the health strategist
institute for strategic health transformation
& digital technology
Joaquim Cardoso MSc.
Chief Research and Strategy Officer (CRSO),
Chief Editor and Senior Advisor
October 31, 2023
What is the message?
Researchers at Stanford University have developed a tool using GPT-4, a large language model, to provide feedback on scientific manuscripts, addressing the challenges in the peer review process and offering a complementary approach to human review.
This tool aims to help researchers, particularly young researchers and those from less well-known institutions, improve their draft manuscripts before official submission to conferences and journals.

What are the key points?
- Peer Review Challenge: The article highlights the issue of a shortage of qualified peer reviewers to evaluate scientific studies, especially affecting young researchers and those from less-established institutions. Many studies are desk rejected without undergoing peer review.
- GPT-4 and Dataset: The researchers at Stanford used the GPT-4 language model and a dataset of thousands of previously published scientific papers, including reviewer comments, to create a tool for “pre-reviewing” draft manuscripts.
- Aim of the Tool: The tool’s purpose is to assist researchers in refining their drafts before submitting them to conferences and journals. The goal is to provide valuable feedback to help improve the quality of scientific papers.
- Comparison with Human Reviewers: The researchers compared the feedback generated by GPT-4 with comments from human peer reviewers. They found that there was a significant overlap between GPT-4’s comments and those of human reviewers.
- User Study: A user study involving researchers from over 100 institutions showed that more than half of the participants found GPT-4’s feedback “helpful/very helpful,” and 82 percent considered it “more beneficial” than certain feedback from some human reviewers.
- Limitations: The article acknowledges some limitations of GPT-4, such as providing more generic feedback and not delving into deep technical challenges. It tends to focus on specific aspects of scientific feedback.
- Complementary Role: The researchers emphasize that GPT-4 is not meant to replace human peer review but rather complement it. They believe that AI feedback can be especially valuable for early-stage paper writing when obtaining timely expert feedback can be challenging.
DEEP DIVE
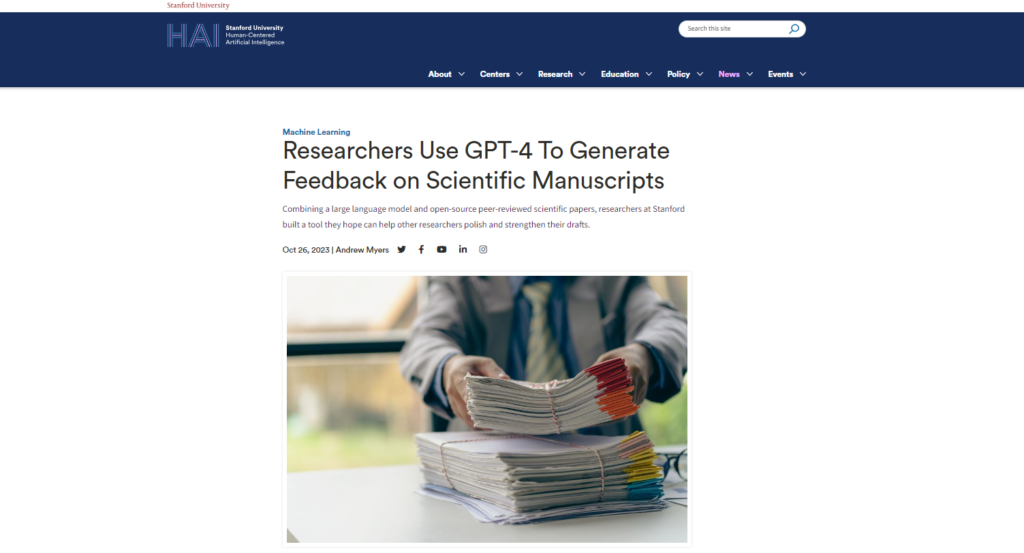
Researchers Use GPT-4 To Generate Feedback on Scientific Manuscripts
Stanford University Human-Centered Artificial Intelligence
Andrew Myers
October 26, 2023
Combining a large language model and open-source peer-reviewed scientific papers, researchers at Stanford built a tool they hope can help other researchers polish and strengthen their drafts.

Scientific research has a peer problem. There simply aren’t enough qualified peer reviewers to review all the studies. This is a particular challenge for young researchers and those at less well-known institutions who often lack access to experienced mentors who can provide timely feedback. Moreover, many scientific studies get “desk rejected” — summarily denied without peer review.
Sensing a growing crisis in an era of increasing scientific study, AI researchers at Stanford University have used the large language model GPT-4 and a dataset of thousands of previously published papers — replete with their reviewer comments — to create a tool that can “pre-review” draft manuscripts.
“Our hope is that researchers can use this pipeline to improve their drafts prior to official submission to conferences and journals,” said James Zou, an assistant professor of biomedical data science at Stanford and a member of the Stanford Institute for Human-Centered AI (HAI). Zou is the senior author of the study, recently published on preprint service arXiv.
Numbers Don’t Lie
The researchers began by comparing comments made by a large language model against those of human peer reviewers. Fortunately, one of the foremost scientific journals, Nature, and its fifteen sub-journals (Nature Medicine, etc.), not only publishes hundreds of studies a year but includes reviewer comments for some of those papers. And Nature is not alone. The International Conference on Learning Representations (ICLR) does the same with all papers — both accepted and rejected — for its annual machine learning conference.
“Between the two, we curated almost 5,000 peer-reviewed studies and comments to compare with GPT-4’s generated feedback,” Zou says. “The model did surprisingly well.”
The numbers resemble a Venn diagram of overlapping comments. Among the 3,000 or so Nature-family papers in the study, there was intersection between GPT-4 and human comments of almost 31 percent. For ICLR, the numbers were even higher, almost 40 percent of comments by GPT-4 and humans overlapped. What’s more, when looking only at the ICLR’s rejected papers (i.e., less mature papers) the overlap in comments between GPT-4 and humans grew to almost 44 percent — nearly half of all GPT-4 and human comments overlapped.
The significance of these numbers comes into sharper focus in light of the fact that even among humans there is considerable variation among comments by any given paper’s multiple reviewers. Human-to-human overlap was 28 percent for Nature journals and about 35 percent for ICLR. By these metrics, GPT-4 performed comparably to humans.
But while computer-to-human comparisons are instructive, the real test is whether the reviewed paper’s authors valued the comments provided by either review method. Zou’s team conducted a user study where researchers from over 100 institutions submitted their papers, including many preprints, and received GPT-4’s comments. More than half of the participating researchers found GPT-4 feedback “helpful/very helpful” and 82 percent found it “more beneficial” than certain feedback from some human reviewers.
Limits and Horizons
There are caveats to the approach, Zou is quick to highlight in the paper. Notably, GPT-4’s feedback can sometimes be more “generic” and may not pinpoint the deeper technical challenges in the paper. GPT-4 also has the tendency to focus only on limited aspects of scientific feedback (i.e., “add experiments on more datasets”) and comes up short on in-depth Insights on the authors’ methods.
Zou was further careful to emphasize that the team is not suggesting that GPT-4 take the “peer” out of peer review and replace human review. Human expert review “is and should continue to be” the basis of rigorous science, he asserts.
“But we believe AI feedback can benefit researchers in early stages of their paper writing, particularly when considering the growing challenges of getting timely expert feedback on drafts,” Zou concludes. “In that light, we think GPT-4 and human feedback complement one another quite well.”
Originally published at https://hai.stanford.edu/news