MIT Sloan Management Review
Thomas H. Davenport and Randy Bean
April 11, 2022
Executive Summary by:
Joaquim Cardoso MSc.
Transform Health — institute for research and strategy
AI Powered Health Care Unit
August 26, 2022
What is the complexity involved in Clinical AI?
- In order for AI-driven advancements to become a clinical reality, they have to be submitted to and approved by the Food and Drug Administration (or similar regulatory authorities outside the United States) as “AI/machine learning-based software as a medical device.”
- Several hundred such applications have already been approved.
- But those tools then have to be accepted by clinicians, merged into their clinical workflows, integrated into electronic health records and other systems, and reimbursed by health insurers.
What is the AI Clinical Maturity Index?
- Few clinical AI systems have successfully run this entire gantlet.
- And until clinical AI systems result in significant productivity advances, the economic value from them is in doubt.
Contrast that situation with the current potential for administrative AI systems in health care.
- These use cases don’t have to be approved by the FDA, or even by insurance companies (indeed, they are used in many cases to reduce friction with payers).
- They don’t have to be accepted by physicians, for the most part.
- And while they do have to be integrated with administrative workflows and systems, cloud- and API-based AI systems make the process much easier.
- In terms of economic value, the case for administrative AI is also much clearer.
What is the potential for Administrative AI in Health Care?
- Administrative costs in the U.S. averaged $2,497 per capita in 2017–34% of total health care costs.
- Health care economists in the U.S. often argue that reducing administrative costs is one of the most feasible ways to cut overall costs for health care.
- Some of these proposals involve automation and AI, such as an automated claims clearinghouse and automated prior-authorization processes. The potential here is significant.
What are some of the opportunities?
- Opportunities for Revenue Cycle AI
- Better Management of Scarce Resources
- AI in the Health Care Supply Chain
Conclusion
- We’re not saying that health care providers and payers should give up on clinical applications of AI, …
- … but the challenges and cycle times for developing and implementing those advances mean that many organizations will want to strongly consider administrative AI as well.
- If that type of AI can substantially reduce the cost of care, it could be as useful to the health care system overall — and many patients individually — as any clinical breakthrough.
ORIGINAL PUBLICATION (full version)
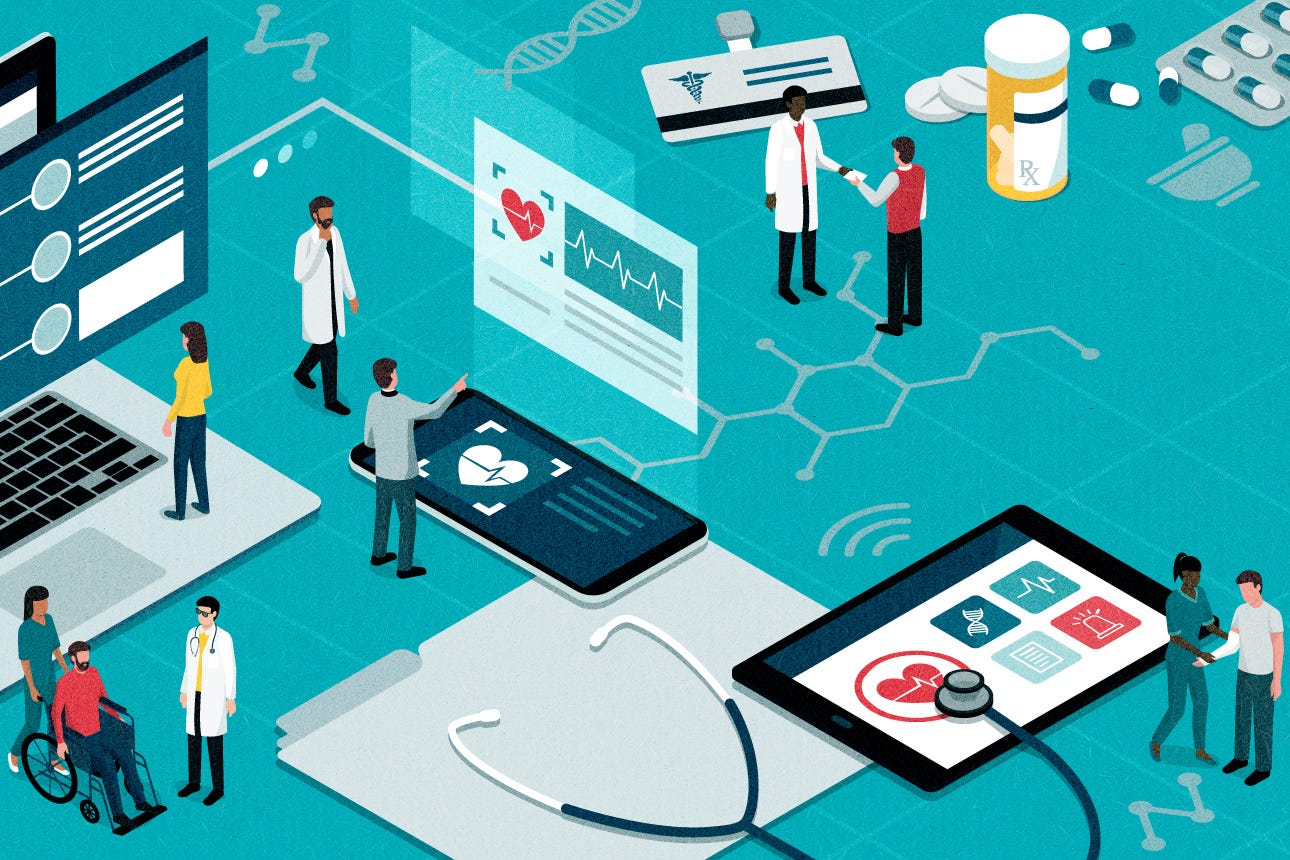
Clinical AI Gets the Headlines, but Administrative AI May Be a Better Bet
MIT Sloan Management Review
Thomas H. Davenport and Randy Bean
April 11, 2022
Introduction
AI for health care is all the rage.
Who wouldn’t be excited about applications that could help detect cancer, diagnose COVID-19 or even dementia well before they are otherwise noticeable, or predict diabetes before its onset?
Machine and deep learning have already been shown to make these outcomes possible.
Possible, that is, in the research lab.
In health care, there is often a long lag between research findings and implementation at the bedside.
In order for AI-driven advancements to become a clinical reality, they have to be submitted to and approved by the Food and Drug Administration (or similar regulatory authorities outside the United States) as “AI/machine learning-based software as a medical device.”
Several hundred such applications have already been approved.
But those tools then have to be accepted by clinicians, merged into their clinical workflows, integrated into electronic health records and other systems, and reimbursed by health insurers.
Few clinical AI systems have successfully run this entire gantlet.
And until clinical AI systems result in significant productivity advances, the economic value from them is in doubt.
Thus far, for example, we’re confident that they haven’t replaced a single human clinician.
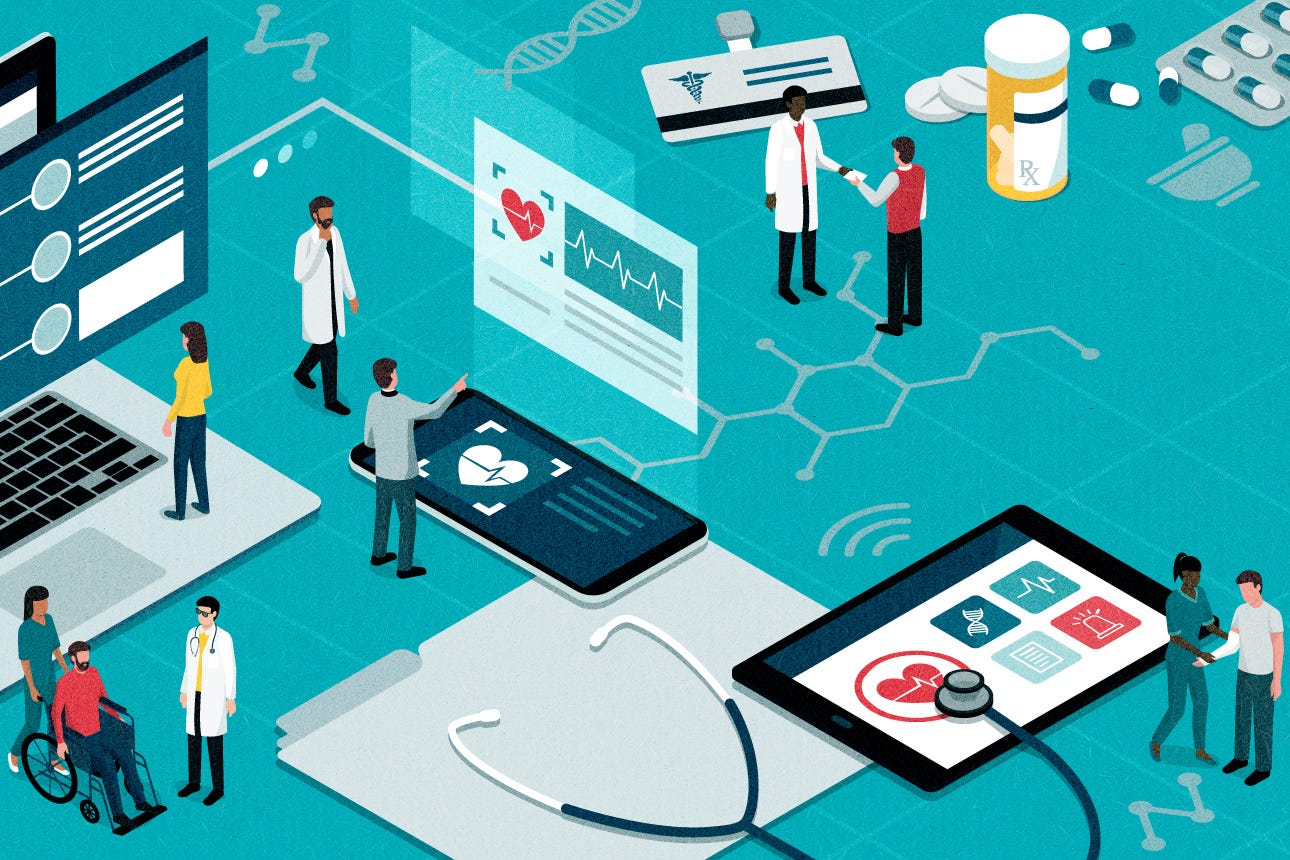
Contrast that situation with the current potential for administrative AI systems in health care.
These use cases don’t have to be approved by the FDA, or even by insurance companies (indeed, they are used in many cases to reduce friction with payers).
They don’t have to be accepted by physicians, for the most part.
And while they do have to be integrated with administrative workflows and systems, cloud- and API-based AI systems make the process much easier.
In terms of economic value, the case for administrative AI is also much clearer.
Administrative costs in the U.S. averaged $2,497 per capita in 2017–34% of total health care costs.
Administrative costs in the U.S. averaged $2,497 per capita in 2017–34% of total health care costs.
Health care economists in the U.S. often argue that reducing administrative costs is one of the most feasible ways to cut overall costs for health care.
For example, David Cutler, a Harvard economist who was one of the architects of the U.S. Affordable Care Act (“Obamacare”) system, has proposed a series of changes to administrative processes that he argues could save $50 billion in costs and “result in greater satisfaction for both patients and providers.”
Some of these proposals involve automation and AI, such as an automated claims clearinghouse and automated prior-authorization processes. The potential here is significant.
… a series of changes to administrative processes that he argues could save $50 billion in costs and “result in greater satisfaction for both patients and providers.”
Some of these proposals involve automation and AI, such as an automated claims clearinghouse and automated prior-authorization processes. The potential here is significant.
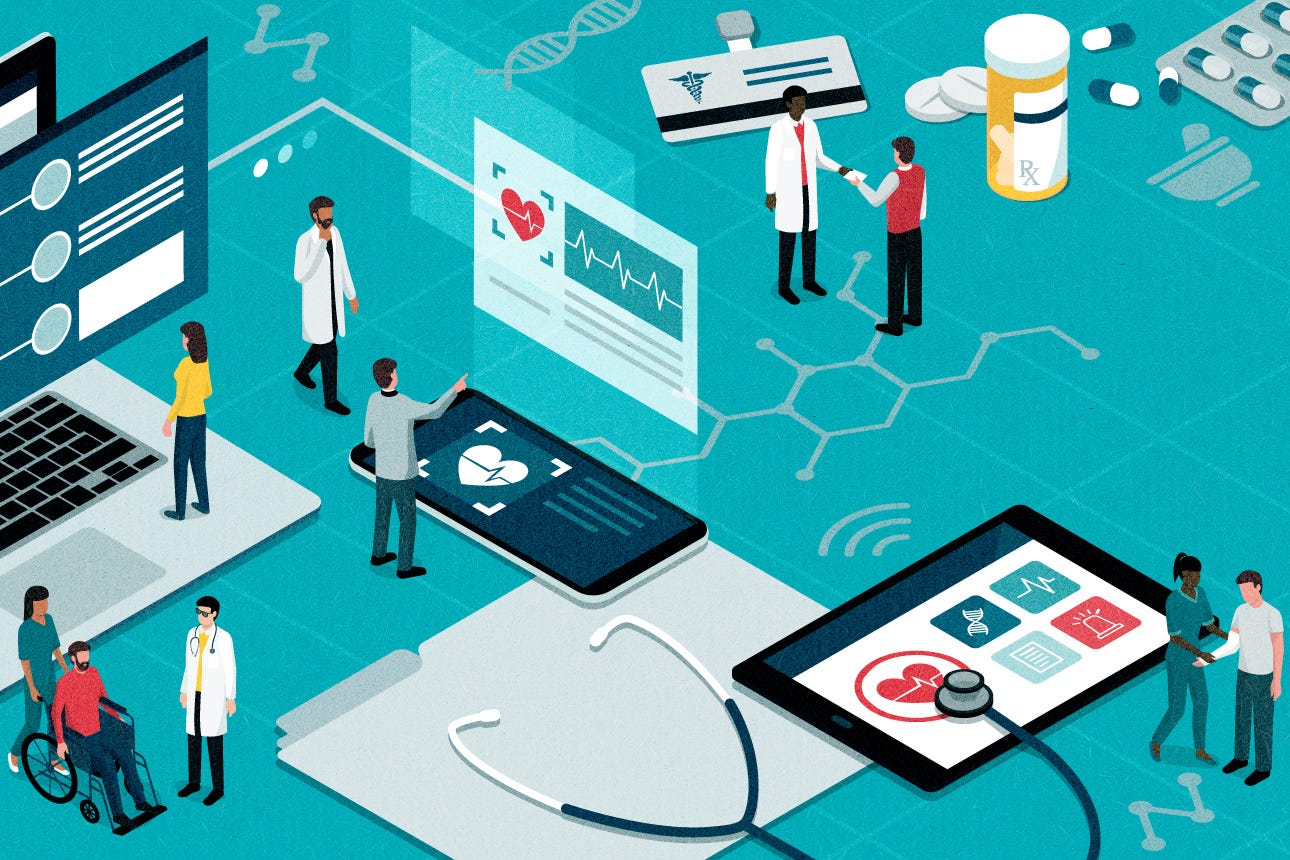
Opportunities
- Opportunities for Revenue Cycle AI
- Better Management of Scarce Resources
- AI in the Health Care Supply Chain
1.Opportunities for Revenue Cycle AI (including FWA)
Foremost among the opportunities for administrative AI is the revenue cycle area — authorization, billing, and payments.
This is a contentious and labor-intensive area for many health care providers and payers.
Several insurance companies, including Anthem, United Healthcare’s Optum, and Florida Blue, are automating the prior-authorization process using AI.
One study estimated that manual prior authorizations can take up to 16 hours per week for physicians.
… manual prior authorizations can take up to 16 hours per week for physicians. Several insurance companies,… are automating the prior-authorization process using AI.
Coding medical treatments for reimbursement and record-keeping is a challenging task for humans, with over 55,000 different codes in the latest version of the International Classification of Diseases.
Several companies have already implemented coding assistance AI systems that translate clinical notes into codes, but for now they still require review by human coders.
Coding medical treatments for reimbursement and record-keeping is a challenging task for humans, with over 55,000 different codes …
Several companies have already implemented coding assistance AI systems that translate clinical notes into codes, but for now they still require review by human coders.
Another challenge for humans in the revenue cycle is the estimation of medical bills before treatment.
Complex billing and payment arrangements make estimation difficult, but patients are more likely to pay medical bills when they have accurate estimates, and the U.S. government now requires them — although many providers are not compliant.
Baylor Scott & White Health’s system, however, has used a machine learning-based system to create accurate estimates based on past billing data.
About 70% of its estimates are created without human intervention, and the estimates have led to a 60% to 100% improvement in point-of-service collections.
Providers and payers also engage in a lot of back-and-forth about payments and when they will be made.
This is another great area for the application of automation and AI. Waystar, the provider of Baylor Scott & White’s estimate tool, automates the process of checking claims statuses with insurance companies’ accounts payable departments to see whether reimbursements have been made — a process previously done via phone.
It seems likely that “Have your AI call my AI” will become a common refrain.
It seems likely that “Have your AI call my AI” will become a common refrain.
While nobody likes to talk about it much, there is also a lot of FWA — fraud, waste, and abuse — in health care payments.
This area is well suited to the use of machine learning (and, in some cases, rule-based expert systems, a previous generation of AI) for claims analysis.
Insurers have told us that the ROI for such systems is among the highest of all AI investments: One large health insurer we spoke with saves a billion dollars annually through AI-prevented FWA.
One large health insurer we spoke with saves a billion dollars annually through AI-prevented FWA.
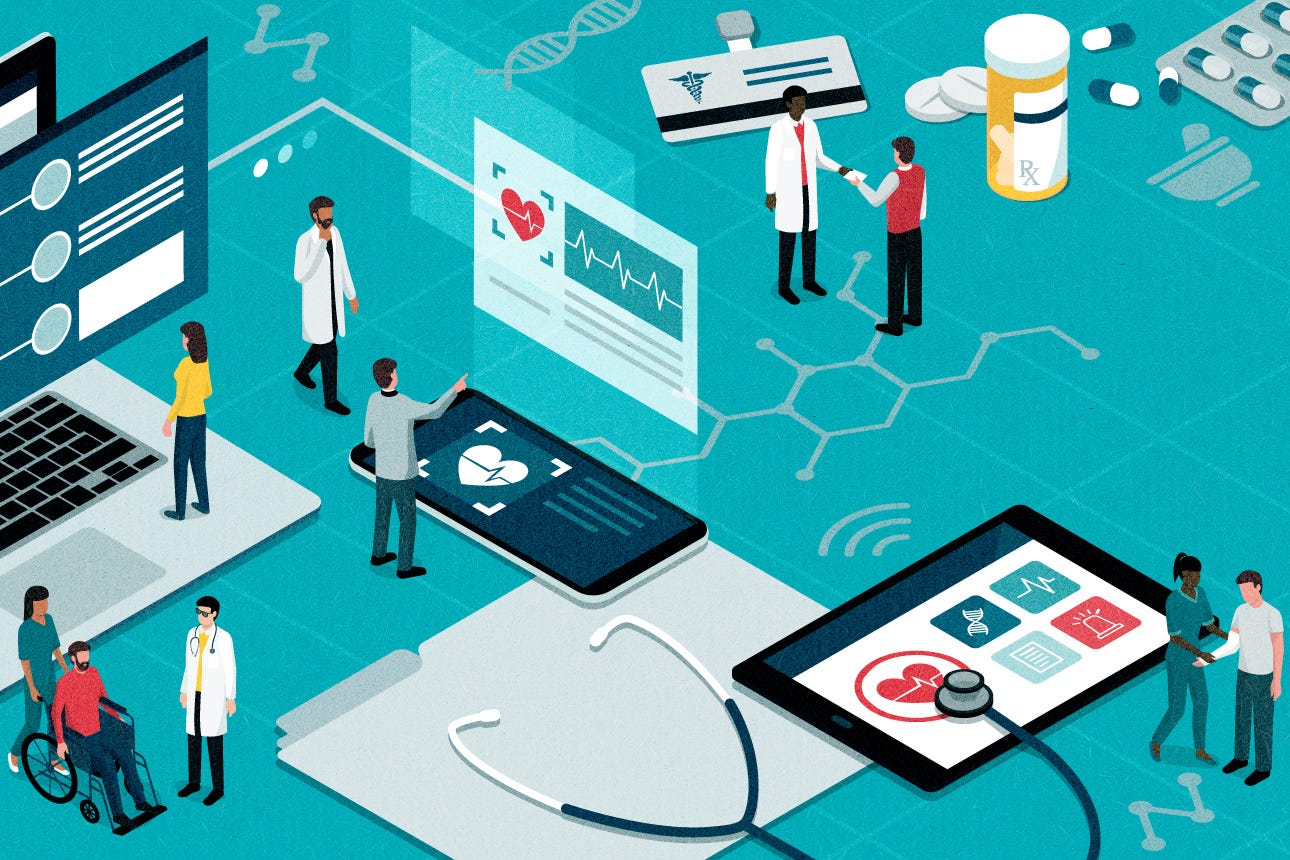
2.Better Management of Scarce Resources
Health care providers have a variety of scarce resources — including clinicians, operating rooms, beds, and expensive equipment — that are challenging to schedule effectively.
All of these resources are increasingly managed with the help of AI. For example, the Mayo Clinic has over 300 surgeons and 139 operating rooms and has used AI to more efficiently organize both staff and space.
In a project using AI to test better ways for spinal surgical scheduling, it was able to reduce doctor overtime by 10% and increase utilization of its space by 19%. (It is also pursuing many clinical AI projects.)
A Norwegian scheduling software company for medical staff, Globus.AI, claims that the increased scheduling precision from its software allows hospitals to fill 40% more shifts.
In a project using AI to test better ways for spinal surgical scheduling, it was able to reduce doctor overtime by 10% and increase utilization of its space by 19%.
Radiology imaging equipment is notoriously expensive (and often profitable to providers), and efficient utilization is critical.
Massachusetts General Hospital has developed an algorithm that predicts whether a particular patient is likely to show up for an appointment.
If the patient has a high likelihood of not showing up, they might receive more reminders or other interventions.
Mass General researchers also developed an automated language translation system that translates radiology instructions for non-English-speaking patients; it resulted in a statistically significant reduction in variability of radiology procedure times for those patients.
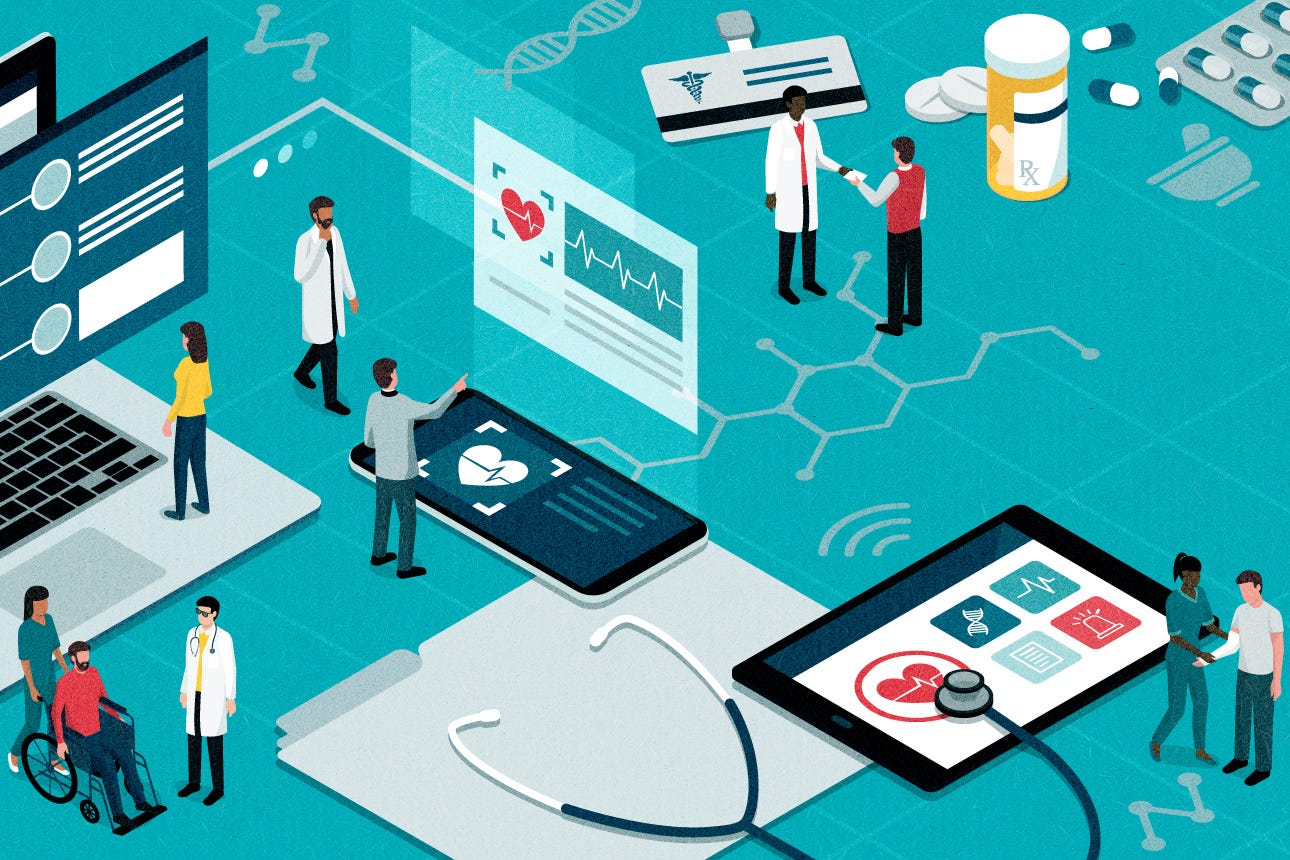
3.AI in the Health Care Supply Chain
Hospital supplies are also an expensive resource that AI can help to manage.
Some supplies, such as a pacemaker or drug-eluting stent, can cost thousands of dollars.
It can cost over $10,000 for a month’s supply of drugs for a U.S. patient.
The consulting firm Navigant estimates that U.S. hospitals spend over $25 billion per year in unnecessary supply chain costs.
Minimizing excess inventory while providing the appropriate supplies for patients’ needs may be too complex to be done well without AI.
… U.S. hospitals spend over $25 billion per year in unnecessary supply chain costs.
Minimizing excess inventory while providing the appropriate supplies for patients’ needs may be too complex to be done well without AI.
There are various ways that AI can improve health care supply chains, including matching demand and supply.
Machine learning models can predict how many patients of what type and with what health care needs will arrive at a hospital or doctor’s office, and the predictions can be matched against supply inventories.
As care increasingly moves from acute-care hospitals to a variety of other settings — including drugstores, rehabilitation facilities, clinics, and patients’ homes — AI can help supply chain managers optimize the transportation methods, frequency, and routing of supplies.
Robotic process automation and machine learning technologies can both help with ordering supplies: They can automatically check availability for back-ordered products, look up clinical equivalent drugs or devices, send out purchase orders and invoices, and match deliveries to invoices.
AI is also extracting key terms from contracts and embedding them in supply chain transaction systems and auditing processes.
One AI startup, Kalderos, keeps track of all relevant drug discounts and assesses whether discounts are compliant with federal and local regulations in the U.S.
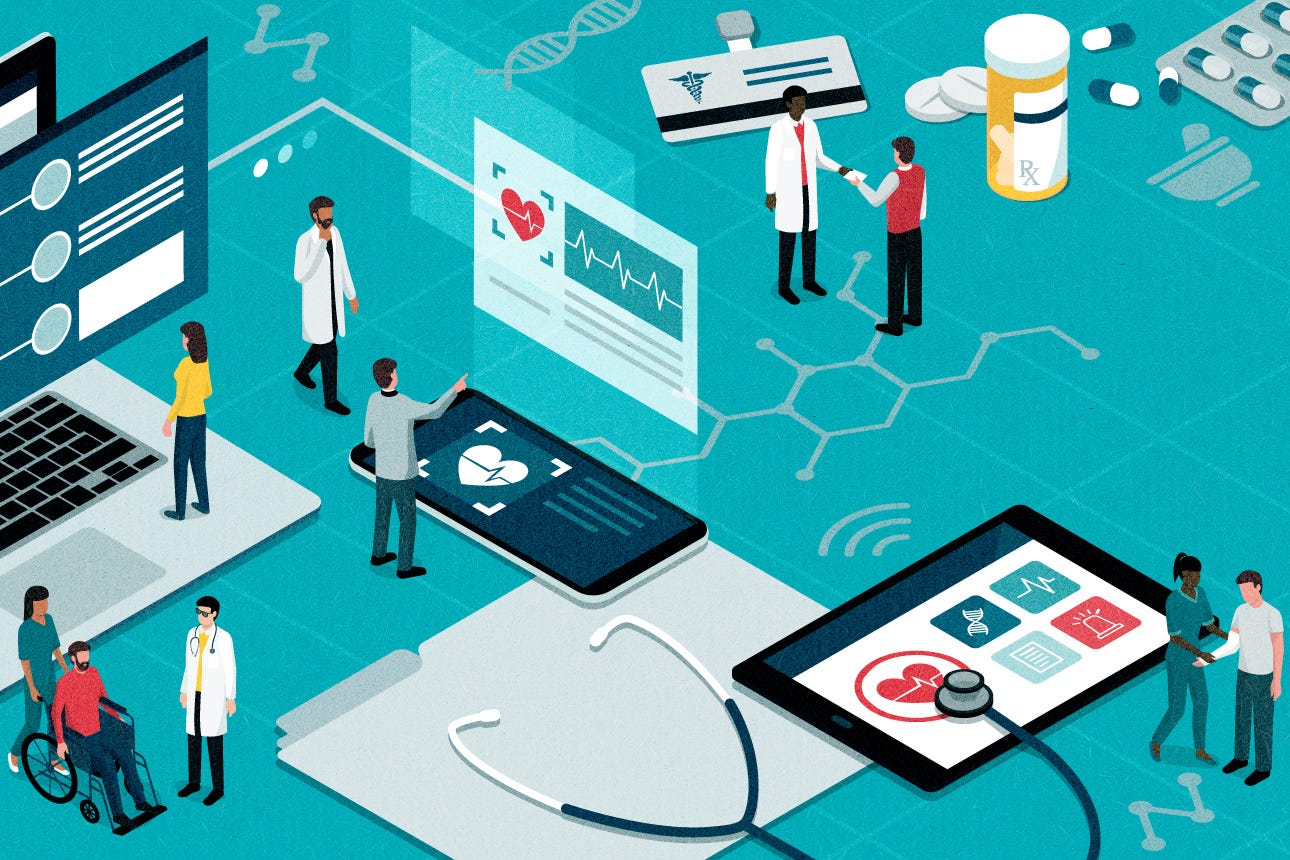
Conclusion
No one will win the Nobel Prize in medicine for applying AI to health care administration. Accolades and much of the media and public attention will go toward clinical applications of the technology.
We’re not saying that health care providers and payers should give up on clinical applications of AI, but the challenges and cycle times for developing and implementing those advances mean that many organizations will want to strongly consider administrative AI as well.
If that type of AI can substantially reduce the cost of care, it could be as useful to the health care system overall — and many patients individually — as any clinical breakthrough.
About the Authors
Thomas H. Davenport (@tdav) is the President’s Distinguished Professor of Information Technology and Management at Babson College, a visiting professor at Oxford’s Saïd Business School, and a fellow of the MIT Initiative on the Digital Economy.
Randy Bean (@randybeannvp) is an industry thought leader, author, and CEO of NewVantage Partners, a strategic advisory and management consulting firm he founded in 2001. He is the author of the book Fail Fast, Learn Faster: Lessons in Data-Driven Leadership in an Age of Disruption, Big Data, and AI (Wiley, 2021).
Originally published at https://sloanreview.mit.edu on April 11, 2022.
Names mentioned:
David Cutler, a Harvard economist who was one of the architects of the U.S. Affordable Care Act (“Obamacare”) system