This is an excerpt of the article below, focused on the topic above, preceded by an Executive Summary, by the author of the blog.
Getting Buy-In for Predictive Analytics in Health Care
Harvard Business Review
by Meetali Kakad, MD, Ronen Rozenblum, David Westfall Bates, MD
June 20, 2017
Executive Summary
by Joaquim Cardoso MSc.
Chief Editor of “The Health Strategy Foundation Blog”
@ The Analytics Informed Health Care Institute
March 15, 2022
What is the problem?
- According to the National Academy of Medicine (formerly the Institute of Medicine), the U.S. health care system spends almost a third of its resources — $750 billion annually — on unnecessary services and inefficient care.
What is the opportunity?
- New predictive analytics tools promise to reduce waste and improve care by forecasting the likelihood of an event — for example, a patient being readmitted to a hospital or developing a life-threatening infection — and allowing providers to tailor treatments and services accordingly.
- These tools are now being used across the continuum of care, from disease surveillance to chronic disease prevention to identifying patients who are at risk of deterioration.
What is the current adoption?
- Despite the tools’ power to improve care, most health care institutions are not yet using them.
What are the barriers?
- Among the impediments to adoption are the bewildering array of options providers face, (i) from mobile applications to (ii) web-based tools to programs that integrate with electronic health records.
- The most important factor is that “Success depends less on the tool itself than on getting buy-in at all levels from the start”, according to a survey with 34 key figures from leading U.S. health systems, policy makers, and predictive analytics vendors.
Among the most important findings of the survey, here are 3 lessons
- Engage the Right People from the Outset: Regardless of whether a provider is developing predictive analytics in-house, as many large academic medical centers have done, or purchasing tools off the shelf, managers should make sure they are involving the right people throughout the entire process.
- Change Agents and Clinical Champions Are Essential: Health care organizations that regularly used implementation experts to support change and improve quality across a range of IT and other types of projects had a head start when implementing predictive analytics.
- The C-Suite Must Commit: Just as important as frontline buy-in is engagement from the top, especially from the CEO. Organizational leaders are often unfamiliar with advanced analytics technology and applications.
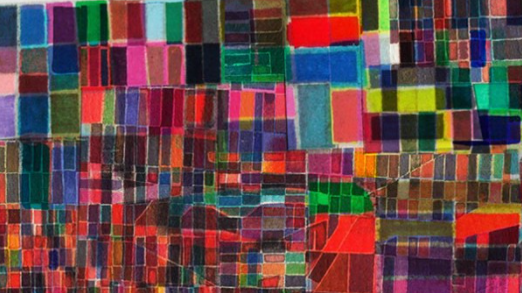
What do we mean by “Engage the C-Level” (“get the commitment and the resources”)?
- Just as important as frontline buy-in is engagement from the top, especially from the CEO. Organizational leaders are often unfamiliar with advanced analytics technology and applications.
- Educating leadership about a tool’s expected benefits is critical in generating support. One large U.S. academic medical center did this by including tool performance measures in the executive dashboard, making its benefits clear to top management.
Ongoing attention from senior management is vital for the long-term sustainability of predictive tools; the models decalibrate over time and require regular maintenance.
- Successful organizations take a lifecycle approach to managing and maintaining these tools, which requires budgeting for long-term resource requirements, including investments in improving data quality and infrastructure, recalibration, and in-house data science and technology capability.
As we’ve shown, the key to successful implementation has little to do with the model itself.
- Success depends on the time, effort, and resources set aside for communication, change management, and making the tool a seamless part of user workflow.
- Clear, committed leadership and a culture strongly supportive of change and learning are also critical factors.
ORIGINAL PUBLICATION (full version)
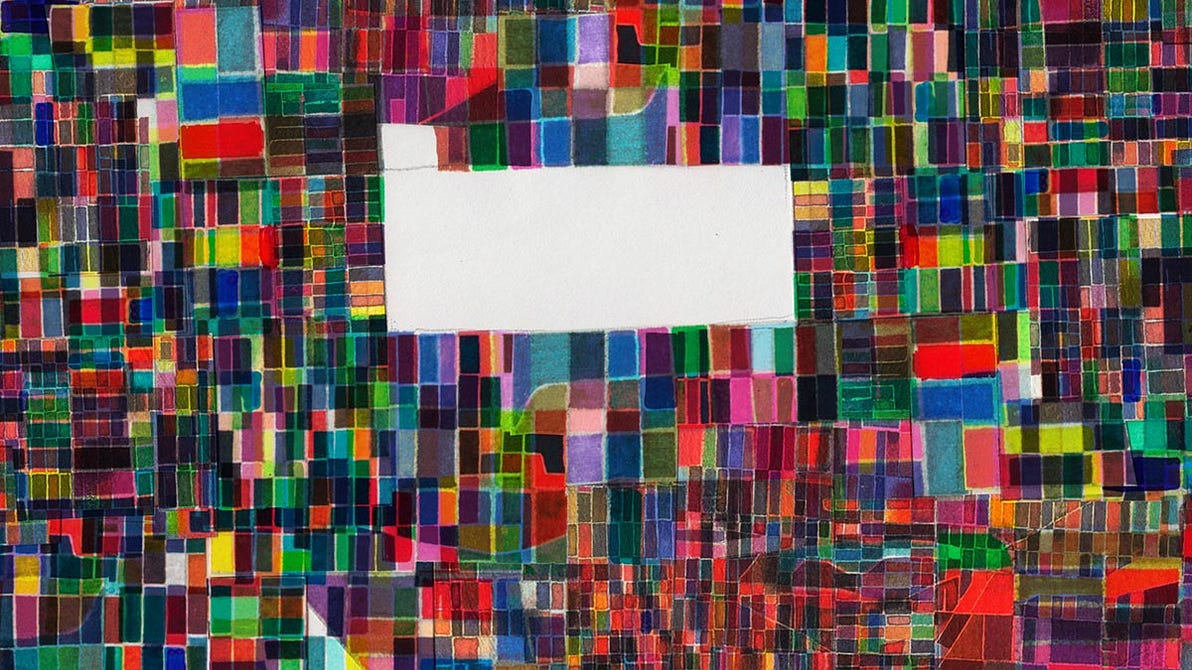
This is an excerpt of the article below, focused on the topic # 3(Engage the C-Level).
Getting Buy-In for Predictive Analytics in Health Care
Harvard Business Review
by Meetali Kakad, MD, Ronen Rozenblum, David Westfall Bates, MD
June 20, 2017
Table of Contents (TOC)
- Introduction
- Engage the Right People from the Outset
- Change Agents and Clinical Champions Are Essential
- The C-Suite Must Commit
According to the National Academy of Medicine (formerly the Institute of Medicine), the U.S. health care system spends almost a third of its resources — $750 billion annually — on unnecessary services and inefficient care.
New predictive analytics tools promise to reduce waste and improve care by forecasting the likelihood of an event — for example, a patient being readmitted to a hospital or developing a life-threatening infection — and allowing providers to tailor treatments and services accordingly.
These tools are now being used across the continuum of care, from disease surveillance to chronic disease prevention to identifying patients who are at risk of deterioration.
According to the National Academy of Medicine (formerly the Institute of Medicine), the U.S. health care system spends almost a third of its resources — $750 billion annually — on unnecessary services and inefficient care.
New predictive analytics tools promise to reduce waste and improve care by forecasting the likelihood of an event — for example, a patient being readmitted to a hospital or developing a life-threatening infection — and allowing providers to tailor treatments and services accordingly.
These tools are now being used across the continuum of care, from disease surveillance to chronic disease prevention to identifying patients who are at risk of deterioration.
But despite the tools’ power to improve care, most health care institutions are not yet using them.
Among the impediments to adoption are the bewildering array of options providers face,
- from mobile applications
- to web-based tools to programs that integrate with electronic health records.
To better understand what stands in the way of adoption, and what facilitates successful implementation, we interviewed 34 key figures from leading U.S. health systems, policy makers, and predictive analytics vendors.
Among our most important findings: Success depends less on the tool itself than on getting buy-in at all levels from the start.
But despite the tools’ power to improve care, most health care institutions are not yet using them.
Among the impediments to adoption are the bewildering array of options providers face, from mobile applications to web-based tools to programs that integrate with electronic health records.
Among our most important findings: Success depends less on the tool itself than on getting buy-in at all levels from the start.
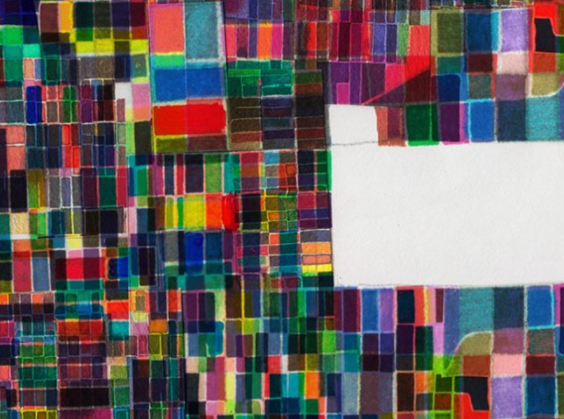
HERE ARE THREE LESSONS:
Engage the Right People from the Outset
See the original publication
Change Agents and Clinical Champions Are Essential
See the original publication
The C-Suite Must Commit
Just as important as frontline buy-in is engagement from the top, especially from the CEO. Organizational leaders are often unfamiliar with advanced analytics technology and applications.
Educating leadership about a tool’s expected benefits is critical in generating support.
One large U.S. academic medical center did this by including tool performance measures in the executive dashboard, making its benefits clear to top management.
A tool’s value may be quantified in terms of quality improvement, improved patient or clinician satisfaction, or efficiency gains.
One measure that is likely to resonate for management is reduced readmissions among Medicare patients, as hospitals may be financially penalized for readmissions.
Models aimed at reducing readmissions among high-risk patients are understandably popular; one model, for example, was shown to reduce the likelihood of readmission for heart failure patients by 26%.
Ongoing attention from senior management is vital for the long-term sustainability of predictive tools; the models decalibrate over time and require regular maintenance.
Successful organizations take a lifecycle approach to managing and maintaining these tools, which requires budgeting for long-term resource requirements, including investments in improving data quality and infrastructure, recalibration, and in-house data science and technology capability.
Where commercial tools are purchased, costs such as software licenses, consulting, or other vendor-related fees also need to be factored into long-term budgets.
Implementing predictive analytic tools in health care is a means to an end — where the end should represent an improvement in health or health care outcomes, including lower costs.
Fully realizing the benefits from a specific tool requires a structured and thoughtful approach, involving the right people, with the right skills sets, at the right time.
As we’ve shown, the key to successful implementation has little to do with the model itself. Success depends on the time, effort, and resources set aside for communication, change management, and making the tool a seamless part of user workflow.
Clear, committed leadership and a culture strongly supportive of change and learning are also critical factors.
Done well, the result can be an increase in high-value care — that is, targeting appropriate health care to those who need it.
Done well, the result can be an increase in high-value care — that is, targeting appropriate health care to those who need it.
Originally published at https://hbr.org on June 20, 2017