A study from the Smidt Heart Institute and Cedars-Sinai’s Division of Artificial Intelligence in Medicine shows that AI was more accurate in diagnosing cardiac function than sonographers.
Cedars Sinai
August 28, 2022
Illustration by Getty (Healthcare And AI Concept. Black physician holding virtual heart in open palms with connected poly geometric dots)
Site editor:
Joaquim Cardoso MSc.
Health Transformation .Foundation
Heart Management Institute
August 29, 2022
Cedars-Sinai and Smidt Heart Institute Study, Presented at European Society of Cardiology Congress 2022, Shows Artificial Intelligence Can Better Assess and Diagnose Cardiac Function
In a first-of-its-kind randomized clinical trial led by researchers at the Smidt Heart Institute and the Division of Artificial Intelligence in Medicine at Cedars-Sinai, artificial intelligence (AI) proved more successful in assessing and diagnosing cardiac function when compared to echocardiogram assessments made by sonographers.
The results, announced today during a late-breaking presentation at the European Society of Cardiology Congress 2022, have immediate translational implications for patients undergoing cardiac function imaging and broader implications for the field of cardiac imaging.
“This is the first randomized clinical trial of artificial intelligence in cardiology,” said David Ouyang, MD, a cardiologist in the Department of Cardiology in the Smidt Heart Institute and principal investigator of the study, known as EchoNet-RCT.
“With this new knowledge, we hope to improve the quality and effectiveness of echocardiogram imaging for patients everywhere.”
Previously, researchers at the Smidt Heart Institute and Stanford University developed one of the first artificial intelligence technologies to assess cardiac function, …
… specifically, left ventricular ejection fraction — the key heart measurement used in diagnosing cardiac function.
Their research was published in the prestigious journal Nature.
Building on this past research, the most recent study assessed the impact of artificial intelligence in clinical deployment as part of a prospective, blinded and randomized controlled clinical trial.
“There has been much excitement about the use of AI in medicine, but the technologies are rarely assessed in prospective clinical trials,” said Ouyang.
“This trial was powered to show non-inferiority of the AI compared to sonographer tracings, and so we were pleasantly surprised when the results actually showed superiority for AI with respect to the pre-specified outcomes.”
In the study, Cedars-Sinai cardiologists evaluated 3,495 transthoracic echocardiogram studies, comparing initial assessment by artificial intelligence or by a sonographer — also known as an ultrasound technician.
One of the major findings was that cardiologists more frequently agreed with the AI initial assessment, such that they corrected only 16.8% of the initial assessments made by AI and simultaneously corrected 27.2% of the initial assessments made by the sonographers.
This difference demonstrated not only non-inferiority but actually superiority of AI.
One of the major findings was that cardiologists more frequently agreed with the AI initial assessment, such that they corrected only 16.8% of the initial assessments made by AI and simultaneously corrected 27.2% of the initial assessments made by the sonographers.
The research team also discovered that cardiologists were unable to distinguish between initial assessments made by the AI and sonographers.
“We asked our cardiologist over-readers to guess if they thought the tracing they had just reviewed was performed by AI or by a sonographer, and it turns out that they couldn’t tell the difference,” said Ouyang.
“This speaks to the strong performance of the AI algorithm as well as the seamless integration into clinical software. We believe these are all good signs for future trial research in the field.”
“This speaks to the strong performance of the AI algorithm as well as the seamless integration into clinical software. We believe these are all good signs for future trial research in the field.”
Additionally, the Smidt Heart Institute research team learned that this type of clinical trial can be seamlessly integrated into the standard clinical workflow.
Additionally, the Smidt Heart Institute research team learned that this type of clinical trial can be seamlessly integrated into the standard clinical workflow.
“We are excited by the implications of this clinical trial and what it means for the future of artificial intelligence and cardiology,” said Susan Cheng, MD, MPH, director of the Institute for Research on Healthy Aging in the Department of Cardiology at the Smidt Heart Institute and EchoNet-RCT investigator.
“When developed in the right way, artificial intelligence offers the opportunity to improve the quality of echocardiogram readings as well as increase efficiencies in the time and effort spent by busy cardiologists and sonographers alike.”
As a next step, Ouyang says the Smidt Heart Institute team plans to evaluate how AI analysis applied to Cedars-Sinai echocardiogram imaging procedures may improve clinical outcomes.
As a next step, Ouyang says the Smidt Heart Institute team plans to evaluate how AI analysis applied to Cedars-Sinai echocardiogram imaging procedures may improve clinical outcomes.
Originally published at https://www.cedars-sinai.org.
Names mentioned:
David Ouyang, MD, a cardiologist in the Department of Cardiology in the Smidt Heart Institute and principal investigator of the study
Susan Cheng, MD, MPH, director of the Institute for Research on Healthy Aging in the Department of Cardiology at the Smidt Heart Institute and EchoNet-RCT investigator.
ORIGINAL PUBLICATION
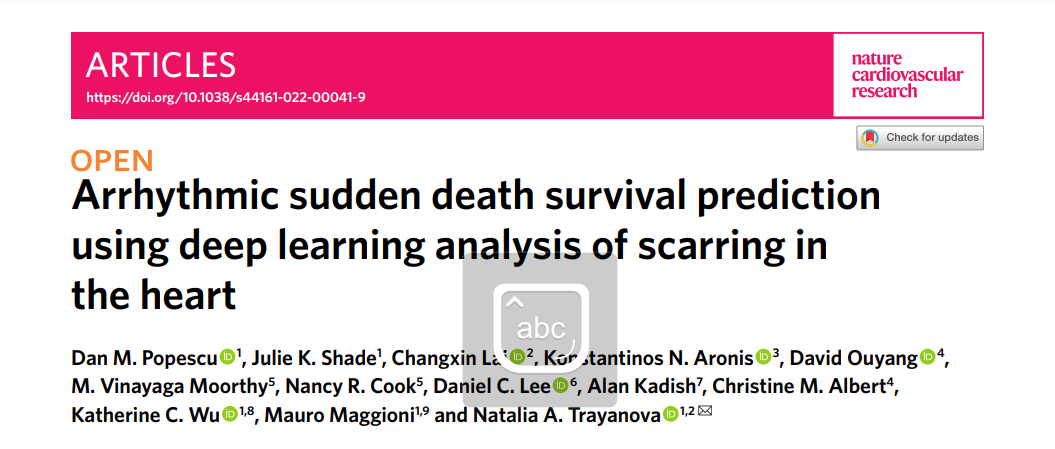
Arrhythmic sudden death survival prediction using deep learning analysis of scarring in the heart
Nature Cardiovascular Research
Dan M. Popescu, Julie K. Shade, Changxin Lai, Konstantinos N. Aronis, David Ouyang, M. Vinayaga Moorthy, Nancy R. Cook, Daniel C. Lee, Alan Kadish, Christine M. Albert, Katherine C. Wu, Mauro Maggioni & Natalia A. Trayanova
Abstract
- Sudden cardiac death from arrhythmia is a major cause of mortality worldwide.
- In this study, we developed a novel deep learning (DL) approach that blends neural networks and survival analysis to predict patient-specific survival curves from contrast-enhanced cardiac magnetic resonance images and clinical covariates for patients with ischemic heart disease.
- The DL-predicted survival curves offer accurate predictions at times up to 10 years and allow for estimation of uncertainty in predictions.
- The performance of this learning architecture was evaluated on multi-center internal validation data and tested on an independent test set, achieving concordance indexes of 0.83 and 0.74 and 10-year integrated Brier scores of 0.12 and 0.14.
- We demonstrate that our DL approach, with only raw cardiac images as input, outperforms standard survival models constructed using clinical covariates.
- This technology has the potential to transform clinical decision-making by offering accurate and generalizable predictions of patient-specific survival probabilities of arrhythmic death over time.
Originally published at https://www.nature.com