The Health Strategist
September, 23, 2021
Edited by Joaquim Cardoso, MSc
Image: National Cancer Institute
This is an excerpt from the paper “The potential use of big data in oncology”, authored by “ Willems SM, Abeln S, Feenstra KA, de Bree R, van der Poel EF, Baatenburg de Jong RJ, Heringa J, van den Brekel MWM, focusing on the topic above.
Authors:
Stefan M. Willemsa,b,⁎;
Sanne Abelnc;
K. Anton Feenstrac ;
Remco de Breed ;
Egge F. van der Poele ;
Robert J. Baatenburg de Jonge ;
Jaap Heringac ;
Michiel W.M van den Brekelf
Edited by: Joaquim Cardoso, MSc
DATA SOURCES OF BIG DATA IN MEDICINE [1]
Sources for big data are plentiful and can be of different kinds. In oncology, the most obvious are patient derived data.
These include various data points/subject and are usually recorded in electronic patient files for clinical purposes.
These data contain the clinical data of patients, tumors, treatment and outcome and may include demographic details such as gender and age, presenting symptoms, family history, comorbidity, radiological data (such as CT, MRI, PET, US) as well as solid and liquid tissue-based analysis (such as histopathological diagnosis/features, immunohistochemistry, DNA/RNA sequencing experiments, blood analyses and whole genome BAM files which contains on average ~ 100 GB).
But also data from in vitro experiments are important and can be an important source.
A second source of big data includes the computational analysis of these data.
These processed data comprise indirect and computed data, including radiomics and digital image analysis as well as genetic expression and mutation analyses.
An increasing source of these processed data come from machine learning and usually contains large computer data files of structured data.
A third source of big data comes from the patients themselves, including patient related outcome measurements (PROMs) and patient related experience measurements (PREMs) who record all kinds of measures using apps on computers and mobile devices, either provided by their caregivers (eHealth, telemedicine) or by their own initiative.
A fourth source is from published literature (IBM project). As every year over 1 M biomedical articles are published, there is no doctor in the world who can read even just a fraction of the knowledge published, let alone all relevant textbooks and other internet sources.
Nevertheless, one key factor stands out for big data in oncology: the depth (volume) of data per patient. In oncology many observables (thousands to even millions) per patient are routinely generated and stored, while typical cohort sizes of patients are relatively small.
This imbalance in the depth of data per patient versus the cohort size is even more prominent for rare cancer types such as head and neck cancer.
However, recent methodological advances in machine learning and neural network are specifically powerful if there are instances to learn over. For example, object recognition in images works very well, but thousands to millions of examples are needed to optimize such methods.
Hence, if we want to translate this to optimize personalized treatments, we also need data depth in the number of samples[2].
This makes good data keeping, data standardization, data sharing, data provenance and data exchange protocols essential for oncology, and absolutely necessary in the field of head and neck oncology.
INTEGRATION OF BIG DATA IN HEAD AND NECK CANCER (HNC) [3]
So as sources are multiple and volumes are high, standardization in data capturing is warranted. This standardization will lead to much more uniform and complete datasets, which are easier to link to other data resources.
Standardization is essential for data integration, which is needed for data interpretation and creating value from the data. E.g. to monitor the quality of care after specific surgical interventions, it’s crucial to understand the case mix of patients in terms of tumor stage, (neo) adjuvant therapies, co-morbidity, etc.
In The Netherlands, several national databases have been established to structurally capture clinical, pathological, genetic/genomic data and PROM/PREMs (Table 1).
Table 1
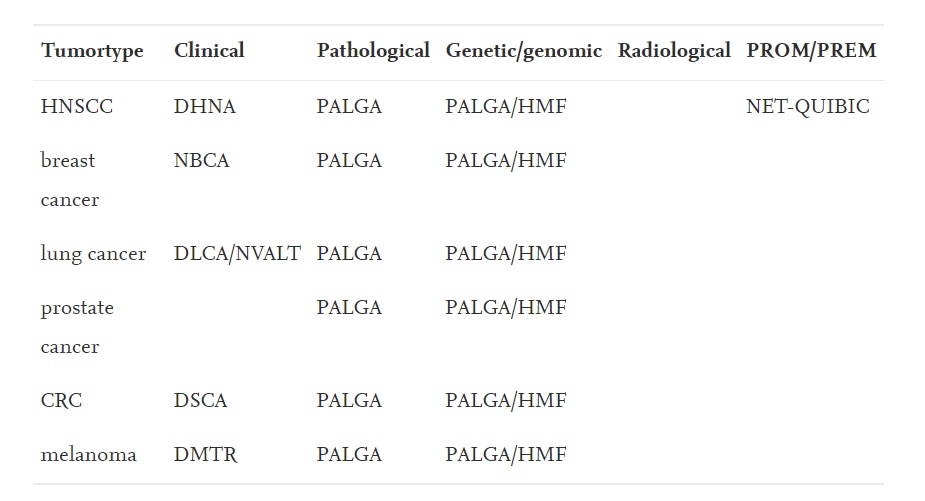
Data sources for most common cancer types in The Netherlands, including HNC.
For most common tumor types, collections of various data (clinical, pathological genomic/genetic) have been well organized, with the exception of radiological data.
DHNA: Dutch Head and Neck Audit; NBCA: National Breast Cancer Audit; DLCA: Dutch Lung Cancer Audit.
DSCA: Dutch Surgical Colorectal Audit; DMTR: Dutch Melanoma Treatment Registry; PALGA: Pathologisch Anatomisch Landelijk Gegevens Archief; HMF: Hartwig Medical Foundation
In The Netherlands, several national databases have been established to structurally capture clinical, pathological, genetic/genomic data and PROM/PREMs
Clinical data are being reported since 2014 in the Dutch Head and Neck Audit (DHNA), which was incorporated in the Dutch Institute for Clinical Auditing (DICA) 2017 who installed subgroups for specific disease types (cancer and non-cancer).
Tumor tissue based data such as pathological and genomic/genetic data have been structurally collected nation-wide, and now also synoptically reported for > 20 different tumor types.
In contrast, structured data basing of radiological data is still lacking.
In addition to other cancer types, for head and neck, the NET-QUBIC consortium has initiated the national platform to report PREMs/PROMs for HNC.
So for HNC in The Netherlands, most patient-derived data sources have now been installed. Internationally, many similar initiatives are underway, e.g. the head and neck squamous cell carcinoma (HNSCC) collection[4] and The Cancer Imaging Archive (TCIA)[5].
The essential next step is to bring these data together now, preferentially on the individual patient-level.
In the Netherlands, head and neck cancer care is centralized in 8 head and neck centers with 6 preferred partners and united within the Dutch Head and Neck Society (NWHHT).
This places the head and neck cancer community in the ideal position to unite the expertise and endeavors to optimize uniform data input and roll out of the current separate databases.
Moreover, head and neck cancer is in the ideal position to establish nationwide linkage of these databases and develop algorithms for integrated data-analysis.
FAIR DATA [6]
To ensure data can be reused in secondary studies, it is essential they adhere to the FAIR (Findable, Accessible, Interoperable, Reusable) principles.
These FAIR data principles have been first published in 2014[7]. Since then, the principles have been recognized and endorsed by the G20 (2016) and the G7 (2017), while the EU has taken FAIR data at the heart of the European Open Science Cloud (EOSC).
An important part of FAIRness of a data resource is concerned with its metadata, where findability (F) is reliant on having a persistent identifier in place; accessibility (A) requires clearly defined access rules (data privacy constraints are within the definition) and licensing; and interoperability (I) is dependent upon employing a community-recognized ontology for describing the data.
Finally, provenance of the data and accurateness and completeness of the meta-data is essential for the reusability (R) of the data.
CHALLENGES AND FUTURE PERSPECTIVES [8]
Though already applied in current clinical practice, and with tremendous promises ahead, producers of big data also face challenges to make them optimally useful in life sciences.
- First of all, with rapidly developing technologies, such as next generation sequencing (especially whole exome/genome sequencing) and radiomics, the volume of data continues to increase exponentially.
- A second challenge comes with the proper governance of data, especially when linked from various sources.
First of all, with rapidly developing technologies, such as next generation sequencing (especially whole exome/genome sequencing) and radiomics, the volume of data continues to increase exponentially.
These huge amounts of data add an increasing complexity that might impede data interpretation.
This holds especially true when the increase in data (velocity and volume) is also paralleled by an increase in the heterogeneity of data (variability), including treatments, outcomes, differences in study design, analytical methods and interpretation pipelines, which hamper drawing firm conclusions from the data.
A second challenge comes with the proper governance of data, especially when linked from various sources.
How and which data are made available, who is the owner of the data? Does the patient still have governance of this own datasets?
Or does the researcher governs it, and if so, which researcher, or the treating physician, the data generator, or is it the person who tries to make sense out of the data (e.g. the computational biologist or medical bioinformatician)?
REPOSITORIES AND DATABASES FOR ARCHIVING AND SHARING BIOMOLECULAR PATIENT DATA [9]
In a (bio)medical research setting, the aim is often to obtain as many data as possible from as many patients and hospitals as possible, while privacy issues as well as security and protection measures (GDPR) often prohibit the availability.
One key challenge is to store patient identifiable data, such as genome sequences, in such a way that the data can be reused for other studies, while safe guarding the privacy of the patients from which the data was collected (www.phgfoundation.org).
Whereas for large data sets in other domains, such as computer science, open accessibility may be preferred, here privacy concerns must clearly outweigh a desire for complete openness.
The European Genome Archive (EGA) is a purposebuilt database to store raw sequencing data.
For each study, with data stored in EGA, there is a strong role for a data access committee (DAC), which is governed by the research initiative that collected the data and can decide to provide access to the data upon request by other researcher[10].
A secondary challenge is posed, when researcher would like to browse processed biomolecular data, without the ability to trace back individual markers.
Here several solution are available that summarise the data without showing individual markers[11], or other repositories solve this by fine graining access control[12].
Lastly, a remaining challenge is to link the different data resources in a privacy aware manner, while being able to track the exact computational processing that has been performed on the data; several initiatives have made the first steps to achieve such linking[13] [14] [15].
The potential use of big data in life sciences and head and neck oncology is tremendous. It might also transform the way we share clinical and research data.
Instead of individuals or organizations physically sharing datasets, the (near) real time/streaming of data together with the huge volume of data, will make it impossible to keep exchanging data sets like we do today.
Instead of bringing together all kind of datasets in a central comprehensive database, a likely scenario might be that big data users will develop more organic, decentralized virtual networks, such as envisioned in the personal health train by the Dutch Techcentre for Life Sciences (DTL)[16].
Within these networks, databases are connected as nodes, accessible under predefined conditions to users. Increased connectivity and (thus) complexity will also demand new ways of interpreting data, as well as translating these data and its interpretations back to the individual patient.
This latter requires big data-derived knowledge computed from all these data sets to be translated to the specific “small data” environment of the individual care dependent patient.
For this last step, crucially also integrating intuitive and emotional aspects, we still need medical professionals. Bed side manners are for the foreseeable future well out of reach of big data or machine learning approaches.
Instead of bringing together all kind of datasets in a central comprehensive database, a likely scenario might be that big data users will develop more organic, decentralized virtual networks …
CONCLUSION [17]
- The value of big data capturing relies on the volume, velocity variety, veracity of various, often complex, data sets.
- Integration of these sources is key and will be beneficial for improvements in biomedical research, patient care and monitoring quality of care.
- In The Netherlands, where head and neck cancer care is centralized and various national big data resources are in place, there is an unique opportunity to unite, link and integrate these data and fulfill this unmet need.
- Such a head and neck cancer infrastructure should optimize data input as well as (bioinformatical) data integration including FAIRification.
References
[1] Willems SM, Abeln S, Feenstra KA, de Bree R, van der Poel EF, Baatenburg de Jong RJ, Heringa J, van den Brekel MWM. The potential use of big data in oncology. Oral Oncol. 2019 Nov;98:8–12. doi: 10.1016/j.oraloncology.2019.09.003. Epub 2019 Sep 12. PMID: 31521885.
[2] Zhang C, Bijlard J, Staiger C, Scollen S, et al. Systematically linking tranSMART, galaxy and EGA for reusing human translational research data. F1000Res. 2017;6. https://doi.org/10.12688/f1000research.12168.1. Aug 16 ELIXIR-1488.
[3] Willems SM, Abeln S, Feenstra KA, de Bree R, van der Poel EF, Baatenburg de Jong RJ, Heringa J, van den Brekel MWM. The potential use of big data in oncology. Oral Oncol. 2019 Nov;98:8–12. doi: 10.1016/j.oraloncology.2019.09.003. Epub 2019 Sep 12. PMID: 31521885.
[4] Grossberg AJ, Mohamed ASR, El Halawani H, et al. Sci. Data 2018;5:180173. https://doi.org/10.1038/sdata.2018.173. Sep 4.
[5] Prior F, Smith K, Sharma A, et al. The public cancer radiology imaging collections of The Cancer Imaging Archive. Sci Data. 2017;19(4):170124https://doi.org/10. 1038/sdata.2017.124. Sep.
[6] Willems SM, Abeln S, Feenstra KA, de Bree R, van der Poel EF, Baatenburg de Jong RJ, Heringa J, van den Brekel MWM. The potential use of big data in oncology. Oral Oncol. 2019 Nov;98:8–12. doi: 10.1016/j.oraloncology.2019.09.003. Epub 2019 Sep 12. PMID: 31521885.
[7] Wilkinson MD, Dumontier M, Aalbersberg IJ, et al. The FAIR guiding principles for scientific data management and stewardship. Sci Data 2016;15(3):160018https:// doi.org/10.1038/sdata.2016.18.
[8] Willems SM, Abeln S, Feenstra KA, de Bree R, van der Poel EF, Baatenburg de Jong RJ, Heringa J, van den Brekel MWM. The potential use of big data in oncology. Oral Oncol. 2019 Nov;98:8–12. doi: 10.1016/j.oraloncology.2019.09.003. Epub 2019 Sep 12. PMID: 31521885.
[9] Willems SM, Abeln S, Feenstra KA, de Bree R, van der Poel EF, Baatenburg de Jong RJ, Heringa J, van den Brekel MWM. The potential use of big data in oncology. Oral Oncol. 2019 Nov;98:8–12. doi: 10.1016/j.oraloncology.2019.09.003. Epub 2019 Sep 12. PMID: 31521885.
[10] Lappalainen I, Almeida-King J, Kumanduri V, et al. The European genome-phenome archive of human data consented for biomedical research. Nat Genet. 2015;47(7):692–5.
[11] Klonowska K, Czubak K, Wojciechowska M, et al. Oncogenomic portals for the visualization and analysis of genome-wide cancer data. Oncotarget 2016;7(1):176–92. Jan 5.
[12] Christoph J, Knell C, Bosserhoff A, et al. Usability and suitability of the omicsintegrating analysis platform tranSMART for translational research and education. Appl Clin Inform. 2017;8(4):1173–83.
[13] He S, Yong M, Matthews PM, et al. TranSMART-XNAT connector tranSMART-XNAT connector-image selection based on clinical phenotypes and genetic profiles. Bioinformatics 2017;33(5):787–8. Mar 1.
[14] Hoogstrate Y, Zhang C, Senf A, et al. Integration of EGA secure data access into galaxy. F1000Res 2016;5. https://doi.org/10.12688/f1000research.10221.1. Dec 12pii: ELIXIR-2841. eCollection 2016.
[15] Zhang C, Bijlard J, Staiger C, Scollen S, et al. Systematically linking tranSMART, galaxy and EGA for reusing human translational research data. F1000Res. 2017;6. https://doi.org/10.12688/f1000research.12168.1. Aug 16 ELIXIR-1488.
[16] Eijssen L, Evelo C, Kok R, et al. The Dutch techcentre for life sciences: enabling dataintensive life science research in the Netherlands. F1000Research 2015. https://doi. org/10.12688/f1000research.6009.2.
[17] Willems SM, Abeln S, Feenstra KA, de Bree R, van der Poel EF, Baatenburg de Jong RJ, Heringa J, van den Brekel MWM. The potential use of big data in oncology. Oral Oncol. 2019 Nov;98:8–12. doi: 10.1016/j.oraloncology.2019.09.003. Epub 2019 Sep 12. PMID: 31521885.
About the authors of the original paper (long version) & affiliations:
Stefan M. Willemsa,b,⁎;
Sanne Abelnc;
K. Anton Feenstrac ;
Remco de Breed ;
Egge F. van der Poele ;
Robert J. Baatenburg de Jonge ;
Jaap Heringac ;
Michiel W.M van den Brekelf
a Department of Pathology, University Medical Center Utrecht, Utrecht University, Utrecht, the Netherlands
b Department of Pathology, Netherlands Cancer Institute, Amsterdam, the Netherlands
c Department of Computer Science, Faculty of Science, Vrije Universiteit, Amsterdam, the Netherlands
d Department of Head and Neck Surgical Oncology, University Medical Center Utrecht, Utrecht, the Netherlands
e Department of Head and Neck Surgery, Erasmus Cancer Center, Erasmus MC, Rotterdam, the Netherlands
f Department of Head and Neck Oncology and Surgery, Netherlands Cancer Institute, Amsterdam, the Netherlands
Originally published at (the long version of the paper)
https://www.sciencedirect.comThe potential use of big data in oncology
Addresses the potential utility of big data in head and neck oncology. * Touches upon the integration of clinical…www.sciencedirect.com
How to cite (the long version of the paper)
Willems, S. M., Abeln, S., Feenstra, K. A., de Bree, R., van der Poel, E. F., Baatenburg de Jong, R. J., Heringa, J., & van den Brekel, M. W. M. (2019). The potential use of big data in oncology. Oral Oncology, 98, 8–12. https://doi.org/10.1016/j.oraloncology.2019.09.003