MIT Sloan Management Review
Thomas H. Davenport and Randy Bean
May 05, 2021
This is a republication of the article above on the second part of this post, preceded by an Executive Summary, by the Editor of the Health Revolution — Institute.
Executive Summary
by Joaquim Cardoso MSc.
Health Revolution
Institute for Continuous Improvement to achieve “Health for All”
May 24, 2022
What is the problem?
- One of the great challenges we have seen businesses face in recent years is how they approach data and analytics (and now artificial intelligence) when their industries are undergoing major transformation.
- It’s hard enough to create a data-driven culture, compete on analytics, develop data-driven products and services, and so forth under normal business conditions
Which industry is one example of Data Transformation and Industry Transformation?
- “Making progress” is what executives told us at Parexel International, one of the world’s leading clinical research organizations, or CROs.
What are the changes that the Clinical Research Industry is going through?
The industry changes that Parexel and its competitors (in the Clinical Research Industry) are experiencing are dizzying.
- New imperatives to process real-world evidence.
- Expectations for longer-term data collection.
- Opportunities to do testing over a larger geographic area.
- Potential around AI-driven simulations for clinical trials.
- Challenges from doing trials directly in individuals’ homes.
- Prospects for making patient recommendations based on genetics.
How has Parexel approached the challenge?
- Hoiseth heads a data science group that she characterizes as “small but mighty” and growing rapidly.
- She and Pretorius are discussing where the data science capability should be placed organizationally.
- They are using data, analytics, and AI not only to keep up with industry changes but to get ahead of and shape the transformation of biopharmaceutical and clinical research for the benefit of patients around the world.
They are using data, analytics, and AI not only to keep up with industry changes but to get ahead of and shape the transformation of biopharmaceutical and clinical research for the benefit of patients around the world.
Structure of the publication
- Introduction
- A Time of Dramatic Industry Change
- The Data and AI Response to Change
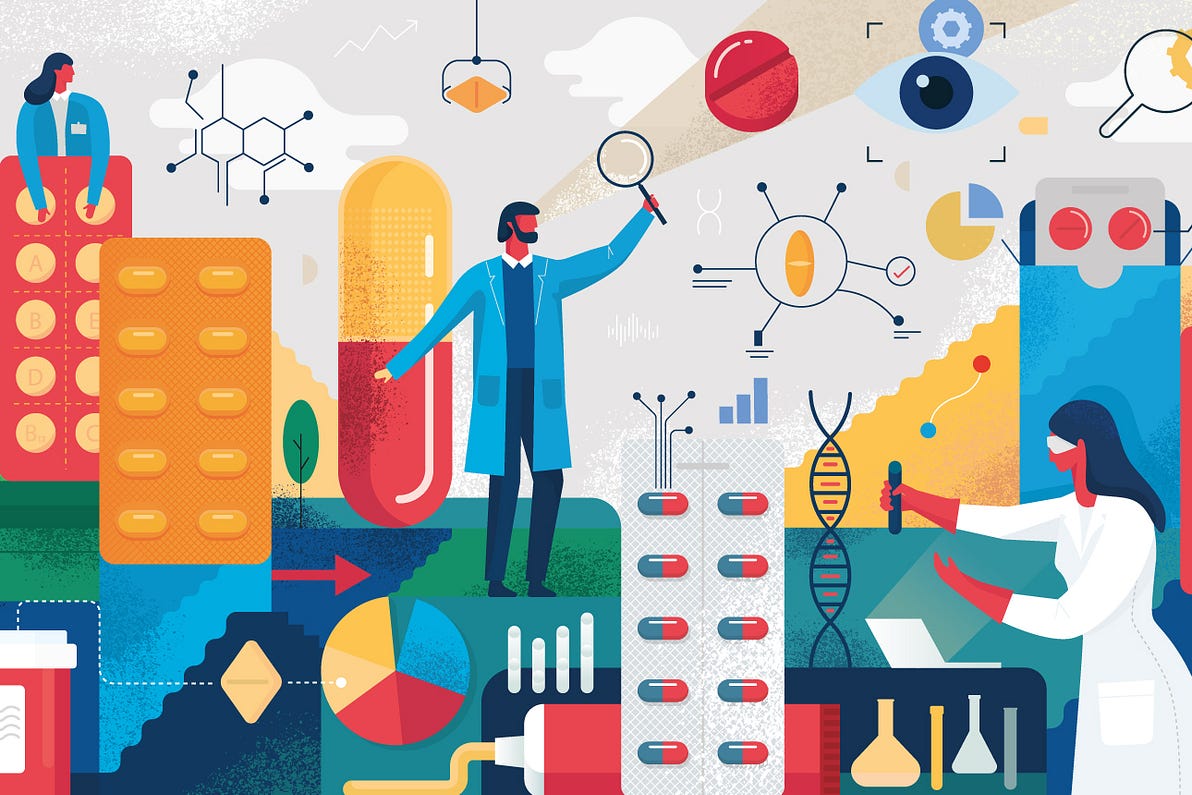
Embracing AI When Your Industry Is in Flux
MIT Sloan Management Review
Thomas H. Davenport and Randy Bean
May 05, 2021
One of the great challenges we have seen businesses face in recent years is how they approach data and analytics (and now artificial intelligence) when their industries are undergoing major transformation.
It’s hard enough to create a data-driven culture, compete on analytics, develop data-driven products and services, and so forth under normal business conditions, as we noted in our March column about the newest NewVantage Partners survey on big data and AI.
But doing it while your business and industry are transforming — the old line of changing out a jet engine while the plane is flying through turbulence at 35,000 feet — is really tough.
It’s so difficult, in fact, that we always have our doubts when executives claim to have done it successfully.
We are much more trusting when we’re told that the organization is simply making progress toward the goal.
“Making progress” is what executives told us at Parexel International, one of the world’s leading clinical research organizations, or CROs.
Dr. Sy Pretorius, the company’s president, clinical development and chief medical officer, shared that Parexel is attempting to take an aggressive approach to artificial intelligence, but he also added, “I’m not sure if we have enough going on with AI.”
That honesty made what he told us about adopting AI while dealing with enormous industry transitions even more convincing.
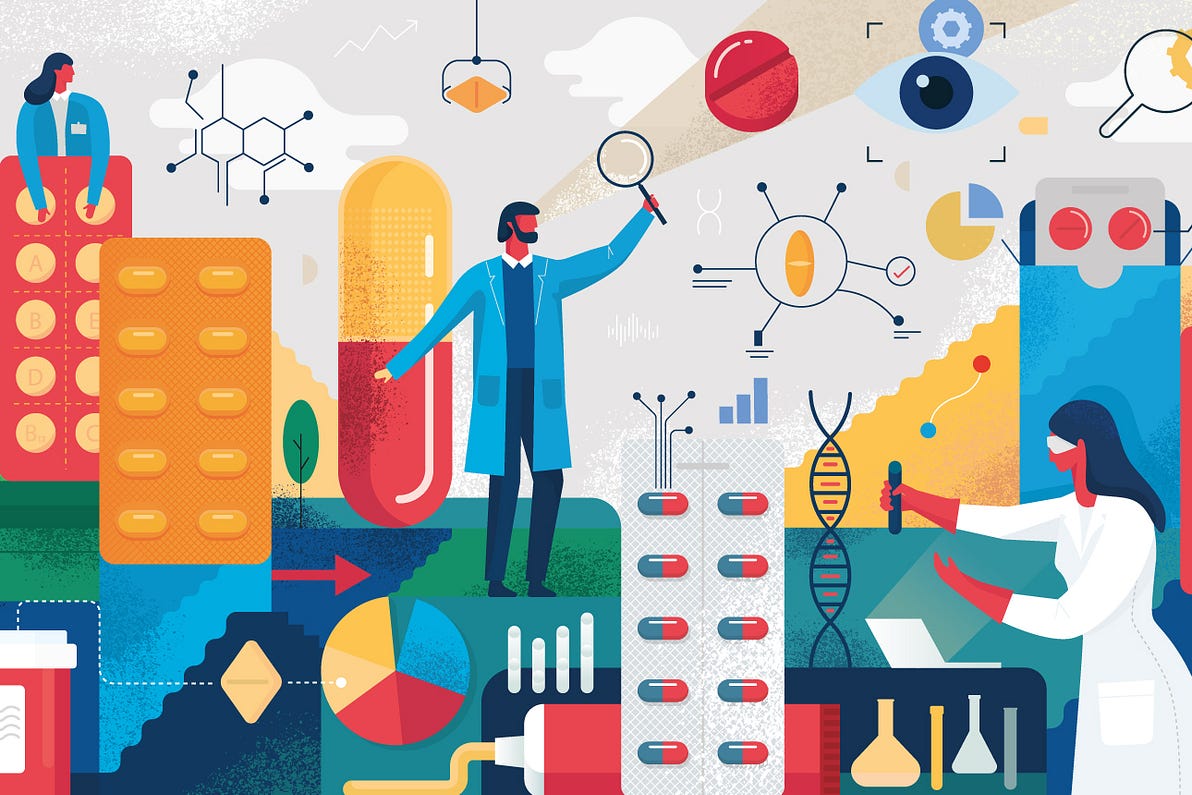
A Time of Dramatic Industry Change
The industry changes that Parexel and its competitors are experiencing are dizzying.
CROs have historically focused on conducting clinical trials for pharmaceutical companies, but an array of new strategies and business models are moving the industry in a more data-driven direction. Let us count (some of) the ways:
- New imperatives to process real-world evidence.
- Expectations for longer-term data collection.
- Opportunities to do testing over a larger geographic area.
- Potential around AI-driven simulations for clinical trials.
- Challenges from doing trials directly in individuals’ homes.
- Prospects for making patient recommendations based on genetics.
1.New imperatives to process real-world evidence.
Clinical trials used to all be conducted in the same way, using the classic randomized, double-blind, placebo-controlled “gold standard” methodology. But pharma firms and CROs are increasingly using new approaches to trials in which everyone gets the experimental treatment, and those patients are compared to a synthetic arm of patients with the disease who are receiving the standard of care for it. This means that the conductors of the trial need to obtain and analyze real-world evidence from sources like electronic medical records to analyze how the synthetic arm patients are doing. As Pretorius noted, “Once health care data can be used directly to build the clinical data set for safety and efficacy analysis, fewer patients will need to be enrolled and randomized, reducing the total duration of the study and the logistical burden on patients.” But the analytical and data management burden on the CRO is increased.
2.Expectations for longer-term data collection.
CROs have traditionally focused on the safety and efficacy of drugs and devices during the clinical trial period. But now their pharmacovigilance often extends indefinitely into the period after the treatments are introduced to the market. This post-market surveillance became well known during the 2004 Vioxx controversy, in which a drug on the market for five years was found to cause serious heart problems. Parexel and other CROs can perform pharmacovigilance services for an extended period, which means monitoring signals of adverse events from a variety of sources.
3.Opportunities to do testing over a larger geographic area.
AI is clearly needed to optimize the selection of sites and countries for clinical trials, which are becoming increasingly global and complex and, as a result, more expensive. There is too much information for any human to keep in mind during trial design. AI is clearly needed to optimize the selection of sites and countries for trials.
4.Potential around AI-driven simulations for clinical trials.
Given the expense and danger of animal and human clinical trials, Parexel and other companies are exploring the use of in silico trials, in which the potential impact of a drug on the body or an organ is simulated with data rather than actually administered to a living being.
5.Challenges from doing trials directly in individuals’ homes.
During the COVID-19 pandemic, the traditional practice of overseeing clinical trials at physical sites has not been feasible. Parexel and others in the industry have pivoted and embraced the opportunity to make trials more patient focused, adopting decentralized approaches in which drugs or devices are delivered to and administered at patients’ homes. Trial procedures are monitored via telemedicine or other digital means. This, of course, generates new types of data: Information that was once collected during checkups from clinicians is increasingly delivered through wearable and home monitoring devices. These voluminous streams of data need to be stored, integrated, and analyzed to ensure patient compliance and engagement with the trial.
6.Prospects for making patient recommendations based on genetics.
The precision medicine movement, in which treatments for diseases are recommended (or not) on the basis of genetic biomarkers, is making slow but steady progress. Parexel is focused on being able to use analytics and AI to know the attributes that might make a particular patient a responder or nonresponder to a drug.
If all of these industrywide changes weren’t enough, in January 2021, Parexel completed the separation of its Parexel Informatics and Medical Imaging business (now known as Calyx) to streamline its business strategy and customer relationships.
Parexel continues to work with Calyx on clinical development offerings.
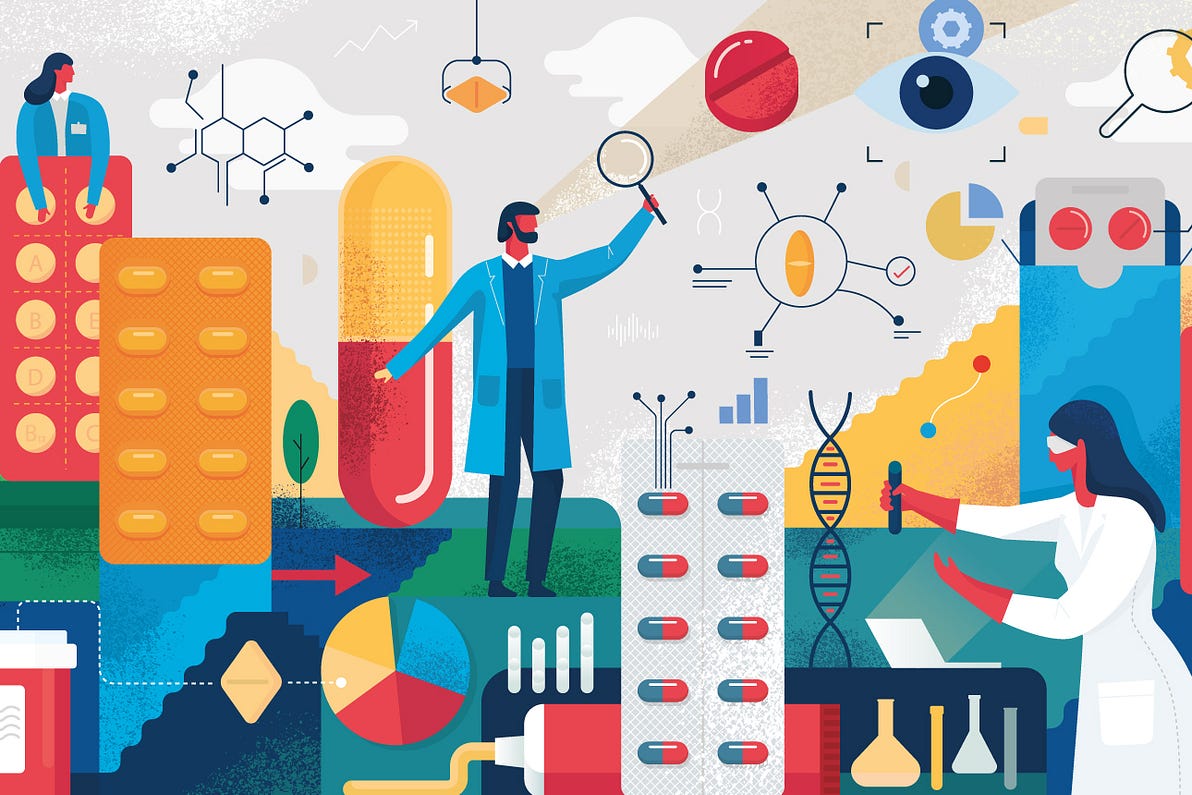
The Data and AI Response to Change
The job of creating the data, analytics, and AI capabilities to deal effectively with these wide-ranging changes belongs to Michelle Hoiseth, Parexel’s senior vice president and chief data officer.
A 23-year veteran of Parexel, Hoiseth was a clinical researcher before moving into technology and data assignments.
Like Pretorius, she doesn’t claim that the company has achieved mastery over all data and AI domains.
But she can certainly describe a good bit of progress. Hoiseth outlined four major areas of AI activity at Parexel.
- The first category of AI work is the simplest and most straightforward — Automation.
- The second category is much more difficult: It involves AI for the detection of risk signals in patient data.
- The third category of AI systems involves the analysis of patient data, particularly for post-market surveillance.
- The fourth area of AI work is in predicting and monitoring patient outcomes.
The first category of AI work is the simplest and most straightforward — Automation.
Automation — robotic process and more intelligent automation — is being used to reduce the manual labor burden in administrative processes.
The second category is much more difficult: It involves AI for the detection of risk signals in patient data.
The data may be in current or old clinical-trial reports, which may involve a need for AI-based data extraction — or it may be in wearable or medical device data streams, electronic medical records, clinical notes, or even social media content.
If AI systems could effectively monitor risk signals to search for fraud and compliance across these data types, for example, it would take a big load off the clinical research associates who generally perform that task, and perhaps provide earlier and stronger signals as well.
The third category of AI systems involves the analysis of patient data, particularly for post-market surveillance.
Parexel now monitors adverse events from clinician reports, but direct monitoring of patients when multiple drugs are co-prescribed and used on different types of patients would yield better pharmacovigilance.
The fourth area of AI work is in predicting and monitoring patient outcomes.
The goal is to understand which patients respond to drugs as predicted, which ones don’t, and what attributes might make them a responder or nonresponder.
Hoiseth noted that this category of AI is of keen interest throughout the industry, and it extends beyond drugs and devices to other medical interventions, such as surgery.
“It’s clear,” she said, “that life science and health care providers will converge on value-based outcomes, which will be a big change for them.
We are partnering with both groups to make progress in this complex domain of precision medicine.”
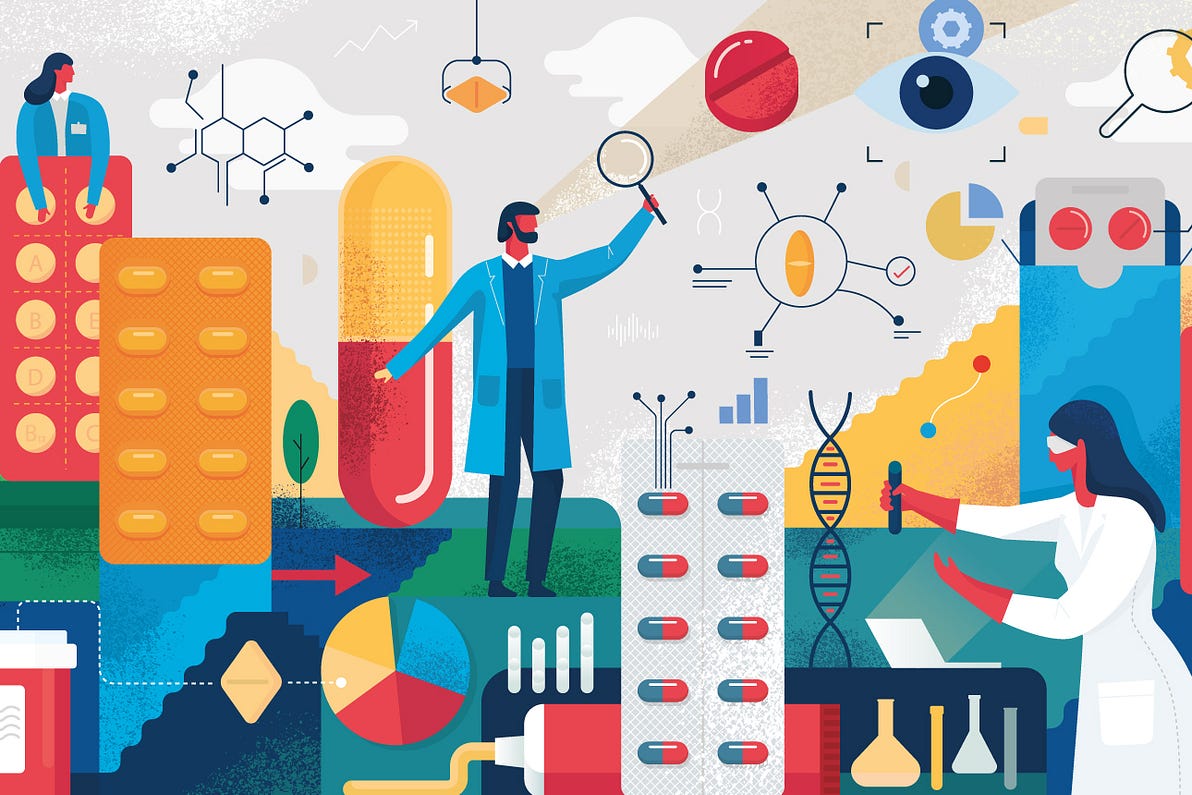
Hoiseth heads a data science group that she characterizes as “small but mighty” and growing rapidly.
She and Pretorius are discussing where the data science capability should be placed organizationally.
Parexel International has been in business for more than 35 years, and Pretorius and Hoiseth both said that they’ve never seen so much change in the industry.
They are using data, analytics, and AI not only to keep up with industry changes but to get ahead of and shape the transformation of biopharmaceutical and clinical research for the benefit of patients around the world.
They are using data, analytics, and AI not only to keep up with industry changes but to get ahead of and shape the transformation of biopharmaceutical and clinical research for the benefit of patients around the world.
About the Authors
Thomas H. Davenport (@tdav) is the President’s Distinguished Professor of Information Technology and Management at Babson College, a visiting professor at Oxford’s Saïd Business School, and a fellow of the MIT Initiative on the Digital Economy.
Randy Bean (@randybeannvp) is an industry thought leader, author, and CEO of NewVantage Partners, a strategic advisory and management consulting firm that he founded in 2001. He is the author of the forthcoming book Fail Fast, Learn Faster: Lessons in Data-Driven Leadership in an Age of Disruption, Big Data, and AI, which will be published by Wiley in August 2021.
Dr. Sy Pretorius, the company’s president, clinical development and chief medical officer,