What is the Message?
Artificial intelligence (AI) companies are shifting their focus from large language models to smaller, more efficient models.
These smaller models are designed to be cost-effective, energy-efficient, and customizable, making them more accessible to businesses concerned about the high costs and data privacy issues associated with large language models.
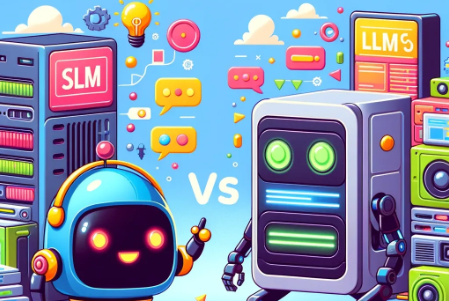
Key Points
- Shift to Small Language Models: Major AI companies like Apple, Microsoft, Meta, and Google are introducing AI models with fewer parameters but maintaining powerful capabilities.
- Cost and Efficiency: Smaller models are more affordable and require less computing power, addressing concerns over the expenses and resources needed for large language models.
- Business Adoption: The move aims to boost AI adoption among businesses by offering solutions that are less costly and easier to manage.
- Performance and Privacy: Smaller models can perform complex tasks locally on devices, which enhances data privacy and meets regulatory requirements.
- Advancing Capabilities: Despite having fewer parameters, new small models are demonstrating capabilities comparable to larger models, with some even outperforming previous versions.
- Practical Applications: Smaller models are suitable for a variety of applications, including mobile devices, making advanced AI accessible in everyday technology.
Key Statistics
- Parameters Comparison: OpenAI’s GPT-4o and Google’s Gemini 1.5 Pro have over 1 trillion parameters, while Meta’s Llama model has 400 billion parameters.
- Smaller Model Performance: Meta’s 8 billion parameter Llama 3 model and Microsoft’s 7 billion parameter Phi-3-small model show performance comparable to larger models like GPT-4 and GPT-3.5, respectively.
- Device Integration: Google’s Gemini Nano model is embedded in the latest Pixel and Samsung smartphones, showcasing the practicality of smaller models for mobile devices.
Small Language Models
Meta’s Llama 3 (8bn parameters)
- Smaller model that is compared favorably to GPT-4 in terms of performance.
- Targeted for more efficient, cost-effective use cases while maintaining high capability.
Microsoft’s Phi-3-small (7bn parameters)
- Outperforms the earlier GPT-3.5 model.
- Designed to be energy-efficient and customizable for various business applications.
Google’s Gemini Nano
- Embedded in the latest Pixel phone and Samsung’s S24 smartphone.
- Showcases the practical integration of small models in mobile devices for on-device AI tasks.
Apple’s OpenELM
- Designed to run on iPhones for text-based tasks.
- Highlights Apple’s entry into the market with a focus on small, efficient AI models suitable for mobile devices.
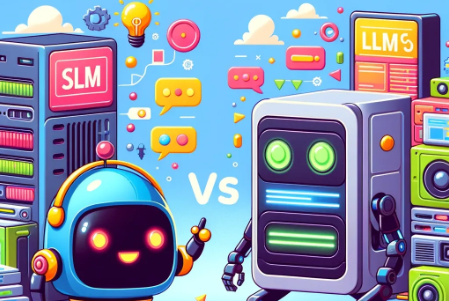
Executive Summary
Artificial intelligence companies are pivoting towards smaller language models to drive profitability and encourage widespread business adoption.
Leading tech giants such as Apple, Microsoft, Meta, and Google have launched new AI models with fewer parameters, designed to be cost-effective and energy-efficient while retaining powerful capabilities.
These models address critical concerns of high costs, substantial computing power requirements, and data privacy issues associated with large language models.
By offering smaller models, AI companies aim to make advanced AI more accessible to businesses, particularly those wary of significant investments in generative AI technologies.
Smaller models, which can operate locally on devices, also enhance data privacy and compliance with regulatory standards, thus appealing to privacy-conscious customers.
Despite having fewer parameters, these new small models demonstrate remarkable capabilities.
For example, Meta’s 8 billion parameter Llama 3 model and Microsoft’s 7 billion parameter Phi-3-small model show performance levels comparable to much larger models, sometimes even outperforming them.
This makes them viable for specific applications and practical for integration into devices like smartphones, exemplified by Google’s Gemini Nano model in its latest Pixel phone.
While small models present significant advantages, companies like OpenAI continue to invest in larger models, aiming for advanced capabilities and the potential to achieve human-level intelligence.
However, the diversification into smaller models represents a strategic move to cater to a broader market, balancing innovation with practical application and accessibility.
Adapted from:
https://www.ft.com/content/359a5a31-1ab9-41ea-83aa-5b27d9b24ef9