the health strategist
institute for strategic health transformation
& digital technology
Joaquim Cardoso MSc.
Chief Research and Strategy Officer (CRSO),
Chief Editor and Senior Advisor
October 10, 2023
One page summary
What is the message?
Radiology is at a crossroads as it grapples with the integration of artificial intelligence (AI) into its practice.
While AI holds immense potential to enhance diagnostic processes and improve patient care, its adoption faces challenges due to differing perspectives on its value and impact within the field of radiology.

Key Takeaways
Differing Views on AI Value:
AI is perceived differently by various stakeholders in the medical field. For hospital executives and AI vendors, AI represents a promising return on investment and revenue generation. However, radiologists, the end users of AI, view it as a potential productivity-enhancing tool, which raises concerns as they are already struggling with high imaging volumes.
Productivity Quagmire:
Radiologists are caught in a productivity dilemma where their performance is assessed based on how many studies they read. AI’s promise of increased productivity may exacerbate their workload, potentially leading to burnout and increased stress.
AI as a Diagnostic Aide:
AI has the potential to assist radiologists in detecting subtle findings and reducing diagnostic errors. However, radiologists may be cautious about AI’s ability to balance sensitivity and specificity, which is crucial in diagnostic accuracy.
The Pitfall of False Positives:
AI’s sensitivity could lead to an increased number of false-positive results, potentially distracting radiologists from true-positive findings and subjecting patients to unnecessary interventions. This could affect radiology’s diagnostic precision.
AI Replacing Radiologists in Selected Tasks:
AI might be used to identify normal study results, allowing radiologists to focus on abnormal cases. However, contextualizing “normal” results with patient symptoms and understanding the broad spectrum of normalcy in medical images is a complex task.
Radiologists’ Shift in Role:
Radiologists may need to evolve from merely generating reports to actively managing diagnostic pathways and patient care. AI can assist by automating certain aspects of the radiologist’s role and allowing them to focus on information management and system coordination.
AI Enabling an Expanded Scope for Radiology:
Automation through AI can create new roles for radiologists, such as population health management and screening coordination. Radiologists may need to adapt to AI technology and delegate tasks to algorithms to harness its full potential.
Challenges of AI Adoption:
In high-income countries like the United States, the incentives for radiologists to adopt AI may not be as compelling due to their established productivity-focused culture. Value-based payment models and shifting healthcare paradigms are expected to encourage greater AI adoption in radiology.
AI’s Ongoing Integration:
AI is already making strides in radiology, from image reconstruction to report generation. As AI matures, it will empower radiologists to perform at the highest level of their capabilities, emphasizing collaboration between human expertise and AI technology.
In conclusion
The integration of AI into radiology presents both challenges and opportunities. Radiologists must navigate the evolving landscape, recognizing the potential of AI to enhance their work and embracing the need for adaptation and collaboration in this dynamic field.
DEEP DIVE

Algorithms at the Gate—Radiology’s AI Adoption Dilemma
JAMA Network
Saurabh Jha, MBBS, MS
October 6, 2023
ChatGPT, an artificial intelligence (AI) chatbot trained on large language models, whose feats include generating radiology reports in the literary style of Emily Brontë, has revived dystopias of AI. Radiologists are accustomed to AI-mediated extinction prophesies. The father of deep learning, Geoffrey Hinton, once advised against training radiologists because he believed that AI would soon replace them.1 Seven years after Hinton’s prophesy, far from extinction, a radiologist shortage exists.2 To understand the adoption trajectory of AI-enabled diagnosis from medical images, it is important to understand the structure of radiology and the incentives radiologists face.
AI and Productivity in Radiology
The value of AI is viewed differently by different stakeholders. Artificial intelligence vendors appealing to hospital executives make the business case for AI: a return on investment, which is invariably revenue generation. Industry sells AI as a productivity-enhancing tool, which is singularly unappealing to radiologists, AI’s end users, who already are at their wits’ end with imaging volumes. However, because of the reimbursement structure, productivity is the lifeblood of radiology. Radiologists are judged and rewarded for how many studies they read. They are in a productivity quagmire: productivity sustains them and productivity ails them.
Three trends have coalesced to shape radiology. Radiologists have become more efficient because of faster integrated reading systems. They view more images, largely from thin-section computed tomography. And the reimbursement per study has declined. The promise of AI’s increasing productivity could sink radiologists deeper into the productivity quagmire.
With efficiency gains from AI, radiologists can read either more studies in the same time or the same number of studies in less time. With the latter, AI could theoretically enable a more pleasant work experience. In practice this is unlikely. Although the link between productivity pressure and burnout seems clear,3 it is far from certain that marginal gains, such as finishing work an hour earlier, will spiritually rejuvenate radiologists. Instead, efficiency gains might extend the dominance of a smaller labor force: fewer radiologists working more efficiently but just as intensely, and similarly predisposed to burnout.
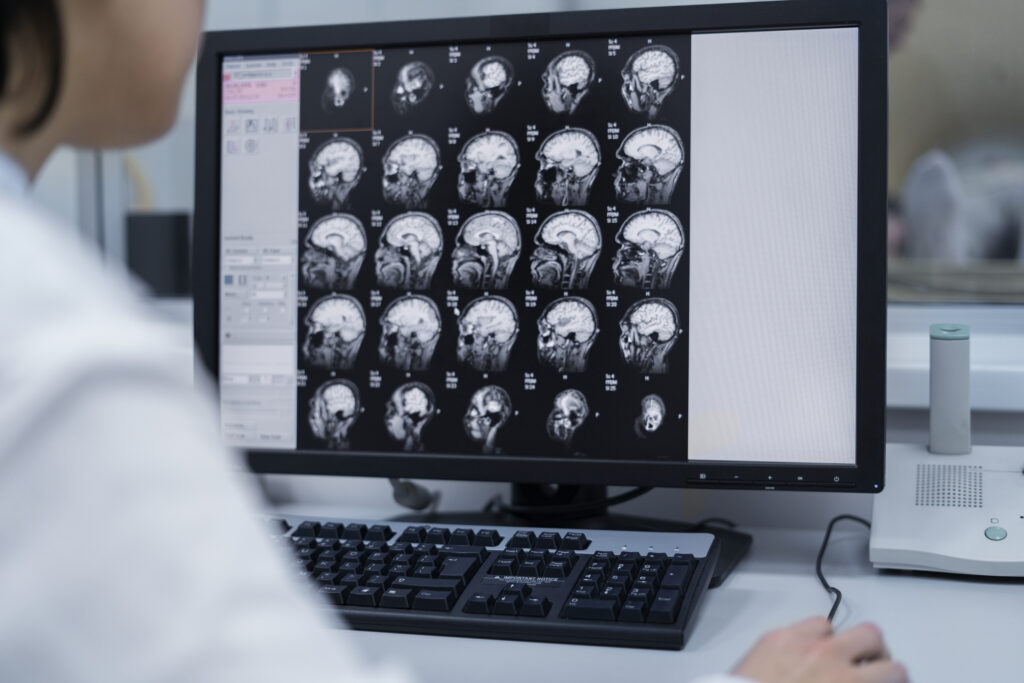
AI as a Diagnostic Aide
Artificial intelligence could be radiologists’ aide, pointing out subtle findings they might miss, such as small pulmonary emboli. Reducing errors is a compelling case for AI use. However, radiologists may be skeptical, aware of the stubbornness of the receiver operating characteristic (ROC) curve where gains in sensitivity often penalize specificity. There is no free ROC lunch. False-positive results overwhelm both radiologists by distracting them from true-positive results and patients by subjecting them to unnecessary interventions. Furthermore, sensitive technologies may overdiagnose pathology (ie, find clinically irrelevant diseases).4
Computer-aided detection for reading mammograms is instructive.5 In an attempt to be more sensitive and “never miss cancer,” it lost specificity and became the proverbial boy who cried wolf. Widespread adoption of AI may change how radiologists interpret images. Inexperienced radiologists in particular may be unable to ignore AI, succumbing to an avalanche of false-positive results.6
Whether AI augments radiologists’ performance or radiologists regress to AI’s level remains speculative. What is not speculative is that an extra pair of eyes, with both AI and radiologists checking each other’s work, may not reduce the net labor conscripted to extract meaningful clinical information from images. Over time, radiologists may reflexively note the possibility that AI will detect something that they will not and may make radiologists, who infamously hedge, hedge even more. Known as the Solow paradox, development in information technology has historically often slowed productivity.
AI Replacing Radiologists in Selected Tasks?
Another use for AI is reading normal study results, decanting the abnormal ones for radiologists. Because many study results are normal, AI could reduce radiologists’ workload. This sounds appealing but only superficially. For one, “normal” must be contextualized with symptoms because a normal study result does not mean the patient does not have an illness. To detect “abnormal,” radiologists must know normal. In fact, “normal” is arguably the most difficult diagnosis to make. On imaging, “normal” has a broad and variegated coastline. The heaviest radiology book I own is Atlas of Normal Roentgen Variants Which May Simulate Disease, which I still have not finished reading.
Just because radiologists may be reluctant to adopt AI does not mean other clinicians will not find AI useful. For instance, as soon as the result of computed tomography has been acquired, AI alerts neurologists about patients with stroke potentially amenable to thrombolysis, often with the patient still on the scanner and sometimes before the radiologist has viewed the images. Promptly informed, neurologists can commence therapy sooner, and because “time is brain,” patients may have better outcomes.7 Removed from the loop, radiologists are left cleaning up the false-positive results and incidental findings.
Radiologists have focused excessively on radiology reports, considering them the sole distillates of their expertise. Nothing is more sacrosanct for the profession than the turnaround time of reports. The report is only 1 part of imaging’s role in care systems. For example, positive imaging findings, such as coronary atherosclerosis, should induce other steps, such as ensuring the patient is taking an optimal dose of statins and directing the patient to the appropriate physician. By interrogating the electronic health record, AI can theoretically do this seamlessly. If the profession changes from being charged with just report generation to system management as well, in which the radiologist’s job is as much activating the next step in the diagnostic pathways as it is deciding whether a study finding is positive or negative, radiologists may find AI more useful.

AI Enabling an Expanded Scope for Radiology
Artificial intelligence enables automation; that is, it reduces the labor required to achieve tasks. Since the industrial revolution, labor and automation have coexisted, and it is labor that has adapted to technology. Automation has encouraged labor to imagine new roles, and labor has often benefitted from the automation. For example, automated teller machines, far from eliminating bank tellers, increased their numbers by growing the demand for banks.8
Radiologists are familiar with automation. Formerly, they were at the scanners guiding image acquisition. As specialized knowledge became more available and technology improved, acquisition was automated, and radiologists became involved less in acquisition and more in imaging interpretation. The automation from AI may displace radiologists once again, this time from imaging interpretation to novel roles such as information management. For instance, AI could scale radiology’s influence in population health, such as lung cancer screening, in which the radiologist’s job will not be reporting as many results of computed tomography as rapidly as possible, but in coordinating screening, leaving AI to seek lung nodules. To harness AI’s full potential, radiologists must relinquish some of their work to algorithms and reimagine the human nonautomatable components of their work.
In low- to middle-income countries, AI detects pathologies such as tuberculosis9 not only because it can but also because radiologists are scarce. The incentives to adopt AI by overoptimized US radiologists are not as compelling but may change with value-based payments, and whatever value will mean, new payment models are certain to extricate radiologists to some degree from the productivity quagmire, changing culture and encouraging adoption.
It took more than a decade for magnetic resonance imaging to travel from research to clinical practice; AI, which is still young, is already being used in the radiology value chain, from image reconstruction to report generation.10 Eventually, AI will allow radiologists to perform at the top of their license.
Originally published at https://jamanetwork.com/journals/jama