the health strategist
institute for strategic health transformation
& digital technology
Joaquim Cardoso MSc.
Chief Research and Strategy Officer (CRSO),
Chief Editor and Senior Advisor
September 25, 2023
One page summary
What is the message?
Artificial intelligence (AI) has shown remarkable capabilities in interpreting medical images, surpassing human expertise in detecting subtle features.
While AI has been predominantly used for image analysis, it’s now evolving into a multimodal AI, combining text and speech capabilities with image analysis.
This expansion paves the way for AI to revolutionize various aspects of medicine.
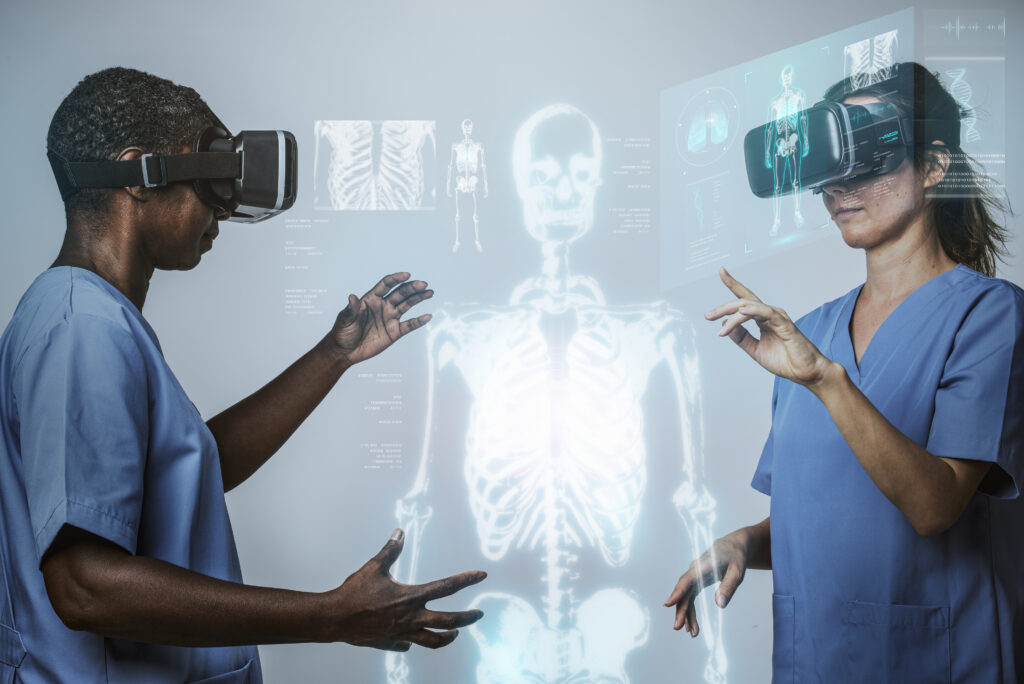
Key Points:
1. The Power of “Machine Eyes”:
AI, despite lacking physical eyes, has demonstrated its prowess in accurately interpreting medical images like x-rays, CT scans, MRI scans, pathology slides, and retinal photos. It can reveal hidden physiological information such as blood pressure, glucose control, and disease risks that may not be apparent to humans.
2. Beyond Image Interpretation:
AI’s role in medicine is evolving beyond image analysis. For instance, AI can analyze electrocardiograms to provide insights into age, sex, anemia, and other conditions. Additionally, it can analyze pathology slides to determine the site of origin, genetic mutations, and prognosis.
3. Multimodal AI:
AI is transitioning from unimodal (image-only) tasks to multimodal, where it can process text, audio, speech, and images. The development of transformers, such as GPT-4, with over 1 trillion parameters, has enabled AI to work with various forms of data.
4. Diverse Health Data:
Multimodal AI can analyze diverse health data, including anatomy through imaging, genetics, microbiome, immunome, electronic health records, exposome (environmental factors), and social determinants. It can also incorporate the vast corpus of medical knowledge.
5. Applications:
Multimodal AI has numerous potential applications in healthcare, including virtual health assistants for preventive care, remote monitoring equivalent to intensive care units, digital twins for personalized treatment, and pandemic surveillance for real-time risk assessment.
6. Challenges and Barriers:
Challenges include overconfidence in AI responses, biases, privacy concerns, model deterioration, regulatory approval criteria, resistance to change in medical practice, and the need for robust evidence of benefit.
7. Future Possibilities:
The convergence of powerful computing and vast self-supervised learning data lays the foundation for groundbreaking medical applications. Multimodal AI may lead to highly accurate and individualized approaches to healthcare, including virtual health assistants and innovative home-based healthcare models.
In conclusion
AI in medicine is entering a new era with multimodal capabilities. It holds immense potential to transform healthcare by leveraging diverse data sources and providing personalized, data-driven insights for both preventive and clinical care. However, addressing challenges and ensuring responsible AI use remain critical as this technology evolves.
DEEP DIVE

This summary was written based on the article “As artificial intelligence goes multimodal, medical applications multiply”, published by Science and written by Eric J. Topol, on Septmeber 15, 2023.
To read the full article, access https://www.science.org/doi/full/10.1126/science.adk6139