Site editor:
Joaquim Cardoso MSc
Health Transformation — institute for research, strategy and advisory
October 6, 2022
MIT Technology Review
Produced in partnership with Databricks
2022
This is an excerpt of the report “CIO vision 2025: Bridging the gap between BI and AI”, focusing on the Chapter 5 “The data priorities”, with the title above.
The data priorities (Chapter 5)
CIOs and other technology leaders clearly understand the necessity of putting their data management and infrastructure in good order to be able to realize their AI ambitions.
Over three-quarters (78%) of the enterprise technology leaders we surveyed say that scaling AI and machine learning use cases to create business value is the top priority of their enterprise data strategy over the next three years.
The AI leaders are almost unanimous on this point, 96% agreeing with the statement.
Figure 5: Data strategy and scaling AI (% of respondents agreeing with the statement)
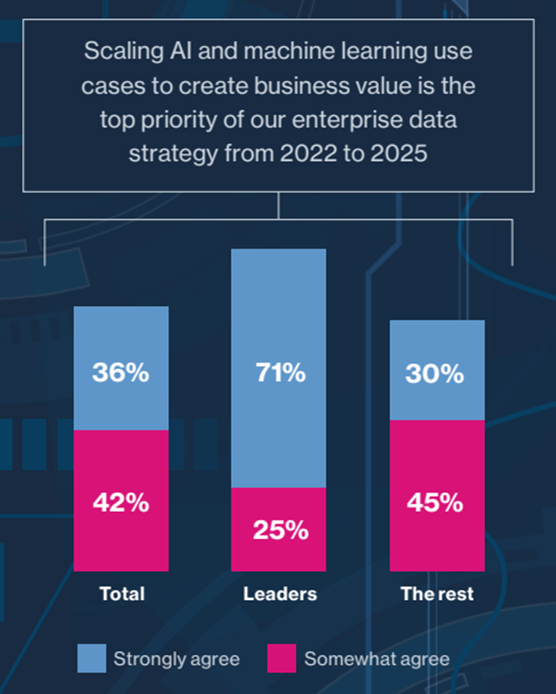
Source: MIT Technology Review Insights survey, 2022
“Companies have so much more to achieve from an AI perspective,” says Prasad Ramakrishnan of Freshworks.
“Challenge number one is to invest to build a good, solid, scalable data architecture and computing architecture, bringing more processing power to bear to handle extremely large volumes of data.”
The survey respondents concur. Asked which aspects of their company’s data strategy is most in need of improvement in order to support their AI goals, speed of data processing is at the top of their list.
This is followed in order of importance by four pressing needs: ensuring the sufficiency of data to feed AI models; improving the monitoring of data lineage in those models; improving the organization’s access to, and integration of, external data; and enabling greater collaboration on development data and AI models.
Over two-thirds of respondents (68%) — and nearly all leaders (99%) — say this is crucial to the success of their enterprise data strategy (see Figure 8, page 17).
Priorities in focus All of the technology leaders we interviewed for the study have overseen modernization of their data platforms and architecture to one degree or another in recent years.
… which aspects of company’s data strategy is most in need of improvement in order to support their AI goals?
speed of data processing is at the top of their list.
Figure 6: The aspects of data strategy most in need of improvement to enable companies to achieve future AI goals (top responses; % of respondents)
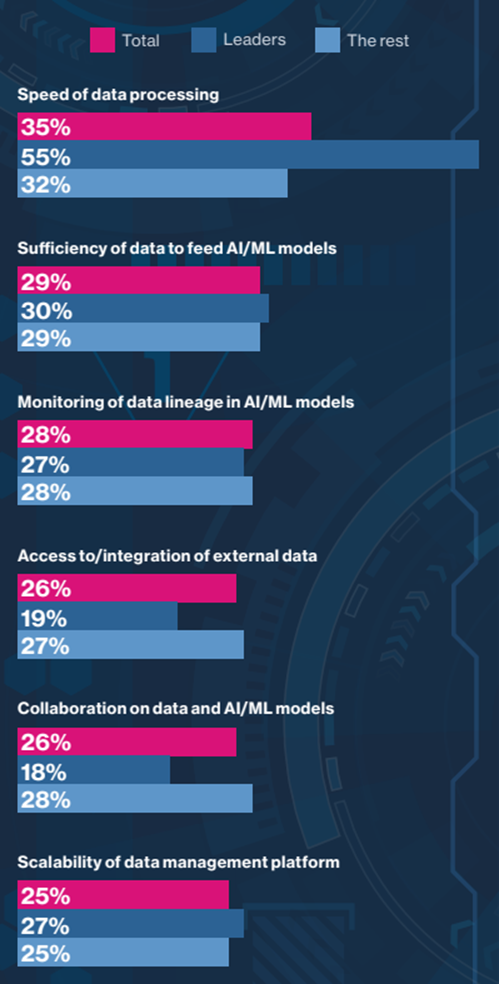
Source: MIT Technology Review Insights survey, 2022
The leaders are categorical about their need to boost data-processing speeds:
- 55%, deem this critical to realizing their AI objectives.
- compared with 32% of the rest
At least some of the platform investment made by companies will go toward unifying their data platform for analytics and AI.
Over two-thirds of respondents — and nearly all leaders — say this is crucial to the success of their enterprise data strategy.
To address these and other data management challenges, respondents’ companies are planning to significantly boost investment in upgrading their data capabilities between now and 2025.
The investment intentions are particularly aggressive among the leaders, providing perhaps the clearest indication of how this group aims to attain AI-driven status within the next three years.
Improving data security is all respondents’ top investment priority. Leaders, however, will increase their spending in this area by an average of 101% over the next three years, nearly double that of the rest of the sample.
The differentials are similar for other investment targets.
The leader group will invest 85% more over this period on data governance, 69% more on new data and AI platforms, and 63% more on existing platforms.
The analogous figures for the rest of the survey sample are 46%, 35%, and 38%, respectively .
Figure 7: Expected change in companies’ investment between 2022 and 2025 in selected aspects of their AI and machine learning capabilities (average [mean] increase across survey sample)
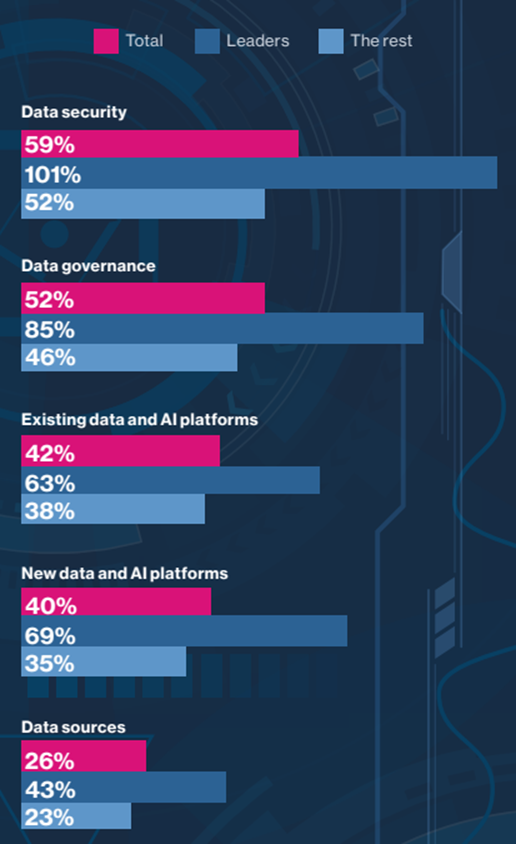
Source: MIT Technology Review Insights survey, 2022
At least some of the aforementioned platform investment by companies will go toward unifying their data platform for analytics and AI.
- Over two-thirds of respondents (68%) say this is crucial to the success of their enterprise data strategy ,
- and nearly all leaders (99%)
Priorities in focus
All of the technology leaders we interviewed for the study have overseen modernization of their data platforms and architecture to one degree or another in recent years.
While not all intend significant new investments in the immediate future, most emphasize that modernization is a work in progress, and they will be acting accordingly to ensure their specific data objectives are met.
For air carrier Virgin Australia, the priorities revolve around improving data management.
In the past 12 months, it has implemented a new, cloud-based data technology platform and a customer data platform to support its personalization objectives. That has also involved building an entirely new data-engineering team.
The ongoing challenge, says David Hogarth, the company’s chief information officer, is bringing together the disparate data sets the airline uses into one model.
“That will put us in a good position to build the long list of AI use cases that our business users are demanding from us,” he says.
With the technology platform it now has, adds Hogarth, properly unifying the data will also enable the company to democratize its use safely and securely.
“Our users don’t see value in acquiring the data themselves. Providing them with high-quality, consolidated insights from the data allows them to rapidly conduct analytics and achieve value quickly. That’s a highly attractive proposition to them.”
“Many companies don’t really know what they have in terms of their entire data estate or how they measure quality across it.
We’re addressing this in our new platform by putting cataloging, searchability, data quality management, and other capabilities around every data element.
We need to do all of this at the speed necessary to respond to customer and business needs.”
Jeremy Pee, Chief Digital and Data Officer, Marks & Spencer
Continuing to build scale and speed of data processing is a top priority for engineering company CNH Industrial, according to its chief information officer Marc Kermisch.
“A lot of the modernization we are engaged in is transforming databases and warehouses to be able to manage both unstructured and structured data in the cloud at scale and at speed,” he says.
“We aim to develop event-based capabilities that allow us to do modeling and analysis at or close to real time.”
At Marks & Spencer, says Jeremy Pee, the focus is on augmenting the data platform it has recently built with additional capabilities.
“What’s harder to do than build models and pipelines is solve for quality, use, and understanding of data.
Many companies don’t really know what they have in terms of their entire data estate or how they measure quality across it.
We’re addressing this in our new platform by putting cataloging, searchability, data quality management, and other capabilities around every data element. We need to do all of this at the speed necessary to respond to customer and business needs.”
Multi-cloud and open
The development of organizations’ AI and machine-learning capabilities is facilitated by a multi-cloud strategy — the use of services from two or more public cloud vendors.
A large majority of the survey respondents (72% overall, and 92% of leaders) believe a multi-cloud approach ensures the most flexible possible foundation for AI development.
Among other advantages, it enables AI teams to choose the most suitable platforms for the development of different use cases having specific resource requirements.
The latter include the sourcing, storage, or processing of data.
A multi-cloud strategy and an open approach to data architecture and standards often go hand-in-hand.
“Having a multi-pronged, multi-cloud approach, and then incorporating APIs and microservices as part of our data architecture, are key for us,” says Rowena Yeo of Johnson & Johnson.
Walgreens Boots Alliance has developed its new data platform with a focus on the use of open standards and open data, says Mike Maresca.
“At our core, we support the use of open source technologies on our cloud data platform, which increasingly supports integration across different cloud providers.
Open source standards and the ability to integrate cloud services across providers is important to our efforts to fully embed AI and machine learning in our business.”
Open standards are also facilitators of API-based data sharing, which companies in the healthcare community such as those mentioned above rely on for AI-based innovation.
It is increasingly becoming the case also in other industries, such as manufacturing (see “CNH Industrial: AI, open data, and the sustainable tractor”, page 18).
“Open-source standards are really important for our ability to take AI and machine learning to the next level,” says Kermisch.
“ Having a multi-pronged, multi-cloud approach, and then incorporating APIs and microservices as part of our data architecture, are key for us.”
Rowena Yeo, Chief Technology Officer & Global Vice President, Technology Services, Johnson & Johnson
Figure 8: The importance of a unified data platform (% of respondents agreeing with the statement)
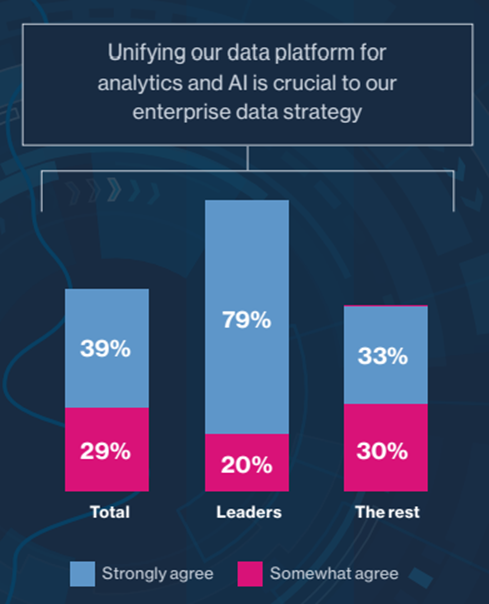
Source: MIT Technology Review Insights survey, 2022
CNH Industrial: AI, open data, and the sustainable tractor
The contributions that AI is making to sustainable agriculture are many and varied.
Precision farming, optimizing water and chemical use, locating carbon sinks, enabling urban farming to reduce deforestation — these are just a few of the existing use cases.
CNH Industrial plans to make another AI-enabled contribution to the cause — a sustainable tractor.
According to Marc Kermisch, the ability to access and analyze external data is vital to develop this use case.
“We need to understand the environmental footprint of each component we put into the tractor,” he says.
“That requires obtaining data from each manufacturer on, for example, its wastewater production, its energy consumption, and its hazardous material treatment.
- If a part contains hazardous materials, are they resolvable over time?
- Does the part need to be treated in a special way at the end of the tractor’s life to avoid damaging the environment?
- Is it recyclable?”
There are large supply chains that agricultural equipment producers like CNH Industrial need to work with to ensure their products leave the lightest possible footprint.
To make the right decisions in creating a sustainable tractor, says Kermisch, the necessary external data must be accessible and accurate, but those conditions do not yet exist.
“Some vendors may have created third-party databases, but we have no way of validating them to ensure we can trust the data,” he says. “Getting this wrong has enormous implications for sustainable farming.”
Kermisch is hopeful that the Science-Based Targets initiative (SBTi)4 — a not-for-profit partnership dedicated to fostering sustainability metrics — can help his industry meet this challenge.
A good solution, he says, would be for SBTi to anonymize the data generated by agricultural equipment producers, resulting in public data sets that can easily be validated.
That would ease concerns about sharing commercially sensitive data.
Such solutions exist in other fields of engineering, he says, and one should be possible for agricultural machinery.
With widely available, accurate, and reliable data, the AIenabled tractor will enable farming that is not just more efficient but also less carbon-intensive.
Precision farming, optimizing water and chemical use, locating carbon sinks, enabling urban farming to reduce deforestation — these are just a few of the existing use cases for AI in sustainable agriculture. CNH Industrial plans to make another: a sustainable tractor.
BOX: An industry lens on data and AI
Among this study’s objectives have been to compare the AI ambitions of different industries and to determine the extent to which industry approaches to removing impediments to AI development vary.
Three areas of difference stand out:
1.Retailers and manufacturers are more ambitious about becoming AI-driven.
2.Data constraints on AI development loom especially large in three industries.
3. Financial service providers display the strongest investment growth intentions.
1.Retailers and manufacturers are more ambitious about becoming AI-driven.
Within the leader group — where AI is expected to be critical in at least five functions by 2025 — respondent companies from the retail/consumer goods and automotive/manufacturing sectors are the most prominent.
Life science and healthcare organizations are also well-represented (see Figure 9).
2.Data constraints on AI development loom especially large in three industries.
- Among real estate and construction respondents, almost double the percentage of those in the overall sample (58% vs 30%) cite limitations of existing data technologies as an impediment to AI development.
- Some 40% of automotive/manufacturing respondents say the same.
- And 82% of executives in the latter sector (compared with 72% overall) state that data-related problems are more likely than other factors to jeopardize achievement of their future AI goals.
- That belief is shared by 80% of financial sector executives and
- 78% of those in real estate and construction firms.
3. Financial service providers display the strongest investment growth intentions.
Expected spending growth on data capabilities in the financial sector dwarfs that in others across several line items.
For example, investment to bolster data governance will increase by 74% between now and 2025, according to financial industry respondents, compared with 52% for the sample as a whole.
The analogous differential relating to existing data and AI platforms is 61% vs 42%, and for new platforms — 58% vs 40%.
Spending growth in these areas by retail/ consumer goods and automotive/manufacturing firms will also exceed the sample average
Figure 9: Where AI leaders are most numerous (% weight of leaders within each surveyed industry)
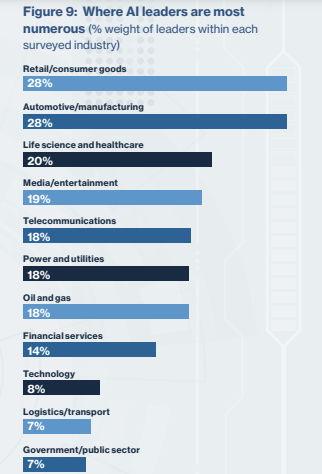
Source: MIT Technology Review Insights survey, 2022
Retailers and manufacturers are more ambitious about becoming AI-driven, but financial service providers display the strongest investment growth intentions.
Retailers and manufacturers are more ambitious about becoming AI-driven, but financial service providers display the strongest investment growth intentions.
Originally published at: https://www.databricks.com
Names mentioned
Prasad Ramakrishnan of Freshworks.
Jeremy Pee, Chief Digital and Data Officer, Marks & Spencer
chief information officer Marc Kermisch.
Marks & Spencer, says Jeremy Pee,
Walgreens Boots Alliance , Mike Maresca.
Rowena Yeo, Chief Technology Officer & Global Vice President, Technology Services, Johnson & Johnson