the health strategist
institute for strategic health transformation
& digital technology
Joaquim Cardoso MSc.
Chief Research and Strategy Officer (CRSO),
Chief Editor and Senior Advisor
October 25, 2023
One page summary
The article “The Future of AI and Informatics in Radiology: 10 Predictions” by Curtis P. Langlotz outlines the transformative impact of artificial intelligence (AI) and informatics on the field of radiology. The article presents ten predictions for the future of AI in radiology, highlighting the profound changes that are reshaping the medical specialty.
What is the message?
AI and informatics are revolutionizing radiology, enabling significant advancements in diagnostic accuracy, efficiency, and patient care.
The integration of AI technologies is poised to redefine the roles of radiologists and enhance the quality of healthcare services provided.
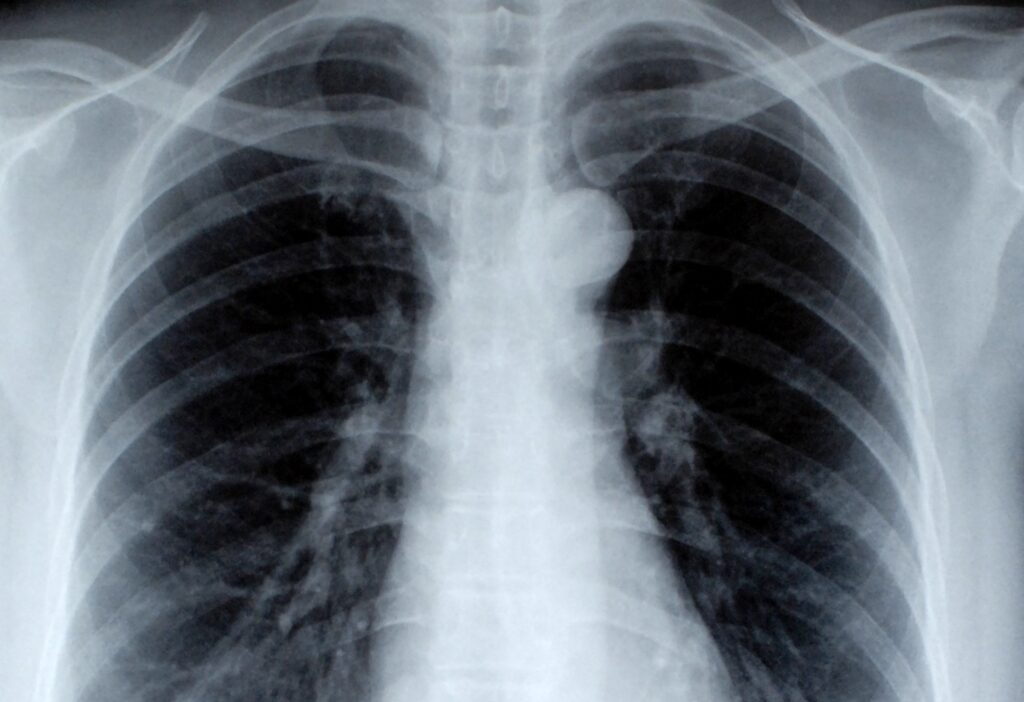
What are the 10 predictions?
1. Radiology Leading AI in Medicine: Radiology is at the forefront of AI in healthcare. Over 500 FDA-cleared AI algorithms, with about 75% designed for radiology, have emerged. Radiology, with its digital imaging data, is an ideal field for AI application due to its objectivity and the combination of images and text reports for machine learning.
2. Virtual Assistants for Radiology Reports: AI-based virtual assistants are set to draft radiology reports, alleviating radiologist burnout and enhancing productivity. These assistants will combine computer vision for image analysis and large language models for text generation.
3. Unified Image Interpretation Cockpit: Advances in cloud computing and storage are enabling the integration of image display, reporting, and AI into a unified radiology workstation. This will streamline radiologist workflows and improve collaboration with clinical colleagues.
4. Highly Sensitive AI Reducing Human Interpretation: AI is becoming highly sensitive, reducing the need for human image interpretation in certain cases, although it won’t replace radiologists. Human-AI collaborations will enhance diagnostic accuracy.
5. Language Models for Patient Understanding: Language models (LLMs) are being deployed to provide patient-friendly explanations of radiology reports, improving patient comprehension and communication with radiologists.
6. Multimodal AI and Precision Health: AI is set to analyze multimodal data, linking imaging studies with genomics, clinical notes, and wearable device data. This will lead to new insights into disease staging and treatment, advancing precision health.
7. Online Image Exchange Reducing Costs: A seamless national network for internet-based image exchange will reduce repeat imaging, potentially saving over $200 million annually and improving patient care.
8. Reformed Regulations for AI in Healthcare: New regulatory frameworks will allow for the fine-tuning of AI algorithms on local data, accelerating improvements in care delivery.
9. Large-Scale Imaging Databases for AI: Initiatives such as MIDRC, N3C, and RadImageNet are addressing data limitations in medical imaging, paving the way for diverse and reliable medical AI algorithms.
10. Collaborative Academic Organizations Driving AI Innovation: Academic institutions with access to clinical data, interdisciplinary teams, and deep technical knowledge are expected to lead AI research and development in healthcare.
Conclusion
The article emphasizes that the rapid progress of AI in radiology is poised to transform the field in the next decade. Radiologists will benefit from AI by automating routine tasks, allowing them to focus on the intellectual aspects of their profession.
These advancements will not only enhance diagnostic accuracy but also improve patient care and reduce costs in the healthcare system. The potential for AI in radiology is vast and holds the promise of a brighter future for the field.
DEEP DIVE
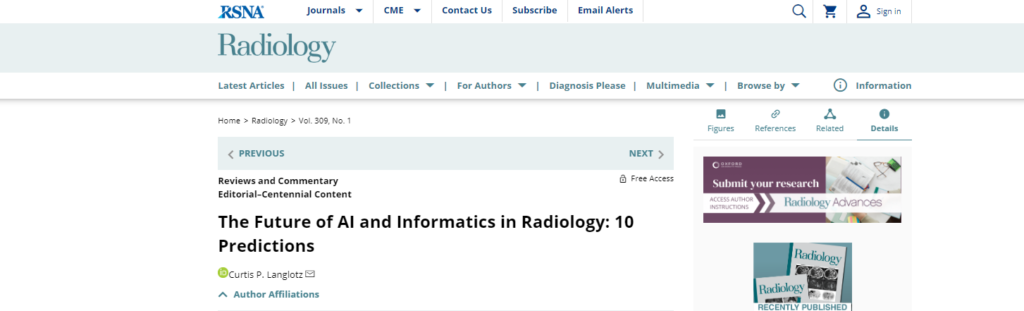
The Future of AI and Informatics in Radiology: 10 Predictions
RSNA
October 24, 2023
The computer as an intellectual tool can reshape the present system of health care, fundamentally alter the role of the physician, and profoundly change the nature of medical manpower recruitment and medical education.
William B. Schwartz, 1970 (1)
Introduction
Artificial intelligence (AI) and informatics are transforming radiology. Ten years ago, no expert would have predicted today’s vibrant radiology AI industry with over 100 AI companies and nearly 400 radiology AI algorithms cleared by the U.S. Food and Drug Administration (FDA). And less than a year ago, not even the savviest prognosticators would have believed that these algorithms could produce poetry, win fine art competitions, and pass the medical boards. Now, as we celebrate the centennial of our specialty’s flagship journal, Radiology, these accomplishments are part of our reality.
Moments of daunting transformation can liberate us to dream big. With that in mind, here are 10 predictions for the future of AI and informatics in radiology (Table).
![]() |
1. Radiology Will Continue to Lead the Way for AI in Medicine
In the current health care market, AI tools for radiologists dominate. Seventy-five percent of the over 500 FDA-cleared AI algorithms target radiology practice, increasing from 70% in 2021 (2). Radiology is a ripe target for AI because imaging data have been digital for decades. Our exabytes of digital imaging data are more objective than other clinical data because they are governed by the laws of physics rather than by subjective signs and symptoms expressed in a narrative note. And pairing images with descriptive text reports makes them ideal for the creation of accurate machine learning algorithms (3).
About 4% of diagnostic interpretations contain clinically significant errors (4). Errors arise because many image interpretation tasks are not well suited for human capabilities. For example, finding a small nodule nestled among the pulmonary vessels is akin to a “needle in a haystack.” Accurately quantifying abnormalities, such as the size of an irregularly shaped tumor or the amount of calcium in the coronary arteries, can also challenge human capabilities. Correlating complex multimodal clinical data sources, such as radiology, genomics, and pathology, may be beyond human capabilities. But AI algorithms can readily perform these tasks. Thus, AI researchers will continue to develop these new capabilities as a complement to human perception.
2. Virtual Assistants Will Draft Radiology Reports and Address Radiologist Burnout
Teaching trainees can be one of the most rewarding aspects of academic radiology. But teaching at the workstation can slow the productivity of attending radiologists (5). Yet, trainees provide powerful productivity advantages: They preview the imaging study, draft a report, edit it based on feedback, and route it to the attending radiologist for final signature, enabling attending radiologists to focus on the big picture—putting the findings in appropriate context (6).
Virtual assistants will bring these same efficiencies to radiologists who do not have the privilege of working with trainees. The combination of computer vision algorithms, which analyze images to identify findings, and large language models (LLMs), which are trained on massive data sets to generate text, will make this possible (Figure). Some computer vision algorithms can detect more than 70 findings in a single imaging study (7). Prompted by this list of findings, an LLM will draft a radiology report (8). Finally, the radiologist will edit and sign the report. The AI models could be periodically retrained from feedback obtained by comparing draft and final reports.

These AI-based virtual assistants will not only relieve the drudgery of dictating long radiology reports but will also upskill advanced practice providers to address the chronic radiologist shortage. These changes will occur first for repetitive studies, such as bedside chest radiographs, and then for a wide variety of common imaging studies.
3. An Intelligent Image Interpretation Cockpit Will Become as Pervasive as Email
When picture archiving and communication systems first became available, they required custom monitors, dedicated high-performance networks, and expensive bespoke storage devices. Early speech recognition systems required powerful desktop computers. And most medical record systems were still on paper. These disparate technologies, today the mainstay of the radiologist’s desktop, evolved separately and have never worked together well. Thus, it is not surprising that radiologists often work with disjointed system integrations and clashing user interfaces.
Recent technological progress makes a unified system possible. See, for example, the editorial on the maturing of imaging informatics by Chang in this issue of Radiology (9). Cloud computing and storage are just as secure as hospital data centers for storing health care data. Siri, Alexa, and Google already use cloud-based speech recognition. And AI algorithms can be deployed easily in the cloud. This progress sets the stage for a unified radiology workstation, with image display, reporting, and AI seamlessly integrated into a cloud-based cockpit (10) that dramatically improves radiologist efficiency. An automatically protocoled imaging study will arrive in the radiologist’s work queue preprocessed by AI algorithms, with patient history summarized, organs segmented and measured, abnormalities highlighted, and a report drafted. The radiologist will modify the report by using speech recognition or by clicking the image and choosing from a structured list of suggested imaging findings. These cloud-native capabilities will make virtual collaboration with clinical colleagues, including live video, immediate and seamless.
4. Highly Sensitive AI Will Reduce the Need for Human Image Interpretation
Until the advent of modern machine learning methods in the past few years, it was unthinkable that some radiology studies would never be viewed by human eyes. But many electrocardiogram (11) and Papanicolaou test (12) interpretations have been human-free for years. Recent research predicts that workflows combining human and AI expertise can forgo human review of 63% of screening mammograms while increasing overall accuracy (13). Because screening is only a small part of radiologist work, these systems may slow the growth of the radiologist workforce but will not displace radiologists.
5. LLMs Will Transform Patients’ Understanding of Radiology
Over the past year, LLMs have captivated the medical world, explaining medical guidelines (14), drafting radiology reports (8), and even passing board examinations (15). But LLMs have trouble with simple math (16), make potentially harmful errors in differential diagnosis (17), and sometimes authoritatively spout falsehoods (8). As we learn more about the capabilities of LLMs, we will likely find more problems that inhibit the regulatory clearance of these incredibly powerful tools for medical diagnosis (18).
But LLMs will soon be deployed for other radiology applications. Regulations against information blocking give patients ready access to their medical information (19). But the terminology radiologists use to communicate with requesting clinicians can mystify patients. The ability of LLMs to summarize information at an arbitrary reading level in the patient’s preferred language will help patients understand their reports (20). For example, here is the response of ChatGPT, an LLM developed by OpenAI, when asked to explain the circle of Willis at a fifth-grade reading level (21):
“The circle of Willis is a group of blood vessels in your brain that helps to provide blood to your brain and keep it healthy. It is shaped like a circle, and it is made up of several large blood vessels that are connected to each other. These blood vessels are called arteries, and they carry blood from your heart to your brain. The circle of Willis helps to make sure that your brain gets enough blood, even if one of the arteries becomes blocked or narrowed.”
These patient-friendly explanations of radiology reports will transform patients’ relationship with their imaging information and will bring radiologists and patients closer together.
6. Multimodal AI Will Discover New Uses for Diagnostic Images
The human visual perception system has evolved over millions of years to discern patterns; thus, AI models only rarely find patterns humans haven’t yet recognized. As a result, the best AI models exhibit performance comparable to expert humans on most visual tasks.
As the mountain of digital health data grows, massive multimodal data sets will link imaging studies to troves of data from genomics, clinical notes, laboratory values, and wearable devices (22). Self-supervised learning methods (23), which do not require expensive data labeling, will produce “generalist” models that encode relationships among many different data types. These models will uncover new associations beyond the capabilities of human information processing, showing how imaging appearances relate to specific genomic signatures and laboratory values.
Insights from multimodal data sets will change how we stage cancer and other complex diseases. For example, the TNM staging of cancer is scaled to fit the human memory system, relying on a small number of categories representing cancer size, location, and spread. Multimodal AI models will precisely quantify disease burden and produce more accurate predictions of disease course.
Likewise, the Response Evaluation Criteria in Solid Tumors, or RECIST, use antiquated methods to measure cancer progression, developed in part for their feasibility when radiologists measured with calipers on film-based images. Instead, AI methods that rapidly quantify total-body tumor burden will replace these primitive measures. Other quantification methods, sometimes called “opportunistic screening,” will process existing imaging studies at little additional cost, identifying markers of undiscovered chronic disease, such as coronary calcium, body composition, and spinal compression fractures (24,25).
New diagnostic associations, staging systems, and quantification methods will bring the advent of precision health. Using each patient’s data, AI-driven precision health will provide optimal patient-specific recommendations for disease prevention, diagnosis, and treatment.
7. Online Image Exchange Will Reduce Health Care Costs by over $200 Million Annually
Electronic image exchange avoids delays in care, improves patient satisfaction, and reduces costs, especially with urgently needed care (26). A trauma patient with available outside imaging uses 29% fewer imaging resources (27). Internet-based image exchange reduces imaging costs by $84.65 per transferred trauma patient (28). Portable media can’t solve this problem because patients with emergent conditions rarely bring images on CDs or DVDs unless transferred directly from another hospital. The United States spends $136.6 billion annually on emergency department care (29). About 8% of those health expenditures are for imaging (30), representing $10.9 billion. About 8% of this expense is for repeat imaging (31), or $872 million. A seamless national network of internet-based image exchange (32) could reduce this repeat imaging by 25% (31), or $218 million annually, in the emergency department alone.
8. Reformed Regulations Will Accelerate AI-based Improvements in Care Delivery
Over the past several years, the most accurate and generalizable AI systems have been trained on large diverse labeled data sets. Recent research suggests that pretraining on massive unlabeled data produces the most accurate systems (33). These systems, often called foundation models (34), can be fine-tuned on data from the deployment site to produce systems that are accurate for a wide range of tasks. These methods to optimize AI accuracy are on a collision course with medical software regulation. The U.S. FDA makes its decisions based on evidence from data about static products. The need to fine-tune foundational AI models to optimize their accuracy at a local site requires that products change after regulatory clearance. In the next decade, AI researchers, clinicians, ethicists, and regulators will devise flexible regulatory frameworks that allow monitoring and fine-tuning of algorithms on local data. The FDA’s proposed predetermined change control plans are a step in the right direction (35).
Difficulty in assembling large clinical data sets needed to train generalizable models is another impediment to progress. National regulatory reform, new local governance structures, and simple methods for patients to express their privacy preferences will foster a new era of open data for medical AI research.
9. A Widely Available Petabyte-scale Imaging Database Will Unleash Unbiased AI
The current wave of AI innovation was driven by a massive imaging database called ImageNet (36). This large set of labeled digital photographs created a benchmark for computer vision algorithms. Privacy risks and regulatory barriers limit the assembly of large medical image data sets, which impairs the diversity, reliability, fairness, and generalizability of medical AI algorithms (37).
New initiatives are addressing this problem. The Medical Imaging and Data Resource Center, or MIDRC, funded by the National Institute of Biomedical Imaging and Bioengineering, has published over 100 000 imaging studies from sites around the United States (38), serving as a model for large, diverse public medical data sets. The National COVID Cohort Collaborative, or N3C, is a similar national repository, aggregating the medical records of patients diagnosed with COVID-19 (39). The RadImageNet database has aggregated imaging studies from over 130 000 patients who underwent CT, MRI, and US examinations (40). The All of Us Research Program has invited 1 million people across the United States to help build one of the most diverse health databases in history (41). And organizations like the Medical Information Mart for Intensive Care, the RSNA, and academic research centers disseminate large data sets for the study of other important diseases (42–44).
These examples will spur health care organizations and patients to aggregate and share vast health data sets for the public good (32), enabling the creation of a medical ImageNet that reduces health disparities and catalyzes the next wave of AI innovation in health care.
10. Flexible and Collaborative Academic Organizations Will Lead AI Innovation
AI algorithms are more likely to be fair and trustworthy when developed by diverse interdisciplinary teams (45). Development of clinically useful algorithms requires not only clinicians who can identify important problems for AI to solve but also computer scientists who can interpret and apply the latest machine learning research. Practicing physicians with formal training in AI and machine learning often lead these highly effective teams. Rounding out these interdisciplinary groups will be ethicists, economists, and philosophers, who can assess the risks and benefits of new technologies and ensure fair algorithms.
Academic institutions will continue to lead AI research and development because of their immediate access to all the necessary raw materials: massive stores of accessible clinical data, a workforce of students with deep technical knowledge, abundant high-performance computing, research teams with interdisciplinary expertise, close partnerships with industry, and relationships with health care delivery systems that serve as showcases and testbeds for their innovations (46).
Conclusion
The breakneck progress of AI makes predictions for even the next 2 years extremely challenging. The next 10 years will bring even more surprises. But radiology, more than any other medical specialty, is poised to capitalize on the strengths of AI, saving us time by performing difficult, menial, or repetitive tasks. These new technologies will allow radiologists to focus on the rewarding work of placing findings in context for our clinical colleagues. Like previous cycles of innovation, highly capable AI tools will refocus radiologists on the intellectual activities that brought us to the profession in the first place.
Author and Affiliation
Curtis P. Langlotz – From the Departments of Radiology, Medicine, and Biomedical Data Science, Stanford University School of Medicine, 300 Pasteur Dr, Stanford, CA 94305.
Originally published at https://pubs.rsna.org