the health strategist
institute for research & strategy
Joaquim Cardoso MSc.
Chief Research and Strategy Officer (CRSO),
Chief Editor and Senior Advisor
August 14, 2023
What is the message?
This article, published by Jama, discusses the need for modernizing administrative risk adjustment models in healthcare using machine learning methods to improve accuracy and equity, highlighting the limitations of current models and providing recommendations for their enhancement.
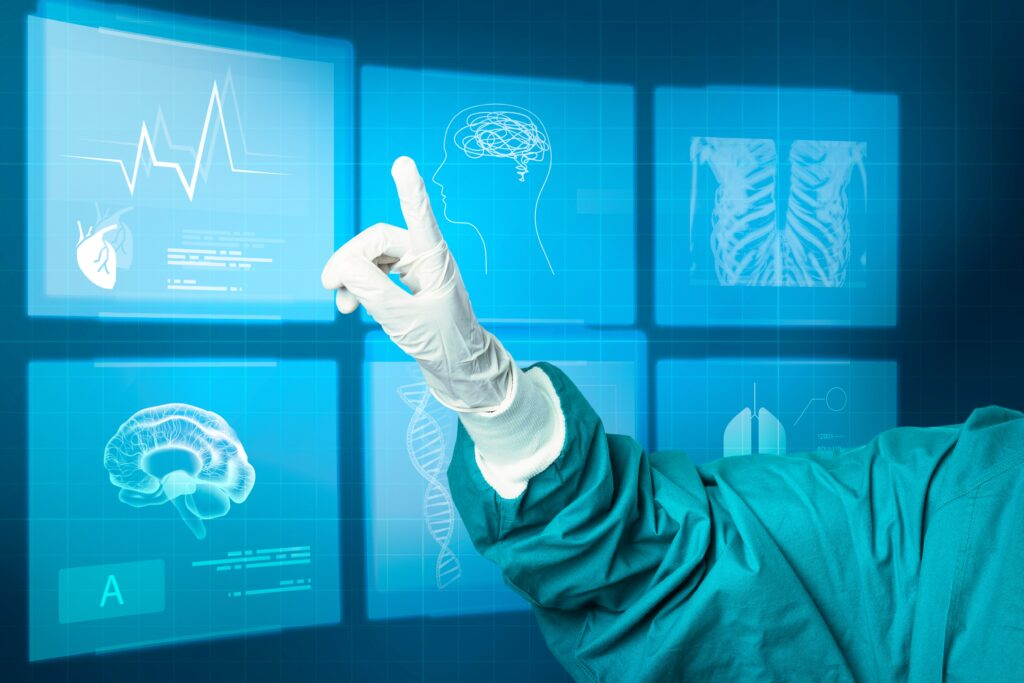
One page summary:
The executive summary highlights the critical need to update and modernize administrative risk adjustment models in healthcare through the integration of advanced machine learning methods.
These models play a crucial role in shaping healthcare spending, resource allocation, and promoting health equity.
- Despite the remarkable advancements in machine learning techniques, the existing risk adjustment models used within the Medicare program have stagnated for nearly two decades.
This viewpoint sheds light on the limitations of the current models, particularly in the context of Medicare, where they fail to accurately predict costs, particularly for patients with high levels of social risk and complex medical conditions.
The Centers for Medicare & Medicaid Services Hierarchical Condition Categories (CMS-HCC) model, a foundational administrative risk model developed in 2004, employs a hierarchy encompassing over 11,000 diagnostic codes grouped into 86 categories.
- While the coefficients representing the importance of each variable are updated annually, the underlying traditional regression approach to predict annual costs has remained unchanged.
Disturbingly, recent analysis reveals that the CMS-HCC model captures only 13% of the variance in observed costs and displays subpar performance in predicting hospital readmissions, with C statistics ranging from 0.5 to 0.6.
- Nonetheless, variations of the CMS-HCC model continue to underpin multiple programs within CMS, including quality reporting, Medicare Advantage payments, and value-based and alternative payment models.
The implications of these models extend significantly, potentially impacting the financial landscape for clinicians, hospitals, and participants in Medicare Advantage. In light of this, the article advocates for a comprehensive overhaul of these risk adjustment models, leveraging the potential of machine learning methods.
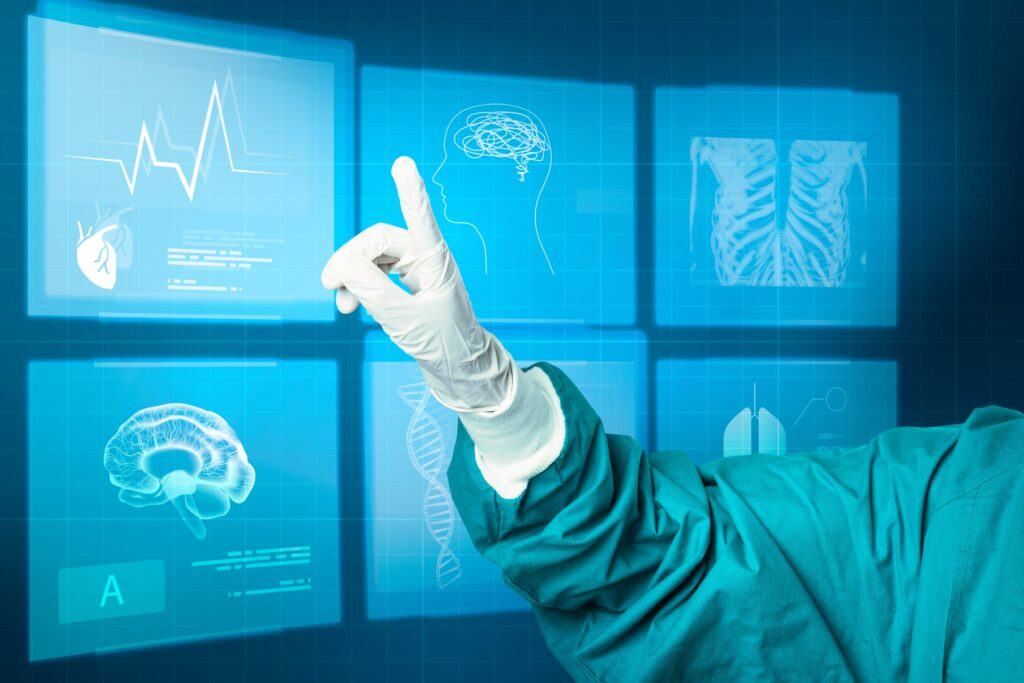
The recommendations for modernization via machine learning involve a multi-pronged approach.
- Firstly, machine learning techniques can effectively mitigate statistical bias in predictions, as their adaptable nature enables tailored modeling, which is particularly suited to complex healthcare datasets.
- A systematic assessment of diverse model types, such as ensembling, presents an opportunity for optimal fit to the data. Moreover, machine learning could also alleviate error due to variance, a phenomenon known as overfitting, which is crucial for translating models’ performance from training data to actual patient populations.
- The article underscores the importance of transparent model development. By enhancing the availability and accessibility of model codes, such as distributing them in programming languages like R or Python, biostatisticians and data scientists can effectively engage with and scrutinize the models.
- Similarly, adherence to Enhancing the Quality and Transparency of Health Research (EQUATOR) network guidelines can enhance transparency and scientific rigor in risk model development and validation.
- The integration of societal values into risk models, an aspect often overlooked, is also advocated. Incorporating social risk factors can potentially optimize risk models’ performance while ensuring that support is channeled towards underserved populations. However, the inclusion of such factors requires careful consideration, as it may inadvertently mask existing inequities.
- The need for transparency in the process of aligning policy goals with modeling choices is emphasized, ensuring that nuanced policy decisions are directly incorporated into risk models.
- In conclusion, the article urges the healthcare industry, specifically CMS, to harness the power of modern machine learning methods to transform risk adjustment models.
By aligning policy goals with analytic methods, these models can be modernized to achieve accurate predictions, transparent practices, and equitable healthcare resource allocation.
- Amidst the evolving landscape of machine learning practices, this transformation holds the promise of optimizing resource utilization while ensuring patient safety, transparency, and equity in healthcare delivery.
DEEP DIVE
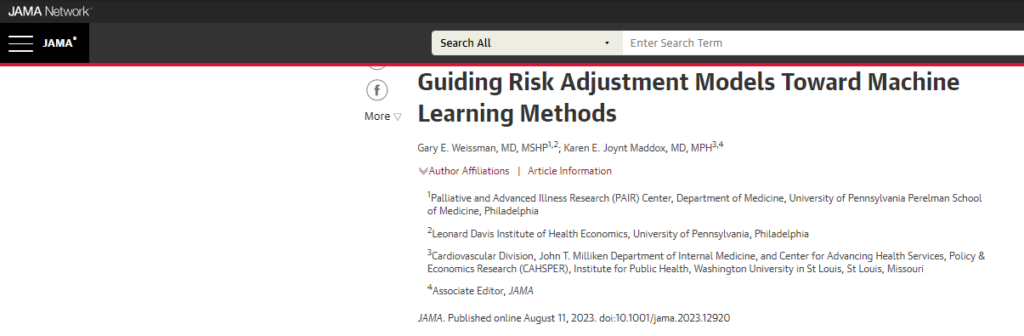
Guiding Risk Adjustment Models Toward Machine Learning Methods [excerpt]
JAMA Network
Gary E. Weissman, MD, MSHP and Karen E. Joynt Maddox, MD, MPH
August 11, 2023
Administrative risk adjustment models are widely used in health care and substantially affect spending, allocation of resources, and health equity. In the Medicare program, quality reporting, value-based and alternative payment models, and Medicare Advantage payments all rely on the same underlying model. However, despite advances in machine learning methods, here referring both to complex modeling approaches and to rigorous statistical practices developed by computer scientists and biostatisticians, the risk adjustment models used in Medicare have not been meaningfully updated. This Viewpoint reviews the current state of these models in the Medicare program and makes concrete suggestions for their modernization.
Historical Perspective
The Centers for Medicare & Medicaid Services Hierarchical Condition Categories (CMS-HCC) model is a paradigmatic administrative risk model first developed in 2004 to predict the health care costs of Medicare Advantage enrollees.1 The most recent version uses a hierarchy of more than 11 000 diagnostic codes grouped into 86 categories and accounts for a patient’s age, sex, disability status, and Medicaid status, among other factors.2 Although the coefficients (measures of each variable’s importance in the model) are updated annually, the underlying traditional regression approach used to predict annual costs has not changed in nearly 20 years.
The most recent analysis from CMS suggests room for improvement, because the CMS-HCC model captures only 13% of the variation in observed costs.3 Further, the model systematically underpredicts costs for beneficiaries with high levels of social risk and high medical complexity.
The model has particularly poor performance for predicting hospital readmissions, with C statistics ranging from 0.5 to 0.6. Despite these limitations, the CMS-HCC model, or variations of it containing different combinations of comorbidity and demographic data, supports several CMS programs. These include reimbursements for Medicare Advantage and for quality and cost measures within all of Medicare’s value-based and alternative payment models, such as the Medicare Shared Savings Program and the Bundled Payments for Care Improvement–Advanced program.
Improvements to the model could therefore have major financial and equity implications for clinicians and hospitals that participate in mandatory or voluntary payment models, as well as for Medicare Advantage participants.
Recommendations for Modernization via Machine Learning
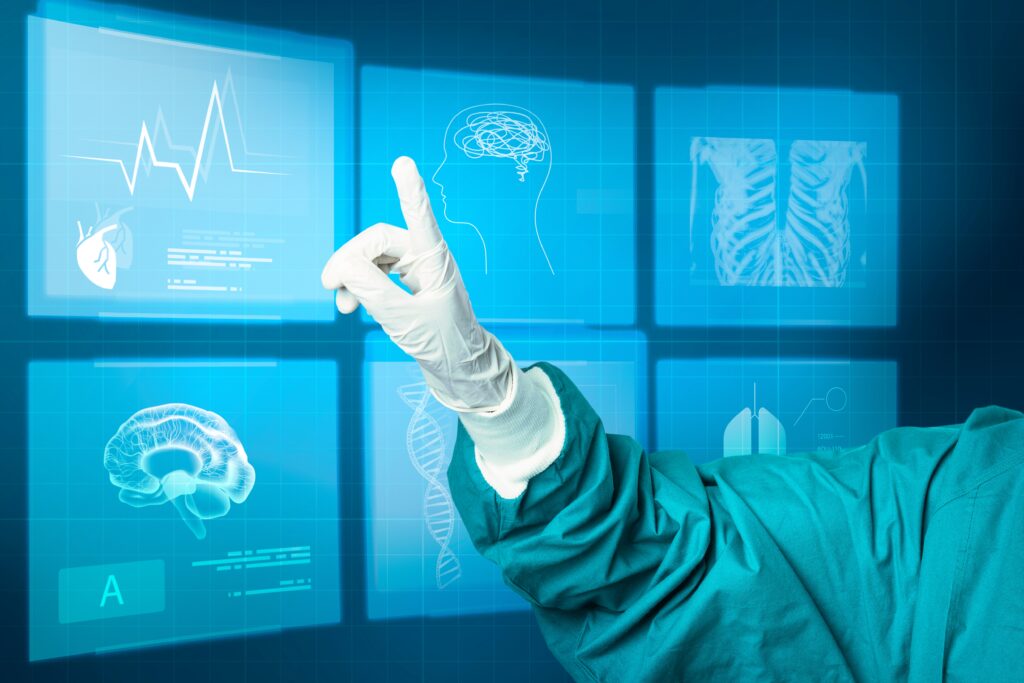
The past decade has witnessed a surge in complex modeling approaches, statistical methods, and the wide availability of statistical software, which have all contributed to advances in predictive modeling tools. With machine learning, models can identify relevant interactions between variables without needing to specify them and can incorporate diverse data sources such as text and images. Prior attempts to improve administrative risk models have incorporated the timing of disease onset (for example, has a patient had heart failure for a year, or is it a new diagnosis), fit less flexible models so they perform better in new data sets, and used complex model types such as neural networks, which learn interactions between disease states and other comorbidities.
Although each of these approaches resulted in some performance improvement, such advanced methods will not have widespread impact unless implemented in a standardized fashion and in concert with several other machine learning best practices. Further, such a process must be guided simultaneously by attention to policy goals, including safety, transparency, and equity.
First, machine learning could improve risk models by reducing statistical bias in the predictions. This requires a more tailored approach to modeling, which can be better achieved with modern machine learning methods than with more traditional approaches. A systematic assessment of many model types, such as those using ensembling—the aggregation of many models at once—may provide a more optimal fit to the underlying data. Relatedly, the best model specification for one scenario may not be ideal for another. For example, the optimal model for predicting health care costs may not be the same as that for mortality or readmission risk.
The optimal approach may also vary by population, where the health and behavior of enrollees may differ, such as between fee-for-service and Medicare Advantage beneficiaries. In each context, how input variables interact with each other, model complexity balanced against the need for interpretability and dissemination, and the likelihood of gaming should be evaluated on a case-by-case basis.
Second, machine learning could improve risk models by reducing error due to variance, or the error that becomes apparent when a model that performs well in the data set on which it was trained is applied to a new data set in which the performance is worse. This phenomenon, known as overfitting, is mitigated with the use of large administrative data sets but becomes more likely as a model’s complexity increases.
Standardizing the use of more advanced statistical practices will ensure that risk models perform well not just in training data but also in their intended populations. This is especially important as models like CMS-HCC are trained using CMS fee-for-service claims but are used to make predictions for Medicare Advantage enrollees, which has had the unintended effect of leading to significant overpayment in the Medicare Advantage program.
Third, a rigorous evaluation of a model’s performance requires choosing suitable performance measures. Although no single measure can capture all aspects of a model’s performance, calibration—how closely the predicted values align with observed outcomes—is most aligned with the task of risk adjustment. Thus, requiring that all risk models are reported using best practices for calibration assessment,6 in addition to other discrimination and composite measures, would provide a more complete view of performance.
Fourth, increasing transparency of every step in the model development process would promote public engagement and accountability. For example, the CMS-HCC model is published with SAS code but would be more readily usable by biostatisticians and data scientists if also distributed using R or Python, the programming languages most widely used for machine learning.
Furthermore, requiring that risk model development and validation are reported according to Enhancing the Quality and Transparency of Health Research (EQUATOR) network guidelines would foster transparency and enhance scientific rigor.
Last, the explicit justification for how societal values are incorporated into risk models would further promote transparency and facilitate public discourse about sensitive policy decisions. For example, including social risk factors may improve the performance of risk models and allocate needed financial support to clinicians, hospitals, or insurance plans caring for disadvantaged populations.
On the other hand, the inclusion of such risk factors may mask inequities as they have in other domains such as pulmonary function testing, which may be counterproductive if resources are allocated according to need. It is also true that perfect prediction may not be the goal; for example, overpredicting, and therefore overpaying, for particularly high-risk populations may further incent clinicians to care for them.
Thus, explicitly weighting errors for overprediction relative to underprediction in the model training process could ensure that these policy choices are incorporated directly into risk models. A transparent process linking nuanced policy goals to modeling choices, and their related trade-offs, is needed.
It is time that modern machine learning methods are brought to bear on payment and resource allocation decisions that affect millions of lives and billions of dollars each year. Despite the current environment of regulatory and scientific uncertainty around best practices for machine learning, CMS has an opportunity to lead in aligning policy goals with analytic methods. Modernizing risk adjustment models would promote the efficient use of resources while ensuring safety, transparency, and equity for all patients.
Article Information
See the original publication (this is an excerpt version)
References
See the original publication (this is an excerpt version)
Authors and Affiliations
Gary E. Weissman, MD, MSHP1,2; Karen E. Joynt Maddox, MD, MPH3,4
1Palliative and Advanced Illness Research (PAIR) Center, Department of Medicine, University of Pennsylvania Perelman School of Medicine, Philadelphia
2Leonard Davis Institute of Health Economics, University of Pennsylvania, Philadelphia
3Cardiovascular Division, John T. Milliken Department of Internal Medicine, and Center for Advancing Health Services, Policy & Economics Research (CAHSPER), Institute for Public Health, Washington University in St Louis, St Louis, Missouri
4Associate Editor, JAMA
Originally published at https://jamanetwork.com/journals/jama