This is an excerpt of the article “Why is AI adoption in health care lagging?”, with a focus on the topic above, preceded by an Executive Summary by the author of the blog.
Brookings
Avi Goldfarb and Florenta Teodoridis
March 9, 2022
Executive Summary of the Excerpt
by Joaquim Cardoso MSc.
The AI Health Care Unit
@ The Digital Health Institute
March 13, 2022
The opportunity for AI in Health Care
- In 2019, 11% of American workers were employed in health care, and health care expenditures accounted for over 17% of gross domestic product.
- If AI technologies have a similar impact on healthcare as in other industries such as retail and financial services, then health care can become more effective and more efficient, improving the daily lives of millions of people.
- However, despite the hype and potential, there has been little AI adoption in health care.
Four important barriers to adoption are:
- algorithmic limitations,
- data access limitations,
- regulatory barriers, and
- misaligned incentives
The Misaligned incentives are described below.
Innovation in algorithmic transparency, data collection, and regulation are examples of the types of complementary innovations necessary before AI adoption becomes widespread.
In addition, another concern that we believe deserves equal attention is the role of decisionmakers.
- Not infrequently, medical professionals are the decisionmakers, and AI algorithms threaten to replace the tasks they perform.
- Why has Hinton’s prediction (“We should stop training radiologists now; it is just completely obvious deep learning is going to do better than radiologists.”) has not yet come to pass? The challenges include lack of trust in the algorithms, challenges in data collection, and regulatory barriers, as noted above.
- They also include a misalignment of incentives.
In our study analyzing AI adoption through job postings, we find that adoption indeed varies by type of job and by hospital management structure.
- AI skills are less likely to be listed in clinical roles than in administrative or research roles.
- Hospitals with an integrated salary model, which are more likely to be led by individuals who have focused their career on management and take a systematic approach to administration, have a higher rate of adoption of AI for administrative and clinical roles but not for research roles
However, we have seen that there are several reasons why AI adoption might be slow in hospitals.
- In other words, even if professional managers are more likely to adopt AI, they are not necessarily right to engage in adoption at this stage.
- … while it may be that doctor-led hospitals have not adopted AI because they view it as a threat to their jobs,
- it may also be that doctor-led hospitals have leaders who have a better grasp of the other adoption challenges-algorithmic limitations, data access limitations, and regulatory barriers.
ORIGINAL PUBLICATION (excerpt)
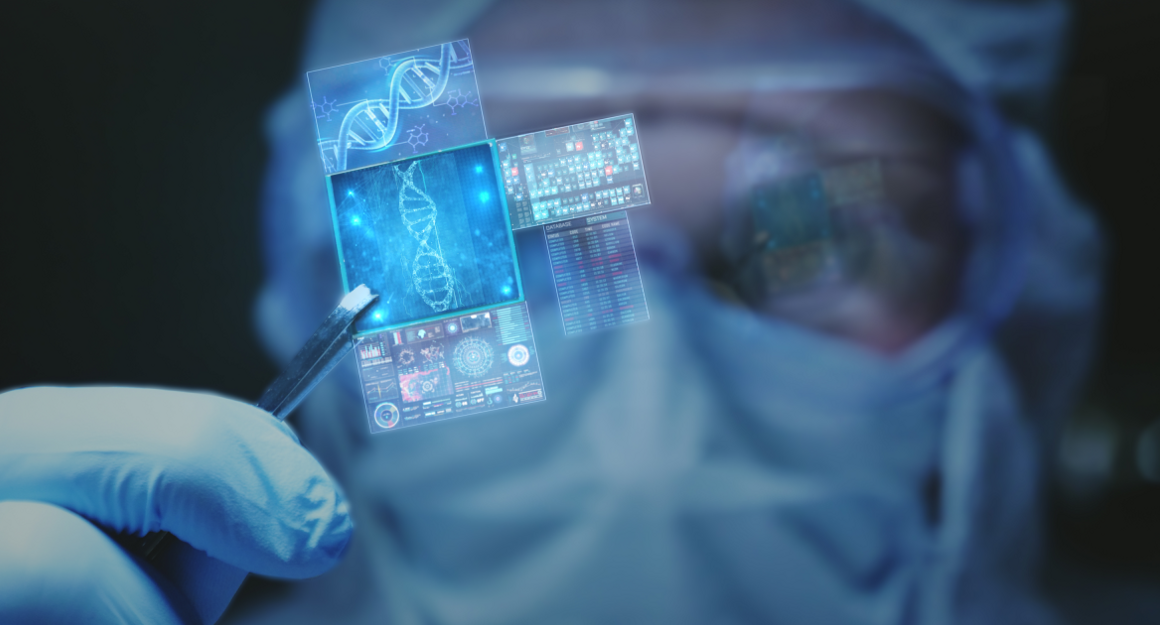
4.Misaligned incentives
Innovation in algorithmic transparency, data collection, and regulation are examples of the types of complementary innovations necessary before AI adoption becomes widespread.
In addition, another concern that we believe deserves equal attention is the role of decisionmakers.
In addition, another concern that we believe deserves equal attention is the role of decisionmakers.
There is an implicit assumption that AI adoption will accelerate to benefit society if issues such as those related to algorithm development, data availability and access, and regulations are solved.
However, adoption is ultimately dependent on health care decisionmakers.
Not infrequently, medical professionals are the decisionmakers, and AI algorithms threaten to replace the tasks they perform.
Not infrequently, medical professionals are the decisionmakers, and AI algorithms threaten to replace the tasks they perform.
For example, there is no shortage of warnings about radiologists losing their jobs.
In 2016, Geoff Hinton, who won computer science’s highest award, the Turing Award, for his work on neural networks, said that “We should stop training radiologists now; it is just completely obvious deep learning is going to do better than radiologists.” 21
This prediction was informed by the very promising advances of AI in image-based diagnosis. Yet there are still plenty of radiologists.
Why has Hinton’s prediction not yet come to pass?
The challenges include lack of trust in the algorithms, challenges in data collection, and regulatory barriers, as noted above.
They also include a misalignment of incentives.
The challenges include lack of trust in the algorithms, challenges in data collection, and regulatory barriers, as noted above.
They also include a misalignment of incentives.
In our study analyzing AI adoption through job postings, we find that adoption indeed varies by type of job and by hospital management structure.
AI skills are less likely to be listed in clinical roles than in administrative or research roles.
AI skills are less likely to be listed in clinical roles than in administrative or research roles.
Hospitals with an integrated salary model, which are more likely to be led by individuals who have focused their career on management and take a systematic approach to administration, have a higher rate of adoption of AI for administrative and clinical roles but not for research roles compared to hospitals more likely to be managed by doctors.
Teaching hospitals are no different from other hospitals in their adoption rate.
Hospitals with an integrated salary model, which are more likely to be led by individuals who have focused their career on management and take a systematic approach to administration, have a higher rate of adoption of AI for administrative and clinical roles but not for research roles
One interpretation of these patterns is that hospitals with an integrated salary model, and hence professional managers, have leaders that recognize the clinical and administrative benefits of AI, while other hospitals might have leaders that do not recognize the benefits.
However, we have seen that there are several reasons why AI adoption might be slow in hospitals.
In other words, even if professional managers are more likely to adopt AI, they are not necessarily right to engage in adoption at this stage.
For example, while it may be that doctor-led hospitals have not adopted AI because they view it as a threat to their jobs, it may also be that doctor-led hospitals have leaders who have a better grasp of the other adoption challenges-algorithmic limitations, data access limitations, and regulatory barriers.
However, we have seen that there are several reasons why AI adoption might be slow in hospitals.
In other words, even if professional managers are more likely to adopt AI, they are not necessarily right to engage in adoption at this stage.
… while it may be that doctor-led hospitals have not adopted AI because they view it as a threat to their jobs,
it may also be that doctor-led hospitals have leaders who have a better grasp of the other adoption challenges-algorithmic limitations, data access limitations, and regulatory barriers.
Overall, relative to the level of hype, AI adoption has been slow in health care.
Policymakers can help generate useful adoption with some innovative approaches to privacy and the path to regulatory approval.
However, it might be the familiar tools that are most useful: clarify the rules, fund research, and enable competition.
About the authors
Avi Goldfarb is a consultant with Goldfarb Analytics Corporation, which advises organizations on digital and AI strategy.
Florenta Teodoridis, Assistant Professor of Management and Organization — USC Marshall School of Business
The authors did not receive financial support from any firm or person for this article or from any firm or person with a financial or political interest in this article. Other than the aforementioned, the authors are not currently an officer, director, or board member of any organization with a financial or political interest in this article.
Originally published at https://www.brookings.edu on March 9, 2022.