the health strategist
institute for continuous health transformation
and digital health
Joaquim Cardoso MSc
Chief Researcher, Editor and Senior Advisor
January 4, 2023
Executive Summary
- According to a study published in Nature Medicine and funded by the National Institutes of Health’s Researching COVID to Enhance Recovery (RECOVER) initiative, …
- … long COVID, also known as post-acute SARS-CoV-2 infection (PASC), has four major subtypes defined by different clusters of symptoms.
- The study dissects the complexity and heterogeneity of newly incident conditions 30–180 days after SARS-CoV-2 infection confirmation into four reproducible subphenotypes based on the EHR repositories from two large CRNs using machine learning.
- These four subphenotypes also covered the major PASC conditions that have been reported from existing independent studies, such as cardiovascular3, respiratory25, neurological26and gastrointestinal27conditions.
- Through machine learning analysis of over 137 symptoms and conditions, we identified four reproducible PASC subphenotypes, dominated by
- (1) cardiac and renal (including 33.75% and 25.43% of the patients in the development and validation cohorts);(2) respiratory, sleep and anxiety (32.75% and 38.48%);
- (3) musculoskeletal and nervous system (23.37% and 23.35%); and
- (4) digestive and respiratory system (10.14% and 12.74%) sequelae.
- Researchers used a machine-learning algorithm to identify symptom patterns in the health records of nearly 35,000 US patients who tested positive for SARS-CoV-2 infection and later developed lingering long-COVID-type symptoms.
- These subphenotypes were associated with distinct patient demographics, underlying conditions before SARS-CoV-2 infection and acute infection phase severity.
- These findings may inform ongoing research on the potential mechanisms of long COVID and potential treatments for it.
Infographic
Fig. 1: Data curation and the subphenotyping pipeline.
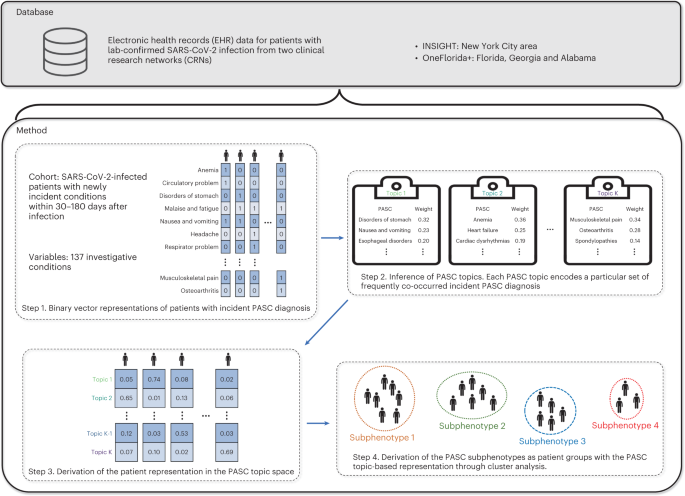
Fig. 2: Heat map of PASC topics learned from the INSIGHT cohort.
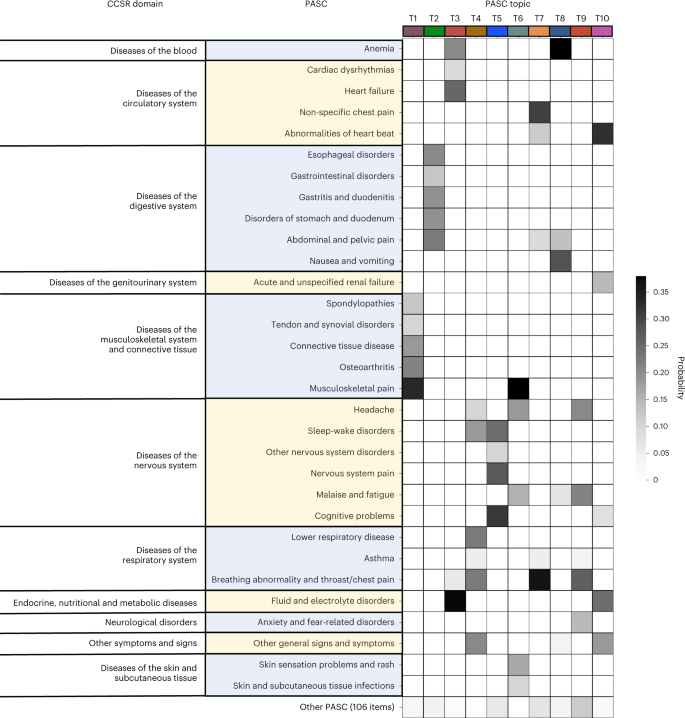
Fig. 4: Differences in incidence patterns of selected PASC conditions (grouped by CCSR domains) in 30–180 days after COVID-19 lab test between positive and matched negative patients on the INSIGHT cohort.
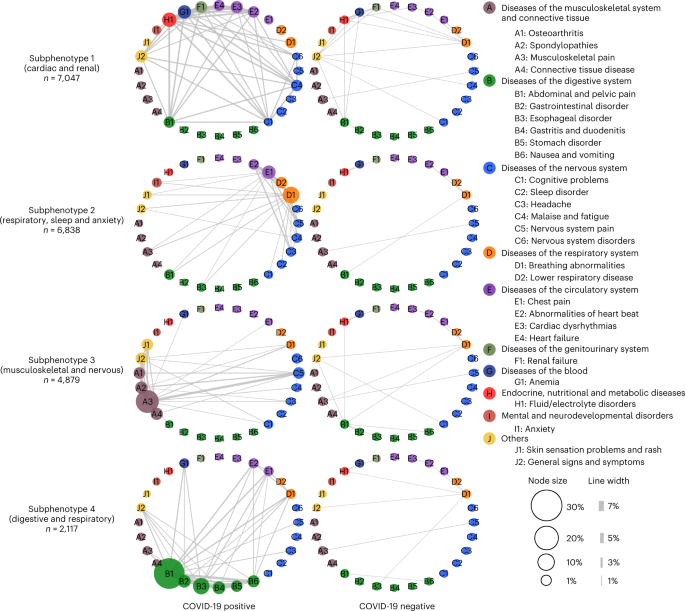
ORIGINAL PUBLICATION
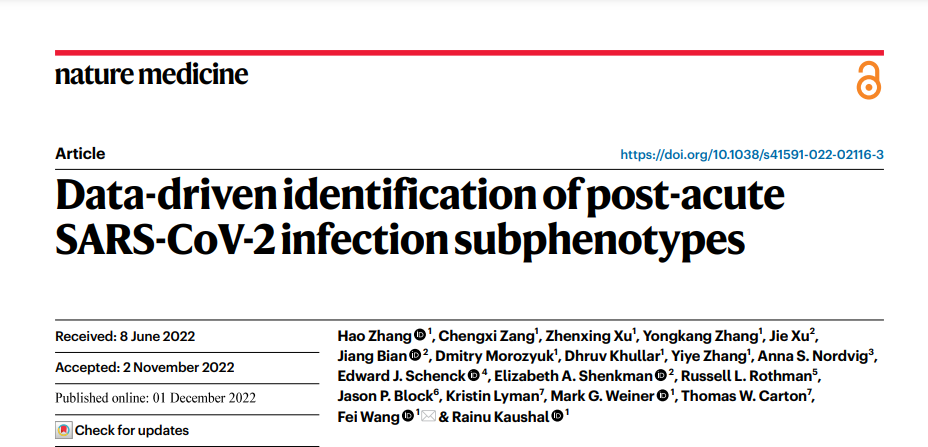
Data-driven identification of post-acute SARS-CoV-2 infection subphenotypes
Nature Medicine
Hao Zhang, Chengxi Zang, Zhenxing Xu, Yongkang Zhang, Jie Xu, Jiang Bian, Dmitry Morozyuk, Dhruv Khullar, Yiye Zhang, Anna S. Nordvig, Edward J. Schenck, Elizabeth A. Shenkman, Russell L. Rothman, Jason P. Block, Kristin Lyman, Mark G. Weiner, Thomas W. Carton, Fei Wang & Rainu Kaushal
December 1, 2022
Abstract
- The post-acute sequelae of SARS-CoV-2 infection (PASC) refers to a broad spectrum of symptoms and signs that are persistent, exacerbated or newly incident in the period after acute SARS-CoV-2 infection.
- Most studies have examined these conditions individually without providing evidence on co-occurring conditions.
- In this study, we leveraged the electronic health record data of two large cohorts, INSIGHT and OneFlorida+, from the national Patient-Centered Clinical Research Network.
- We created a development cohort from INSIGHT and a validation cohort from OneFlorida+ including 20,881 and 13,724 patients, respectively, who were SARS-CoV-2 infected, and we investigated their newly incident diagnoses 30–180 days after a documented SARS-CoV-2 infection.
- Through machine learning analysis of over 137 symptoms and conditions, we identified four reproducible PASC subphenotypes, dominated by
- (1) cardiac and renal (including 33.75% and 25.43% of the patients in the development and validation cohorts);
- (2) respiratory, sleep and anxiety (32.75% and 38.48%);
- (3) musculoskeletal and nervous system (23.37% and 23.35%); and
- (4) digestive and respiratory system (10.14% and 12.74%) sequelae.
- These subphenotypes were associated with distinct patient demographics, underlying conditions before SARS-CoV-2 infection and acute infection phase severity.
- Our study provides insights into the heterogeneity of PASC and may inform stratified decision-making in the management of PASC conditions.

Main
The ongoing global pandemic of Coronavirus Disease 2019 (COVID-19) caused by severe acute respiratory syndrome coronavirus 2 (SARS-CoV-2) infection has impacted hundreds of millions of people’s lives. Existing studies have provided evidence that many symptoms and signs could be persistent, exacerbated or newly present after the acute phase of SARS-CoV-2 infection, referred to as post-acute sequelae of SARS-CoV-2 infection (PASC)1,2, which involve multiple organ systems, including cardiovascular3, mental4, metabolic5, renal6and others. There have been various ongoing efforts into investigating the underlying biological mechanisms of PASC7,8,9, which have typically been conducted in small patient cohorts. Large-scale clinical observational cohort studies can provide useful insights into PASC that may help develop effective mechanistic hypotheses and inform targeted treatments. Most existing observational studies investigated the PASC conditions individually (for example, by examining the incidence10, excess burden11or prevalence12of each symptom or condition in the post-acute period for patients infected with SARS-CoV-2 relative to non-infected individuals). Their co-appearance patterns (or subphenotypes)-that is, to what extent PASC symptoms and conditions co-appear or develop disproportionately in certain patient populations-can potentially help reveal the pathophysiology behind PASC by disentangling their phenotypical heterogeneities. Existing studies on this topic have been limited, with one study that identified PASC subphenotypes from the reported symptoms of a cohort including 233 patients with COVID-19 (ref. 13). More comprehensive analysis involving broader sets of PASC conditions with larger patient cohorts is needed.
We developed a machine learning approach to derive PASC subphenotypes based on newly incident conditions in the post-acute SARS-CoV-2 infection period (defined as 30–180 days after the confirmed infection) of patients with COVID-19. We leveraged the electronic health record (EHR) repositories of two large clinical research networks (CRNs) from the national Patient-Centered Clinical Research Network (PCORnet): the INSIGHT network14, which includes 12 million patients in the New York City (NYC) area, and the OneFlorida+ network15, which includes 19 million patients from Florida, Georgia and Alabama. We examined the incidence of 137 diagnosis categories derived from the Clinical Classifications Software Refined (CCSR) categories16that were potentially related to PASC, and we leveraged a topic modeling (TM) approach17to learn the co-incidence patterns of these diagnosis conditions, based on which the PASC subphenotypes were derived by clustering.
Results
See the original publication (this is an excerpt version)

Discussion
Several studies have found that PASC could include a diverse set of symptoms and signs involving many organ systems10,11,12.
Unlike existing research that has studied these conditions independently, we developed a data-driven framework to identify subphenotypes of SARS-CoV-2-infected patients based on newly incident signs and symptoms 30–180 days after the date of confirmed infection. With the EHR from INSIGHT and OneFlorida+ CRNs, we identified four subphenotypes dominated by new conditions of the cardiac and renal systems (Subphenotype 1); respiratory system, sleep and anxiety problems (Subphenotype 2); musculoskeletal and nervous systems (Subphenotype 3); and digestive and respiratory systems (Subphenotype 4).
In both cohorts, Subphenotypes 1 and 2 are the largest two subphenotypes. Subphenotype 1 contains 33.75% and 25.43% of the patients in INSIGHT and OneFlorida+, respectively.
It includes older patients with more baseline comorbidities, greater severity of acute illness in medical utilizations and higher proportion of males23. Many patients in this subphenotype were confirmed with SARS-CoV-2 infection during the early pandemic (March-September 2020) when NYC was the epicenter, which may explain the observation that its size is larger for INSIGHT than OneFlorida+. Early cases had greater acute phase severity as treatment protocols were still evolving, which may explain more severe incident conditions in the post-acute infection period of these patients, possibly caused by hyperinflammation24. Subphenotype 2 occupies 32.75% and 38.48% of the patients in INSIGHT and OneFlorida+. It includes younger patients who had SARS-CoV-2 infection confirmed mostly during July-November 2021.
Subphenotypes 3 and 4 were less prevalent. Subphenotype 3 included musculoskeletal and neurological conditions, whereas Subphenotype 4 was associated with gastrointestinal conditions.
Patients in Subphenotype 3 also displayed dermatologic conditions and had the highest rates of related conditions at baseline, including autoimmune diagnoses such as rheumatoid arthritis and allergy conditions. Patients in Subphenotype 4 had the mildest acute phase severity (for example, lowest rates of mechanical ventilation and critical care admissions).
Our results suggest that the identified subphenotypes are highly consistent across the two cohorts with distinct patient populations and geographical characteristics.
These four subphenotypes also covered the major PASC conditions that have been reported from existing independent studies, such as cardiovascular3, respiratory25, neurological26and gastrointestinal27conditions.
Our study verified the co-existence of these dominate subphenotypes and can inform focused disease areas of treatment development for PASC.
These four subphenotypes also covered the major PASC conditions that have been reported from existing independent studies, such as cardiovascular3, respiratory25, neurological26and gastrointestinal27conditions.
There is also an existing study on identifying Long-COVID symptom clusters with information reported from 233 patients enrolled in the All-Ireland Infectious Disease cohort13, whereas our study is based on the diagnosis information from the EHR of large general civilian patient populations.
Some of these diagnoses were with a clear diagnostic criterion, whereas others were not.
For example, the conditions in Subphenotype 1, such as heart failure, pneumonia and renal failure, were mostly with objective diagnostic criteria according to underlying disease etiologies. Many conditions in Subphenotype 2 (such as breathing abnormality and non-specific chest pain), Subphenotype 3 (such as musculoskeletal and nervous system pain) and Subphenotype 4 (such as abdominal and pelvic pain, nausea and vomiting) were more subjective to diagnose. In addition, the diagnosis of certain conditions, such as esophageal and gastrointestinal disorders in Subphenotype 4, was likely to encompass functional disorders rather than clearly defined disease etiologies. This meant that our identified subphenotypes, which separated severe COVID-19 complications (Subphenotype 1) and milder PASC conditions (Subphenotypes 2, 3 and 4) that could not be explained by alternative disease etiologies and were closer to those patient-reported symptoms. These subphenotypes would help tease out the heterogeneity of these conditions and provide guidance on patient management in practice.
Our study has several strengths.
First, we adopted a TM approach to derive compact patient representations based on their co-incidence patterns across different diagnoses. Unlike other dimensionality reduction techniques, such as principal component analysis (PCA)28, TM is designed specifically for data samples with binary or count features29,30and, thus, would be appropriate for our analysis. Second, INSIGHT and OneFlorida+ include patients from distinct geographic regions in the United States with different characteristics, allowing us to validate the robustness of the derived subphenotypes. Third, our study period (March 2020 to November 2021) covers different COVID-19 waves associated with different SARS-CoV-2 virus variants.
Our study also has limitations.
First, our analysis is based on longitudinal observational patient data, which cannot explain the biological mechanisms behind PASC. Second, the PASC diagnoses that we investigated were encoded as CCSR categories, which may not reflect the co-incidence patterns of fine-grained diagnosis conditions. Third, we focused on new incidences of conditions in the post-acute infection period for patients with COVID-19 and did not evaluate pre-existing conditions that may persist or worsen due to acute SARS-CoV-2 infection. Fourth, the goal of our study was to identify potential PASC subphenotypes, and we did not conduct rigorous analysis on the predictability of these subphenotypes, which was left as a future research topic. Finally, our study period did not include the COVID-19 wave dominated by the Omicron SARS-CoV-2 variant.
In conclusion, our study dissects the complexity and heterogeneity of newly incident conditions 30–180 days after SARS-CoV-2 infection confirmation into four reproducible subphenotypes based on the EHR repositories from two large CRNs using machine learning.
These findings could be useful for clinicians and health systems in developing care models to meet the needs of patients with PASC.
In conclusion, our study dissects the complexity and heterogeneity of newly incident conditions 30–180 days after SARS-CoV-2 infection confirmation into four reproducible subphenotypes based on the EHR repositories from two large CRNs using machine learning.
Methods
See the original publication
Acknowledgements
This research was funded by NIH Agreement Other Transactions Authority (OTA). OT2HL161847 (contract no. EHR-01–21) is part of the Researching COVID to Enhance Recovery (RECOVER) research program. The PCORnet study reported in this work was conducted using PCORnet, the National Patient-Centered Clinical Research Network. PCORnet was developed with funding from the Patient-Centered Outcomes Research Institute (PCORI). This work was conducted through use of data from the INSIGHT Clinical Research Network and supported, in part, by a PCORI PCORnet grant to the INSIGHT Clinical Research Network (grant no. RI-CORNELL-01-MC). The statements presented in this work are solely the responsibility of the author(s) and do not necessarily represent the views of other organizations participating in, collaborating with or funding PCORnet, or of PCORI.
Authors and Affiliations
Department of Population Health Sciences, Weill Cornell Medicine, New York, NY, USA
Hao Zhang, Chengxi Zang, Zhenxing Xu, Yongkang Zhang, Dmitry Morozyuk, Dhruv Khullar, Yiye Zhang, Mark G. Weiner, Fei Wang & Rainu Kaushal
Department of Health Outcomes Biomedical Informatics, University of Florida, Gainesville, FL, USA
Jie Xu, Jiang Bian & Elizabeth A. Shenkman
Department of Neurology, Weill Cornell Medicine, New York, NY, USA
Anna S. Nordvig
Department of Medicine, Division of Pulmonary and Critical Care Medicine, Weill Cornell Medicine, New York, NY, USA
Edward J. Schenck
Center for Health Services Research, Vanderbilt University Medical Center, Nashville, TN, USA
Russell L. Rothman
Department of Population Medicine, Harvard Pilgrim Health Care Institute, Harvard Medical School, Boston, MA, USA
Jason P. Block
Louisiana Public Health Institute, New Orleans, LA, USA
Kristin Lyman & Thomas W. Carton
Cite this article
Zhang, H., Zang, C., Xu, Z. et al. Data-driven identification of post-acute SARS-CoV-2 infection subphenotypes. Nat Med (2022). https://doi.org/10.1038/s41591-022-02116-3
Originally published at https://www.nature.com on December 1, 2022.