, Vancouver, Canada s Institute of Pathology, University Hospital Heidelberg, Heidelberg, Germany t Department of Pathology, Sonic Healthcare, Austin, TX, USA u Department of Pathology, Yale University School of Medicine, New Haven, CT, USA v Department of Pathology, Henry Ford Hospital, Detroit, MI, USA w Department of Histopathology, Leeds Teaching Hospitals NHS Trust, Leeds, UK x Department of Plastic and Reconstructive Surgery, La Paz University Hospital, Madrid, Spain y Department of General Surgery and Digestive Tract, Puerta de Hierro-Majadahonda University Hospital, Madrid, Spain z Department of Integrated Diagnostics, HT Médica, Clínica Las Nieves, Jaén, Spain aaDepartment of Pathology and Center for Artificial Intelligence in Medicine & Imaging, Stanford University School of Medicine, Stanford, CA, USA