Editor in Chief:
Joaquim Cardoso MSc.
Health Transformation News
Data Health Section
October 5, 2021
MIT Technology Review Insights
Produced in partnership with Databricks
2021
Key findings of this research:
- 1.Just 13% of organizations excel at delivering on their data strategy
- 2.Technology-enabled collaboration is creating a working data culture
- 3.ML’s business impact is limited by difficulties managing its end-to-end lifecycle
- 4.Enterprises seek cloud-native platforms that support data management, analytics, and machine learning
- 5.Open standards are the top requirement of future data architecture strategies
The research offers some lessons for CDOs looking to use their assets to nurture a data culture in their business.
- Keep it simple and flexible
- Keep it well-governed
- Explain and evangelize
- Bond with C-suite peers
Infographic
Figure 8: Respondents currently evaluating or implementing a new data platform to enable their enterprise-wide data strategy or solutions to address their current challenges (% of respondents)
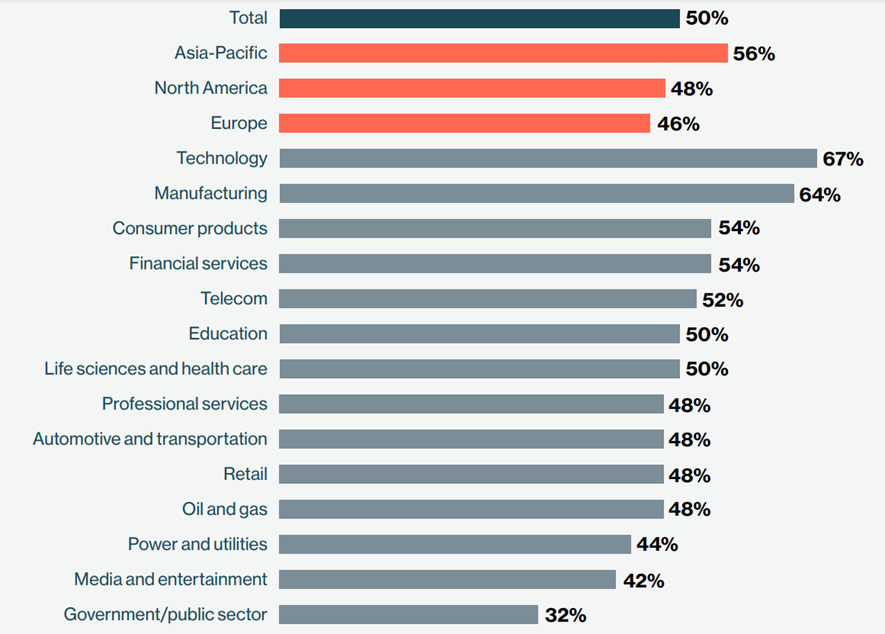
Figure 9: The most critical advantages of respondents’ ideal new architecture over the existing one (top responses; % of respondents)
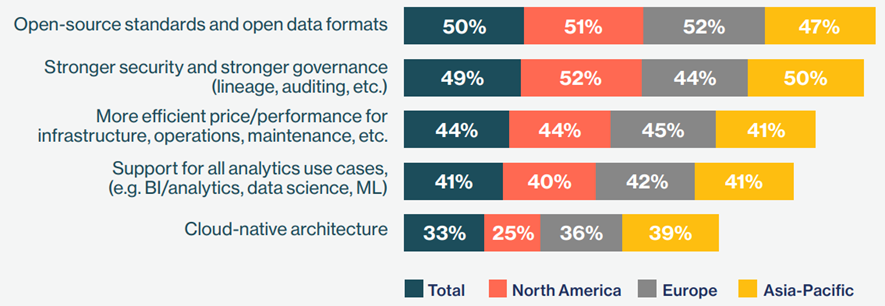
Preface
“Building a high-performance data and AI organization” is an MIT Technology Review Insights report sponsored by Databricks.
- To produce this report, MIT Technology Review Insights conducted a global survey of 351 chief data officers, chief analytics officers, chief information officers, and other senior technology executives.
- The respondents are evenly distributed among North America, Europe, and Asia-Pacific.
- There are 14 sectors represented in the sample and all respondents work in organizations earning $1 billion or more in annual revenue.
The research also included a series of interviews with executives who have responsibility for their organizations’ data management, analytics, and related infrastructure.
Denis McCauley was the author of the report, Francesca Fanshawe was the editor, and Nicola Crepaldi was the producer. The research is editorially independent, and the views expressed are those of MIT Technology Review Insights.
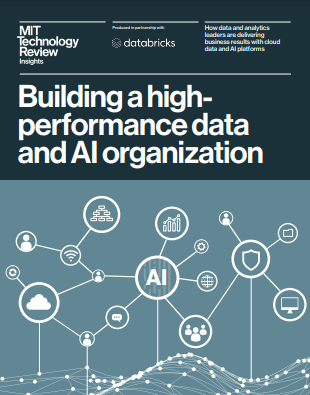
Executive Summary
CxOs and boards recognize that their organization’s ability to generate actionable insights from data, often in real-time, is of the highest strategic importance.
If there were any doubts on this score, consumers’ accelerated flight to digital in this past crisis year have dispelled them.
To help them become data driven, companies are deploying increasingly advanced cloud-based technologies, including analytics tools with machine learning (ML) capabilities.
What these tools deliver, however, will be of limited value without abundant, high-quality, and easily accessible data.
To help them become data driven, companies are deploying increasingly advanced cloud-based technologies, including analytics tools with machine learning (ML) capabilities.
What these tools deliver, however, will be of limited value without abundant, high-quality, and easily accessible data.
In this context, effective data management is one of the foundations of a data-driven organization.
But managing data in an enterprise is highly complex.
- As new data technologies come on stream, the burden of legacy systems and data silos grows, unless they can be integrated or ring-fenced.
- Fragmentation of architecture is a headache for many a chief data officer (CDO), due not just to silos but also to the variety of on-premise and cloud-based tools many organizations use.
- Along with poor data quality, these issues combine to deprive organizations’ data platforms — and the machine learning and analytics models they support — of the speed and scale needed to deliver the desired business results.
In this context, effective data management is one of the foundations of a data-driven organization. But managing data in an enterprise is highly complex.
To understand how data management and the technologies it relies on are evolving amid such challenges, MIT Technology Review Insights surveyed 351 CDOs, chief analytics officers (CAOs; we refer to these and CDOs as “data leaders” at various points in the report) as well as chief information officers (CIOs), chief technology officers (CTOs), and other senior technology leaders.
We also conducted in-depth interviews with several other senior technology leaders.
Following are the key findings of this research:
- 1.Just 13% of organizations excel at delivering on their data strategy
- 2.Technology-enabled collaboration is creating a working data culture.
- 3.ML’s business impact is limited by difficulties managing its end-to-end lifecycle.
- 4.Enterprises seek cloud-native platforms that support data management, analytics, and machine learning.
- 5.Open standards are the top requirement of future data architecture strategies.
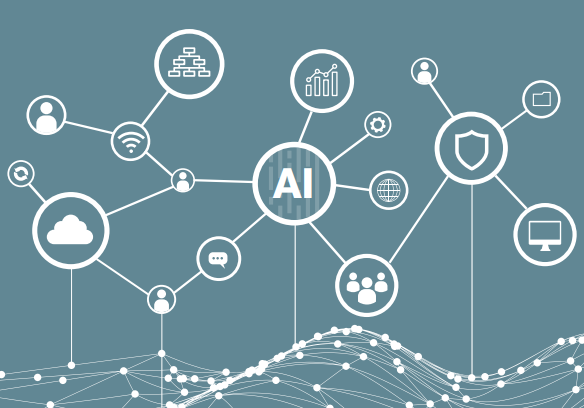
1.Just 13% of organizations excel at delivering on their data strategy.
This select group of “high-achievers” deliver measurable business results across the enterprise.
They are succeeding thanks to their attention to the foundations of sound data management and architecture, which enable them to “democratize” data and derive value from machine learning.
The foundations ensure reduced data duplication, easy access to relevant data, the ability to process large amounts of data at high speeds, and improved data quality.
The high-achievers are also advanced cloud adopters, with 74% running half or more of their data services or infrastructure in a cloud environment.
Organizations’ top data priorities over the next two years fall into three areas, all supported by wider adoption of cloud platforms:
- improving data management,
- enhancing data analytics and ML, and
- expanding the use of all types of enterprise data, including streaming and unstructured data.
2.Technology-enabled collaboration is creating a working data culture.
The CDOs interviewed for the study ascribe great importance to democratizing analytics and ML capabilities.
Pushing these to the edge with advanced data technologies will help end-users to make more informed business decisions — the hallmarks of a strong data culture.
This is only possible with a modern data architecture.
One CDO sums it up by saying that successful data management is achieved when the right users have access to the right data to quickly generate insights that drive business value.
3.ML’s business impact is limited by difficulties managing its end-to-end lifecycle.
Scaling ML use cases is exceedingly complex for many organizations.
The most significant challenge, according to 55% of respondents, is the lack of a central place to store and discover ML models.
That absence, along with error-prone hand-offs between data science and production and a lack of skilled ML resources — both cited by 39% of respondents — suggest severe difficulties in making collaboration between ML, data, and business-user teams a reality.
Scaling ML use cases is exceedingly complex for many organizations. The most significant challenge, according to 55% of respondents, is the lack of a central place to store and discover ML models.
… along with error-prone hand-offs between data science and production and a lack of skilled ML resources … suggest severe difficulties in making collaboration between ML, data, and business-user teams a reality.
4.Enterprises seek cloud-native platforms that support data management, analytics, and machine learning.
Organizations’ top data priorities over the next two years fall into three areas, all supported by wider adoption of cloud platforms:
- improving data management,
- enhancing data analytics and ML, and
- expanding the use of all types of enterprise data, including streaming and unstructured data.
For “low-achievers” — organizations having difficulty delivering on data strategy — improving data management overshadows all other priorities, cited by 59% of this group.
Most high-achievers, by contrast (53%), are focused on advancing their ML use cases.
For “low-achievers” — organizations having difficulty delivering on data strategy — improving data management overshadows all other priorities, cited by 59% of this group.
5.Open standards are the top requirement of future data architecture strategies.
If respondents could build a new data architecture for their business, the most critical advantage over the existing architecture would be a greater embrace of open-source standards and open data formats.
Data leaders now realize the value of open-source standards to accelerate innovation and enable choice in leveraging best-of-breed third-party tools.
Stronger security and governance, not surprisingly, are also near the top of respondents’ list of requirements.
If respondents could build a new data architecture for their business, the most critical advantage over the existing architecture would be a greater embrace of open-source standards and open data formats.
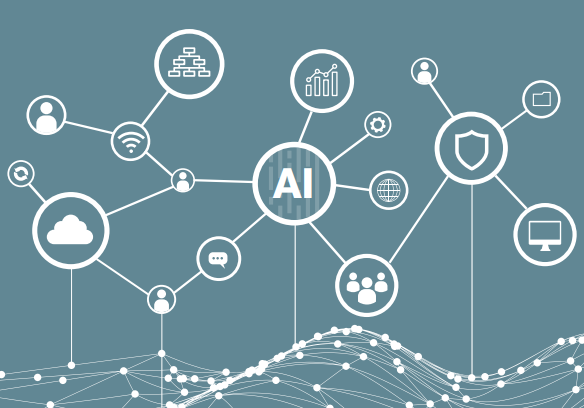
Contents (of the full version)
01 Executive summary
02 Growth and complexity
03 Aligning and delivering on strategy
04 Scaling analytics and machine learning
05 Visions of the future
06 Conclusion
Chapters 2 to 4
See the original publication (this is an excerpt version)
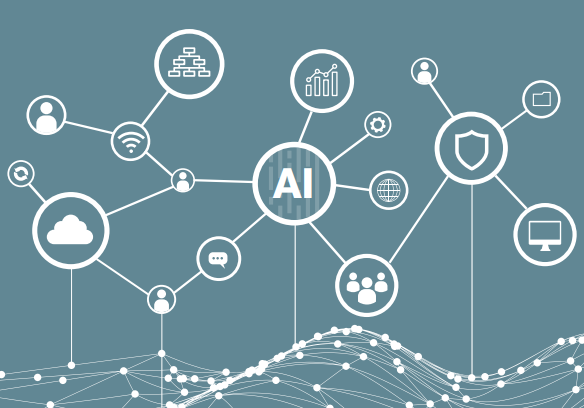
5.Visions of the future
Half of the surveyed executives (and around two-thirds of those in technology and manufacturing firms) say they are currently evaluating or implementing a new data platform to address their current data challenges.
Another 9% would do so but face blockers in changing their architecture.
Here again, high- and low-achievers diverge markedly.
- Most high-achievers (56%) are satisfied with their current architecture, although nearly one-third of these are, nevertheless, researching new platforms or evaluating vendors.
- Just 24% of low-achievers, by contrast, are happy with the existing architecture, and 59% are actively seeking to change it.
Technology firms and manufacturers are far more likely than those in other sectors to be on the path of upgrading their platform.
Half of the surveyed executives (and around two-thirds of those in technology and manufacturing firms) say they are currently evaluating or implementing a new data platform to address their current data challenges.
A CDO wish-list for a new architecture
What if data and technology leaders could build a new data architecture for their business? We asked them what its key advantages would be over their existing one.
- At the top of their wish-list, cited by 50% (and by 60% or higher among technology, utilities, and government respondents), are open-source standards and open-data formats.
What if data and technology leaders could build a new data architecture for their business? … open-source standards and open-data formats [are on the top of the list, due to several advantages] …
This is no surprise to Andy McQuarrie of Hivery:
- “Open standards are what allows you to move through the maturity curve quite easily. You can consume services with a managed service in the short term.
- When your business matures and you find you need to add a component, with open source you’ve got that option without it affecting the entire architecture.
- You don’t need to be changing technologies the way that you might have had to in the past.”
Next on the wish-list are not new capabilities, but rather strengthened ones in areas that technology leaders never stop seeking improvement in:
- stronger security, stronger governance, and
- better price/performance for infrastructure, operations, maintenance, and other architecture elements.
- The respondents are also insistent that any new architecture supports all analytics use cases, whether based on ML, data science, or business intelligence.
Open-source standards and open-data formats are top of the wish-list for technology leaders, followed by stronger security and governance, and better price/performance for architecture elements.
Figure 8: Respondents currently evaluating or implementing a new data platform to enable their enterprise-wide data strategy or solutions to address their current challenges (% of respondents)
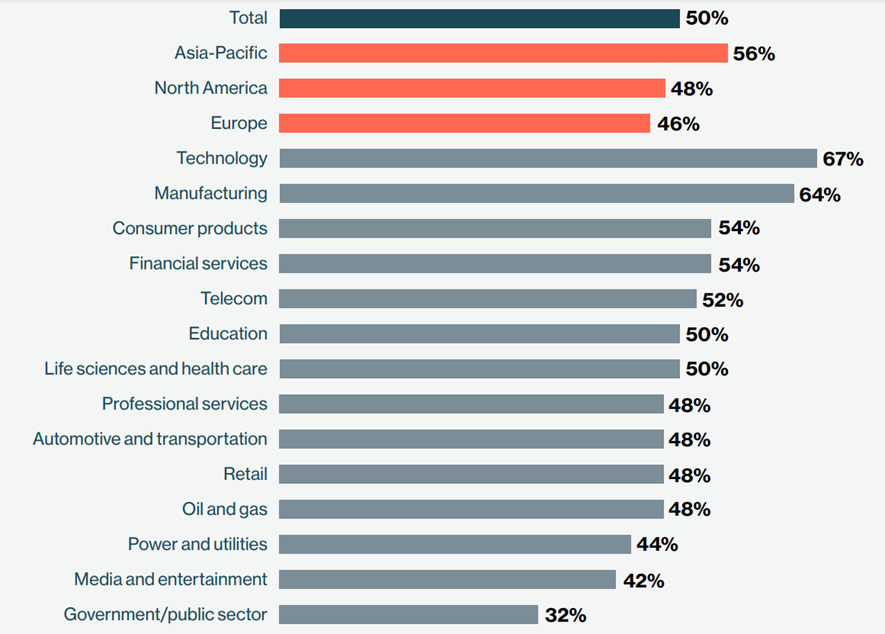
MIT Technology Review Insights survey, 2021
Figure 9: The most critical advantages of respondents’ ideal new architecture over the existing one (top responses; % of respondents)
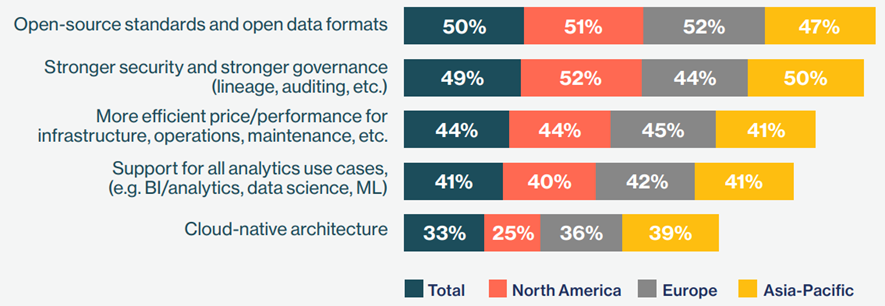
MIT Technology Review Insights survey, 2021
“Native integration with customer systems is something we are really focused on. How can we make it easier for our customers to transfer their data to us?”
– Andy McQuarrie, Chief Technology Officer, Hivery
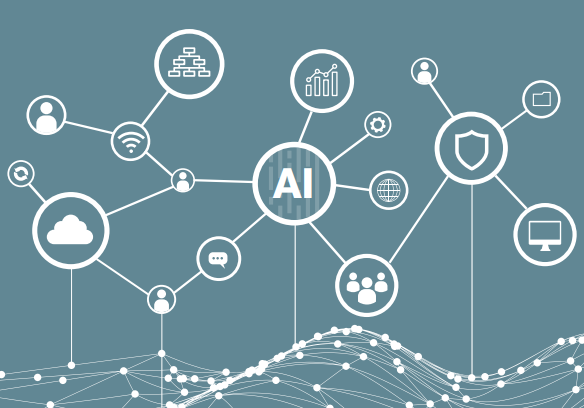
The CDOs we interviewed have additional hopes and plans as their data management and infrastructure evolve.
Michel Lutz, group chief data officer at energy major Total, aims to expand ML-based automation of its metadata management.
This has already been done for geoscience data, but he plans to extend it to all the group’s data domains in the next year.
The potential of data mesh architecture is also on Lutz’s radar. “It’s certainly a next step for our data architecture because it allows a higher organizational scalability and enables more domain specialization,” he says.
L’Oréal’s Naveen Jayaraman similarly sees the advantages of domain-oriented data products for enterprises like his that look to distribute data management functions widely.
Architectural approaches using data mesh have potential for his purposes, Jayaraman says, provided that some elements, such as polyglot storage, can be centralized.
Mainak Mazumdar of Nielsen looks forward to implementing “AI for AI” — essentially the automated selection of AI models.
“We hope to build in another layer of intelligence that will make the best decisions about models to use.”
On a more distant horizon, Ashwin Sinha thinks about the possibilities of “agile and real-time architecture”, one that is agile enough to adapt to future innovation in data engineering.
It would be a way, he says, of future-proofing a recently designed architecture, at least for the medium term.
“We’d like to be able to adopt an architectural approach flexible enough to ensure it won’t become outdated in two or three years.”
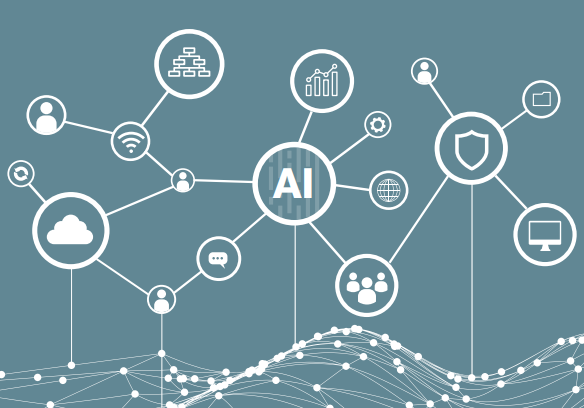
6.Conclusion
A common thread in our research, and particularly our discussions with CDOs, has been recognition of a direct line running from data infrastructure through data science to the attitudes towards data held by employees up and down the organization, and tying it all back to business impact — i.e. the data culture.
The research offers some lessons for CDOs looking to use their assets to nurture a data culture in their business.
- Keep it simple and flexible
- Keep it well-governed
- Explain and evangelize
- Bond with C-suite peers
- Keep it simple and flexible. Many CDOs want to simplify overly complex architectures, but it is just as important to ensure that interfaces for analytics end-users are easy to use and, to the extent possible, fun. The architecture must also remain flexible enough to ensure future business needs are met without requiring large migrations or redesigns to add new technologies. As more users are able to pull and play with constantly refreshed data using their preferred tools, and derive insights from it on-demand, engagement will grow.
- Keep it well-governed. Nothing will mar employees’ experience of analytics more than the recurring discovery of errors in the data. CDOs do not need to be reminded of the importance of sound data governance, but not all may realize how far the implications of governance failures can reach in the organization. Building and maintaining a single source of truth matters to everyone.
- Explain and evangelize. Some CDOs task parts of their teams to educate business units and other staff about data science and train them in the use of analytics and other tools. Many also find that advertising widely the positive outcomes achieved, and value gained, from the use of data and analytics can expand their employees’ desire to use them. Advertising data accuracy achieved through strong governance will also help build trust.
- Bond with C-suite peers. The virtue of deepening ties with fellow C-levels may seem self-evident to CDOs and CAOs, but any disconnects on data management with CIOs and CTOs could, if not addressed, become sources of mistrust. The data strategy needs to factor in the existing technology infrastructure and ensure it is always focused on delivering the corporate priorities.
About the authors:
Denis McCauley was the author of the report,
Francesca Fanshawe was the editor, and
Nicola Crepaldi was the producer.
Acknowledgements:
We would like to thank the following individuals for providing their time and insights:
Patrick Baginski, Senior Director Data Science, McDonald’s (United States)
Bob Darin, Chief Data Officer, CVS Health, and Chief Analytics Officer, CVS Pharmacy (United States)
Naveen Jayaraman, Vice President — Data, CRM & Analytics, L’Oréal (United States)
Michel Lutz, Group Chief Data Officer, Total (France)
Mainak Mazumdar, Chief Data and Research Officer, Nielsen (United States)
Andy McQuarrie, Chief Technology Officer, Hivery (Australia)
Sol Rashidi, Chief Analytics Officer, The Estée Lauder Companies (United States)
Ashwin Sinha, Chief Data and Analytics Officer, Macquarie Bank (Australia)
Don Vu, Chief Data Officer, Northwestern Mutual (United States)
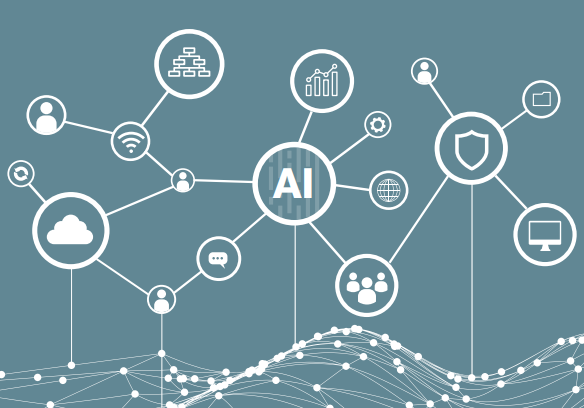