Frontiers in Digital Health
Stephane Doyen and Nicholas B. Dadario
03 May 2022, yale
Abstract
The healthcare field has long been promised a number of exciting and powerful applications of Artificial Intelligence (AI) to improve the quality and delivery of health care services.
AI techniques, such as machine learning (ML), have proven the ability to model enormous amounts of complex data and biological phenomena in ways only imaginable with human abilities alone.
As such, medical professionals, data scientists, and Big Tech companies alike have all invested substantial time, effort, and funding into these technologies with hopes that AI systems will provide rigorous and systematic interpretations of large amounts of data that can be leveraged to augment clinical judgments in real time.
The healthcare field has long been promised a number of exciting and powerful applications of Artificial Intelligence (AI) to improve the quality and delivery of health care services.
However, despite not being newly introduced, AI-based medical devices have more than often been limited in their true clinical impact that was originally promised or that which is likely capable, such as during the current COVID-19 pandemic.
… despite not being newly introduced, AI-based medical devices have more than often been limited in their true clinical impact that was originally promised or that which is likely capable, such as during the current COVID-19 pandemic.
There are several common pitfalls for these technologies that if not prospectively managed or adjusted in real-time, will continue to hinder their performance in high stakes environments outside of the lab in which they were created.
To address these concerns, we outline and discuss many of the problems that future developers will likely face that contribute to these failures.
There are several common pitfalls for these technologies that if not prospectively managed or adjusted in real-time, will continue to hinder their performance in high stakes environments outside of the lab in which they were created.
Specifically, we examine the field under four lenses: approach, data, method and operation.
If we continue to prospectively address and manage these concerns with reliable solutions and appropriate system processes in place, then we as a field may further optimize the clinical applicability and adoption of medical based AI technology moving forward.
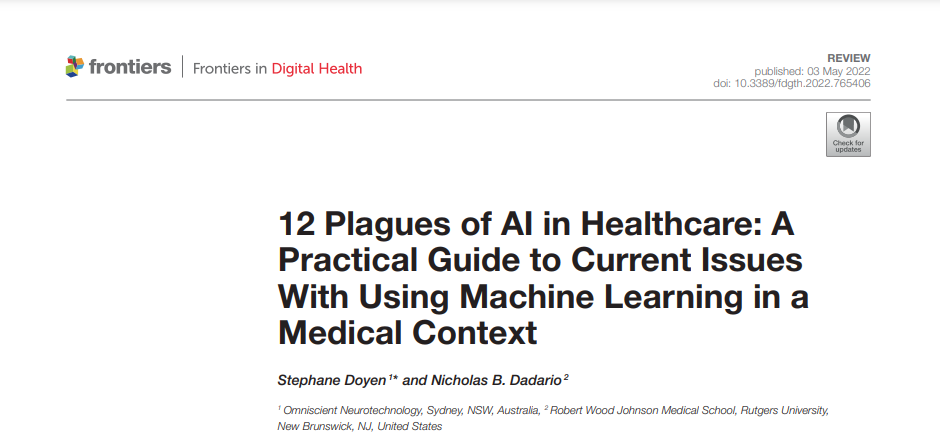
Introduction
The powerful applications of artificial intelligence (AI) have long been promised to revolutionize the healthcare field.
AI has been met with a surge of interest in the scientific and medical communities due to the increasing number of patients receiving healthcare services and the concomitant increases in complexity of data, which is now available, but often uninterpretable by humans alone.
These technologies demonstrate the ability to
- identify malignant tumor cells on imaging during brain surgery ( 1),
- unravel novel diseases into explainable mechanisms of viral mutations for therapeutic design ( 2),
- predict the progression of neurodegenerative diseases to begin earlier treatments ( 3), and
- assist with the interpretation of vast amounts of genomic data to identify novel sequence patterns ( 4), among a number of many other medical applications.
Ultimately, the applications of AI in medicine can generally be grouped into two bold promises for healthcare providers:
(1) the ability to present larger amounts of interpretable information to augment clinical judgements while also
(2) providing a more systematic view over data that decreases our biases.
In fact, one could argue that improving our ability to make the correct diagnoses if given an opportunity can be seen as a key duty and moral of the medical field in principle ( 5).
Given this enormous potential, it is unsurprising that Big Tech companies have matched the enthusiasm of scientific experts for AI-based medical devices by investing substantial efforts and funding in their product development over recent years ( 6).
Unfortunately, despite these exhilarating observations and promises, we have yet to truly harness the full potential of AI as a tool in current practices of medicine.
Many loosely utilize the label “AI” for the medical applications described above, but providing more focused definitions for a few important terms may be beneficial moving forward in this work.
The term “AI” in fact more broadly refers to the idea of machines being able to intelligently execute tasks in a manner similar to human thinking and behavior. To date, such applications are not currently found in the field.
Instead, applications where machines learn from data can more accurately be labeled as machine learning (ML) based solutions.
For simplicity, we will use these two terms interchangeably throughout the current manuscript, and outline commonly used terms in Figure 1 which are considered under the umbrella of “AI.”
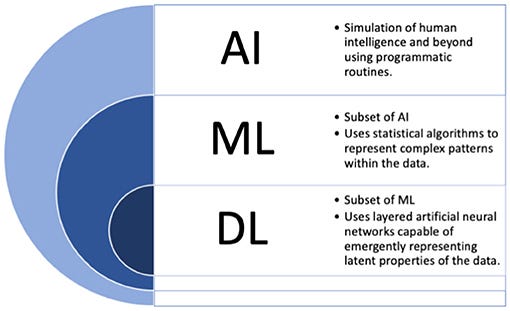
While not newly described, ML based applications have re-surfaced interest in the medical community with the rise of the recent SARS-CoV-2 pandemic ( 2).
International collaborative efforts from Data Scientists have attempted to take advantage of differences in disease prevalence across the world as a way of utilizing early access to data to improve the quick diagnosis and prognostication of patient outcomes in soon-to-be overwhelmed hospitals.
In fact, novel approaches had immediately begun to tackle concerns on vaccine developments early in the pandemic according to possible viral mutations that allow escape from the human immune system.
By framing SARS-CoV-2 protein sequence data in the context of linguistic rules used in the human natural language space, ML algorithms may be able to present to us interpretable mechanisms of how a virus mutates while retaining its infectivity, similar to how a word change in a human language may dramatically alter the meaning of a sentence without changing its grammar ( 2).
Indeed, thousands of exciting models with increasing collaboration and data sharing had been immediately proposed throughout the pandemic to improve the prognostication and clinical management of COVID-19 patients ( 7, 8).
While few clinical applications were found to demonstrate a true clinical impact on patient outcomes for the current pandemic, it is important to note that ML applications have elsewhere demonstrated important clinical applications in similar contexts ( 9), such as
- for improving the allocation of limited resources ( 10),
- understanding the probability of disease outbreak ( 11),
- and the prediction of hospital stay and in-hospital mortality ( 12).
Therefore, while these models continue to demonstrate enormous potential to manage large scale clinical scenarios, further work is still necessary to ensure they can be quickly and effectively leveraged for clinical translation, a concern which has been encountered in the field previously.
The limitations mentioned above are not to say that we should abandon the field of AI-based medical technologies as a whole.
The ability for human intuition alone to map the brain for instance, would take insurmountable amounts of time and effort compared to that which is now possible with AI-based technology ( 18– 20).
As original goals previously hoped that these technologies would resemble human based intelligence, it is important to remember To Err is Human.
Instead, as with all emerging fields, further work is necessary to optimize the clinical applicability of these tools as we move forward to minimize unnecessary errors and harm.
… as with all emerging fields, further work is necessary to optimize the clinical applicability of these tools as we move forward to minimize unnecessary errors and harm.
Unfortunately, there is limited information available in the literature that presents a clear guide of the common problems that will be encountered with AI-based software, and more specifically how to overcome them moving forward in medical practices.
Such a gap possibly reflects the separation of expertise and focus between medical professionals and data scientists all together.
… there is limited information available in the literature that presents a clear guide of the common problems that will be encountered with AI-based software, and more specifically how to overcome them moving forward in medical practices.
Such a gap possibly reflects the separation of expertise and focus between medical professionals and data scientists all together.
To address this gap, we provide a clear guide below on the most common pitfalls to medical AI solutions based on the previous literature.
Specifically, we examine the field under four lenses: approach, data, method and operation ( Figure 2).
Within these core components, we outline a number of different issues that must be considered in the development and application of machine learning technologies for medical based applications, and we elucidate how to identify, prevent, and/or solve them moving forward to ultimately create safer, more trustworthy, and stronger performing models.
Specifically, we examine the field under four lenses: approach, data, method and operation
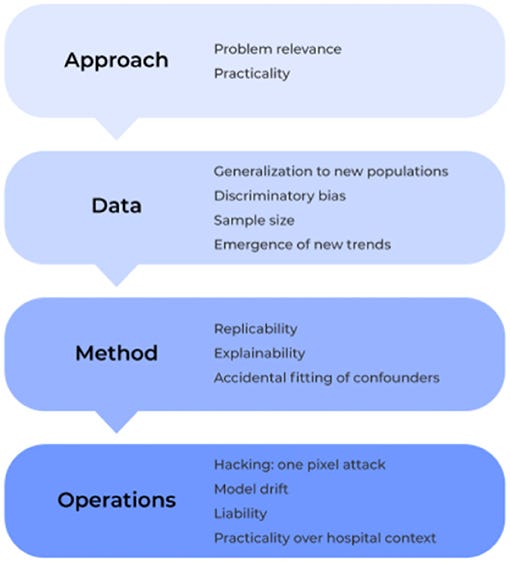
APPROACH: What Is the Plan?
Problem 1. Relevance: Cutting Cake With a Laser
Due to a rise in the amount of open-source and advanced statistical software, it has become increasingly easy to develop highly elegant and powered computational models. However, when created with only technical solutions in mind, models can easily be created to solve an non-existent or irrelevant problem. In turn, the ultimate users of the technology, such as the practicing physician in the clinic, will have no interest in the solution being answered by a specific model. Problems with a model’s relevance can render even the most elegant application of data science irrelevant ( Figure 3).
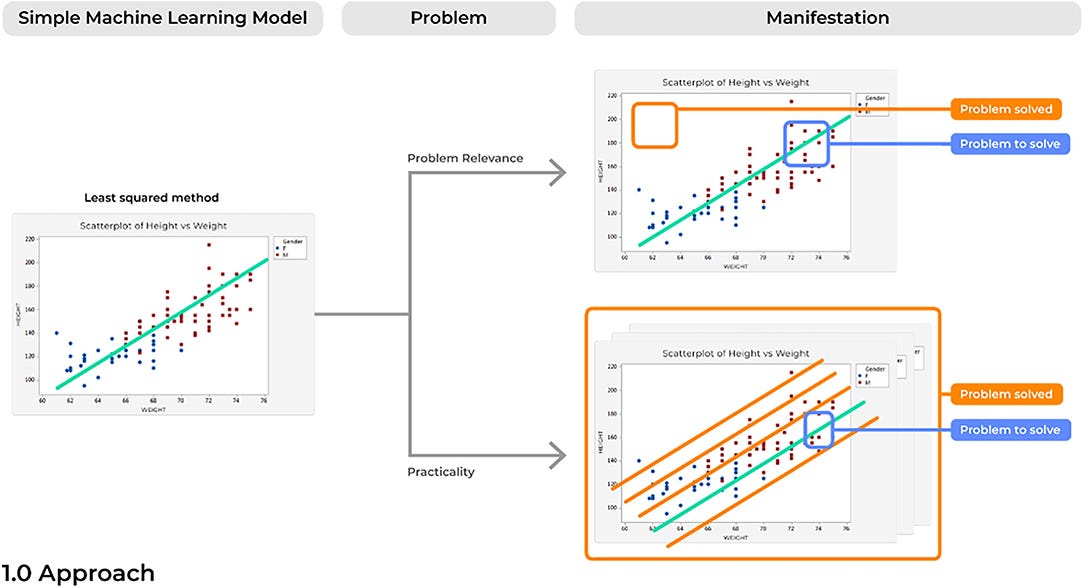
Instead, the ultimate users of the technology should be included at the very beginning of the development of the model. Research and Development goals should be grounded in what has already been suggested in the medical field as important avenues of future work for clinical improvements. For instance, rather than attempting to predict an illness which can already be clearly identified in clinical practice, ML tools can reduce the complexity of patient information presented in a specific pathological states ( 24, 25) and then present statistical irregularities that can be used by physicians to make more informed decisions for clinical treatment ( 26, 27). Ultimately, it must be remembered that AI is a powerful tool that can be leveraged to answer a difficult questions, the usefulness of the tool is a function of the appropriateness of the question being asked.
Problem 2. Practicality: Not Everyone Has a Cyclotron
Similar to the concerns of relevance mentioned above, advances in ML abilities have also created concerns of practicality. This refers to building a model which has limited practical applications in the environment of interest due to logistical constraints that were not considered outside of the environment that the model was originally created in, such as requiring more computation than necessary or that which can be feasibly run in a clinic. Reasonable solutions must commonly consider the current state of the field and the technical constraints of the model proposed.
To create and train advanced ML models, disparate data is often harmonized from varying sources and formats to create a single large dataset of valuable information. Data harmonization is particularly important in medicine given that small amounts of data on specific topics are often managed by acquiring data from varying records and from a variety of centers, all of which also commonly utilize different electronic health record (EHR) systems ( 28). Therefore, to build a model that aims to compare specific patients to a template/control group, algorithms must first harmonize large amounts of a patient’s data from varying datasets to perform a comprehensive comparison. However, to do this, many algorithms require datasets to be harmonized as large cohorts. Unfortunately, outside of the lab, these methods can become impractical when single ( N = 1) patients present to the clinic. Therefore, these algorithms can fail unless additional practical solutions are present. Importantly, improved super computers may hold the computing power to execute a highly complex model in a lab for a single patient, but a physician may not be able to analyze a patient’s data with these algorithms on a less powerful hospital-issued laptop in a time critical environment where it must perform at the highest level. On that same note, it is easy to optimistically consider the intricate problems that can be tackled with ML in the future due to the improved abilities of modern computational algorithms to digest highly complex data; however, medical data is often not available or too limited for specific topics at the current time. When just utilizing the limited available data obtained specifically from academic studies to train a model, illusory results may be obtained given these data are cleaner than that which would be obtained from the actual target field. Therefore, investing time in an approach that is too far into the future may inherently cause difficulties in model preparation due to the lack of available feedback from the clinical field of interest.
To prospectively mitigate problems of practicality, implementation should always be considered from the very start of any analytical solution. This will prevent any waste of Research and Development efforts given that alternative solutions were already considered. A notable increase in recent efforts has been put forth by cloud providers and hardware manufactures to provide development frameworks which bridge the gap between an approach to a problem and the hardware to support it ( 29). Ultimately, including the intended users in the initial development stages will also provide insight on practicality during model development as the goal environment will have already been considered.
Data: What Are We Using and for What Purpose?
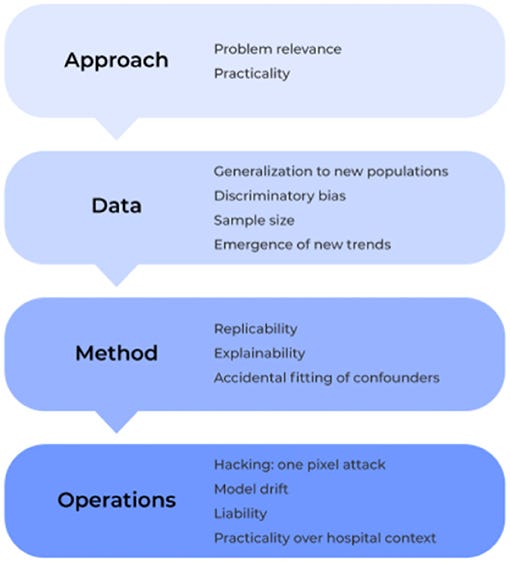
Problem 3. Sample Size: Looking at the World Through a Keyhole
A concern inherent to most analytical solutions includes issues of sample size. When creating and training a model using a limited sample size, inflated results may be demonstrated when actually testing it against a control sample. Subsequently, when introduced into a different, clinical environment, the model’s accuracy can lessen, and it can fail. This is because the small sample size may cause a model to present results that are merely due to chance. Consideration of this is paramount to acquire reliable results ( Figure 4).
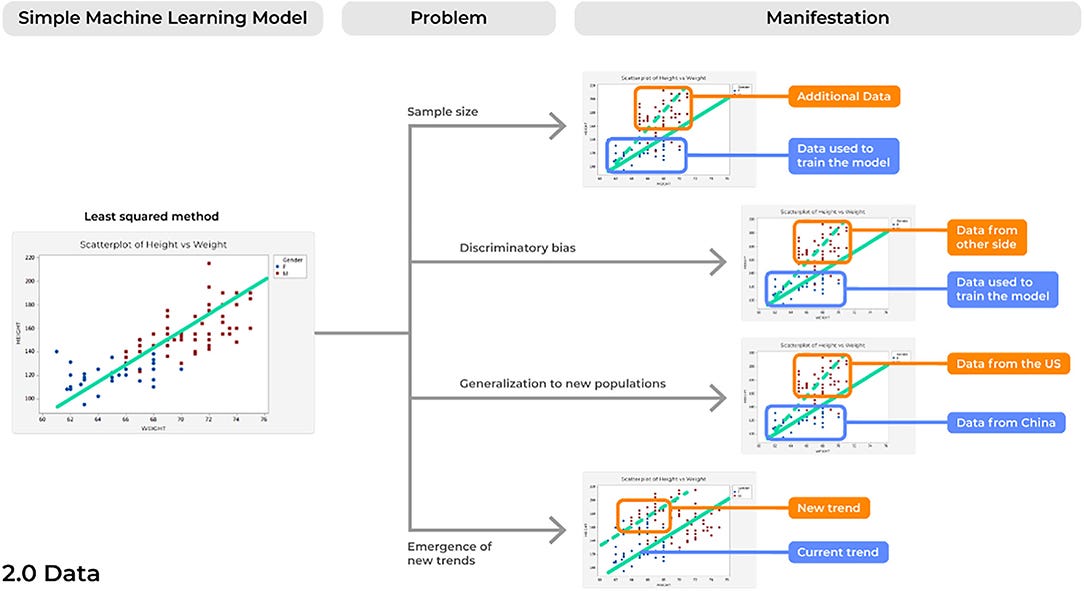
In an age of increasingly available data, ML algorithms must be trained on adequately sized and diverse datasets. While this will likely require the harmonization of data from multiple centers, this point is imperative if we are to believe our models are robust enough to consistently provide reliable results at different time points and in a number of different environments. A recent systematic review assessed 62 comprehensive studies detailing new ML models for the diagnosis or prognosis of COVID-19 utilizing chest x-rays and/or computed tomography images and found a common problem among studies was in the limited sample size utilized to train models ( 8), with other reviews noting similar observations ( 30). Amongst the 62 models assessed, more than 50% of diagnostic focused studies (19/32) utilized <2,000 data points. Unlike rare brain tumors which may require years of small amounts of data collection, there have been more than 2 million cases of COVID-19 to date ( 31). Therefore, it is unsurprising that developing a model to understand a complex biological phenomenon on only 2,000 data points can lead to unreliable results in the intended environment ( 8).
As in all aspects of medical research, newer ML models must be trained on a large and diverse dataset to provide reliable results. A clear solution is to implement an active and ongoing effort to acquire more data from a variety of sources. ML models, unlike physical medical devices, can be continually updated and improved to provide more reliable and accurate performances. As more data becomes available, models can be retrained with larger sample sizes and then updated for the best performance in the field. Ultimately, this may require a model to be pulled from a clinical practice, retrained and tested based on more data which is now available, and then placed back into the environment of interest. However, with the increased demand for more data, one must consider the possibility that an ML modeler may acquire and include data for which they have no patient consent. Once the new data is incorporated into the model and the model is input into the field, it is very difficult to identify which data was utilized. A possible solution to these patient consent concerns with increasing data is implementing in each image a certificate stating patient consent, such as through non-fungible tokens (NFTs) ( 32).
Problem 4. Discriminatory Biases: Rubbish in, Rubbish Out
Perhaps the most commonly discussed problem concerning AI technologies is their potential to exacerbate our current societal biases in clinical practice. Even more alarmingly, developing models with historical data may perpetuate our historical biases on a variety of different patients in ways we have since improved from.
Improved patient related factors must be considered when building datasets for the training of a model. By building models on data from a variety of different sites with increased awareness of the specific populations included, we may begin to mitigate the potential biases in our results. Ultimately, improvements in DL algorithms remain relatively nascent, and there has been an increased focus on classification performance across gender and race, providing us with impetus to ensure that DL algorithms can be successfully used to mitigate health care disparities based on demographics ( 35– 37).
Problem 5. Generalization to New Populations: From the Few, but Not the Many
Expanding on concerns of discriminatory biases, problems with generalization may occur due to the expansion of global software markets. Aside from differences in gender and skin color alone, a model may fail based on datasets trained on individuals from a single population due to underestimating the differences in population driven variability.
To prevent and manage issues of generalization, a number of solutions exists. First, similar to what was described above, data must be accumulated from a variety of sources. However, to provide the most generalizable results, these sources must span several different sites inside and outside of the country of origin where the model was developed. Surely, inter-individual factors must be considered during production to improve the robust ability of a model in different environments. Nonetheless, it is also likely that site-specific training should also be considered as an optimal avenue to tailor models based on the specific populations where a model is going to be implemented. Then, external validation testing in separate adequately sized datasets ( 43) can ensure that an algorithm can model data from different sites similarly to that which it trained on.
Problem 6. Emergence of New Trends: Surfacing Creatures From the Depth
This problem is likely the most relevant to the current conditions of the world with the recent SARS-CoV-2 pandemic. As such, problems related to the emergence of new trends refers to when a new trend emerges in the data that the initial model was not built to account for, thus altering the new statistical comparisons being made between variables.
Models in production should be created with a set of test reflective environmental data to ensure expected performances in situ. Furthermore, alongside changes in current clinical practices, models must be continually monitored and tested with new data to assess for reliability and validity. This continual external validation testing with separate adequately sized datasets than which it was trained on provides a necessary avenue for improvement as the field of healthcare and the environment itself is continually changing.
Method: How Does the Tech Approach Play Out?
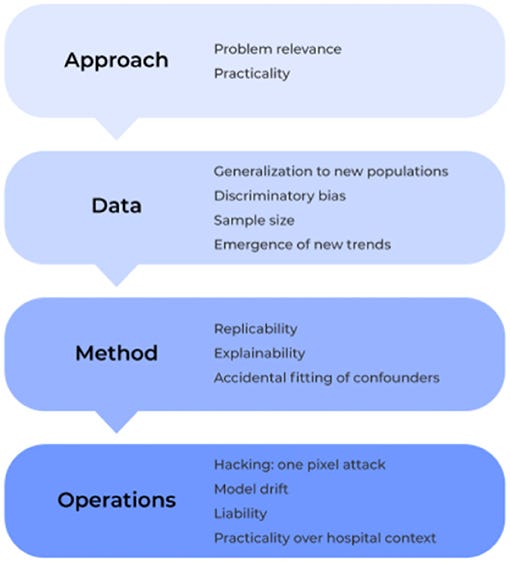
Problem 7. Reproducibility: Bad Copies
Concerns of replicability are not a newly discussed phenomena for a number of different fields that require the processing of large amounts of data ( 48). However, failure of a model to demonstrate the same results time and time again presents a profound risk of inconsistencies in the delivery and quality patient care, and therefore should be considered in future ML developments ( Figure 5).
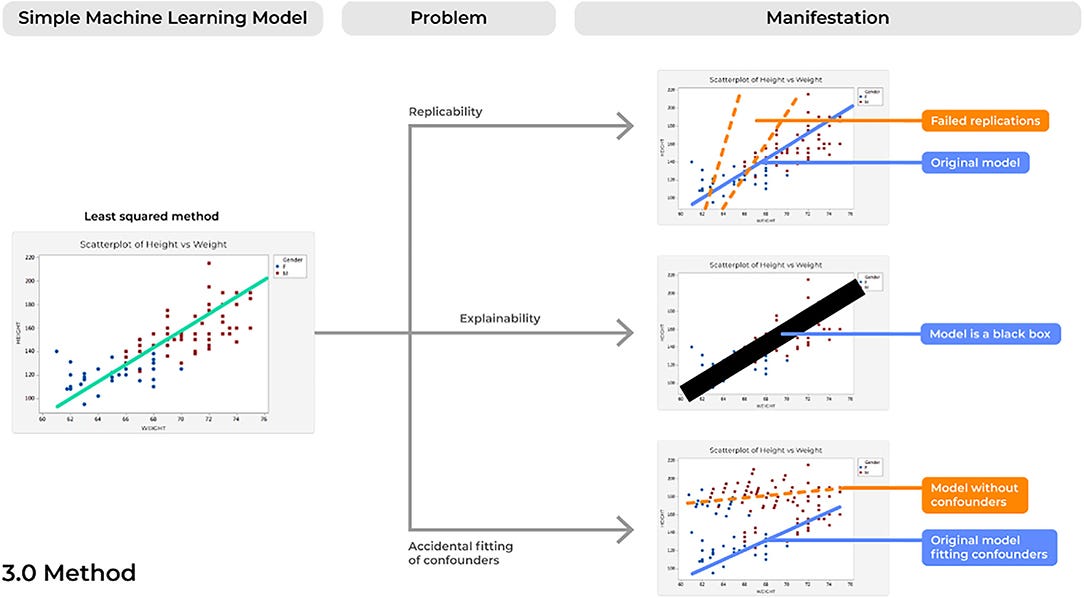
In addition to the obvious potential improvements in patient safety following more rigorous evaluations of clearly reported methodology, improved reporting of ML algorithms can also provide an important way to advance the field as a whole. A number of different Data Scientists may spend countless hours in designing complex ML models to address an imminent question, such as what we saw for COVID-19, yet increasing errors will commonly be identified from just the smallest differences across algorithms ( 8). Instead, if models and datasets are clearly reported following a study, then others can appropriately assess these models and collectively improve upon them to produce more robust pipelines. This will ultimately improve our ability to bring these tools to clinical practice as a model becomes more accurate without repeating the same mistakes. The increased requirements for adherence to rigorous, ML reporting guidelines across many major peer-reviewed journals is a promising improvement moving forward.
Problem 8. Explainability: The Black Box Problem
One of the largest concerns of AI-based devices in medicine concerns physicians’ lack of trust for model performance ( 52). Unfortunately, as ML models have increased in complexity, this improvement has often been met with a trade-off in explainability, in which there is increasing uncertainty regarding the way these models actually operate ( 53).
This problem is often described as a model operating in a “black box,” in which irrespective of model performance, very little can be elucidated about why a model made a specific decision. A common example of this can be seen with a highly powered ML technique known as deep learning (DL). DL applications can maintain hundreds of stacked representations across hundreds of layers, a relationship that no human can truly accurately comprehend in full detail. However, a number of important improvements can be made in the field as we improve concerns of lack of explainability, to which a whole field has been dedicated known as Explainable Artificial Intelligence (XAI) ( 53). Ultimately, ML tools are capable of taking highly dimensional data and quickly making accurate decisions in highly time-critical medical scenarios, a feat that humans may never physically nor cognitively be capable of performing ( 54). However, if we could explain the various decisions being executed by a certain model and the specific features being analyzed to produce a certain outcome ( 24), physicians can better interpret these results based on logic and previous knowledge. Then, healthcare providers may not only be able to better trust these algorithms, but providers may also continually improve the model’s performance when the system presents an error that is likely based on a specific wrong answer possibly being executed in a portion of a decision tree. In fact, since these models are highly capable of detecting novel patterns in large amounts of data which are invisible to the human eye ( 4), interpretable and explainable models may also unlock new insights in the scientific world that spur further improved ML developments in the future, creating a positive reinforcing cycle of innovation.
Outside of the trust of a practicing healthcare provider, the patient themself, if diagnosed by a ML tool to have a malignant skin lesion, may too require an interpretable and justifiable reason why specific results were provided, such as why the tumor was diagnosed as malignant. Thus, it is important that a clinician is able to interpret the decisions made by a specific algorithm, but this also raises concerns of violating patient-physician relationships and liability for AI technology in general ( 55). A core component of the Hippocratic Oath requires physicians to do no harm and act upon their greatest judgement for improved patient care. With the incorporation of machine-learning guided clinical care, failure to understand a model’s decision making can shift fiduciary duties away from the physician and hurt the patient-physician alliance ( 56). Furthermore, if a model provides a piece of information that leads to a poor outcome for a patient, is it the machine’s fault or is it the healthcare provider’s medical error? Unsurprisingly, promotion of interpretability of a model is outlined as a main principle within the recent World Health Organization (WHO) guidelines on Ethics & Governance of Artificial Intelligence for Health ( 57). Both the model and provider must be able to clearly elucidate these findings to the patient if we are to truly incorporate ML into standard medical practices.
Movement toward white-box, also called glass-box, models provides a solution to address concerns of explainability. These models can often be seen with linear ( 58) and decision-tree based models ( 24), although a number of other applications are increasingly being developed ( 53). In fact, DL based networks make up the majority of the highly sought after radiological-AI applications for the medical field ( 1), such as the systems that can diagnose brain cancer during surgery. Such networks provide the enthusiasm for the recent large scale efforts in the field to improve the explainability of advanced ML techniques ( 59). Specifically, by utilizing white box models as first line modeling techniques, one can ensure findings are being appropriately made based on ground truths provided by current scientific knowledge. For example, a number of recently developed practical approaches have been introduced using input vector permutation to better understand how specific inputs impact the predictions of a model and may be particularly useful to gain insight into how models make specific decisions ( 60, 61). Explainable AI approaches, such as deconvolution methodology, can be applied to more complicated models, such as convolutional neural networks (CNNs) and ensembles, to improve the interpretability of the more complex models ( 62). However, further research is needed in the field of explainable AI to better understand model-specific techniques that can be leveraged to ultimately improve the transparency of these models in the healthcare setting.
Problem 9. Accidental Fitting of Confounders: Guilt by Association
ML tools are able to digest highly complex datasets by continually assessing and scanning different features until optimal performance is achieved. As such, concerns of accidentally fitting confounders can easily surface and a model that was thought to be capable of predicting an outcome is instead making a prediction based on factors unrelated to that outcome of interest. If so, these models can produce not only unreliable results in clinical practice, but can also present profound risks of patient harm, such as by under- or over-estimating specific diagnoses.
First, an ML specialist must have a strong understanding of the data being modeled. Then, when actually developing the model, one should carefully explore and rule out any concerns for confounders. In addition to previous descriptions of “white-box” models, improved understanding of the features being mapped may allow further appropriate critical evaluations of model performances and in turn lead to increased trust in the medical community.
Operation: In the Field
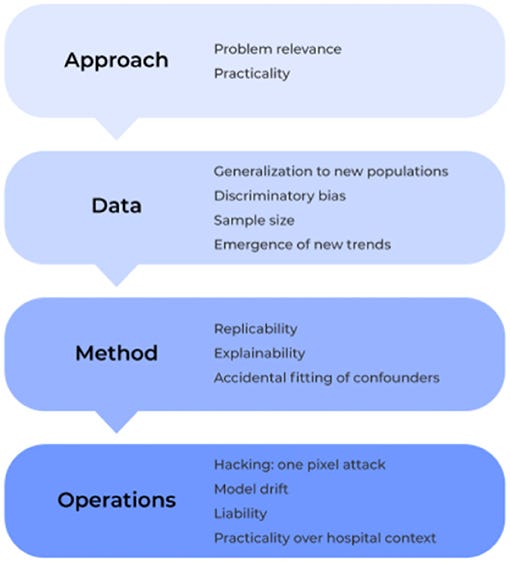
Problem 10. Model Drift: Like a Rolling Stone
For many of the reasons discussed above, over time a model will likely begin to make an accumulating number of errors. This could be due to issues with model drift, in which a model that was deployed into production many years ago would begin to show performance decay over time ( Figure 6). Different than problems with the emergence of a new trend, model drift represents a multifactorial issue that likely reflects the relationship between two variables changing with time, ultimately causing a model to become increasingly unstable with predictions that are less reliable over time.
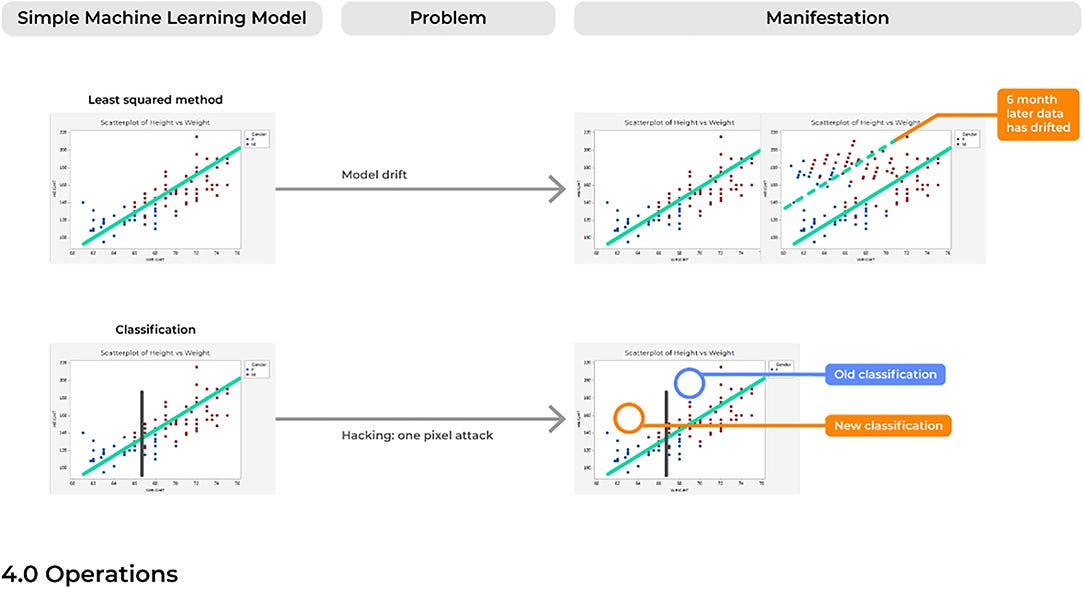
Generally, the training of ML models follows an assumption of a stationary environment; however, two types of model drift based on non-stationary environments have been described, including: (1) virtual concept drifts and (2) real concept drifts ( 68). Virtual drifts refer to when the statistical characteristics or marginal distributions of the actual data changes according to a change in time without the target task itself also adjusting similarly (e.g., the regression parameters). Real drifts refer to situations when the relationships between two or more variables in a model are based on a function of time, such that parameters in which the model was trained now becomes obsolete at different points in time (e.g., Pre-Covid vs. Post-Covid) ( 69). Without considering the possibility of a model drifting, a model can begin to predict outcomes in an unexpected way, which in a healthcare setting could immediately represent incorrect diagnoses being made.
To account for model drift, both active and passive methods have been proposed, of which the later represents the easiest solution to implement ( 68). Active methods refer to the methodology for detecting this drift and then self-adjusting its parameters to retrain the system to account for this shift, such as by forgetting old information and then updating based on new data ( 70). However, this methodology is more practical when data is available as a continual stream that will allow a model to continually adapt to recent data inputs. Differently, passive learning methods are reliable in that the performance of a model will be continually or periodically monitored by developers, such as through each release cycle, thus ensuring consistent and reliable results according to the model’s original results. As more data becomes available, passive methods could allow users to adapt the model and retrain it based on new data and updated scientific knowledge. Thus, this method could allow for more transparency over time concerning the model’s performance, avoiding scenarios where a model may make decisions on new relationships that are non-interpretable or even scientifically unsound.
Problem 11. Practicality Over Hospital Context: Will the IT Department Say Yes?
Systems should be developed according to the environment in which they will be deployed. While this may seem intuitive, there are a number of strict requirements that technology must follow in a healthcare setting that may not be accounted for, especially with cloud-based computing software. Thus, a system should be developed based on how hospitals are organized and specifically how healthcare providers will plan to use these models.
To consider impending concerns of meeting the rigorous standards and requirements contained in the hospital context, developers should meet with the end users and product stakeholders at the beginning of production. In turn, this will allow a clear delineation of the current restraints of an environment that will allow developers to prospectively include user requirements in solution designs.
Problem 12. Hacking: One Voxel Attack
Despite the novelty of advanced ML systems that are highly capable of managing complex data relationships, it must be remembered that ML systems are inherently IT systems which can be similarly fooled and hacked by outsider users.
A number of methods have been proposed to prevent the damage from these adversarial attacks. Re-training the model with robust optimization methodology can increase the resistance of a model to these attacks. Increase detection methods to identify attacks may also be appropriate ( 76). Other methods have also been similarly described, but it remains uncertain the degree to which these methods are better than others for a given scenario. Nonetheless, what is certain is that the integrity and robustness of an AI system must be rigorously examined against known attacks to achieve further safety and trust with applications in the medical field.
Future Directions: a Standard Labeling System for Medical AI
Addressing each of the concerns above provides a way to rigorously create a robust model that performs safely and accurately in a field full of potential concerns.
However, one way to further advance the field and improve the widespread adoption of these robust technologies is through a standard labeling system that can accurately detect and then convey anomalies in an ML-based system’s performance and quality ( 77).
Common to the most successful business plans are the use of key performance indicators (KPIs) as a way to document success and efficiency.
KPIs demonstrate the achievement of measurable landmarks toward reaching company and consumer goals.
For an ML model, standard labeling could display KPIs possibly related to the (1) sample it trained and tested on, (2) its quantitative accuracy including information on false positive and negatives, and (3) its risk of specific biases ( Figure 7).
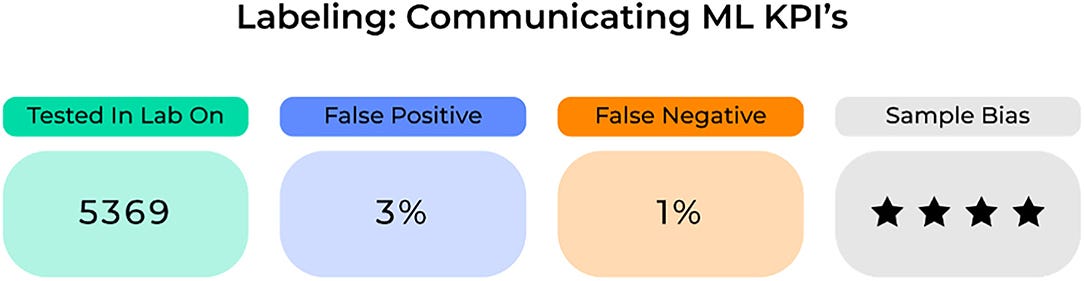
Importantly, these KPIs need to be clearly defined and continuously updated in order for healthcare providers to appropriately examine and incorporate specific ML-based systems into standard clinical practices.
Ultimately clinicians will need to assess a model’s success to understand where and when to apply it in a given scenario.
To make this decision, it will require the use of accurate performance metrics for each model on the target population.
An important concern where standard medical labeling may be of use is in determining medical liability.
While it is justified to create incentives for risk control based on the environment of application, the degree of responsibility is less clear cut, and who is ultimately responsible: the machine or the healthcare provider?
While the ethics of AI are outside the scope of this paper, further objective information on a model’s performance for a given population with a standard labeling system, possibly contained in the legal section of an ML system, will ultimately improve our objective insight into their performance abilities.
In turn, this can give healthcare providers more complete information on whether or not to incorporate these advanced systems in specific scenarios or not.
Conclusion
Advancements in the field of artificial intelligence have promised a number of exciting and promising applications for the medical field to improve the quality and delivery of health care services.
While there have been remarkable advances in previous years, these applications have yet to fully demonstrate their true potential in clinical applications due to failures in demonstrating reproducible and reliable results as well as the general mistrust of these technologies in the medical community.
We outline many of the problems that future developers will likely face that contribute to these failures, specifically related to the approach, data, methodology, and operations of machine learning based system developments.
If we continue to prospectively address and manage these concerns with reliable solutions and appropriate system processes in place, then we as a field may further optimize the clinical applicability and adoption of medical based AI technology.
References and additional information
See the original publication
About the authors
Stephane Doyen1 * and
Nicholas B. Dadario2
1 Omniscient Neurotechnology, Sydney, NSW, Australia,
2 Robert Wood Johnson Medical School, Rutgers University, New Brunswick, NJ, United States
Originally published at https://www.frontiersin.org.