the health strategist . institute
for continuous health transformation & digital health
Joaquim Cardoso MSc
Chief Research & Strategy Officer (CRSO),
Chief Editor and Senior Advisor
August 24, 2023
What is the message?
The introduction and explanation of Federated Machine Learning (FedML) as a solution to overcome the disadvantage that smaller companies and those with limited access to big data face in the field of artificial intelligence (AI) and machine learning (ML).
Major advancements in AI and ML have often been driven by large tech companies with extensive access to vast amounts of data. These companies are able to develop sophisticated AI models due to the sheer volume of data they can utilize.
In contrast, smaller companies and those in traditional industries often lack sufficient data to train powerful AI models, putting them at a significant disadvantage.
The concept of FedML is introduced as a solution to this problem. FedML enables smaller companies and organizations to collaborate and share their decentralized data while ensuring data privacy.
This collaborative approach allows them to collectively build AI models without directly sharing their raw data. This way, each participant can contribute their unique data without compromising sensitive information.
FedML has the potential to level the playing field by enabling a broader range of companies, including those without access to massive datasets, to benefit from AI and ML technologies.
The passage implies that this approach could bridge the “digital divide” that currently exists between companies with abundant data resources and those without.
Overall, the central message underscores how FedML presents a solution to the AI scale disadvantage faced by smaller companies, enabling them to harness the power of AI and ML through collaborative and privacy-preserving methods.
ORIGINAL PUBLICATION [excerpt]
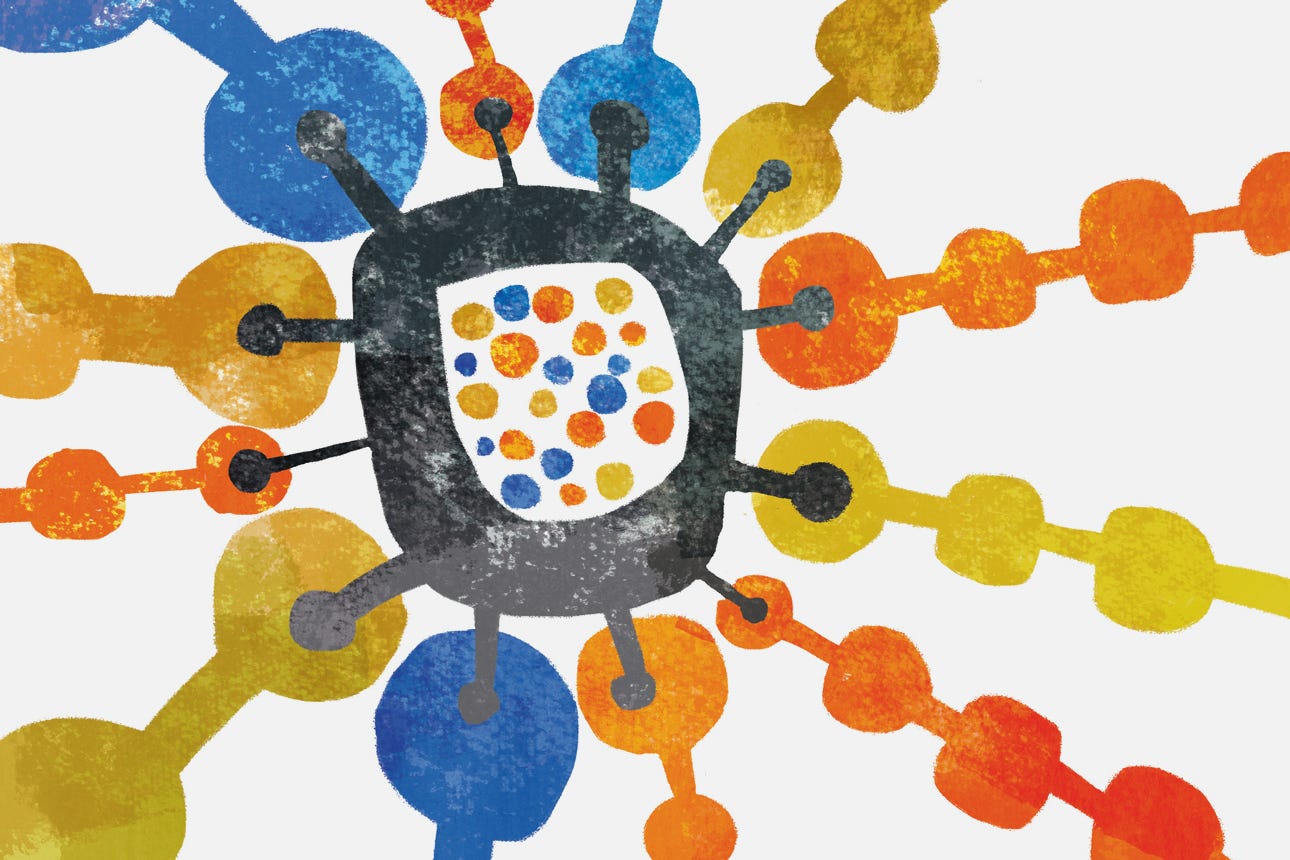
Using Federated Machine Learning to Overcome the AI Scale Disadvantage
A promising new approach to training AI models lets companies with small data sets collaborate while safeguarding proprietary information.
MIT Sloan Management Review
Yannick Bammens and Paul Hünermund
August 22, 2023
Deep pockets, access to talent, and massive investments in computing infrastructure only partly explain why most major breakthroughs in artificial intelligence have come from a select group of Big Tech companies that includes Amazon, Google, and Microsoft.
What sets the tech giants apart from the many other businesses seeking to gain an edge from AI are the vast amounts of data they collect as platform operators.
Amazon alone processes millions of transactions each month on its platform. All of that big data is a rich strategic resource that can be used to develop and train complex machine learning algorithms — but it’s a resource that is out of reach for most enterprises.
Access to big data allows for more sophisticated and better-performing AI and machine learning models, but many companies must make do with much smaller data sets.
For smaller companies and those operating in traditional sectors like health care, manufacturing, or construction, a lack of data is the biggest impediment to venturing into AI.
For smaller companies and those operating in traditional sectors like health care, manufacturing, or construction, a lack of data is the biggest impediment to venturing into AI.
The digital divide between big and small-data organizations is a serious concern due to self-reinforcing data network effects, where more data leads to better AI tools, which help attract more customers who generate more data, and so forth. 1
This gives bigger companies a strong competitive AI advantage, with small and midsize organizations struggling to keep up.
The idea of multiple small-scale companies pooling their data in a jointly controlled central repository has been around for a while, but concerns about data privacy may quash such initiatives. 2
Federated machine learning (FedML) is a recent innovative technology that overcomes this problem by means of privacy-preserving collaborative AI that uses decentralized data.
FedML might turn out to be a game changer in addressing the digital divide between companies with and without big data and enabling a larger part of the economy to reap the benefits of AI.
It’s a technology that doesn’t just sound promising in theory — it has already been successfully implemented in industry, as we’ll detail below. But first, we’ll explain how it works.
To continue reading …
Originally published at https://sloanreview.mit.edu on August 22, 2023.