health transformation institute (HTI)
the most comprehensive knowledge portal, for health transformation
Joaquim Cardoso MSc*
Founder, Chief Researcher and Editor
November 28, 2022
MSc* from London Business School — MIT Sloan Masters Program
Key messages:
- Tumor cells, such as the ones illustrated here, can present immune-stimulating proteins called antigens on their surface.
- CCR and McGill University researchers developed a machine learning program to predict how these antigens might impact immune cells.
- Their tool could aid in the development of better cancer immunotherapies.
ORIGINAL PUBLICATION
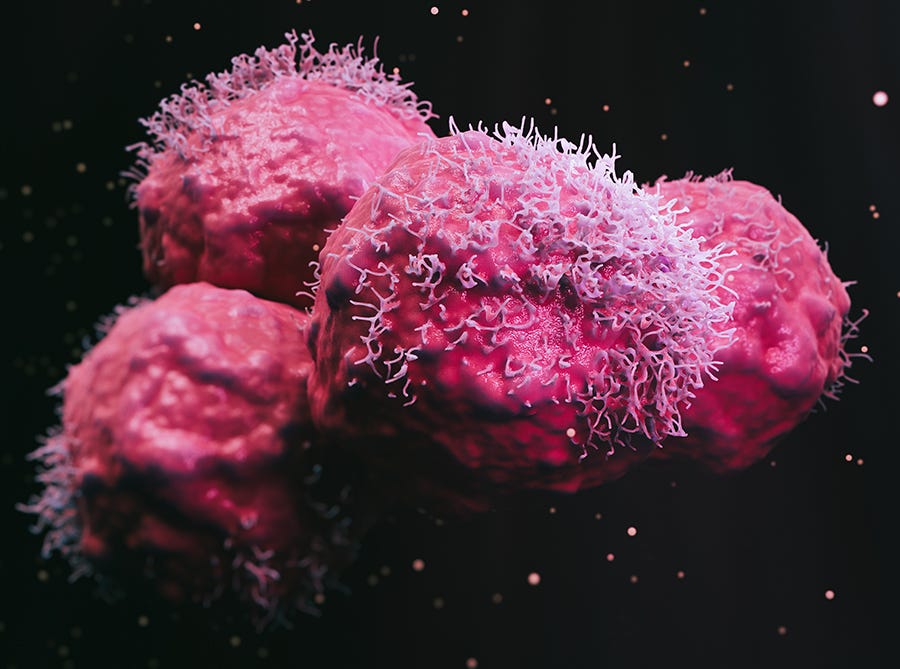
Machine learning reveals rules of T cell activation
National Cancer Institute
Fri, 05/20/2022
To understand complex communications between tumors and immune cells, some cancer researchers are turning to machine learning, a form of artificial intelligence that can learn from and find patterns in data without being explicitly programmed.
A new model developed by CCR and McGill University scientists uses machine learning to predict how tumor antigens — immune-stimulating molecules on the surface of tumor cells — impact the behavior of T cells.
The model is based on extensive experimental data tracking signals that T cells generate after they encounter particular antigens.
Those signals ultimately determine how the immune cells respond to a potential threat.
A new model developed by CCR and McGill University scientists uses machine learning to predict how tumor antigens — immune-stimulating molecules on the surface of tumor cells — impact the behavior of T cells.
The model, reported May 20, 2022, in Science, was developed by the group of Grégoire Altan-Bonnet, Ph.D., Senior Investigator in the Laboratory of Integrative Cancer Immunology, in collaboration with Paul François’ group at McGill University in Montréal.
It has helped clarify the factors that shape T cell responses to tumors, which Altan-Bonnet says could accelerate the development of cancer immunotherapies.
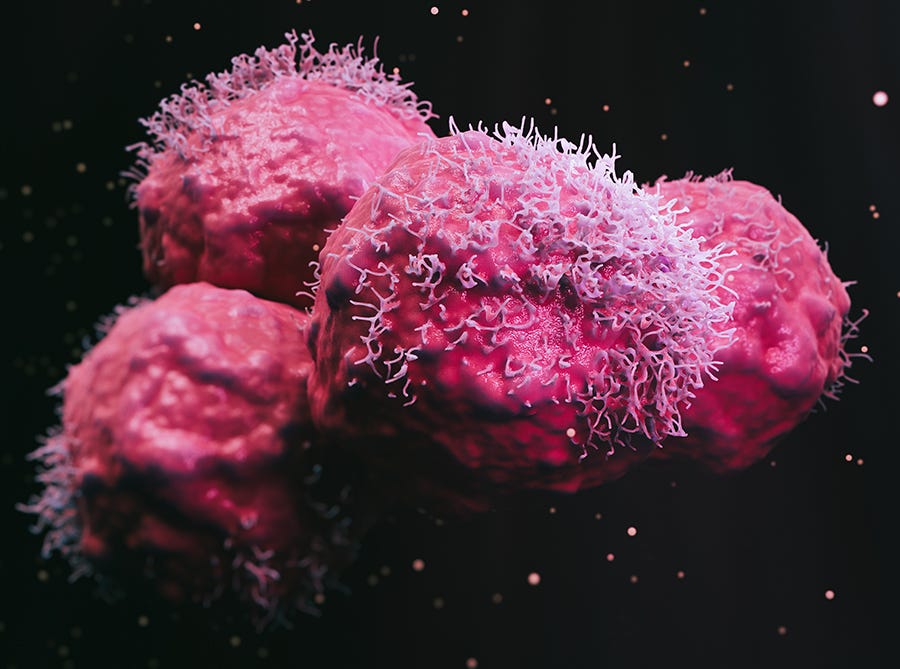
Cancer immunotherapies can help patients’ T cells find and destroy cancer cells, but even with these treatments, some patients’ immune systems fail to mount an effective anticancer response.
Researchers have struggled to develop immunotherapies that work for a majority of patients, partially due to the complexity of the signaling triggered when a T cell encounters a cancer cell, Altan-Bonnet says.
Dozens of signaling molecules communicate via intersecting pathways to shape a T cell’s response to an antigen.
That signaling not only determines whether a T cell attacks a tumor but also how it alerts other immune cells to the threat and how quickly it divides in order to amplify the body’s defenses.
“T cells do many, many different things when they get activated. And we don’t know how to tackle this complexity to predict what really matters in terms of cancer immunotherapy,” says Altan-Bonnet.
“We know a lot about the way these T cells are wired, but we can’t take a T cell and predict if it’s going to be a good T cell that can specifically attack a particular tumor, or if it will confuse tumors with healthy tissues.”
Since no single signal decides the outcome of a battle between a T cell and a tumor, Altan-Bonnet & François’ teams knew that they needed a comprehensive view of the signaling launched by different antigens to make better predictions.
Graduate student Sooraj Achar, an NIH/Oxford fellow from the Altan-Bonnet laboratory, developed an automated system to monitor immune signals and amassed an enormous amount of data detailing how T cells from mice responded to an array of antigens.
Then, graduate students François Bourassa and Thomas Rademaker from François’ group used machine learning to develop a model that processed all the complex patterns of the immune signals.
The model they built accurately predicts the behavior of T cells in both mice and humans, as well as the kinds of engineered T cells that are used for a form of immunotherapy known as CAR T-cell immunotherapy.
The model they built accurately predicts the behavior of T cells in both mice and humans, as well as the kinds of engineered T cells that are used for a form of immunotherapy known as CAR T-cell immunotherapy.
Altan-Bonnet notes that in addition to predicting T cell behavior, the model helps explain how T cells make the decisions that shape that behavior.
“In a nutshell, we’ve learned the rules of the way T cells see the world,” he says.
“In a nutshell, we’ve learned the rules of the way T cells see the world,” he says.
Originally published at https://ccr.cancer.gov.
Names mentioned
Grégoire Altan-Bonnet, Ph.D., Senior Investigator in the Laboratory of Integrative Cancer Immunology, in collaboration with
Paul François’ group at McGill University in Montréal.
REFERENCE PUBLICATION
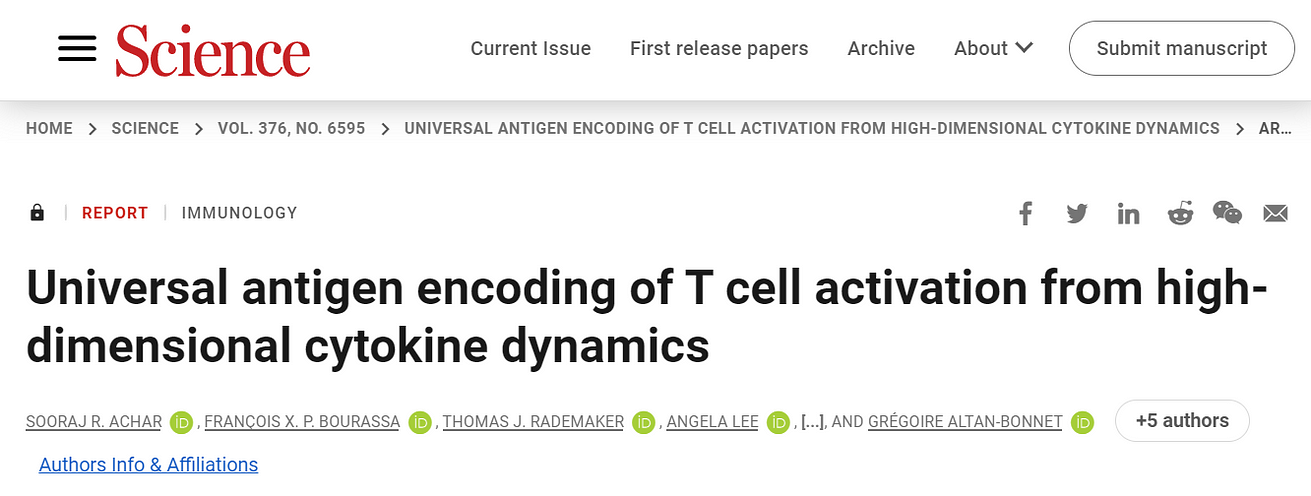
Universal antigen encoding of T cell activation from high-dimensional cytokine dynamics
Science
SOORAJ R. ACHAR ,
FRANÇOIS X. P. BOURASSA
THOMAS J. RADEMAKER
ANGELA LEE
TAISUKE KONDO
EMANUEL SALAZAR-CAVAZOS
JOHN S. DAVIES
NAOMI TAYLOR
PAUL FRANÇOIS
AND GRÉGOIRE ALTAN-BONNET
19 May 2022
How T cells respond to antigens
Although they are important tools in immunotherapy, we still have an incomplete understanding of how T cells respond to antigens in different temporal and molecular contexts.
Achar et al. combined robotic sampling of mouse and human T cell responses over time with machine learning and modeling to gain insights into how T cells process information derived from the particular antigens to which they are exposed (see the Perspective by Nourmohammad).
The analysis showed that patterns of cytokine release carried information about the type of antigen encountered and distinguished six distinct cellular responses rather than the three types usually recognized. Such understanding is likely to enhance strategies for immunotherapies that rely on altered and designed T cell responses. — LBR
Abstract
- Systems immunology lacks a framework with which to derive theoretical understanding from high-dimensional datasets.
- We combined a robotic platform with machine learning to experimentally measure and theoretically model CD8+ T cell activation.
- High-dimensional cytokine dynamics could be compressed onto a low-dimensional latent space in an antigen-specific manner (so-called “antigen encoding”).
- We used antigen encoding to model and reconstruct patterns of T cell immune activation.
- The model delineated six classes of antigens eliciting distinct T cell responses.
- We generalized antigen encoding to multiple immune settings, including drug perturbations and activation of chimeric antigen receptor T cells.
- Such universal antigen encoding for T cell activation may enable further modeling of immune responses and their rational manipulation to optimize immunotherapies.
Originally published at https://www.science.org