Chapter 2 — Part 1: of “Transforming healthcare with AI”
EIT Health
March 3, 2020
This is an excerpt of the report “ Transforming healthcare with AI. Impact on the workforce and organisations”, published in 2020 by EIT Health. Survey of 175 healthcare professionals, health investors and AI start-up founders and executives
Executive Summary
by Joaquim Cardoso MSc.
Chief Editor of “The Health Revolution” Institutes
AI Health Unit @ Digital Health Institute
March 24, 2022
Introduction
- However, higher quality data available in greater volumes and more connected than ever, combined with more computing power and advances in algorithms, is enabling a wave of innovation.
- Consequently, we see venture capital (VC) firms and tech giants increasing their funding of AI in healthcare, clinical trials testing the benefits of applying AI and, since 2017, a series of US Food and Drug Administration (FDA) approvals for AI.[1]19
- Despite all the noise, the effect to date on healthcare organizations has been limited.
How recent advances have made AI in healthcare a reality
- Improvements in data, processing power and algorithms are rapidly changing what is feasible.
- The scope and quality of healthcare data produced and the potential to link datasets are opening new possibilities.
- By the 1950s, medical knowledge had doubled in about 50 years. In 2020, the volume of medical knowledge will double in 73 days.[4]23
- Both the quality and consistency of data are improving as more data are machine generated.
- However, the lack of linked and comprehensive datasets limit AI’s potential, leading to Silicon Valley investments in creating distinctive linked datasets.
- The ability of algorithms to interpret data has transformed over the past decade. Dramatic improvement is also occurring in NLP.
- Similar improvements are being made in reading, sound and video comprehension, translation and the ability to answer questions with an algorithm trained on text innovation
- Advances in deep learning mean that algorithms can generate layers of abstract features that enable computers to recognise complicated concepts. This enables them to learn discriminative features automatically and approximate highly complex relationships.
- Explainable and causal AI is another area of advancement.
- AI advancements have generated significant excitement around the world and many are being fast-tracked and adopted into healthcare delivery.
Implementation around the world
- AI in healthcare — and beyond — has become an important focus for policy makers and investors, with implications for research and further pipeline development.
Government action
- Governments around the world have, in recent years, published national strategy documents on AI that set out their aspirations. The EU itself has published a wealth of reports on the topic since 2018. In addition, there are a series of initiatives underway…
- Governments are not just developing strategies, they are investing strategically in AI, with large investments in multidisciplinary AI research centres in countries as diverse as the US, Russia, China and Qatar.
The case of Israel
- In Israel, for example, the government announced a $264 million initiative in 2018 to combine national digital medical records in a unified system to maximise the usefulness of data for AI/advanced analytics, and ensure healthcare can be made cheaper, more effective and more personalised.
- The Israeli government has long encouraged partnerships and cooperation between foreign and domestic business, Israeli startups, research institutes, hospitals and Health Maintenance Organisations (HMOs).
- It has contributed to the birth of a vibrant and productive digital and AI ecosystem, with Israeli digital-health companies raising more than $400 million, and many now expanding internationally.[15]39
Private-sector investments
- The private sector is also playing a leading role — not just AI innovators and startups, but established payors/health insurers and healthcare providers, leading pharmaceutical and MedTech companies, digital natives and telecommunications companies …
Investors are also leading the way.
- VC funding into AI in healthcare has been rising steadily: by December 2019, the 50 largest cumulative investments had reached $8.5 billion, with the top five companies receiving more than 40 percent of the total funding and the top ten, 58 percent . When split by region,we see VC funding increased in the US and Asia in 2017, and in Europe in 2018.
A diverse research pipeline
- Just as VC firms broaden their geographic scope, healthcare AI research studies and clinical trials also reflect a more diverse picture.
- Initially, the US dominated AI-related research, but China now outpaces the rest of the world in the number of healthcare research studies with an AI focus, followed by the US and Europe, which taken collectively is on par .
- China is emerging as a leader in healthcare AI, with large datasets, strong government support, a large pool of tech talent and an innovation-friendly environment.
- Chinese tech leaders and conglomerates have evolved as major healthcare players. They aim to change the paradigm for access to healthcare and address system inefficiencies through customer-centric solutions, e.g., scheduling appointments, e-triage, access to primary care.
The view from Europe
- Europe is making significant progress in key areas. The EU has sought to develop a pan-European approach, strategy and plan for AI, including in healthcare.
- It has established working groups with a balance of expert input and stakeholder representation and sought to develop “the EU way” for AI, focusing on transparent, ethical and trustworthy applications.
- Private investment in Europe is increasing fast, albeit from a smaller base than the US, and EU countries have significant research strengths, with established digital and innovation clusters and collaborations at the regional and pan-European level and — as we saw above — AI-related healthcare research and trials are on a par with the US.
- At the same time, the pace of change reflects the complexity of aligning the approach and aspirations of diverse Member States and other stakeholders.
- Valuable datasets are not linked, with critical data-governance, access and security issues not yet addressed, delaying further AI adoption.
- European investment and research in AI are strong when grouped together but fragmented at the country or regional level.
The opportunity for Europe, and barriers
- EU public healthcare systems hold a treasure trove of healthcare information at the population level that, if linked, could provide distinctive advantages in speeding up AI adoption and impact in healthcare.
- There is a significant opportunity for EU health systems, but AI’s full potential remains to be explored and the impact on the ground remains small.
- For instance, despite great interest in AI among the survey group, 44 percent of healthcare professionals have yet to experience deployment or development of AI solutions in their organisation
- Where AI is being used in European healthcare today, just over one in five of the healthcare professionals surveyed mentioned its application in diagnostics (imaging, pathology, sequencing), one of the earlier uses of AI in healthcare, with clinical decision making and data management the next most common uses (Exhibit 2.6).
Main use cases in Europe
Diagnostics is the most common field for AI solutions in healthcare today, in Europe.
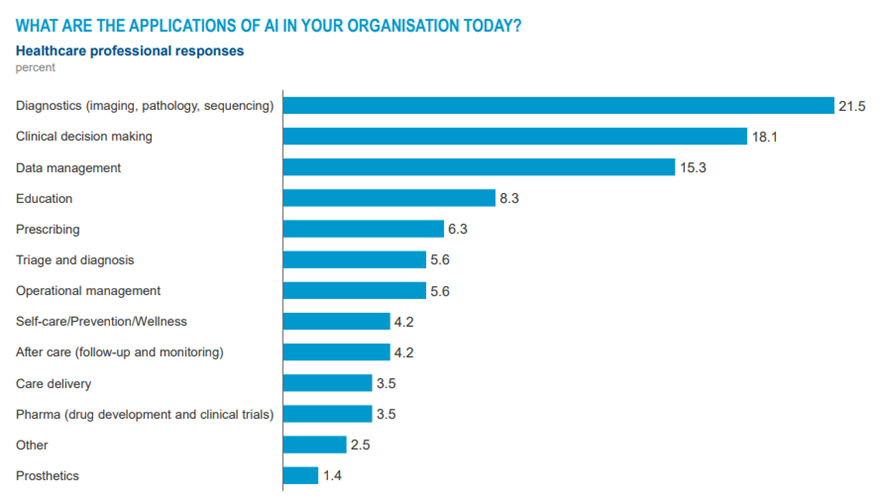
- Startup executives, on the other hand, put more emphasis on consumer-facing solutions, such as self-care, prevention and wellness, or triage (including e-triage solutions) and diagnosis.
- This partly reflects a consumer orientation and a focus on empowering individuals to take more care of themselves, but also reflects an expectation of faster adoption and the potential to scale AI through this route (Exhibit 2.7).
Startups are focusing their efforts on clinical decision-making solutions
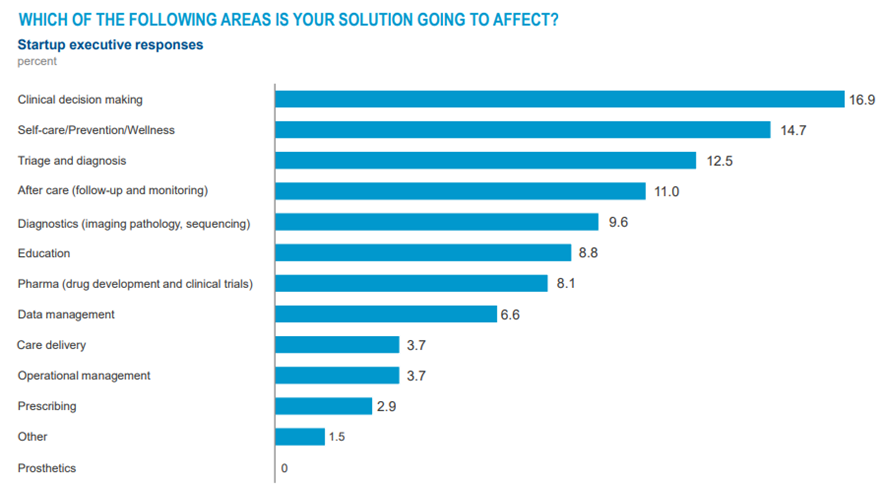
- More than two-thirds are invested in one or two AI in healthcare solutions, and in almost half the cases, the average ticket size for these investments is below €1 million.
- Overall, the progress of AI in Europe would appear marred by fragmentation, both in terms of investment and the pooling of critical resources to develop, test and implement — but with significant potential to scale based on the strengths of the European health systems, and of their practitioners, researchers and investors.
ORIGINAL PUBLICATION (full version of Chapter 2)
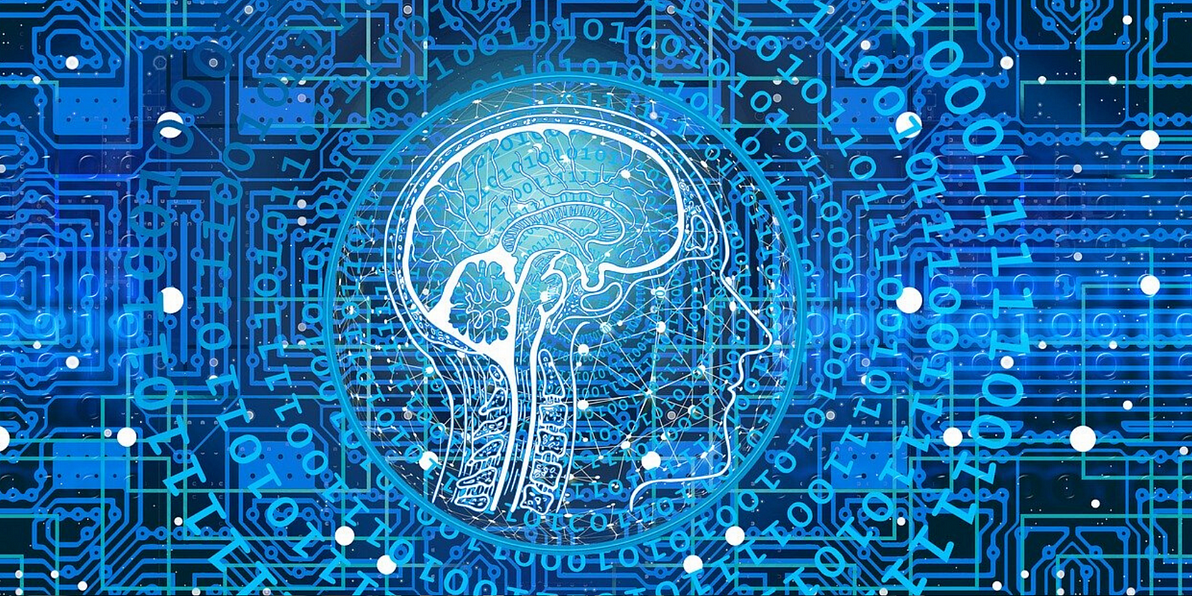
Introduction
The prospect of computers deriving insights from data to transform efficiency and improve operational and clinical decision making has excited computer scientists for decades, but the idea initially raised sceptical eyebrows among physicians.
However, higher quality data available in greater volumes and more connected than ever, combined with more computing power and advances in algorithms, is enabling a wave of innovation.
Consequently, we see venture capital (VC) firms and tech giants increasing their funding of AI in healthcare, clinical trials testing the benefits of applying AI and, since 2017, a series of US Food and Drug Administration (FDA) approvals for AI.[1]19
Despite all the noise, the effect to date on healthcare organizations has been limited.
Of the healthcare professionals we surveyed, chosen for their interest in healthcare innovation and AI, 44 percent had never been involved in the development or deployment of an AI solution in their organisation. When we asked to what extent AI affects the healthcare workforce today in Europe on a scale of one to five (1=high impact), most responses hovered around three (“somewhat”).
This chapter sets out how we define AI in healthcare, considers why its relevance is increasing around the world, and then looks in detail at a series of examples of how AI is being applied in healthcare.
2.1 What do we mean by AI in healthcare?
The term “artificial intelligence” has been given many definitions, and applications have been included or excluded according to the definition used.
As we said in the introduction, our working definition describes AI as “the capability of a computer programme to perform tasks or reasoning processes that we usually associate with intelligence in a human being.”[2] 20
Our scope includes applications that affect care delivery, including both how existing tasks are performed and how they are disrupted by changing healthcare needs or the processes required to address them.
It also includes applications that work across areas enabling, augmenting or improving healthcare delivery, from healthcare operations to population-health management and broader healthcare innovation (e.g., R&D).
This definition is deliberately broad; it includes the application of rules-based systems as well as cutting-edge methodologies that include classic machine learning, representation learning and deep learning.
Examples of AI applications would include NLP, image analysis and predictive analytics based on machine learning.
We apply such a broad definition for two reasons.
First, we believe the wider use of rules-based systems will be one of the greatest changes to delivering care in the medium term, enabled by richer data and improved computing.
Second, we see AI in healthcare as a spectrum, where encoding clinical guidelines or existing clinical protocols often provides a starting point, which then can be augmented by models that learn from data and demonstrate the distinctive properties of AI:
· autonomy (the ability to perform tasks in complex environments without constant user guidance) and
· adaptivity (improving performance by learning from experience).[3]21
This transition from rules drawn up by experts to systems that learn from data is exemplified in healthcare AI today by applications to triage patients, applications to support clinical decision making, and by robots that restock a nurse’s station automatically while using AI to navigate their environment.
We have not included in our working definition digitisation efforts that do not generate insights, such as the transition from paper-based to digital patient charts or the introduction of single-sign-on, although these may be crucial enablers of some of the approaches described.
Nor do we consider digital approaches driven purely by improved connectivity, such as video consultations.
Applications beyond the scope of care delivery, such as claims processing, are also not considered.
For the purpose of this report, AI therefore comprises a spectrum of solutions that start with rules-based approaches, but increasingly introduce the capacity to learn by training a specific task on large datasets.
Exhibit 2.1 illustrates this point, mapping the key learning components of AI and what is and is not included in a “pure AI” solution, while showcasing the growing overlap between fields, e.g., in AI and automation-enabled solutions such as self-driving cars and broader “smart automation” applications.
Exhibit 2.1 — AI exists on a spectrum, with algorithms displaying increasing learning capabilities
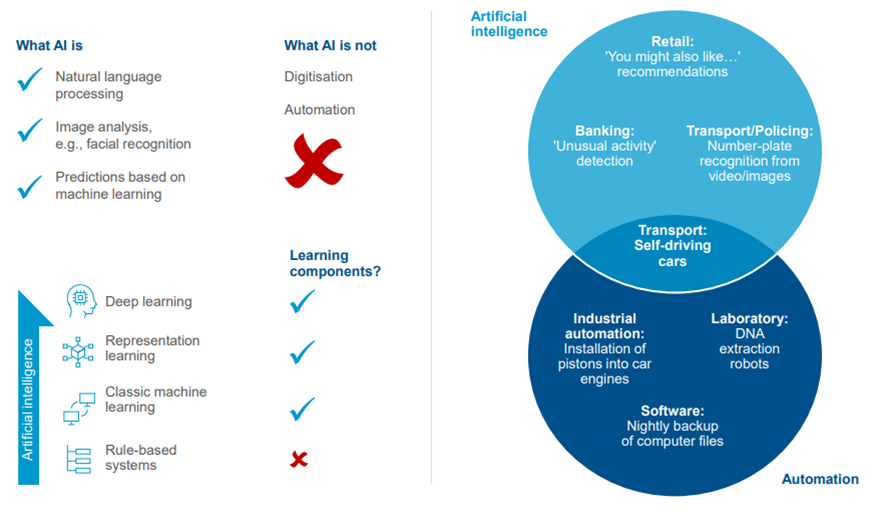
2.2 How recent advances have made AI in healthcare a reality
Improvements in data, processing power and algorithms are rapidly changing what is feasible.
The scope and quality of healthcare data produced and the potential to link datasets are opening new possibilities.
By the 1950s, medical knowledge had doubled in about 50 years. In 2020, the volume of medical knowledge will double in 73 days.[4]23
Both the quality and consistency of data are improving as more data are machine generated.
Wearables produce new types of longitudinal data.
Genomics data are becoming more accessible as the costs of sequencing and bioinformatic techniques have plummeted (US company Illumina announced the $1,000 genome in 2014 and promises a $100 genome sequencing within a decade[5]24).
One application of AI is improving image acquisition and reconstruction, which in turn improves that potential for the use of data produced for clinical decision support.
The increased adoption of electronic health records (EHRs) and personal healthcare records that can connect longitudinal data and genomics data provides further possibilities for AI to grow.
However, the lack of linked and comprehensive datasets limit AI’s potential, leading to Silicon Valley investments in creating distinctive linked datasets.
Verily’s Project Baseline project, for example, aims to “map human health and disease“ by building a detailed dataset from 10,000 participants over four years and the Healthy Nevada Project aims to enrol 250,000 participants to generate combined genomic, EHR and environment data.[6]25 Europe may take a different tack, given that its public healthcare systems could provide datasets of unique breadth and depth, creating a distinctive advantage in using AI to improve healthcare.
“Healthcare leaders today can steer the next wave of progress in healthcare, by harnessing advances in data science to generate valuable insights from the large, complex data sets accruing in health systems.” WISH Report, 2018[7] 22
The ability of algorithms to interpret data has transformed over the past decade.
In 2015, algorithms overtook humans in visual recognition in the ImageNet Challenge Large Scale Visual Recognition Competition, improving from an error rate of 28 percent in 2010 to 2.2 percent in 2017, versus a typical human error rate of about 5 percent.[8]26
Dramatic improvement is also occurring in NLP: in 2019, algorithms overtook human performance on General Language Understanding Evaluation, a metric for measuring language understanding.[9]27
Similar improvements are being made in reading, sound and video comprehension, translation and the ability to answer questions with an algorithm trained on text innovation: new solutions, for example, include neural networks (a form of AI) that can read scientific papers and provide a full summary.[10]28
Advances in deep learning mean that algorithms can generate layers of abstract features that enable computers to recognise complicated concepts (such as a diagnosis) by building on simpler ones that are accessible in the data (such as the colour of a pixel).
This enables them to learn discriminative features automatically and approximate highly complex relationships.
Such algorithms have been around since the 1940s, but the recent expansion of datasets and computational resources have enabled a series of breakthrough improvements that are now being applied to augment healthcare provision.[11] 29
Explainable and causal AI is another area of advancement.
A challenge of applying insights from deep learning is that abstracting features through multiple layers means it can be hard (if not impossible) to interpret why an algorithm reaches a specific conclusion.
Innovations in explainable and causal AI have sought to combine the benefits of the accuracy of deep-learning algorithms with visibility on the factors that are important to the algorithm’s conclusion in a way that is accessible to physicians and other practitioners.[12]30
For example, an algorithm assessing the chance of cancer would be more useful to physicians if it highlights the most relevant biomarkers used in making its assessment and the reference patients from a dataset that it has identified as most similar.
AI advancements have generated significant excitement around the world and many are being fast-tracked and adopted into healthcare delivery.
The following sections illustrate the latest view on implementation in different regions and specific use cases that show the potential of AI to transform care.
2.3 Implementation around the world
AI in healthcare — and beyond — has become an important focus for policy makers and investors, with implications for research and further pipeline development.
It is instructive to assess what various players in the sector are doing to drive AI in healthcare forward.
2.3.1 Government action
Governments around the world have, in recent years, published national strategy documents on AI that set out their aspirations.
The EU itself has published a wealth of reports on the topic since 2018.
In addition, there are a series of initiatives underway in areas such as health policy (e.g., regulation around medical devices, Health Technology Assessments, clinical trials, European reference networks, healthcare workforce); the digital agenda — a Europe “fit for the digital age”; digital health policy (digital transformation of health and care); the Digital Europe programme (on AI, digital skills, digital take-up, cybersecurity & trust, supercomputing); the Horizon Europe programme (including funding on R&D and innovation on health and ageing); as well as plans on AI, data and industrial-policy implementation (see sidebar “Europe and AI in healthcare — the EU’s strategy for AI”).[13]31
Governments are not just developing strategies, they are investing strategically in AI, with large investments in multidisciplinary AI research centres in countries as diverse as the US, Russia, China and Qatar.[14]38
In Israel, for example, the government announced a $264 million initiative in 2018 to combine national digital medical records in a unified system to maximise the usefulness of data for AI/advanced analytics, and ensure healthcare can be made cheaper, more effective and more personalised.
The Israeli government has long encouraged partnerships and cooperation between foreign and domestic business, Israeli startups, research institutes, hospitals and Health Maintenance Organisations (HMOs).
It has contributed to the birth of a vibrant and productive digital and AI ecosystem, with Israeli digital-health companies raising more than $400 million, and many now expanding internationally.[15]39
2.3.2 Private-sector investments
The private sector is also playing a leading role — not just AI innovators and startups, but established payors/health insurers and healthcare providers, leading pharmaceutical and MedTech companies, digital natives and telecommunications companies, all making commitments, investments and announcements of plans to scale AI to improve healthcare.
Investors are also leading the way.
VC funding into AI in healthcare has been rising steadily: by December 2019, the 50 largest cumulative investments had reached $8.5 billion, with the top five companies receiving more than 40 percent of the total funding and the top ten, 58 percent (Exhibit 2.2). When split by region, Exhibit 2.3 shows how dramatically VC funding increased in the US and Asia in 2017, and in Europe in 2018.
The US dominates the list of VC deals in AI in healthcare, accounting for 221 deals from the start of 2010 to the end of 2019, followed by 108 in Europe and 76 in Asia.
Although the number of deals has doubled since 2015 in the US and Europe, there has been a five-fold increase in Asia over the same period.[16]40
BOX: Europe and AI in healthcare — The EU’s strategy for AI
On April 10, 2018 — Digital Day 2018 — EU countries signed the “Declaration of Cooperation on AI”, aiming to build a framework through which Member States can cooperate on areas ranging from AI’s impact on the labour market, sustainability and trustworthiness, to ethics and funding.[17]32
Following the European Council’s call to put forward a European approach to AI, the European Commission (EC) published its Communication on AI, setting out its strategic approach to AI and aiming to boost the EU’s technological and industrial capacity and AI uptake, prepare Europe for the socioeconomic changes associated with it and ensure an appropriate ethical and legal framework.[18]33
A second strategic document, the Coordinated Plan on AI (December 2018), outlines the European approach to AI and areas of implementation that will be supported by Member States.[19]34
The EC also established two working groups: the European AI Alliance, built around a diverse multistakeholder online platform and open to all members of society, and the High-Level Expert Group on AI (AI HLEG), which is advising the EC on short- and long-term challenges and opportunities arising from AI, acting as the steering group to the European AI Alliance and publishing its draft suggestions on the Alliance’s platform for stakeholder feedback.
The EU’s vision of itself as a leader in “ethical AI” is also reflected in the AI HLEG’s Draft Ethics Guidelines for Trustworthy AI (December 2018).[20]35
In terms of setting up a broader environment to enable digital- and analytics-led innovation in healthcare, the Communication on enabling the digital transformation of health and care in the Digital Single Market (April 2018) looks at how the meaningful use of digital innovation could promote health, prevent and control disease, help address patients’ unmet needs and make it easier for citizens to have equal access to high quality care.
This communication is the basis for the funding of health dataspace work under the Digital Europe Programme (2021–2027).[21]36
The Digital Europe Programme is an EU innovation and deployment programme focused on five areas: AI, digital skills, digital take-up, cybersecurity & trust, and supercomputing. Last, in terms of education, the Commission adopted a Digital Education Action Plan (January 2018) that includes 11 actions to support technology use and the development of digital competences in education.
In particular, Action 10 (on AI and analytics) defines how the Commission will pilot data analytics projects to predict the learning journeys and training requirements for future competence profiles.[22]37
Exhibit 2.2 — Since 2010, $8.5 billion of VC funding has been invested in the 50 best-funded AI companies in healthcare
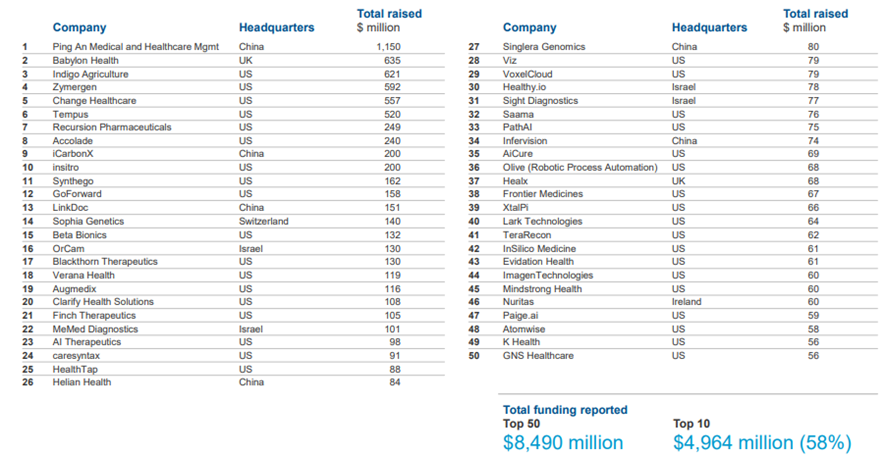
SOURCE: PitchBook Data, Inc., http://pitchbook.com/research-process
Exhibit 2.3 — The venture capital invested in AI companies in healthcare has grown significantly since 2015
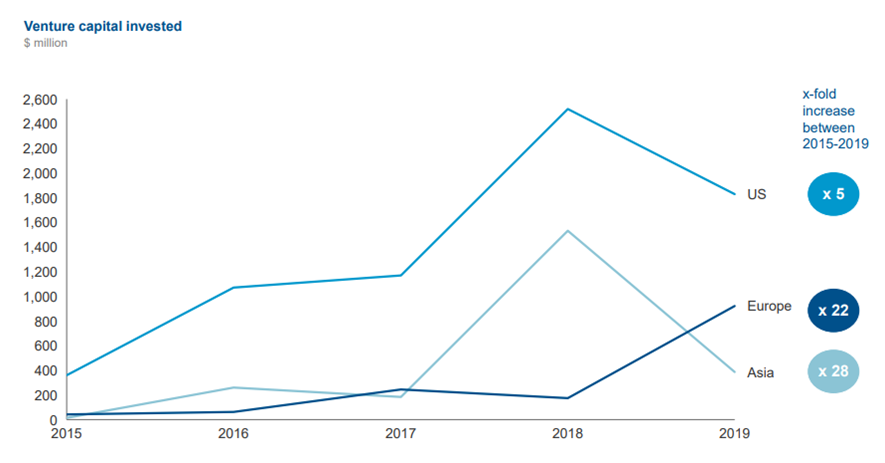
SOURCE: PitchBook Data, Inc., http://pitchbook.com/research-process; team analysis
2.3.3 A diverse research pipeline
Just as VC firms broaden their geographic scope, healthcare AI research studies and clinical trials also reflect a more diverse picture.
Initially, the US dominated AI-related research, but China now outpaces the rest of the world in the number of healthcare research studies with an AI focus, followed by the US and Europe, which taken collectively is on par (Exhibit 2.4).
Exhibit 2.4 — China has the largest number of healthcare research studies with an AI focus
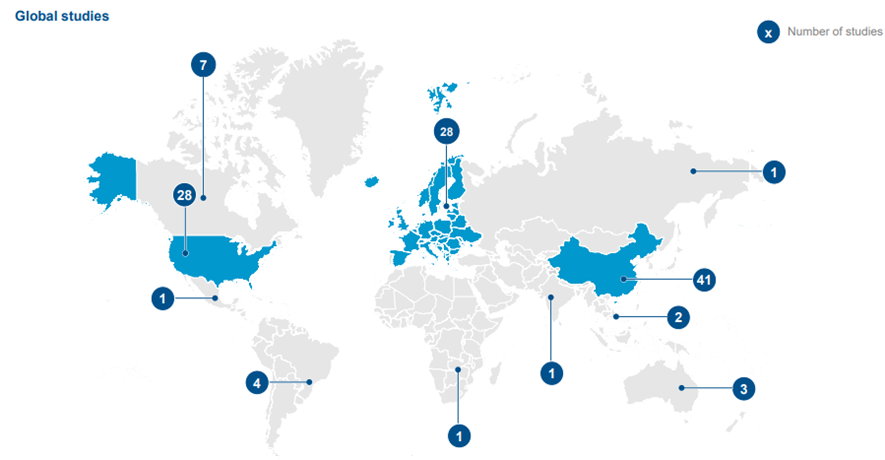
SOURCE: http://clinicaltrials.gov, as of December 16, 2019. Study types included in search results from Clinicaltrials.gov are interventional (clinical trials) and observational studies.
BOX: China and AI in healthcare
China is emerging as a leader in healthcare AI, with large datasets, strong government support, a large pool of tech talent and an innovation-friendly environment.
Interviewees also pointed out that in some areas, China’s healthcare system faces poor access to care, lack of awareness of treatment options, bottlenecks in patient referral and gaps in long-term disease management.
A particular challenge is access to primary care, especially in rural areas, and variability in quality. At the same time, Chinese consumers of all ages are aware of and broadly accept digital AI solutions in different areas, through self-pay models in China’s cashless landscape.
As Dr. Alexander Ng, from Tencent Healthcare, explains, “Making the leap to using AI in healthcare for the Chinese consumer will be easier than elsewhere. Everyone is used to having chatbots and AI in many services.”
The Chinese government has developed a range of policies showing its commitment to the use of AI in healthcare, setting the goal of becoming a global innovation centre by 2030 and laying out recommendations for the use of AI to improve population health.
In recent years, the government has also invested not only in academic collaborations, but also directly in innovative startups.
Chinese tech leaders and conglomerates have evolved as major healthcare players.
They aim to change the paradigm for access to healthcare and address system inefficiencies through customer-centric solutions, e.g., scheduling appointments, e-triage, access to primary care.
· Tencent uses its WeChat platform to engage and manage users of its healthcare services across the care continuum, aiming to diagnose and prevent a broad range of diseases.
It offers online AI-based triage and appointment scheduling, is building a WeChat-based physician community for patient management and has an AI imaging platform.
· Alibaba has used its e-commerce platform to set up an online business-to-consumer healthcare platform (e.g., over-the-counter drugs, plastic surgery), adding telemedicine, traceable barcodes for healthcare products, and the use of Alipay to pay for services.
· Ping An has used its insurance customer base and data to build a complete health ecosystem, connecting patients, payors, hospitals and other care providers. Ping An’s Good Doctor, its online health-management platform, is the largest globally, with more than 300 million users.[23]41
Such companies are now increasingly developing AI solutions, such as the AI-powered cloud doctor in Ping An’s One-Minute-Clinics in shopping centres, which registers medical history and symptoms and offers e-triage, diverting complex cases to physicians at Ping An’s network/call-centre.
It also offers access to 200 medicines within the clinic and home delivery through local pharmacies, creating an end-to-end primary access experience for its users.
2.3.4 The view from Europe
Europe is making significant progress in key areas. The EU has sought to develop a pan-European approach, strategy and plan for AI, including in healthcare.
It has established working groups with a balance of expert input and stakeholder representation and sought to develop “the EU way” for AI, focusing on transparent, ethical and trustworthy applications.
EU public healthcare systems hold a treasure trove of healthcare information at the population level that, if linked, could provide distinctive advantages in speeding up AI adoption and impact in healthcare.
Private investment in Europe is increasing fast, albeit from a smaller base than the US, and EU countries have significant research strengths, with established digital and innovation clusters and collaborations at the regional and pan-European level and — as we saw above — AI-related healthcare research and trials are on a par with the US.
At the same time, the pace of change reflects the complexity of aligning the approach and aspirations of diverse Member States and other stakeholders.
Valuable datasets are not linked, with critical data-governance, access and security issues not yet addressed, delaying further AI adoption.
European investment and research in AI are strong when grouped together but fragmented at the country or regional level.
There is a significant opportunity for EU health systems, but AI’s full potential remains to be explored and the impact on the ground remains small.
“Europe has large public healthcare systems, which should put us at an advantage regarding data, compared to nations with more fragmented systems.“
Julián Isla Gómez, Microsoft EMEA
These themes were echoed in both the interviews and the survey of EU-based stakeholders, which provides a view from the front line of the AI applications present in European healthcare today and where investors and startups who work in Europe see the most potential.
For instance, despite great interest in AI among the survey group, 44 percent of healthcare professionals have yet to experience deployment or development of AI solutions in their organisation (Exhibit 2.5).
Exhibit 2.5 — Many healthcare professionals have had limited exposure to developing or deploying an AI solution
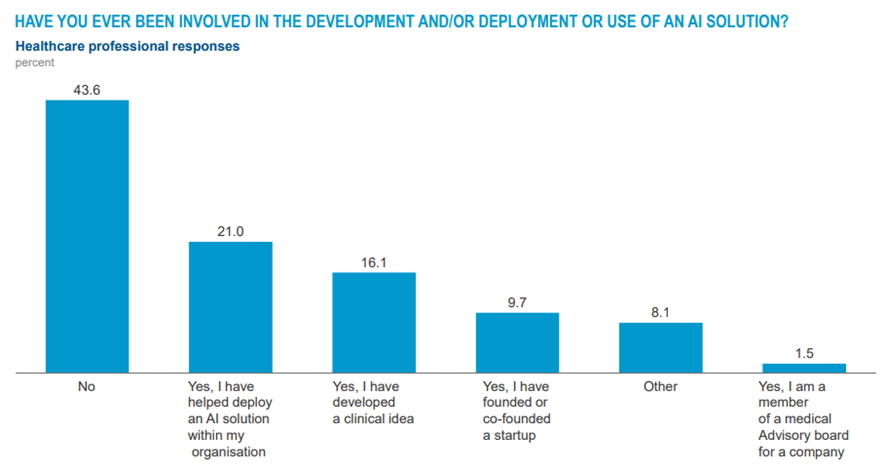
SOURCE: Survey of healthcare professionals, healthcare investors and startup executives across European countries, conducted in December 2019 and January 2020
Where AI is being used in European healthcare today, just over one in five of the healthcare professionals surveyed mentioned its application in diagnostics (imaging, pathology, sequencing), one of the earlier uses of AI in healthcare, with clinical decision making and data management the next most common uses (Exhibit 2.6).
Exhibit 2.6 — Diagnostics is the most common field for AI solutions in healthcare today
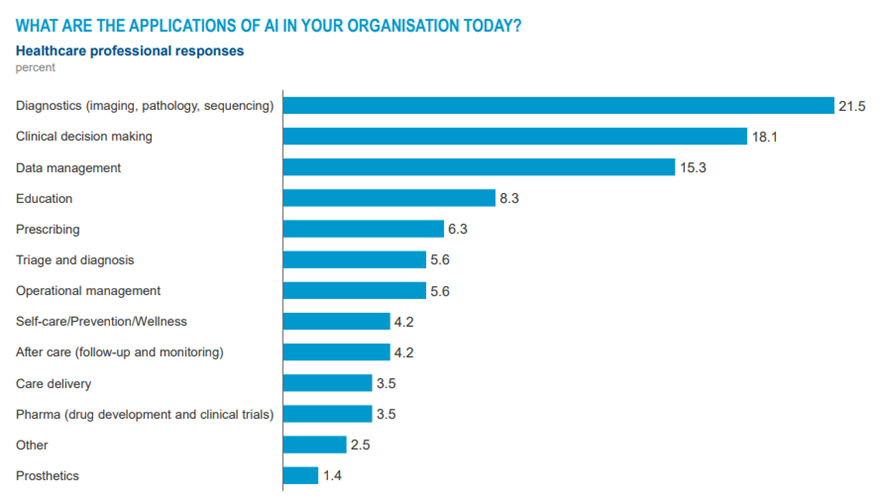
SOURCE: Survey of healthcare professionals, healthcare investors and startup executives across European countries, conducted in December 2019 and January 2020
Startup executives, on the other hand, put more emphasis on consumer-facing solutions, such as self-care, prevention and wellness, or triage (including e-triage solutions) and diagnosis.
This partly reflects a consumer orientation and a focus on empowering individuals to take more care of themselves, but also reflects an expectation of faster adoption and the potential to scale AI through this route (Exhibit 2.7).
Exhibit 2.7 — Startups are focusing their efforts on clinical decision-making solutions
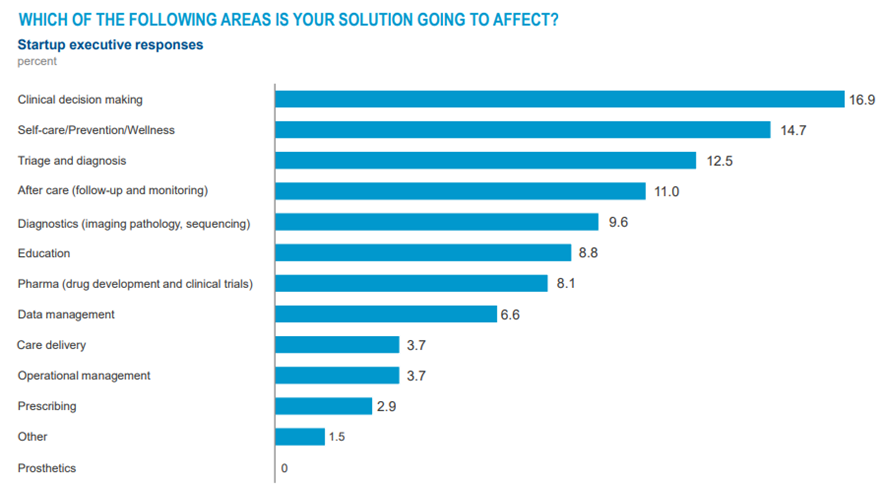
SOURCE: Survey of healthcare professionals, healthcare investors and startup executives across European countries, conducted in December 2019 and January 2020
The investors surveyed were focused mainly on Europe and most are already investing in AI in healthcare, however, for just under three-quarters, the investments represent less than 20 percent of their total portfolio.
More than two-thirds are invested in one or two AI in healthcare solutions, and in almost half the cases, the average ticket size for these investments is below €1 million (Exhibit 2.8).
Exhibit 2.8 — The average investment in healthcare AI made by investors surveyed is less than €1 million
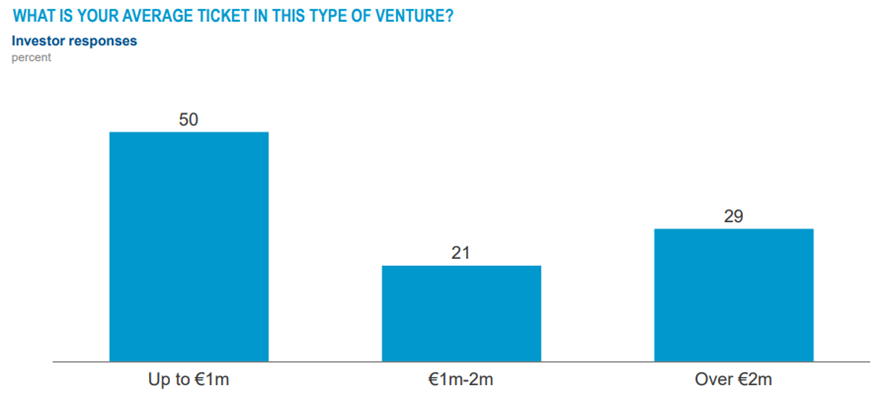
SOURCE: Survey of healthcare professionals, healthcare investors and startup executives across European countries, conducted in December 2019 and January 2020
Overall, the progress of AI in Europe would appear marred by fragmentation, both in terms of investment and the pooling of critical resources to develop, test and implement — but with significant potential to scale based on the strengths of the European health systems, and of their practitioners, researchers and investors.
This is an excerpt of the report “ Transforming healthcare with AI. Impact on the workforce and organisations; EIT Health and McKinsey & Company, (2020). Survey of 175 healthcare professionals, health investors and AI start-up founders and executives
References
[1] FDA approvals for smart algorithms in medicine in one giant infographic, The Medical Futurist, June 6, 2019, https:// medicalfuturist.com/fda-approvals-for-algorithms-in-medicine/.
[2] Artificial intelligence: Potential benefits and ethical considerations, European Parliament Legal Affairs briefing, Policy Department C: Citizens’ Rights and Constitutional Affairs, PE 571.380, 2016, http://www.europarl.europa.eu/RegData/ etudes/BRIE/2016/571380/IPOL_BRI(2016)571380_EN.pdf.
[3] A simple and clear definition of AI offered by the University of Helsinki and Reaktor, “Elements of AI”, https://course. elementsofai.com/.
[4] Mesko, B., “A Guide to Artificial Intelligence in Healthcare”, The Medical Futurist, 2019; P. Densen, “Challenges and opportunities facing medical education”, Transactions of the American Clinical and Climatological Association, vol. 122, pp. 48–58, 2011.
[5] Liat Clark, “Illumina announces landmark $1,000 human genome sequencing”, Wired, January 15, 2014, https://www. wired.co.uk/article/1000-dollar-genome.
[6] https://www.projectbaseline.com/; https://healthynv.org/.
[7] Colclough, G. et al., Harnessing data science and AI in healthcare from policy to practice, World Innovation Summit for Health, 2018 http://www.wish.org.qa/wp-content/uploads/2018/11/IMPJ6078-WISH-2018-Data-Science-181015.pdf.
[8] S. Dodge and L. Karam, “Understanding how image quality affects deep neural networks,” International Conference on Quality of Multimedia Experience (QoMEX), 2016 http://image-net.org/challenges/LSVRC/2010/results; http://image-net. org/challenges/LSVRC/2017/results.
[9] Daniel Nelson, “Baidu Beats Out Google And Microsoft, Creates New Technique For Language Understanding”, Unite. AI, 28 December 2019, https://www.unite.ai/baidu-beats-out-google-and-microsoft-creates-new-technique-for-languageunderstanding/.
[10] “Can science be automated?”, ScienceDaily, April 2019, https://www.sciencedaily.com/ releases/2019/04/190418105730.htm.
[11] Hinton, G., Deep learning — a technology with the potential to transform health care. Jama, 2018, 320(11), pp.1101- 1102; Gottesman, O., et al., “Guidelines for reinforcement learning in healthcare”. Nature Medicine, 2019, 25(1), pp.16–18.
[12] Holzinger, A. et al., “What do we need to build explainable AI systems for the medical domain?”, arXiv:1712.09923, 2017.
[13] US reports include, National artificial intelligence research and development strategic plan: 2019 update, a report by the Select Committee on AI of the National Science and Technology Council, June 2019; Proposed regulatory framework for modifications to AI/ML based software as a medical device (SaMD) — US Food & Drug Administration, discussion paper, May 2019, https://www.fda.gov/medical-devices/software-medical-device-samd/artificial-intelligence-and-machinelearning-software-medical-device. EU Member State reports include, Joshi, I., Morley, J.,(eds) Artificial intelligence: How to get it right. Putting policy into practice for safe data-driven innovation in health and care, NHSX, 2019, https://www. nhsx.nhs.uk/assets/NHSX_AI_report.pdf; AI sector deal, UK Government, Policy Paper updated May 2019, https:// www.gov.uk/government/publications/artificial-intelligence-sector-deal/ai-sector-deal; Germany: Artificial Intelligence Strategy, German Federal Government, April 2019, https://ec.europa.eu/knowledge4policy/publication/germany-artificialintelligence-strategy_en. Finland’s age of artificial intelligence Turning Finland into a leading country in the application of artificial intelligence. Objective and recommendations for measures”, Finnish Ministry of Economic Affairs and Employment, December 2017, https://julkaisut.valtioneuvosto.fi/bitstream/handle/10024/160391/TEMrap_47_2017_verkkojulkaisu. pdf. And in China, New Generation of Artificial Intelligence Development Plan”, Chinese State Council, Document 2017, no 35, in Foundation for Law and International Affairs, https://flia.org/notice-state-council-issuing-new-generation-artificialintelligence-development-plan/.
[14] “Here’s how Qatar is investing in AI research and advanced technology”, Business Insider, 16 October 2019, https:// www.businessinsider.com/sc/how-qatar-is-investing-in-ai-research-and-advanced-technology-2019-10.
[15] “Israel Prepares to Unleash AI on Health Care”,Wall Street Journal, September 15, 2019.
[16] PitchBook Data, Inc., https://pitchbook.com/research-process.
[17] https://ec.europa.eu/jrc/communities/en/node/1286/document/eu-declaration-cooperation-artificial-intelligence.
[18] Communication from the Commission to the European Parliament, the European Council, the Council, the European Economic and Social Committee and the Committee of the Regions on Artificial intelligence for Europe (COM(2018) 237 final) https://ec.europa.eu/digital-single-market/en/news/communication-artificial-intelligence-europe.
[19] Communication from the Commission to the European Parliament, the European Council, the Council, the European Economic and Social Committee and the Committee of the Regions on a Coordinated plan on artificial intelligence (COM(2018) 795 final) https://ec.europa.eu/digital-single-market/en/news/coordinated-plan-artificial-intelligence.
[20] Draft ethics guidelines for trustworthy AI, High-Level Expert Group on Artificial Intelligence, https://ec.europa.eu/ digital-single-market/en/news/draft-ethics-guidelines-trustworthy-ai. Other core EU publications on AI include: Artificial intelligence: Potential benefits and ethical considerations, European Parliament Legal Affairs briefing, Policy Department C: Citizens’ Rights and Constitutional Affairs, PE 571.380, 2016, http://www.europarl.europa.eu/RegData/etudes/ BRIE/2016/571380/IPOL_BRI(2016)571380_EN.pdf; Artificial Intelligence: A European Perspective, JRC, December 2018, https://publications.jrc.ec.europa.eu/repository/handle/JRC113826; The European AI Landscape, European Commission Workshop Report, April 2018, https://ec.europa.eu/digital-single-market/en/news/european-artificial-intelligencelandscape; Artificial intelligence: Challenges for EU citizens and consumers, European Parliament Committees Briefing Notes, January 2019, http://www.europarl.europa.eu/RegData/etudes/BRIE/2019/631043/IPOL_BRI(2019)631043_ EN.pdf. Communication on building trust in human-centric AI, European Commission, April 2019, https://ec.europa. eu/digital-single-market/en/news/communication-building-trust-human-centric-artificial-intelligence; and Policy and investment recommendations for trustworthy AI, June 2019, https://ec.europa.eu/digital-single-market/en/news/policyand-investment-recommendations-trustworthy-artificial-intelligence.
[21] Communication on enabling the digital transformation of health and care in the Digital Single Market, European Commission, April 25, 2018, https://ec.europa.eu/digital-single-market/en/news/communication-enabling-digitaltransformation-health-and-care-digital-single-market-empowering.
[22] Digital Europe Programme: a proposed €9.2 billion of funding for 2021–2027, European Commission, June 26, 2019, https://ec.europa.eu/digital-single-market/en/news/digital-europe-programme-proposed-eu92-billion-funding-2021-2027; Digital education action plan, European Commission, https://ec.europa.eu/education/education-in-the-eu/digitaleducation-action-plan_en.
[23] “Ping An Good Doctor has become the first online healthcare platform with more than 300 million registered users”, PR newswire, September 2019, https://www.prnewswire.com/news-releases/ping-an-good-doctor-has-become-the-firstonline-healthcare-platform-with-more-than-300-million-registered-users-300923026.html.