the health strategist
research institute for health strategy and
digital health
Joaquim Cardoso MSc.
Chief Research and Strategy Officer (CRSO),
Chief Editor and Senior Advisor
August 18, 2023
What is the message?
Generative AI, with enhanced natural-language capabilities, is projected to drive automation in the modern workplace, significantly impacting knowledge work and higher-wage occupations.
The article discusses McKinsey’s analysis of how generative AI accelerates automation potential, influences adoption timelines, and could contribute to productivity growth by reshaping work activities.
Key takeaways:
- Evolution of Technological Impact on Work: Technology has been reshaping work dynamics for decades, enhancing human capabilities through automation. Machines and computers have enabled workers to perform tasks that were previously beyond their physical or cognitive abilities.
- Generative AI’s Role in Work Automation: Generative AI, a technology with enhanced natural-language capabilities, is poised to follow a similar pattern of augmenting work through automation. It will impact a different set of activities and occupations compared to older technologies.
- McKinsey’s Analysis: McKinsey Global Institute studied the impact of technological automation on work activities and adoption scenarios starting in 2017. The institute initially estimated that 50% of work activities could be automated with existing technology, and it modeled different scenarios for the adoption pace of automation across the global economy.
- Technology Adoption Challenges: Integrating technological capabilities into automated solutions takes time, and economic feasibility plays a role. Adoption of technology across the global economy is a gradual process influenced by factors like investments, deployment, and regulations.
- Acceleration of Automation Potential: Developments in generative AI have accelerated the potential for automation. The percentage of hours that could be automated using existing technology has risen from 50% to 60-70%, largely due to generative AI’s natural-language capabilities.
- Shift in Adoption Timelines: The range of time between early and late adoption scenarios has narrowed, indicating increased confidence in technology capabilities arriving within certain periods.
- Impact on Occupations: Generative AI’s impact will be most significant on knowledge work involving decision-making and collaboration, areas with previously low automation potential. It is likely to affect more-educated workers and higher-wage jobs.
- Automation’s Role in Productivity Growth: The deployment of generative AI and other technologies could boost productivity growth, compensating for declining employment growth. Depending on the rate of adoption, the global economy could experience an annual productivity boost of 0.2% to 3.3% from 2023 to 2040, with generative AI contributing to this growth.
- Shift in Work Activities: To maximize productivity gains, individuals affected by automation need to shift to other work activities that match or exceed their previous productivity levels, potentially involving changes in occupation or activity mix.
- Economic Implications: Generative AI’s potential to reshape work and increase productivity growth could have profound economic implications, particularly in addressing challenges like declining employment growth and slowing economic growth.
Infographics:

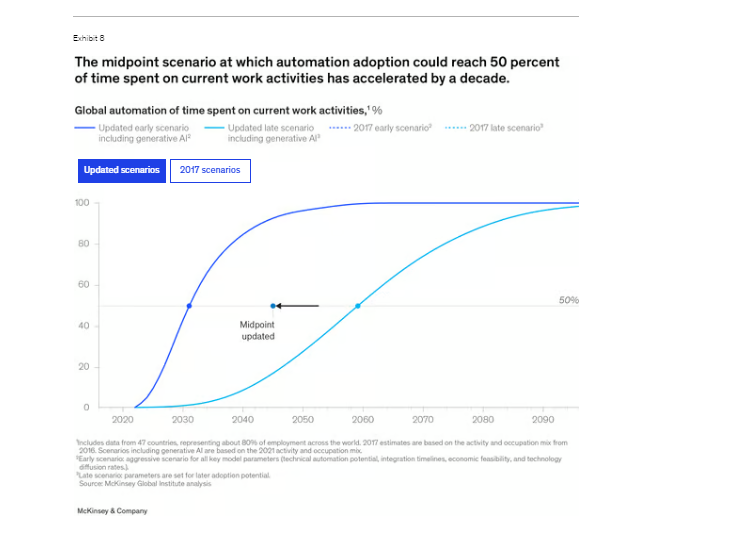

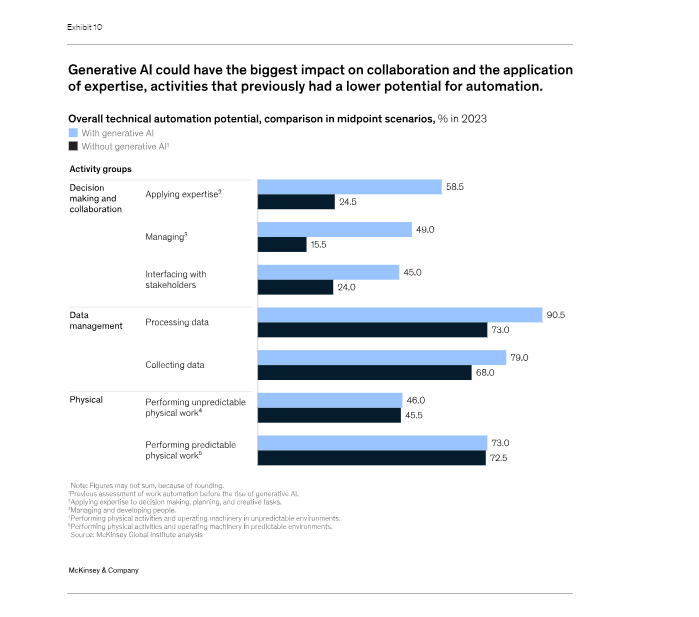
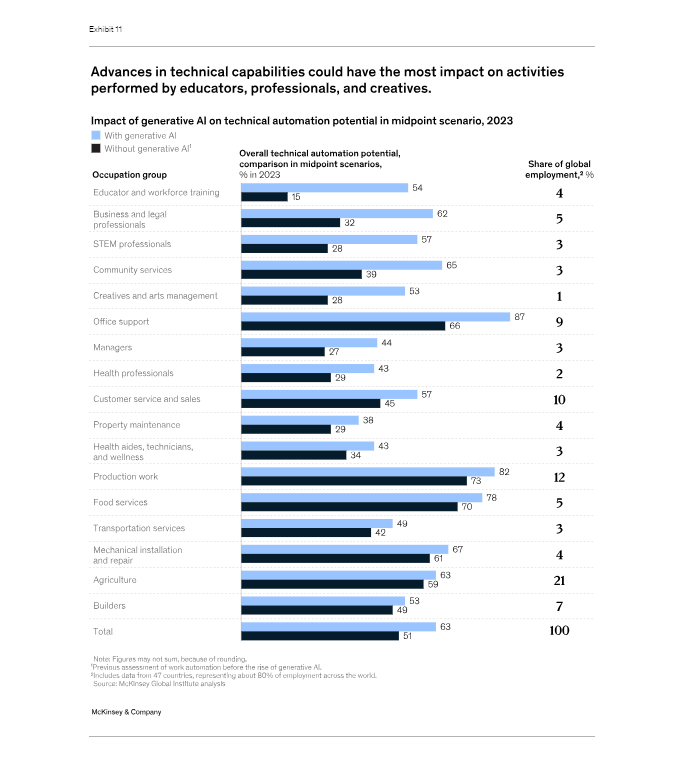
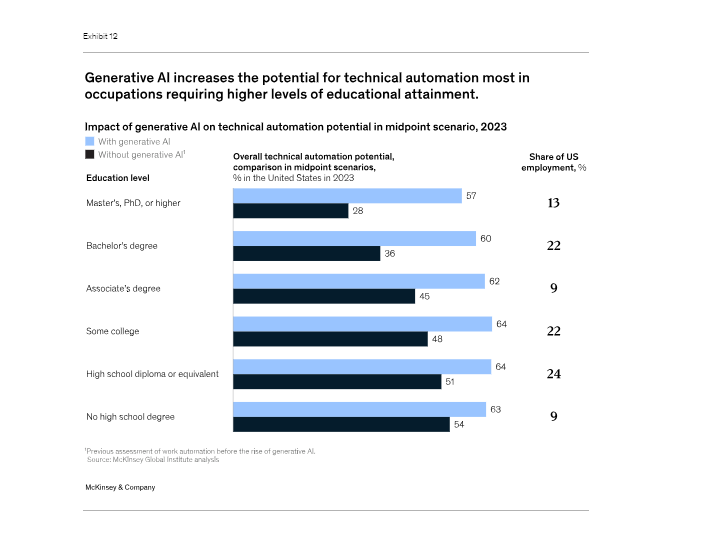
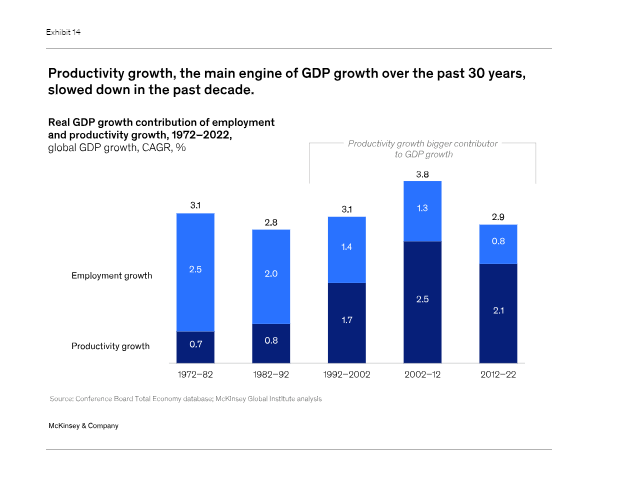
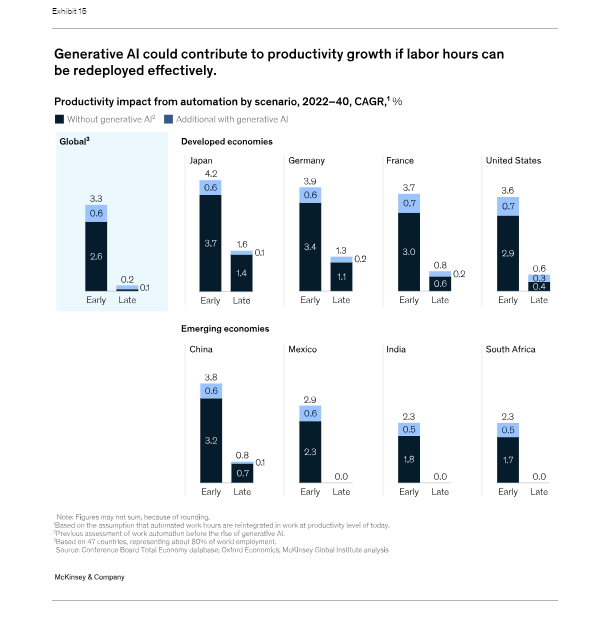
DEEP DIVE
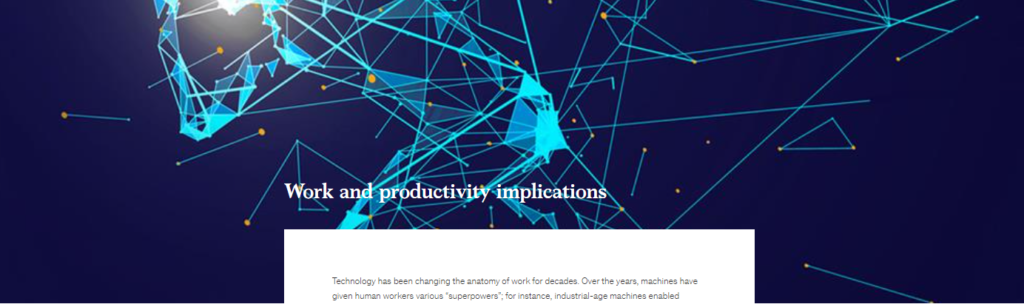
Work and productivity implications [Generative AI Reports: MGI 2023 – Part 4]
This is an excerpt of the report “The state of AI in 2023: Generative AI’s breakout year”, published by MGI (McKinsey Global Institute), authored by Michael Chui, Lareina Yee; Bryce Hall, Alex Singla and Alexander Sukharevsky, an issued on August, 2023.
MGI
Michael Chui, Eric Hazan, Roger Roberts, Alex Singla, Kate Smaje, Alex Sukharevsky, Lareina Yee, and Rodney Zemmel
June 14, 2023
Technology has been changing the anatomy of work for decades. Over the years, machines have given human workers various “superpowers”; for instance, industrial-age machines enabled workers to accomplish physical tasks beyond the capabilities of their own bodies. More recently, computers have enabled knowledge workers to perform calculations that would have taken years to do manually.
These examples illustrate how technology can augment work through the automation of individual activities that workers would have otherwise had to do themselves. At a conceptual level, the application of generative AI may follow the same pattern in the modern workplace, although as we show later in this chapter, the types of activities that generative AI could affect, and the types of occupations with activities that could change, will likely be different as a result of this technology than for older technologies.
The McKinsey Global Institute began analyzing the impact of technological automation of work activities and modeling scenarios of adoption in 2017. At that time, we estimated that workers spent half of their time on activities that had the potential to be automated by adapting technology that existed at that time, or what we call technical automation potential. We also modeled a range of potential scenarios for the pace at which these technologies could be adopted and affect work activities throughout the global economy.
Technology adoption at scale does not occur overnight. The potential of technological capabilities in a lab does not necessarily mean they can be immediately integrated into a solution that automates a specific work activity—developing such solutions takes time. Even when such a solution is developed, it might not be economically feasible to use if its costs exceed those of human labor. Additionally, even if economic incentives for deployment exist, it takes time for adoption to spread across the global economy. Hence, our adoption scenarios, which consider these factors together with the technical automation potential, provide a sense of the pace and scale at which workers’ activities could shift over time.
About the research
The analyses in this paper incorporate the potential impact of generative AI on today’s work activities. The new capabilities of generative AI, combined with previous technologies and integrated into corporate operations around the world, could accelerate the potential for technical automation of individual activities and the adoption of technologies that augment the capabilities of the workforce. They could also have an impact on knowledge workers whose activities were not expected to shift as a result of these technologies until later in the future (see sidebar “About the research”).
Automation potential has accelerated, but adoption to lag
Based on developments in generative AI, technology performance is now expected to match median human performance and reach top-quartile human performance earlier than previously estimated across a wide range of capabilities (Exhibit 6). For example, MGI previously identified 2027 as the earliest year when median human performance for natural-language understanding might be achieved in technology, but in this new analysis, the corresponding point is 2023.
As a result of these reassessments of technology capabilities due to generative AI, the total percentage of hours that could theoretically be automated by integrating technologies that exist today has increased from about 50 percent to 60–70 percent. The technical potential curve is quite steep because of the acceleration in generative AI’s natural-language capabilities.
Interestingly, the range of times between the early and late scenarios has compressed compared with the expert assessments in 2017, reflecting a greater confidence that higher levels of technological capabilities will arrive by certain time periods (Exhibit 7).
Exhibit 7
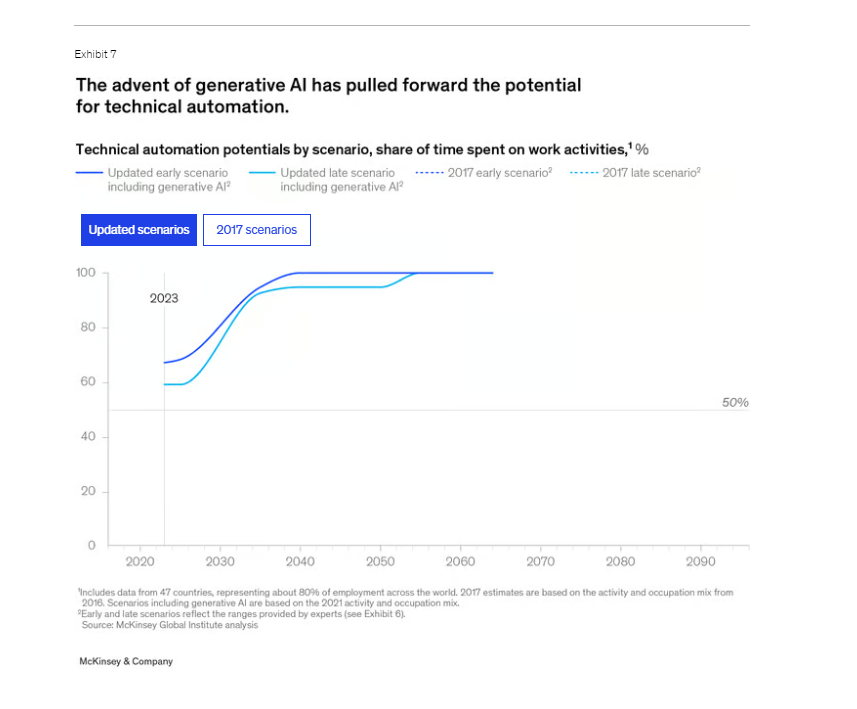
Our analysis of adoption scenarios accounts for the time required to integrate technological capabilities into solutions that can automate individual work activities; the cost of these technologies compared with that of human labor in different occupations and countries around the world; and the time it has taken for technologies to diffuse across the economy. With the acceleration in technical automation potential that generative AI enables, our scenarios for automation adoption have correspondingly accelerated. These scenarios encompass a wide range of outcomes, given that the pace at which solutions will be developed and adopted will vary based on decisions that will be made on investments, deployment, and regulation, among other factors. But they give an indication of the degree to which the activities that workers do each day may shift (Exhibit 8).
Exhibit 8
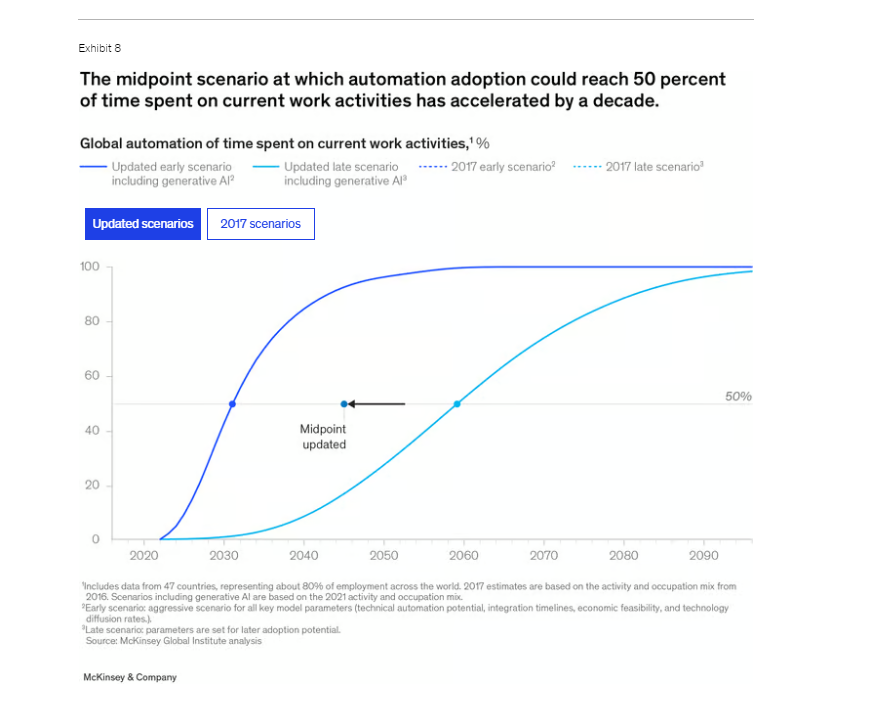
We strive to provide individuals with disabilities equal access to our website. If you would like information about this content we will be happy to work with you. Please email us at: McKinsey_Website_Accessibility@mckinsey.com
As an example of how this might play out in a specific occupation, consider postsecondary English language and literature teachers, whose detailed work activities include preparing tests and evaluating student work. With generative AI’s enhanced natural-language capabilities, more of these activities could be done by machines, perhaps initially to create a first draft that is edited by teachers but perhaps eventually with far less human editing required. This could free up time for these teachers to spend more time on other work activities, such as guiding class discussions or tutoring students who need extra assistance.
Our previously modeled adoption scenarios suggested that 50 percent of time spent on 2016 work activities would be automated sometime between 2035 and 2070, with a midpoint scenario around 2053. Our updated adoption scenarios, which account for developments in generative AI, models the time spent on 2023 work activities reaching 50 percent automation between 2030 and 2060, with a midpoint of 2045—an acceleration of roughly a decade compared with the previous estimate.6
Adoption is also likely to be faster in developed countries, where wages are higher and thus the economic feasibility of adopting automation occurs earlier. Even if the potential for technology to automate a particular work activity is high, the costs required to do so have to be compared with the cost of human wages. In countries such as China, India, and Mexico, where wage rates are lower, automation adoption is modeled to arrive more slowly than in higher-wage countries (Exhibit 9).
Exhibit 9
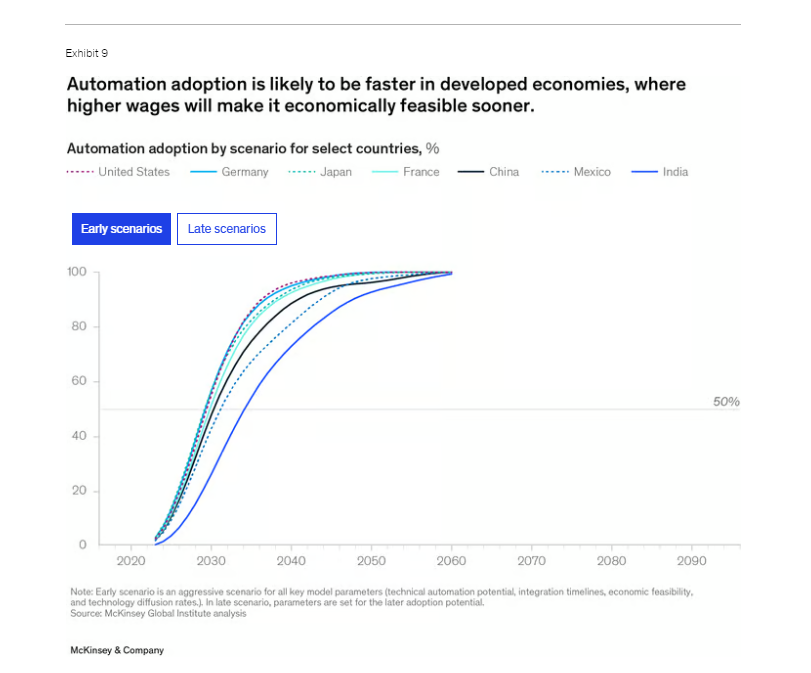
Generative AI’s potential impact on knowledge work
Previous generations of automation technology were particularly effective at automating data management tasks related to collecting and processing data. Generative AI’s natural-language capabilities increase the automation potential of these types of activities somewhat. But its impact on more physical work activities shifted much less, which isn’t surprising because its capabilities are fundamentally engineered to do cognitive tasks.
As a result, generative AI is likely to have the biggest impact on knowledge work, particularly activities involving decision making and collaboration, which previously had the lowest potential for automation (Exhibit 10). Our estimate of the technical potential to automate the application of expertise jumped 34 percentage points, while the potential to automate management and develop talent increased from 16 percent in 2017 to 49 percent in 2023.
Exhibit 10
Generative AI’s ability to understand and use natural language for a variety of activities and tasks largely explains why automation potential has risen so steeply. Some 40 percent of the activities that workers perform in the economy require at least a median level of human understanding of natural language.
As a result, many of the work activities that involve communication, supervision, documentation, and interacting with people in general have the potential to be automated by generative AI, accelerating the transformation of work in occupations such as education and technology, for which automation potential was previously expected to emerge later (Exhibit 11).
Exhibit 11
Labor economists have often noted that the deployment of automation technologies tends to have the most impact on workers with the lowest skill levels, as measured by educational attainment, or what is called skill biased. We find that generative AI has the opposite pattern—it is likely to have the most incremental impact through automating some of the activities of more-educated workers (Exhibit 12).
Exhibit 12
Another way to interpret this result is that generative AI will challenge the attainment of multiyear degree credentials as an indicator of skills, and others have advocated for taking a more skills-based approach to workforce development in order to create more equitable, efficient workforce training and matching systems.7 Generative AI could still be described as skill-biased technological change, but with a different, perhaps more granular, description of skills that are more likely to be replaced than complemented by the activities that machines can do.
Previous generations of automation technology often had the most impact on occupations with wages falling in the middle of the income distribution. For lower-wage occupations, making a case for work automation is more difficult because the potential benefits of automation compete against a lower cost of human labor. Additionally, some of the tasks performed in lower-wage occupations are technically difficult to automate—for example, manipulating fabric or picking delicate fruits. Some labor economists have observed a “hollowing out of the middle,” and our previous models have suggested that work automation would likely have the biggest midterm impact on lower-middle-income quintiles.
However, generative AI’s impact is likely to most transform the work of higher-wage knowledge workers because of advances in the technical automation potential of their activities, which were previously considered to be relatively immune from automation (Exhibit 13).
Exhibit 13
Generative AI could propel higher productivity growth
Global economic growth was slower from 2012 to 2022 than in the two preceding decades.8 Although the COVID-19 pandemic was a significant factor, long-term structural challenges—including declining birth rates and aging populations—are ongoing obstacles to growth.
Declining employment is among those obstacles. Compound annual growth in the total number of workers worldwide slowed from 2.5 percent in 1972–82 to just 0.8 percent in 2012–22, largely because of aging. In many large countries, the size of the workforce is already declining.9 Productivity, which measures output relative to input, or the value of goods and services produced divided by the amount of labor, capital, and other resources required to produce them, was the main engine of economic growth in the three decades from 1992 to 2022 (Exhibit 14). However, since then, productivity growth has slowed in tandem with slowing employment growth, confounding economists and policy makers.10
Exhibit 14
The deployment of generative AI and other technologies could help accelerate productivity growth, partially compensating for declining employment growth and enabling overall economic growth. Based on our estimates, the automation of individual work activities enabled by these technologies could provide the global economy with an annual productivity boost of 0.2 to 3.3 percent from 2023 to 2040, depending on the rate of automation adoption—with generative AI contributing 0.1 to 0.6 percentage points of that growth—but only if individuals affected by the technology were to shift to other work activities that at least match their 2022 productivity levels (Exhibit 15). In some cases, workers will stay in the same occupations, but their mix of activities will shift; in others, workers will need to shift occupations.
Exhibit 15
Originally published at https://www.mckinsey.com/capabilities/mckinsey-digital