the health
transformation
knowledge portal
Joaquim Cardoso MSc
Founder and Chief Researcher, Editor & Strategist
March 27, 2024
What are the key points?
Magnitude of Diagnostic Errors: The article highlights a significant issue in the medical field, with studies estimating that nearly 800,000 Americans suffer from permanent disability or death due to diagnostic errors each year.
Nature of Diagnostic Errors: Diagnostic errors encompass inaccurate assessments of patients’ illnesses, ranging from missing critical conditions like heart attacks to misdiagnosing common ailments.
Persistent Problem: Despite the increasing use of medical imaging and laboratory tests, there has been no apparent improvement in diagnostic accuracy since 2015, as reported by the National Academies of Sciences, Engineering, and Medicine.
Contributing Factors: One of the primary reasons behind diagnostic errors is the lack of time for thorough evaluation during clinic visits, often leading to reliance on automatic, intuitive thinking (System 1 thinking) rather than analytical thinking (System 2 thinking).
Role of AI in Improving Diagnostic Accuracy: The article discusses the potential of artificial intelligence (AI) to enhance diagnostic accuracy. Studies have shown that AI, particularly deep learning models, can aid in interpreting medical images and identifying anomalies, resulting in improved accuracy and reduced workload for clinicians.
Examples of AI Applications: The article presents examples of AI applications in medical diagnosis, including the use of language models like ChatGPT to assist in diagnosing rare conditions and improving diagnostic accuracy compared to experienced physicians.
Challenges and Concerns: Despite the promise of AI, there are challenges related to bias propagation and automation bias, where clinicians may overly rely on AI predictions or be influenced by biased AI models.
Future Outlook: While acknowledging the potential of AI to enhance diagnostic accuracy, the article emphasizes the need to address biases and ensure AI models are continually updated with the latest medical knowledge. The ultimate goal is to leverage AI as a tool for providing second opinions and reducing diagnostic errors in medicine.

This article was published by Ground Truths and written by Eric Topol on January 28, 2024.
The medical community does not broadcast the problem, but there are many studies that have reinforced a serious issue with diagnostic errors. A recent study concluded: “We estimate that nearly 800,000 Americans die or are permanently disabled by diagnostic errors each year.”
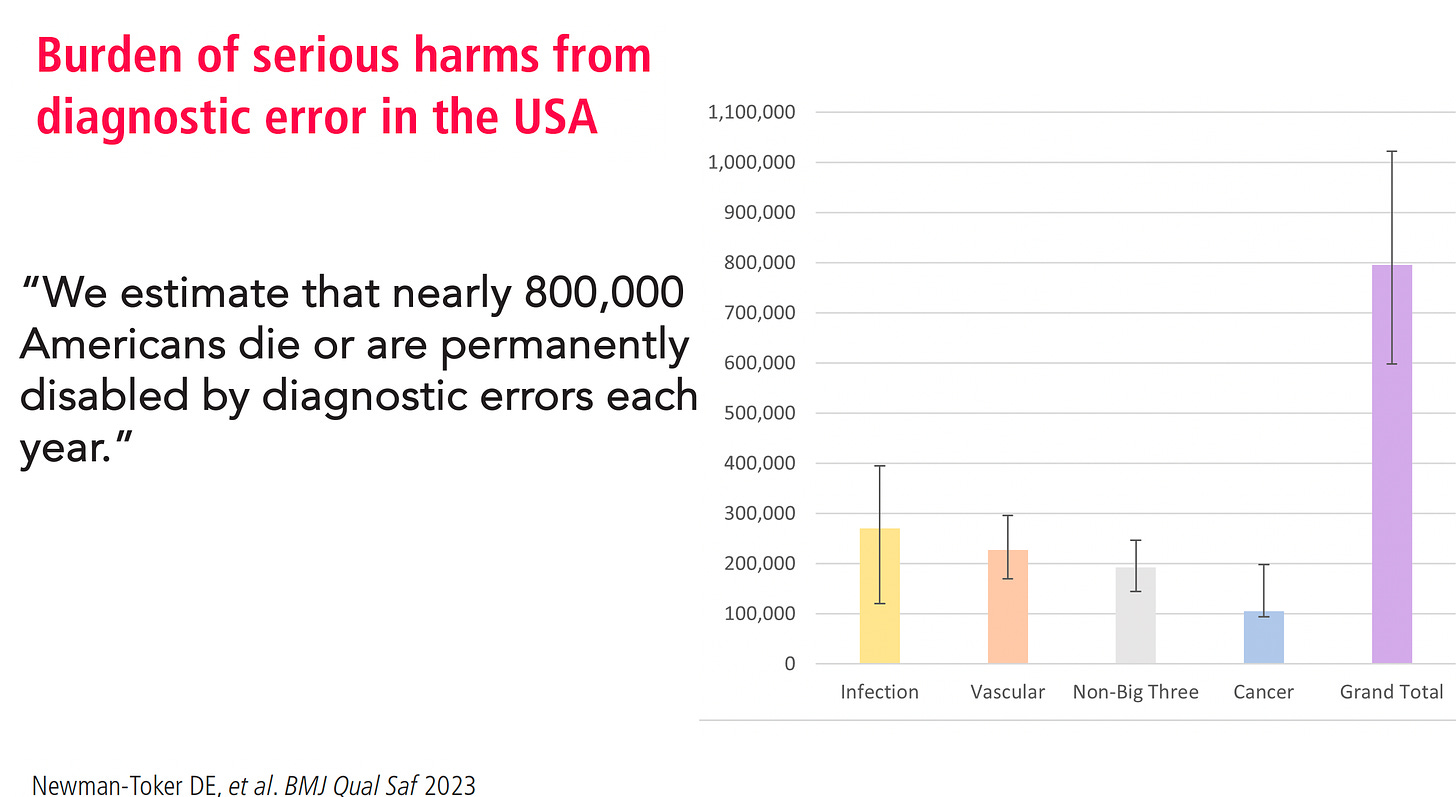
Diagnostic errors are inaccurate assessments of a patient’s root cause of illness, such as missing a heart attack or infection or assigning the wrong diagnosis of pneumonia when the correct one is pulmonary embolism.
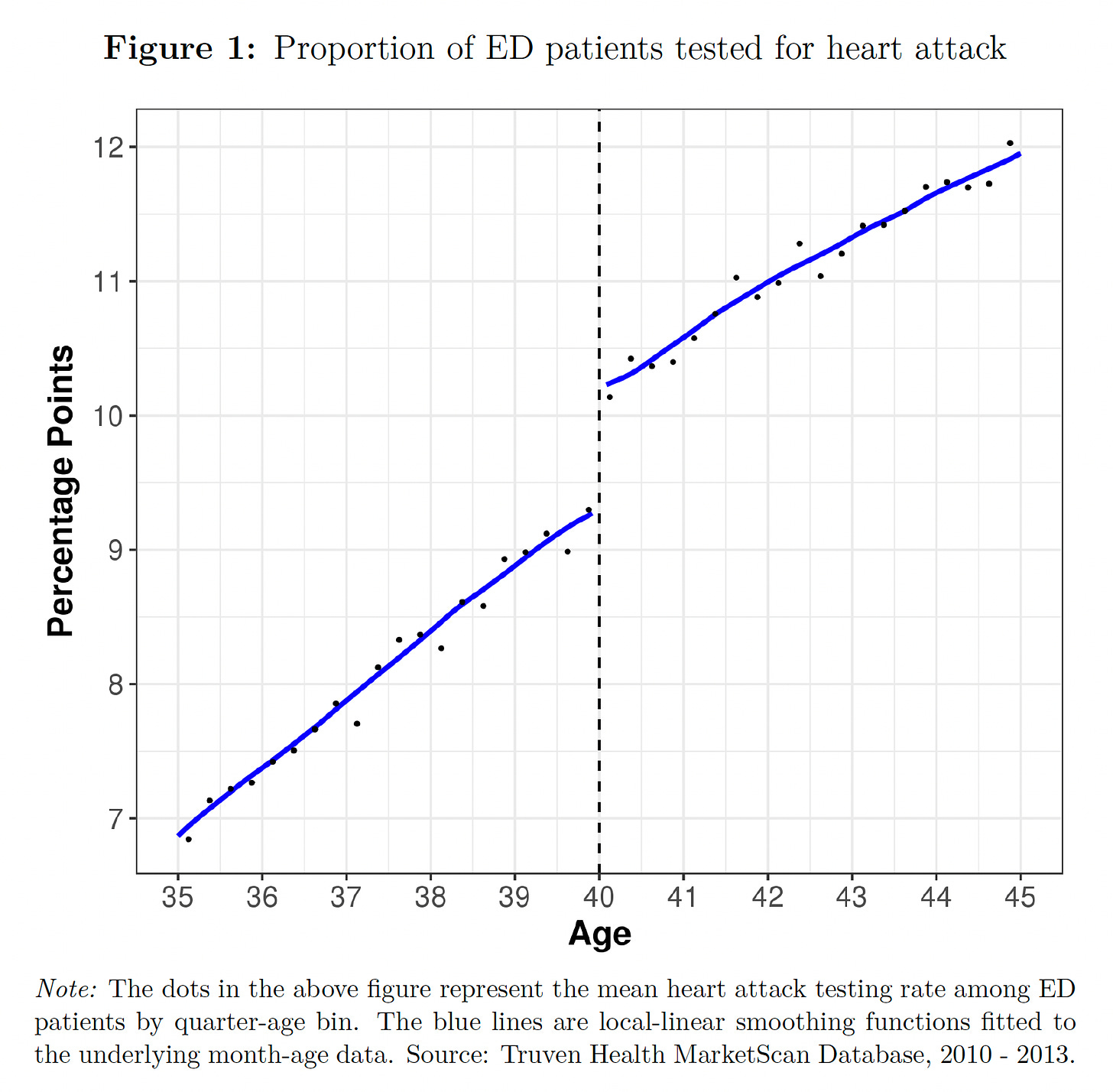
Despite ever-increasing use of medical imaging and laboratory tests intended to promote diagnostic accuracy, there is nothing to suggest improvement since the report by the National Academies of Sciences, Engineering and Medicine in 2015, which provided a conservative estimate that 5% of adults experience a diagnostic error each year, and that most people will experience at least one in their lifetime.
One of the important reasons for these errors is failure to consider the diagnosis when evaluating the patient. With the brief duration of a clinic visit, it is not surprising that there is little time to reflect, because it relies on System 1 thinking, which is automatic, near-instantaneous, reflexive, and intuitive. If physicians had more time to think, to do a search or review the literature, and analyze all of the patient’s data (System 2 thinking), it is possible that diagnostic errors could be reduced.
There are a few ways that artificial intelligence (AI) is emerging to make a difference to diagnostic accuracy. In the era of supervised deep learning with convolutional neural networks trained to interpret medical images, there have been numerous studies that show accuracy may be improved with AI support beyond expert clinicians working on their own. A large randomized study of mammography in more than 80,000 women being screened for breast cancer, with or without AI support to radiologists, showed improvement in accuracy with a considerable 44% reduction of screen-reading workload.
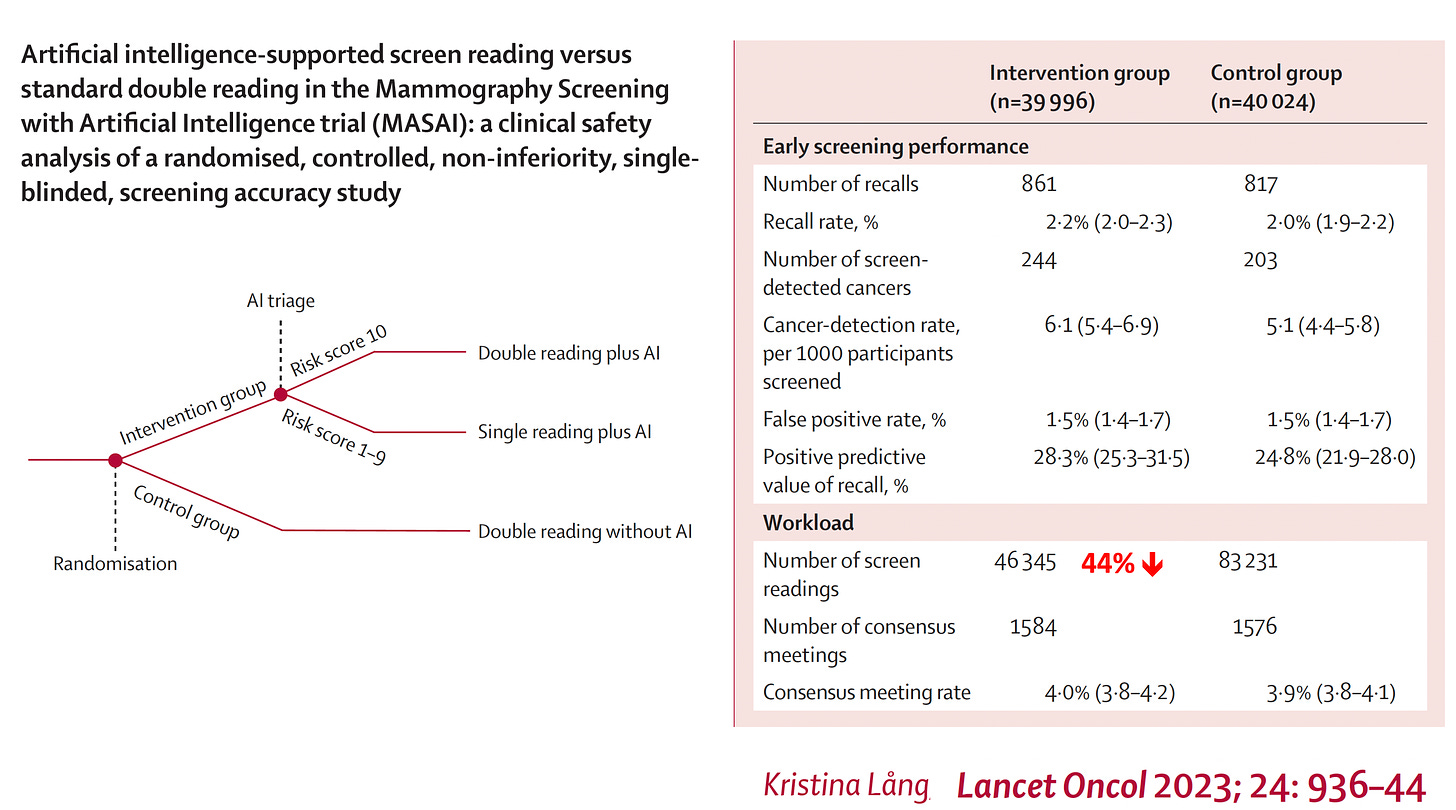
A systematic analysis of 33 randomized trials of colonoscopy, with or without real-time AI machine vision, indicated there was more than a 50% reduction in missing polyps and adenomas, and the inspection time added by AI to achieve this enhanced accuracy averaged only 20 s.
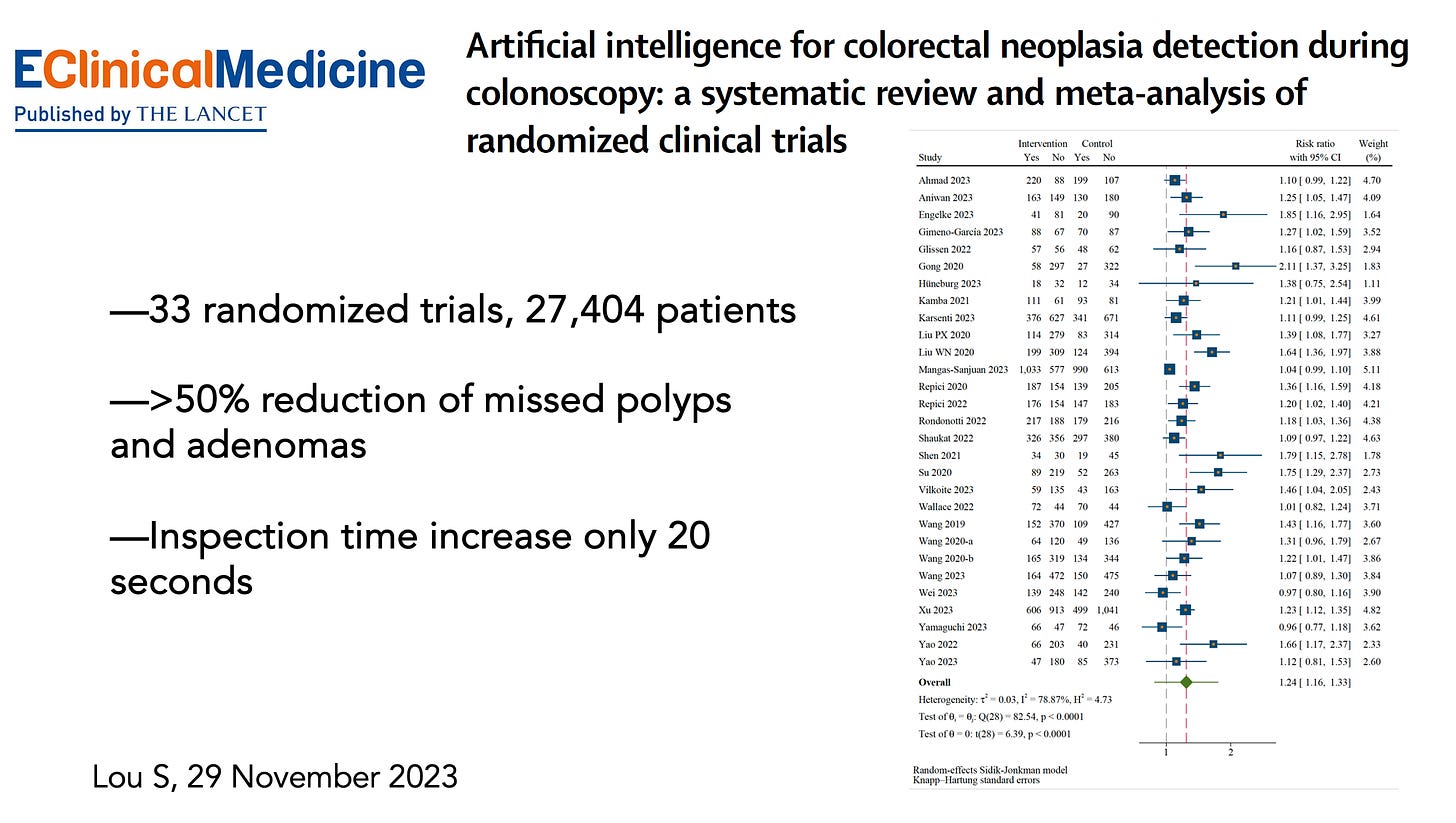
Those studies used unimodal, image-based, deep neural network models. Now, with the progress that has been made with transformer models, enabling multimodal inputs, there is expanded potential for generative AI to facilitate medical diagnostic accuracy. That equates to a capability to input all of an individual’s data, including electronic health records with unstructured text, image files, lab results, and more. Not long after the release of ChatGPT, anecdotes appeared for its potential to resolve elusive, missed diagnoses. For example, a young boy with severe, increasing pain, headaches, abnormal gait, and growth arrest resulted in his being evaluated by 17 doctors over 3 years without a diagnosis. The correct diagnosis of occult spina bifida was ultimately made when his mother put his symptoms into ChatGPT, which led to neurosurgery to untether his spinal cord and marked improvement. Similarly, a woman saw several primary care physicians and neurologists and was assigned a diagnosis of Long Covid for which there is no validated treatment. But her relative entered her symptoms and lab tests into ChatGPT and got the diagnosis of limbic encephalitis, which was subsequently confirmed by antibody testing, and for which there is a known treatment (intravenous immunoglobulin) that was used successfully.
Such anecdotal cases will not change how medicine is practiced, and may be skewed for positive results, with misdiagnoses by ChatGPT less likely to receive attention. Alternatively, how about using Case Records of the Massachusetts General Hospital, which involve complex diagnostic challenges presented to master clinicians, have a 100-year lineage, and are published biweekly in the New England Journal of Medicine as clinicopathological conferences (CPCs)? This was the focus of a recent randomized study published in preprint form. The objective was to come up with a differential diagnosis, which included the correct diagnosis, for over 300 CPCs, comparing performance by 20 experienced internal medicine physicians (average time in medical practice of 9 years) with that of a large language model (LLM).
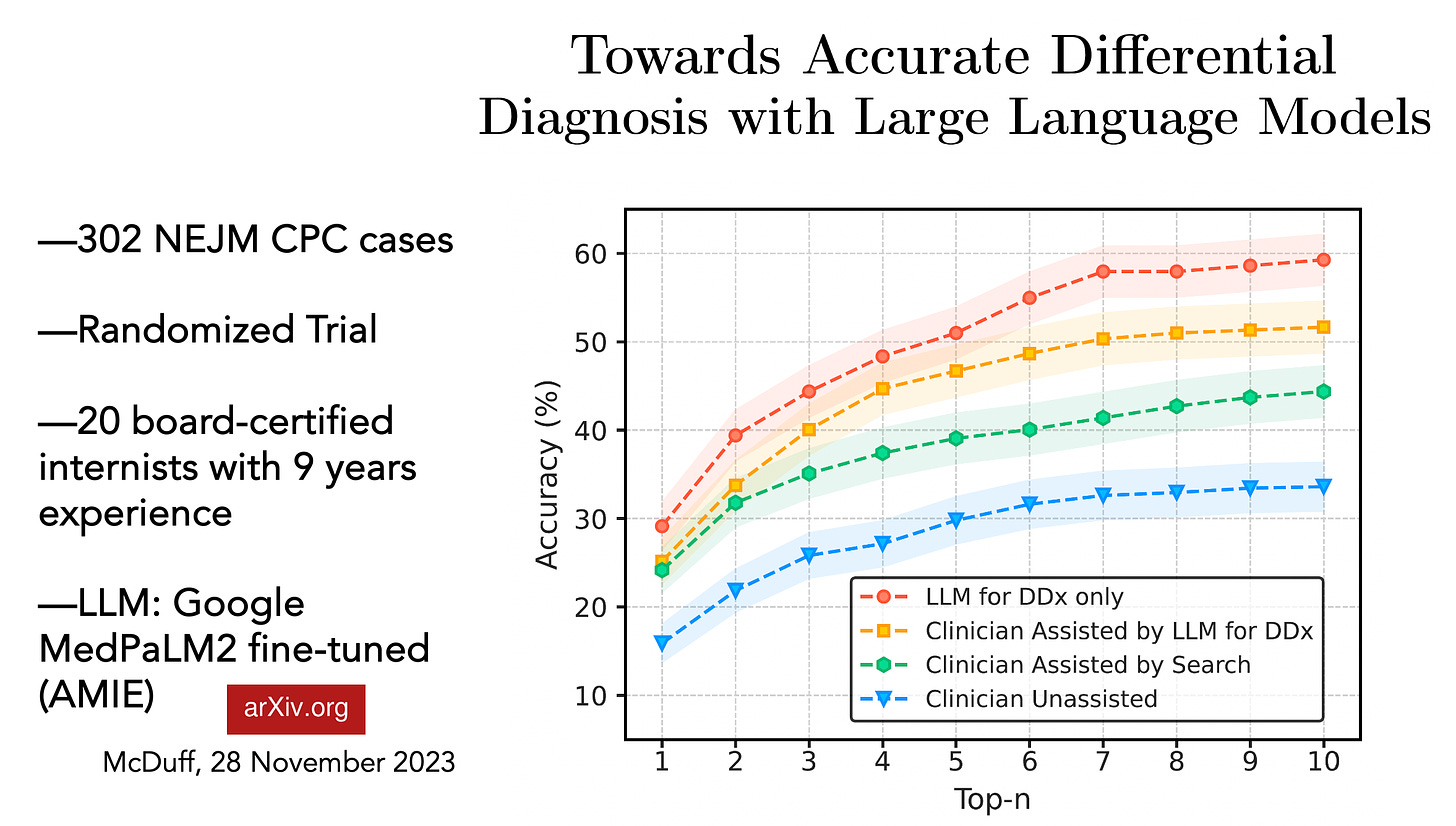
The LLM was nearly twice as accurate as physicians for accuracy of diagnosis, 59.1 versus 33.6%, respectively. Physicians exhibited improvement when they used a search, and even more so with access to the LLM. This work confirmed and extended prior LLM comparison with physicians for diagnostic accuracy, including a preprint study of 69 CPCs using GPT-4V and publication of a study evaluating 70 CPCs with GPT-4. But CPCs are extremely difficult diagnostic cases, and not generally representative of medical practice. They may, however, be a useful indicator for correct diagnosis of rare conditions, such as has been seen with rare diseases (preprint) and rare eye conditions using GPT-4.
A new preprint report by Google DeepMind researchers took this another step further. Using 20 patient actors to present (by text) 149 cases to 20 primary care physicians, with a randomized design, the LLM (Articulate Medical Intelligence, AMIE) was found to be superior for 24 of 26 outcomes assessed, which included diagnostic accuracy, communication, empathy, and management plan.
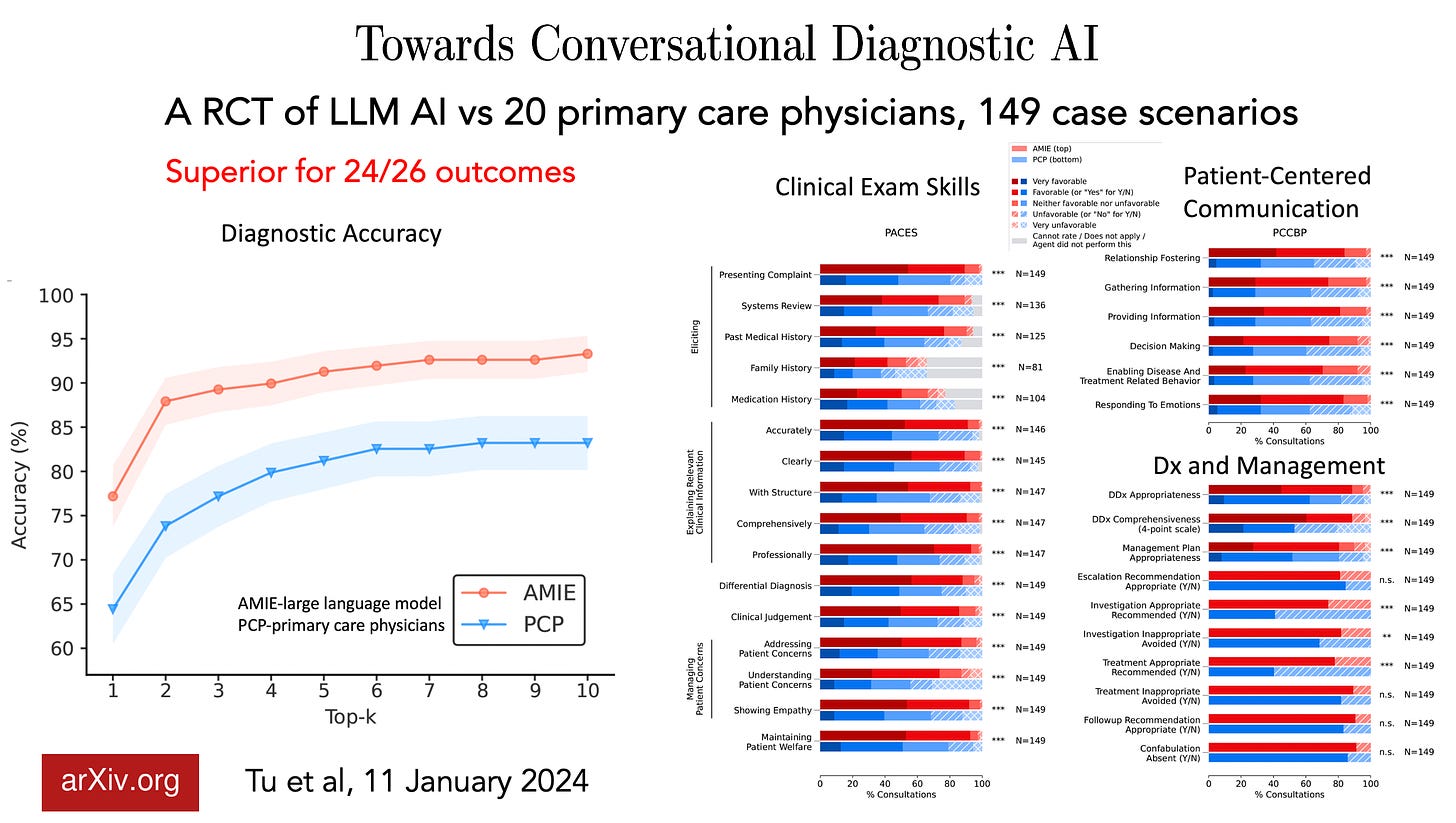
An alternative approach has been to use medical case vignettes for common conditions in hospitalized patients. This was carried out using a randomized design, to determine whether a patient had pneumonia, heart failure, or chronic obstructive pulmonary disease. Use of a standard AI model (not an LLM) improved diagnostic accuracy. However, some of the vignettes purposely used systematically biased models, such as giving higher diagnostic probability for pneumonia based on advanced age, which led to marked reduction in accuracy that was not mitigated by providing model explainability to the clinician. This finding raised the issue of automation bias, erroneously placing trust in the AI, with doctors’ willingness to accept the model’s diagnosis. Another study using clinical vignettes comparing clinicians with GPT-4 found the LLM to exhibit systematic signs of age, race, and gender bias.
Notably, the bias of physicians toward AI can go in both directions. A recent randomized study of 180 radiologists, with or without a convolutional neural network support, gauged the accuracy for interpreting chest x-rays. Although the AI outperformed the radiologists for the overall analysis, there was evidence of marked heterogeneity, with some radiologists exhibiting “automation neglect,” highly confident of their own reading and discounting the AI interpretations.
In aggregate, the evidence to date suggests that there is real potential for generative AI to improve the accuracy of medical diagnoses, but the concerns for propagating bias need to be addressed. Well before the adjunctive use of AI was considered, there was ample evidence that physician biases contributed to medical diagnostic errors, such as misdiagnosis of heart attacks in the emergency room in people younger than 40 years of age. Base models such as GPT-4, Llama2, and most recently, Gemini, train with these human content biases, and few if any LLMs have specialized fine-tuning for improving medical diagnoses, no less the corpus of up-to-date medical knowledge. It is easy to forget that no physician can possibly keep up with all the medical literature on the approximately 10,000 types of human disease.
When I spoke to Geoffrey Hinton recently about the prospects for AI to improve accuracy in medical diagnosis, he provided an interesting perspective: “I always pivot to medicine as an example of all the good it can do because almost everything it’s going to do there is going to be good. …We’re going to have a family doctor who’s seen a hundred million patients and they’re going to be a much better family doctor.” Likewise, the cofounder of OpenAI, Ilya Sutskever, was emphatic about AI’s future medical superintelligence: “If you have an intelligent computer, an AGI [artificial general intelligence], that is built to be a doctor, it will have complete and exhaustive knowledge of all medical literature, it will have billions of hours of clinical experience.”
We are certainly not there yet. But in the years ahead, as we fulfill the aspiration and potential for building more capable and medically dedicated AI models, it will become increasingly likely that AI will play an invaluable role in providing second opinions with automated, System 2 machine-thinking, to help us move toward the unattainable but worthy goal of eradicating diagnostic errors.
The above essay was published at Science on 25 January 2024 as part of their Expert Voices series. This version is annotated with figures and updated with an important new report that came out after I wrote the piece.
To read the original publication, click here.