A proposed home-recovery surgical care model in which AI-driven activity-monitoring systems are used to support surgeon decisions, help patients recover safely in their homes, and reduce post-acute rehabilitation costs for the health care system.
NEJM Catalyst
Kyung Mi Kim, PhD, RN, Maria Yefimova, PhD, RN, Feng Vankee Lin, PhD, MB, RN, Jeffrey Kyle Jopling, MD, and Erik Nathan Hansen, MD
June 8, 2022
Executive Summary
Joaquim Cardoso MSc.
Health and Care Institute
Research and Strategy for Better Health for All
June 8, 2022
What is the problem?
- Half of Americans over the age of 65 years will undergo operative procedures at some point in their lives, and these numbers are projected to grow.
- Older adults often wish to recover in their homes after surgery.
- However, there are numerous barriers to safe discharge home.
What is the current model?
- Traditional methods of assessing a patient’s ability to perform basic activities of daily living are performed at static, defined points in the perioperative period.
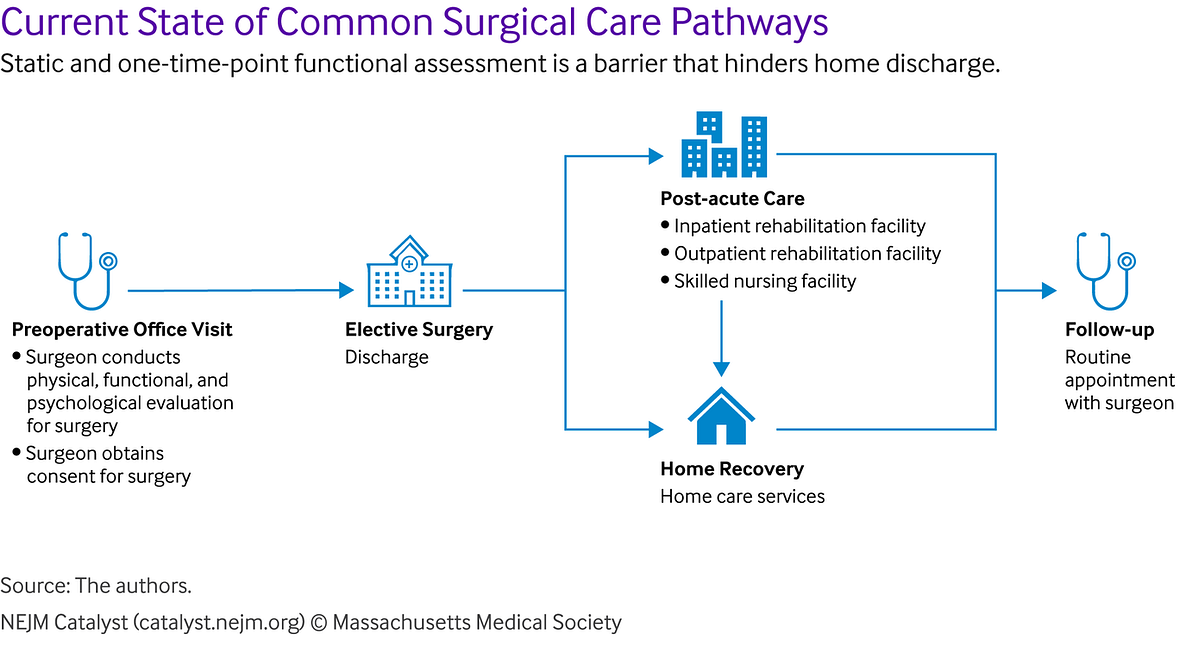
What is the proposed model?
- To address innovations and challenges with respect to the current and AI-driven ADL measures discussed above, we evaluated the current state of common surgical care pathways (Figure 3) and developed a framework for a future home-recovery care model after surgery (Figure 4).
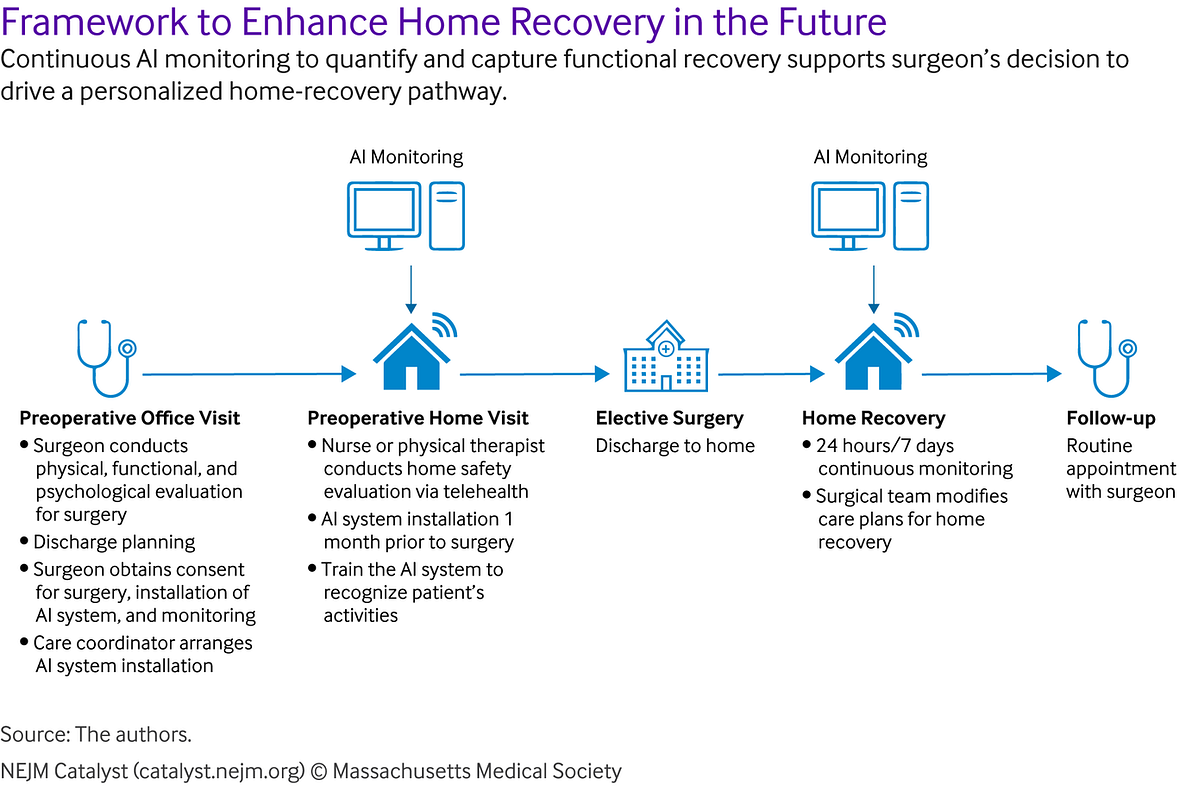
- Shifting the focus to a personalized continuous assessment spanning the continuum of perioperative care could be the key to allowing older adults to recover safely in their homes.
What are the benefits?
- The potential benefits of this novel approach include improved patient satisfaction, improved functional outcomes, decreased adverse events, and reduced cost of care.
An overview of this article?
- In this article, the authors examine the innovative artificial intelligence (AI)–driven activities of daily-living monitoring systems and propose a home-recovery surgical care model using AI-driven daily activity measures.
- The use of multidimensional AI data has the potential to measure many activities of daily life.
- Further advances and refinements in the technology are warranted to expand the cadre of activities that can be measured.
- This approach has the potential to increase the number of patients who can safely recover at home after surgery.
- It further allows clinicians to predict and directly address individual patient needs regarding basic activities of daily living within the window for timely initiation of care.
Structure of the publication:
- Introduction
- The Challenge of Monitoring ADLs at Home
- The Paradigm Shift in Monitoring ADLs for Safe Home Recovery After Surgery
- The Evidence on Innovations
- Framework: AI-Enabled Surgical Care Innovations
- A Home-Recovery Surgical Care Model Using AI-Driven ADL-Monitoring Systems
- Clinical Implications of AI-Driven Measures of ADLs
- Potential Challenges in the Implementation of the Home-Recovery Surgical Care Model and Strategies to Overcome Challenges
- Enhance Home Recovery After Surgery Utilizing AI
ORIGINAL PUBLICATION (full version)
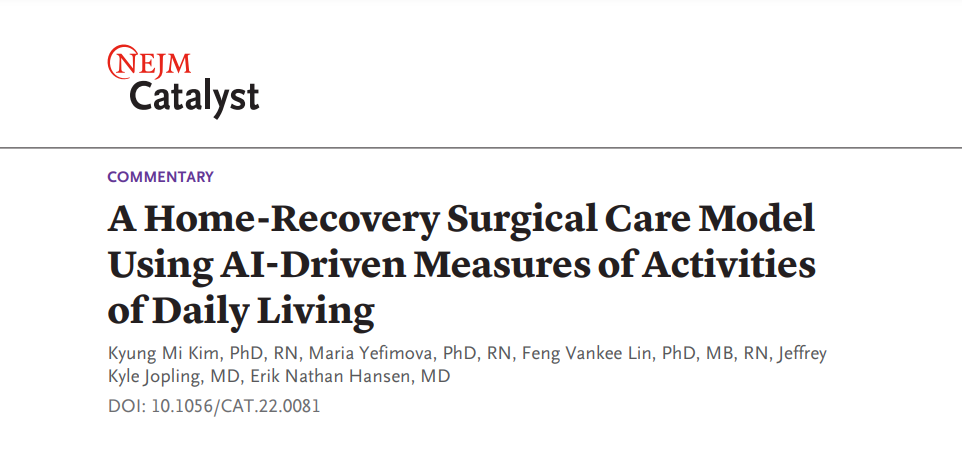
Introduction
Half of Americans over the age of 65 years will undergo operative procedures at some point in their lives, and these numbers are projected to grow.1
Older adults may face challenges with activities of daily living (ADLs) after surgery due to limited physiological reserve, and their recovery can be hampered by multiple comorbidities.2 Participation in basic ADLs, such as bathing, dressing, or transferring, can be reflective of daily functioning. Returning to individual baseline ADLs after surgery can take 3 to 6 months or longer,3 so it is imperative to monitor older patients who are at higher risk for adverse postoperative outcomes.4 Comprehensive and continuous assessment of older patients’ functional abilities offers us the opportunity to better understand and evaluate the trajectory of their postoperative recovery.
Older adults often wish to recover in their homes after surgery.
Unfortunately, home discharge is limited due to a multitude of interrelated factors, including functional and physical conditions, safety concerns, care coordination, family cohesion, and caregiver support. This desire to discharge home following surgery has been further amplified by the recent Covid-19 pandemic and concerns related to discharge to a communal living environment. Understanding how older patients manage daily activities can help the care team to engineer care plans that facilitate the patient’s recovery at home.
To be effective for assessing progress of postoperative recovery, ADL measures must be reliable and sensitive to change.
However, current measurement approaches are often time-consuming and crude because they rely on either self-reporting or direct observation by a clinician.
Overcoming these challenges may be possible with technological innovations in artificial intelligence (AI) and computer vision.
Novel technologies currently on the market may allow for capture of daily behaviors performed at home that are indicative of functional abilities in older individuals.
Such tools may be used in ADL measurements from the preoperative period to the post-acute care period after surgery.
For example, innovative AI systems may be used to establish preoperative baseline function, which can then be used to determine the needs for pre-habilitation and to monitor postoperative recovery.
However, current measurement approaches are often time-consuming and crude because they rely on either self-reporting or direct observation by a clinician.
Overcoming these challenges may be possible with technological innovations in artificial intelligence (AI) and computer vision.
Half of Americans over the age of 65 years will undergo operative procedures at some point in their lives, and these numbers are projected to grow.
It is important for clinicians and researchers to understand the strengths and limitations of various approaches to monitoring ADLs.
This article describes current methods in the context of surgical care. It further discusses novel AI-driven ADL-measurement approaches, including wearables or sensors embedded in the home environment that are coupled with AI algorithms.
These innovations are designed to overcome challenges in measurement and could be a key to the delivery of effective, high-quality, and personalized surgical care.
The AI-driven ADL-measurement approaches have a limited effect on their own, but, when purposefully deployed as part of a patient-care model, they have a significant potential to affect patient care positively.
Therefore, after describing and analyzing the innovations in ADL measurement, we propose one such model to support surgeon decisions and to enable personalized home recovery.
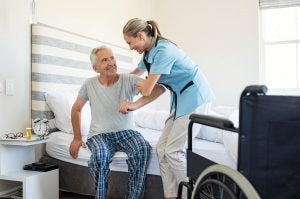
The Challenge of Monitoring ADLs at Home
To help older adults recover optimally and safely at home after surgery, we need an accurate and timely detection system that measures ADLs, detects changes in ADLs, and predicts ADL deficit-associated adverse events.
This system needs to be coupled with a reliable and easily understood clinical support system that helps clinicians make rapid, efficient care decisions based on changes in individuals’ ADLs.
Various measures for ADLs exist (e.g., questionnaire measures based on self, caregiver, or clinician reporting and performance-based measures).
Although these measures are known to represent valid indices of an individual’s health status and need for services, they suffer from multiple associated limitations.
- Specifically, they are often time-consuming and resource-intensive, highly subjective, and susceptible to bias.
- In addition, they often have varying reliability, weak reproducibility, and weak sensitivity to change.
- Finally, they often focus only on narrow aspects of activities and usually lack documentation.
These problems limit the utility of current ADL measures in dynamically capturing an individual’s functional status over time and introduce the risk that clinicians might miss the critical time for intervention.
This delay ultimately can result in a patient’s otherwise avoidable functional decline and subsequent loss of independence after surgery.
These challenges can be especially pernicious for surgical patients who experience inevitable functional declines postoperatively.
Failure to meet a surgical patient’s individual care needs regarding ADLs and missing the window for timely initiation of care can lead to
- exacerbations of functional impairment,
- adverse events,
- poor health-related quality of life,
- ineffective resource utilization (e.g., rehabilitation in a high-cost setting,
- avoidable readmission and hospitalization, and increased costs of care), and
- unnecessary suffering.
In sum, while an important first step, current ADL measures have limited power for predicting ADL-associated health outcomes (e.g., falls, returns to baseline function) and informing post-acute surgical care planning.
In sum, while an important first step, current ADL measures have limited power for predicting ADL-associated health outcomes (e.g., falls, returns to baseline function) and informing post-acute surgical care planning.
ADL monitoring that can automatically capture a continuous stream of activity-related data would aid in the detection of changes and the recognition of a pattern consistent with adverse event-related functional recovery after surgery.
Such a system would allow clinicians, including surgeons and primary care providers, to offer supportive interventions to prevent or minimize adverse events, thereby allowing older surgical patients to recover safely at home.
There is a need to shift from traditional diagnostic ADL assessments that are static in time to ecologically valid, personalized, and continuous measures of everyday function from the preoperative period to the post-acute care period after surgery.
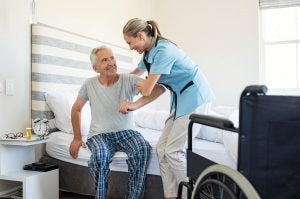
The Paradigm Shift in Monitoring ADLs for Safe Home Recovery After Surgery
Shifting the focus from traditional diagnostic ADL assessments at one point in time to personalized everyday assessments,spanning the entire perioperative period could be the key to allowing older adults to recover safely and effectively in their homes.
Innovations in ADL measurement can help us to (1) continuously collect real-time data on ADLs that are already validated, (2) open the possibility for more nuanced ways of looking at these established measures and identifying the most relevant and useful ADLs, and (3) enable new innovative measures and a ubiquitous ADL monitoring system for safe home recovery after surgery.
This desire to discharge home following surgery has been further amplified by the recent Covid-19 pandemic and concerns related to discharge to a communal living environment.
This desire to discharge home following surgery has been further amplified by the recent Covid-19 pandemic and concerns related to discharge to a communal living environment
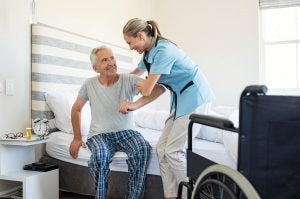
The Evidence on Innovations
AI-driven ADL monitoring systems allow clinicians to collect and analyze continual patterns of individual activities effectively, learn individuals’ behavioral norms, quickly detect and predict events associated with changes in ADLs (e.g., falls and near falls), and integrate the findings from monitoring into clinical care pathways to provide actionable items supporting home-care decisions and facilitating personalized interventions.,
Innovations that are designed to facilitate home recovery after surgery must be (1) able to detect ADL changes accurately and frequently to prevent adverse events and (2) cost-effective.
We created an innovation matrix to visualize and identify the optimal strategy to achieve these goals ().
This matrix illustrates that commonly used measures are less costly but rely heavily on human effort from patients, interviewers, or clinicians.
In contrast, AI-driven measures are highly automated. Computer vision technology classifies, localizes, and detects an object in an image and interprets visual and location data simultaneously.
The cost of computer vision is on the middle-to-high side for all measures, but it is not the highest when the required clinician and patient times, other labor, and overhead costs are considered.
Furthermore, the benefits of time efficiency and continuous data capture could exceed the costs of the technologies.
Financial and human costs related to the limitations of current ADL measures, such as measurement errors, undetected functional decline, and missed opportunities to intervene for the purpose of maintaining independence should also be considered.
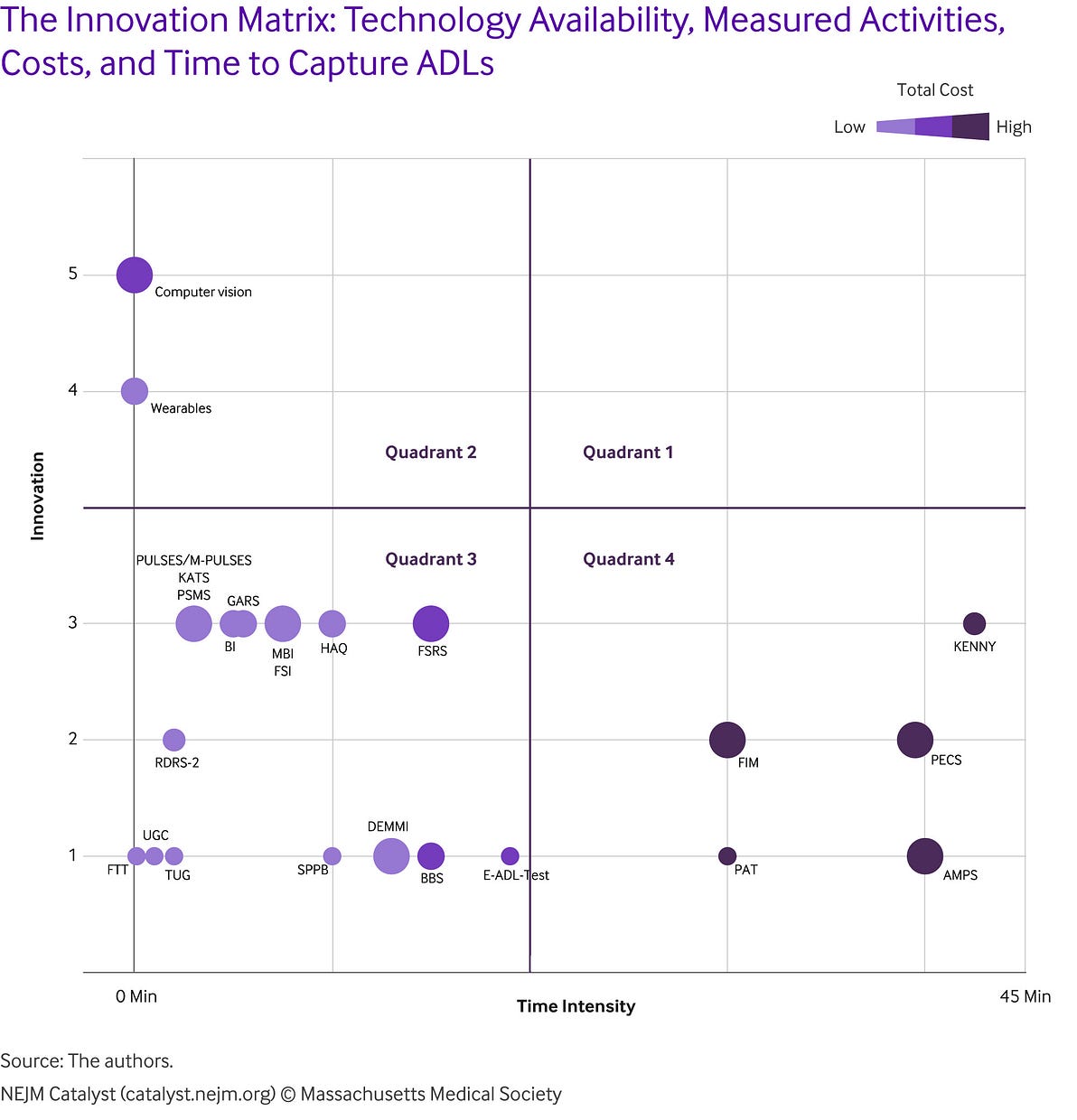
In , the data are based on 23 current measures (see Appendix) and two innovative ADL measures (wearable and computer vision).
For the innovation presented on the Y axis, points are assigned to each instrument to highlight the extent of automation for both providers and patients in measuring ADLs (1 point indicates that the clinician measures activities or that the patient is required to make an effort to conduct certain performances, 2 points indicates that the clinician measures activities, 3 points indicates that the patient self-reports the level of activities or that a nonclinical interviewer measures activities using a questionnaire, 4 points indicates that measuring activities is automated but that human effort is required [e.g., as is the case with wearables], and 5 points indicates that the measurement of activities is automated and that no human effort is required).
Time, presented on the X axis, indicates the average time required to measure ADLs using each instrument.
The times that were used to generate this matrix are the approximate times as reported in the literature or elsewhere; when such information was not available, we used estimated times, which were calculated as the total number of items multiplied by 30 seconds. The horizontal scale starts at 0, indicating that no time effort is necessary.
The size of the circle indicates the number of ADLs measured by each instrument. The color of the circle indicates the cost of the instrument (darker = costlier). Costs that are accounted for include the costs of labor (e.g., provider), facility (e.g., rent), equipment (e.g., computer vision cameras, Wi-Fi unit), AI model development, and the license fee for use of the instrument, if applicable. The total number of circles may not appear to be 25 because of overlaps in time and innovation scores. The matrix incorporates the best available evidence.-Quadrant 2 shows time-efficient and technologically innovative measures, which are the least human-intensive. Quadrant 3 includes measures that are time-efficient but more human-intensive than those in Quadrant 2. Quadrant 4 includes measures that are time-intensive and human-intensive. No measures are included in Quadrant 1, which represents time-intensive but technologically innovative measures.
The AI-driven ADL-measurement approaches have a limited effect on their own, but, when purposefully deployed as part of a patient-care model, they have a significant potential to affect patient care positively.
As data credibility is fundamental to determining the value of AI-driven ADL monitoring, we next assess how reliably current AI technology measures ADLs. Scaling an innovation that does not provide reliable data in measuring ADL-related outcomes (e.g., functional change) would just add cost instead of adding benefits.
The Value of AI-Driven ADL Measures
It is important to examine which activities can be measured reliably and which cannot. To that end, we created the “value proposition canvas” () to assess activities that AI can appropriately measure and thus add value.
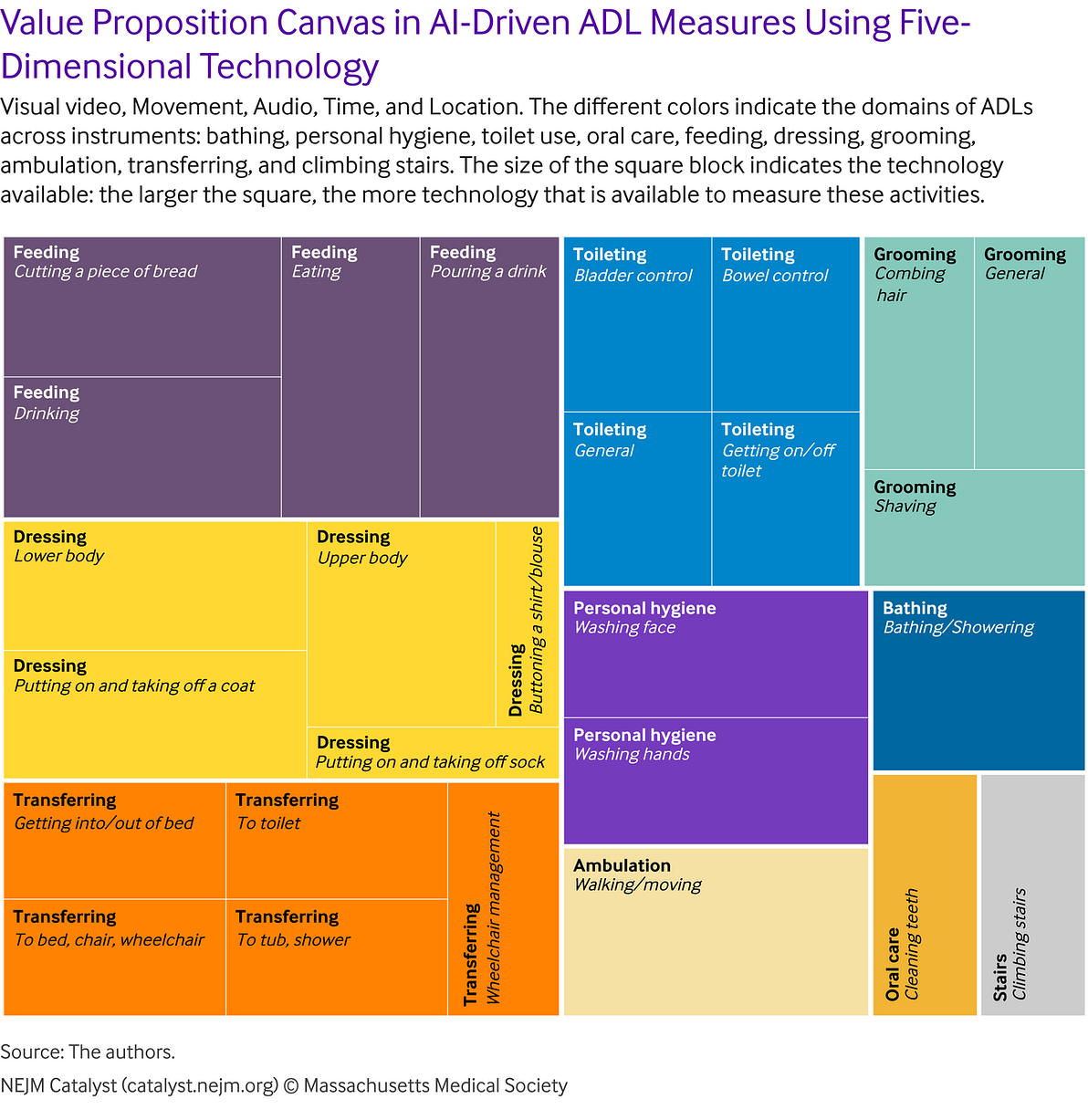
We identified 78 activities that were measured with the 25 ADL instruments presented in .
We categorized these activities into 11 domains: bathing, personal hygiene (e.g., washing hands), toilet use, oral care, feeding, dressing, grooming, ambulation, transferring, climbing stairs, and other (e.g., sitting, rising, standing and balancing, and organizing) (see Appendix).
We focused on 29 activities from 10 domains, excluding the “other” domain. Focused activities are either functions that patients reported as important or those that are frequently measured. We assigned 1 point for each activity measured and added the points across the measures to examine the frequency of measures in each activity.
The value matrix indicates that various technology options are available to measure certain ADLs, including dressing, personal hygiene, feeding, bathing, and ambulation, whereas limited technology options are available to measure toilet use (e.g., perineal care) and grooming (e.g., cleaning feet and nails). Based on the value matrix that we created, capturing dressing, feeding, and ambulation behaviors with AI can be effective, whereas measuring toilet use with AI may not yet be reliable or appropriate. It is worth noting that using multidimensional technology (e.g., ambient sensors that harvest multidimensional data, such as video, audio, and time) enables automated measuring of bathing and personal hygiene. Furthermore, innovative technology, such as switching between video and privacy modes using audio, time, or thermal imaging, could alleviate privacy concerns and be an effective solution to measure ADLs in the privacy of the home (e.g., dressing, bathing, and toilet use). Such technology is also commercially available.
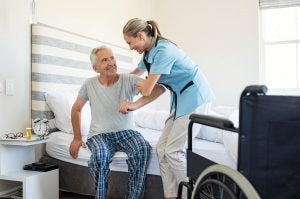
Framework: AI-Enabled Surgical Care Innovations
To address innovations and challenges with respect to the current and AI-driven ADL measures discussed above, we evaluated the current state of common surgical care pathways () and developed a framework for a future home-recovery care model after surgery ().
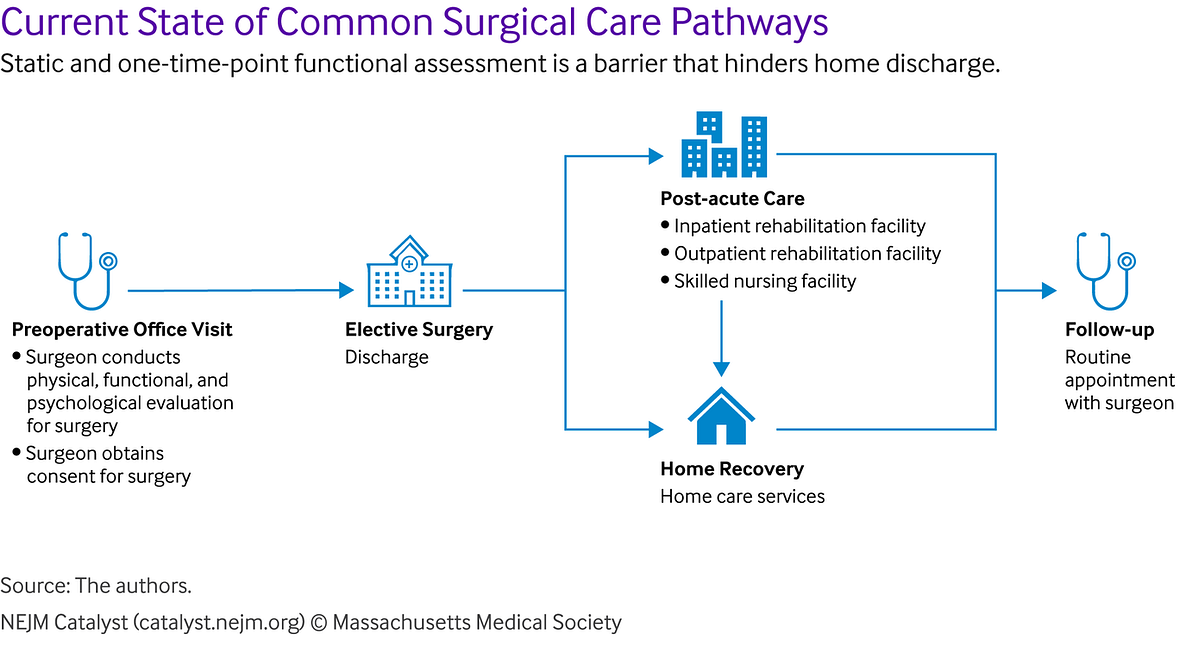
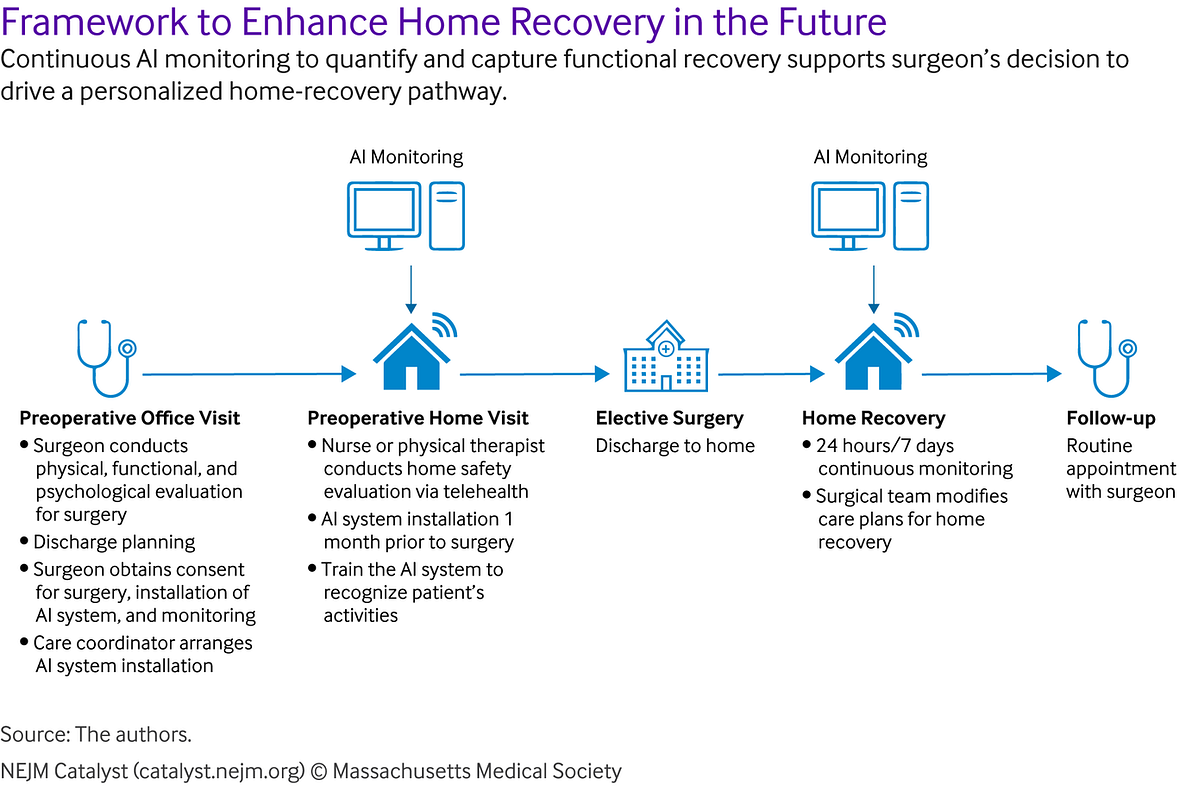
The home-recovery surgical care model utilizes AI that captures credible ADL data, detects changes in functional recovery, and predicts relevant adverse events with an interface that is capable of providing personalized and clinically interpretable information to aid clinician decision-making for postoperative management plans.
AI-captured ADL data and detected ADL changes help to develop AI models to predict relevant adverse events.
This information, displayed in a personalized interface, can support clinicians and patients in their decision-making about in-home recovery for each patient.
Information and analysis that is meaningfully integrated into the electronic health record and properly blended into frontline workflows might support effective and efficient use by clinicians.
While an important first step, current ADL measures have limited power for predicting ADL-associated health outcomes (e.g., falls, returns to baseline function) and informing post-acute surgical care planning.
This framework sheds light on the importance of ADL monitoring not only postoperatively, but also preoperatively, as it helps to guide the development of the in-home recovery protocol based on AI-supported functional status monitoring.
In the next section, we discuss how this framework can be applied in practice.
A Home-Recovery Surgical Care Model Using AI-Driven ADL-Monitoring Systems
We propose implementing AI-driven ADL-monitoring systems that could help clinical decision-making for a home-recovery surgical care model.
Its primary goals are to optimize patient functional status before surgery, improve patient functional recovery outcomes after surgery, maximize patient independence at home, and provide quality care at a lower cost than other rehabilitation settings (e.g., inpatient or a skilled nursing facility). Based on our innovation and value matrices, we propose that the optimal application of AI-driven ADL-monitoring systems employs computer vision technology utilizing multidimensional data.
Target Population
The primary target population of this home-recovery surgical care model comprises community-dwelling older adults (≥55 years of age) who undergo elective surgery and need assistance in performing ADLs after surgery but can still recover in their homes, navigate their communities with the help of caregivers, and have high-speed Internet access or the ability to install access. In particular, patients who undergo elective orthopedic surgery (e.g., total hip or knee replacement) might benefit the most in this home-recovery model because elective surgical procedures provide time to train AI to establish a personalized baseline prior to surgery. Such procedures are the most commonly performed and are growing among the aging population. Furthermore, loss of function and deteriorated health-related quality of life are common complications following these procedures, and the costs involved in post-acute care rehabilitation settings are greater than those for other procedures, such as coronary artery bypass grafting or colectomy.
Additionally, a widely implemented value-based payment scheme promotes financial incentives for organizations to adopt innovative technology. Hospitals are more likely to bear the financial risk of adopting an innovation that supports early discharge to home and prevents ADL-related complications and readmissions. Nonelective operations (e.g., hip replacement for a fracture after a fall or colectomy for perforated diverticulitis) are not targeted in our proposed home-recovery care model because in such cases we can only begin assessing ADLs while the patient is in the hospital and thus cannot compare the preoperative baseline data to the postoperative data.
Preoperative Monitoring
Computer vision technology-enabled ADL monitoring that uses the multidimensional technology addressed above would be installed in the patient’s home a month before the planned surgery to capture preoperative baseline data on functional status (ADL performance). The initial 2 weeks following the installation would be used to train the system to recognize each person’s activities. This step is critical for identifying the patient and excluding other family members or visitors. Consent for the installation and monitoring as well as surgery would be obtained during the preoperative visit from participants and their household members (e.g., spouses, children, caregivers) to track all household members during the initial 2 weeks. Obtaining consenting from both participants and their household members is essential to protect the privacy of all parties. Features that protect personal privacy and data security (such as using audio of running water to detect bathing while using visual video and location data for walking) are validated and commercially available.
Preoperative monitoring will provide a basis for clinicians when determining whether an individual is able to be discharged home safely for recovery, needs more assistance in the form of in-house caregivers, or is likely to need to be discharged to a post-acute care rehabilitation facility.
Shifting the focus from traditional diagnostic ADL assessments at one point in time to personalized everyday assessments spanning the entire perioperative period could be the key to allowing older adults to recover safely and effectively in their homes.
Postoperative Monitoring
Patients who have been evaluated to be safe to recover at home would be continuously monitored with use of an AI-enabled ADL-detection system. This system would continuously detect ADLs following discharge to home, detect adverse events that occur in the home environment, and capture deviations from expected recovery trajectories so that potential complications can be better anticipated and potentially prevented. This monitoring system would display the data in a clinical support system via a Web interface validated by clinicians (e.g., surgeons). This information would be used when making decisions about the adjustment in clinical management plans for postoperative recovery.
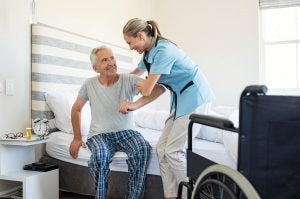
Clinical Implications of AI-Driven Measures of ADLs
Important clinical implications of improving the utility of AI-driven ADL measures for surgical patients are discussed below.
AI Can Yield Clinically Important Data That Can Inform Clinicians
AI-Driven ADL Measures Can Help Surgical Patients Recover at Home After Surgery
Older surgical patients have heterogeneous health conditions, and their postoperative recovery depends highly on individual factors. Technology-enabled ADL measures can provide personalized, continuous, and automated monitoring of ADLs in the home environment, monitor normal postoperative progression in recovery, detect abnormal progression and behaviors, and enable early mitigation to prevent adverse events and avoidable readmissions.32 AI-driven measures can be used to detect individual-specific ADLs with varying sensitivity to provide a basis for clinicians when evaluating whether an individual is able to recover at home safely and independently or needs more help in the form of in-house caregivers or alternatively is anticipated to require disposition to a specialized nursing facility for postoperative rehabilitation.
AI-Driven ADL Measures Can Help Surgical Patients Recover at Home After Surgery
Notably, one-third of older adults receiving home-care services report a fear of adverse events, which further restricts their performance of ADLs.33 As part of the recuperative process, management of ADLs in the home after surgery (e.g., with home physical therapy and home aide services) is well established. AI-driven ADL measures and monitoring systems can ease such fears, enhance functional recovery, and improve functional outcomes by shifting from traditional diagnostic ADL assessment to ecologically valid, personalized, and continuous measures of everyday function after surgery.
AI-Driven ADL Measures May Be Cost-Effective
AI technology can be costly but may be cost-effective if it facilitates home recovery over admission to a post-acute care facility, as shown in the innovation matrix.
Depending on the procedure, 40% to 99% of older patients use post-acute care after surgery.
Furthermore, post-acute care costs are high, ranging from $3,871 to $8,215, with inpatient rehabilitation facilities having the highest cost and in-home environment care having the lowest cost.,,
Depending on the procedure, 40% to 99% of older patients use post-acute care after surgery.
Furthermore, post-acute care costs are high, ranging from $3,871 to $8,215, with inpatient rehabilitation facilities having the highest cost and in-home environment care having the lowest cost.,,
Fortifying postoperative home discharges with AI-driven ADL monitoring and timely interventions can support patients’ choices to recover in their homes, can lower the cost of post-acute care, and might be an efficient and cost-effective option.
The benefits of time efficiency and continuous data capture could exceed the costs of the technologies.
Financial and human costs related to the limitations of current ADL measures, such as measurement errors, undetected functional decline, and missed opportunities to intervene for the purpose of maintaining independence should also be considered.
The benefits of time efficiency and continuous data capture could exceed the costs of the technologies.
Financial and human costs related to the limitations of current ADL measures, such as measurement errors, undetected functional decline, and missed opportunities to intervene for the purpose of maintaining independence should also be considered.
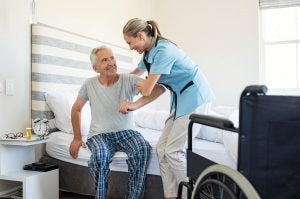
AI-Driven ADL Measures Are Not Yet Appropriate for All Activities, but Advanced Technology May Alleviate Some Privacy Concerns
Privacy and security are of the highest importance for AI-driven ADL measures, and concerns about 24–7 monitoring and their appropriateness in certain settings, unintended negative consequences, and legal liabilities exist.26 The deployment of AI models that poorly understand the targeted users’ needs and objectives is an obstacle to the use of AI-driven ADL measures. In the value-proposition canvas, we confirm the limited available technology that can be used to measure certain activities, such as toilet use (e.g., perineal care) and grooming (e.g., cleaning feet and nails). Nevertheless, multiple reliable technology options exist to detect norms and anomalies related to other basic activities, including dressing and bathing. Utilizing advanced technology may help to address privacy concerns while capturing important ADLs. Privacy protection is discussed in detail below.
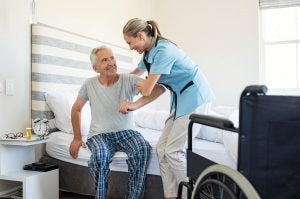
Potential Challenges in the Implementation of the Home-Recovery Surgical Care Model and Strategies to Overcome Challenges
Patients
Privacy Protection and Data Security
Privacy protection and data security are perpetual concerns for patients and their caregivers. We present a few means to address this concern.
First, using technology that protects privacy and de-identifies and encrypts data might be a partial solution to this problem. One example of such technology involves the use of stick figures — for example, when an individual is in the bathroom or wandering around in various stages of undress, anyone reviewing the video would only see a stick figure moving and interacting with the surrounding environment — and switching from video to audio and time in the privacy of the home (e.g., bathroom and toilet). Other examples include edge computing and cryptography-based encryption. Edge computing stores, computes, and analyzes data at the source of the data, rather than in a centralized cloud server. Cryptography-based encryption, such as identity-based encryption, utilizes an arbitrary unique identity as a digital signature to authenticate, encrypt, transmit, and decrypt data.,
Trust
Trust lies at the center of successful and sustainable implementation of an AI-based home-recovery surgical care model that aims to (1) reduce the hours that people spend collecting data, and (2) produce cost savings while maintaining or improving safety. Patients tend to view AI technology as less accountable,and less adaptable to unique needs than clinicians. Patients also might be uncomfortable with the collection of their private data and distrust the results that AI technology generates. AI-enabled ADL measures emphasizing personalized assessments and transparent explanations about innovative technology are some of the ways to gain patient trust. Such trust is necessary because patients must embrace and be willing to consent to the use of the technology for their care.
Clinicians
Trust
Provider trust in AI-driven measures and critical thinking about AI-generated results are necessary to promote the use of a clinical support system based on these measures and to translate this care model effectively into clinical practice.
While the evidence and value of the AI-driven ADL measures discussed above are crucial to acceptance, the implementation of the proposed home-recovery model of surgical care is a collective process.
Peer Pressure
When adopting innovations, clinicians often place greater value and trust in the perspectives and experience of their peers (i.e., fellow clinicians) as compared to the industry producing the innovative technology.51 While organizations may promote innovation as a way of attracting patients with cutting-edge technology, the influence that clinicians have on one another is greater than the influence that such organizations have on clinicians.51 The successful implementation of the proposed care model could be impacted by its demonstration and validation in the clinical setting, followed by the encouragement of open feedback to reflect the experience of frontline clinicians with the real-world workflow. The use of technology as a tool to support clinicians’ decisions also may prevent clinicians from perceiving it as a threat to their autonomy,37 thereby improving perceptions of the technology itself.
Health Care Systems
Compatibility Networks and Communications Leadership Engagement
To adopt this care innovation successfully, leadership engagement is required to build an AI-competent workforce, provide a help desk to support staff, and create a new role when mapping out the workflow for a home-recovery care model. Education and training are also essential to build an AI-competent workforce. The skill set required for an AI-enabled care model may differ from that of existing clinical teams. Staff will require skills in curating swathes of data, analyzing summaries of abnormal events, and escalating communication with surgeons and other clinicians for timely intervention and care plan modification. Critical thinking will be crucial for clinicians when using AI-generated results and making clinical judgments. Various training courses need to be developed to meet varying levels of competencies and clinicians’ learning curves. Providing continuing education credits for eligible staff members might provide an opportunity to leverage training for clinicians and other staff who will play pivotal roles in the successful implementation of this care model. A home-recovery surgical care model using AI-enabled monitoring of daily activities also requires the creation of new roles to coordinate AI system installations preoperatively and to conduct home safety evaluations.
The use of multidimensional AI data is promising for the measurement of many ADLs. For others, further advances in technology are necessary before AI-driven measurement can occur.
External Policies and Incentives
There is uncertainty about reimbursement for the use of AI technologies. Clinicians may use remote and continuous monitoring tools, but the current payment system may not necessarily pay for the use of these technologies because they are implicitly included in physical visits. However, a window of opportunity is open for payment reform that incentivizes the adoption of a home-recovery surgical care model using AI technology. The Covid-19 pandemic has exponentially increased the use of telehealth, including remote patient monitoring, and has paved the way for payment reform for remote patient monitoring. The Centers for Medicare & Medicaid Services have implemented payment models for remote patient monitoring, which includes payment for clinicians and other staff to review data, complete initial setup, provide patient education on equipment, manage care, and remotely communicate with patients. This payment applies to the use of AI-enabled remote monitoring devices that meet the U.S. Food and Drug Administration’s definition of a medical device.-
Technology Developers
Adaptability
As AI technology is more widely adopted over time in various settings, it is essential to keep the core components of the home-recovery surgical care model proposed above, including the establishment of a workflow that ensures ADL monitoring during the preoperative and postoperative periods. However, the design of the clinical support-system interface and the data presented on the dashboard can be adapted, tailored, and refined to meet needs at the clinician and organizational levels.
Trialability
A small-scale pilot of the care model in an organization is key to building user trust in its features and will give developers time to refine the model based on clinician and patient feedback before it is expanded to multiple health system settings.
Processes
Engagement
Stakeholder engagement at an early stage is vital for the successful implementation of the proposed care model. Engaging key stakeholders entails their involvement in priority-setting as well as evaluation of the model’s initial acceptability, education, and training.
Reflecting and Evaluating
Iterative collaboration between frontline users and developers (in addition to open feedback about progress and experience) is important to promote shared learning throughout the implementation process and to enable developers to modify the system accordingly.
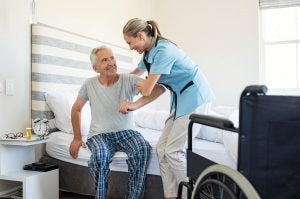
Enhance Home Recovery After Surgery Utilizing AI
AI-driven ADL measurement approaches can detect functional changes or critical events for surgical patients in a continuous and timely fashion.
The use of multidimensional AI data is promising for the measurement of many ADLs.
For others, further advances in technology are necessary before AI-driven measurement can occur.
The use of multidimensional AI data is promising for the measurement of many ADLs.
The AI-driven ADL measurement approaches embedded in a well-thought-out and well-implemented surgical care model could increase the percentage of patients who can safely recover in the comfort of their homes after surgery.
The care model using innovative AI-driven ADL measurement further provides important opportunities for clinicians (e.g., surgeons, nurses, physical therapists, and primary care physicians) to address individual patients’ needs regarding ADLs within the window for timely initiation and maintenance of care.
Such a surgical care model also could reduce post-acute rehabilitation costs for the health care system.
The care model using innovative AI-driven ADL measurement further provides important opportunities for clinicians… to address individual patients’ needs regarding ADLs within the window for timely initiation and maintenance of care.
Such a surgical care model also could reduce post-acute rehabilitation costs for the health care system.
About the authors
Kyung Mi Kim, PhD, RN
- Postdoctoral Scholar, Clinical Excellence Research Center, School of Medicine, Stanford University, Stanford, California, USA
Maria Yefimova, PhD, RN
- Lead Nurse Scientist, Center for Nursing Excellence and Innovation, UCSF Health, Stanford, California, USA
Feng Vankee Lin, PhD, MB, RN
- Clinical Professor, Department of Psychiatry and Behavioral Sciences, School of Medicine, Stanford University, Stanford, California, USA
Jeffrey Kyle Jopling, MD
- Assistant Professor, Department of Surgery, School of Medicine, Johns Hopkins University, Baltimore, Maryland, USA
Erik Nathan Hansen, MD
- Associate Professor, Department of Orthopaedic Surgery, School of Medicine, University of California, San Francisco, USA
Originally published at https://catalyst.nejm.org on June 8, 2022.