This is a republication of the paper “Data Rich, Information Poor: Can We Use Electronic Health Records to Create a Learning Healthcare System for Pharmaceuticals?”, with the title above.
Site editor:
Joaquim Cardoso MSc
The Health Institute — for continuous health transformation
October 2, 2022
Clinical Pharmacology & Therapeutics (ASCPT)
Hans-Georg Eichler,Brigitte Bloechl-Daum,Karl Broich,Paul Alexander Kyrle,Jillian Oderkirk,Guido Rasi,Rui Santos Ivo,Ad Schuurman,Thomas Senderovitz,Luke Slawomirski,Martin Wenzl,Valerie Paris
04 September 2018
Abstract
- Judicious use of real-world data (RWD) is expected to make all steps in the development and use of pharmaceuticals more effective and efficient, including research and development, regulatory decision making, health technology assessment, pricing, and reimbursement decisions and treatment.
- A “learning healthcare system” based on electronic health records and other routinely collected data will be required to harness the full potential of RWD to complement evidence based on randomized controlled trials.
- We describe and illustrate with examples the growing demand for a learning healthcare system;
- we contrast the exigencies of an efficient pharmaceutical ecosystem in the future with current deficiencies highlighted in recently published Organisation for Economic Co-operation and Development (OECD) reports; and
- we reflect on the steps necessary to enable the transition from healthcare data to actionable information.
- A coordinated effort from all stakeholders and international cooperation will be required to increase the speed of implementation of the learning healthcare system, to everybody’s benefit.
Outline of the publication
- Abstract
- What Is a Learning Healthcare System?
- Why Do We Need a Learning Healthcare System That Harnesses RWD?
- Case study 1. Oncology treatment combinations
- Case study 2. Duration of anticoagulant therapy
- Case study 3. Gene therapy for thalassemia
- Can We Overcome the Current Research vs. Practice Divide?
- Consent, Data Protection, and the Patient Position
- Are We Ready to Implement the Learning Healthcare System?
- Proposals for Action
- Who Stands to Benefit From the Learning Healthcare System?
- Conclusions
Can We Overcome the Current Research vs. Practice Divide? [What can we learn from other industries? ]- [excerpted]
Before addressing the question, let us contextualize the issue of a learning system and consider how data support both the use and the development of products in other high-technology sectors.18
- For example, aircraft manufacturers evaluate and manage systems on board their aircraft as well as manage electronic controls and mechanical features.
- They can analyze in real time sensor information received from planes that are in the air to support safe operation.
- However, integrated with their own historical data on aircraft performance and maintenance, the analyses also support the development of new services or products.
- In agriculture, farmers generate data that are used by companies producing machinery, such as tractors or harvesters.
- For example, sensors in some of the latest equipment can help farmers manage their fleet of vehicles, reduce tractor downtime, and save resource consumption.
- Geocoded maps of fields and real-time monitoring of every activity, from seeding to harvesting, are used to raise agricultural productivity.
- The same sensor data can then be reused and linked with historical and current data on weather patterns, soil conditions, fertilizer use, and crop features, to predict and optimize production.
- At the same time as cultivation methods are improved and the know-how of skilled farmers is made widely available, these data support R&D into next-generation products.
- Although comparison of these industries with health care has obvious limitations, the examples illustrate how everyday use and R&D are not deemed two separate activities in these sectors; …
- … the concepts of future learning and rapid feedback loops between use and R&D are built into the system, which is often referred to as data-driven innovation.
- Contrast this with the traditional way pharmaceuticals are developed and used: based on a series of high-profile and disturbing experiences, legislation, policy, and mindsets have evolved to establish a separation of research (“learning”) from everyday practice (“using”).
- Can we learn from the other industries? Are there possible analogies to e.g., the aircraft or agricultural industries, or is health care too different and are the issues too sensitive?
Conclusions [excerpted]
- Perpetuating the 20th-century model of the dedicated research setting and relying (almost exclusively) on RCTs will not allow for translating the current pace of progress in the life sciences into new and better treatments for patients.
- It will also not enable decision makers to navigate complex scenarios in the future, be they related to investment, regulatory, financing, or patient-level decisions.
- We have discussed key aspects of the future learning healthcare system as it could apply to pharmaceuticals and complement RCT information.
- Although the vision described above and in Figure 1 may appear ambitious, we have no doubt that the learning healthcare system is achievable, with a concerted effort on the part of key players.
- There are additional caveats. We have limited the scope of this article to data that are routinely collected throughout the delivery of health care. However, even with “perfect” eHRs, other elements and data sources would be essential for a true learning health system.43
- These are expected to include omics data, data from wearable sensors, clinical trials, registries, and others.
- To grapple with such a broader, and messier, range of elements may also require advancements in computing power, data analytics, and data security protections, to advance governance and operational readiness within and between healthcare systems.44
- Many stakeholders would likely agree on the potential benefits and on what should be in place; all the basic technology is available; there are many steps taken in the right direction, yet progress remains patchy.
- A coordinated effort from all stakeholders and international cooperation will be required to reduce resource requirements for individual actors; reduce uncertainty and associated concerns about the adoption of new approaches and methods; and help sharing failures and success.
- On the upside, this will increase the speed of implementation of the learning healthcare system, to everybody’s benefit.
Infographic (Figure 1)
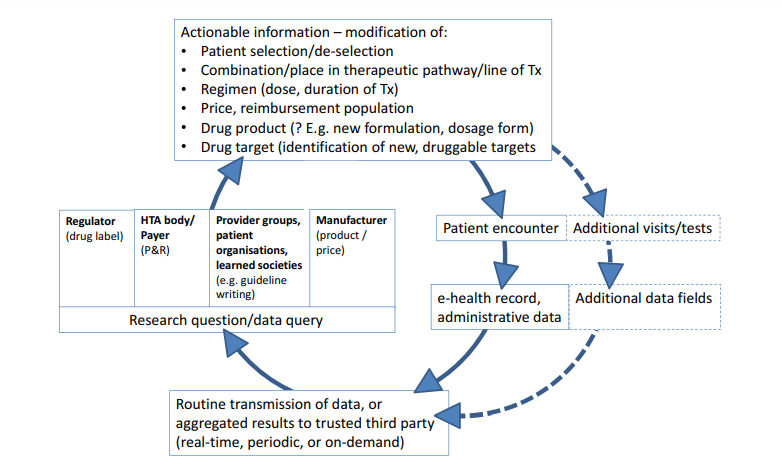
ORIGINAL PUBLICATION
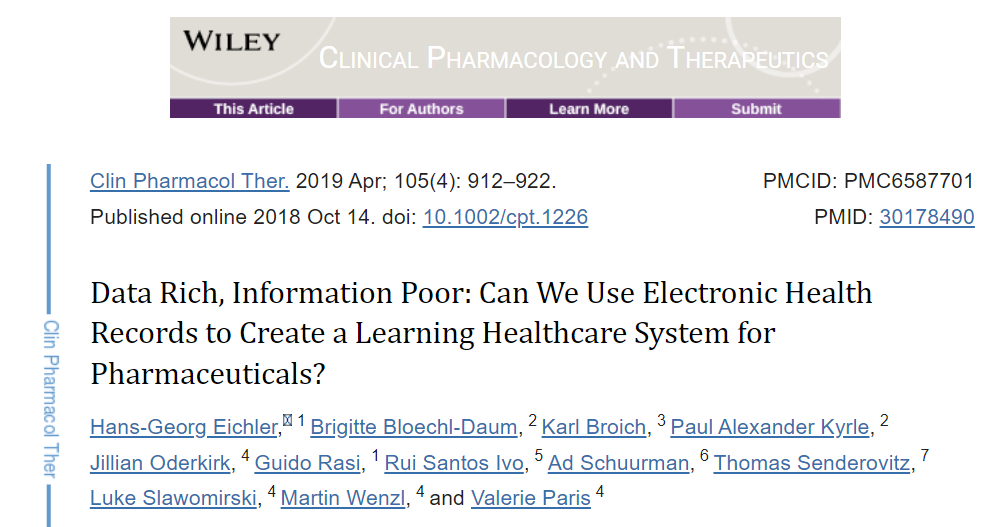
Introduction
High hopes are currently riding on the use of “real-world data” (RWD) to improve the delivery of health care.
For pharmaceuticals, there is an expectation that judicious use of RWD will make all steps in the development and use chain more effective and efficient, including research and development (R&D), regulatory decision making, health technology assessment (HTA), pricing and reimbursement decisions, and treatment.
We share many of these expectations and consider RWD and especially electronic health records (eHRs) as an important but underused resource.
The increased opportunity to analyze RWD and the development in analytics methods will undoubtedly create many advantages for patients.
Increased focus on the importance of patient involvement and the widespread discussion on patient insight and engagement will complement the technical developments.
However, we are somewhat sobered by findings on the use of healthcare data recently published by the Organisation for Economic Co-operation and Development (OECD):
A report on the “Readiness of electronic health record systems to contribute to national health information and research”1 and a complementary study on “Health data governance; privacy, monitoring and research”2 shed light on the usability of various forms of RWD in developed economies.
Although all countries are investing in health data infrastructure, and eHRs are now widely used, significant cross-country differences exist in data availability for secondary use.
Even the best positioned countries still face challenges that may limit their future success in harnessing the potential of RWD.
In this article, we describe and illustrate with examples the growing demand for a “learning healthcare system” that makes full use of RWD to complement evidence based on randomized controlled trials (RCTs); we contrast the exigencies of an efficient pharmaceutical ecosystem in the future with current deficiencies highlighted in the OECD reports; and we reflect on the steps necessary to enable the transition from healthcare data to actionable information.
RWD are often understood to comprise a wide range of data sources, including data from social media, wearable devices, and Internet searches.
Although such data may add value in the future, this article will focus on data that are collected in routine health care, mainly eHRs generated in primary care physician offices, specialist offices, and hospital settings as well as electronic prescribing systems, insurance claims, and other data collected routinely.
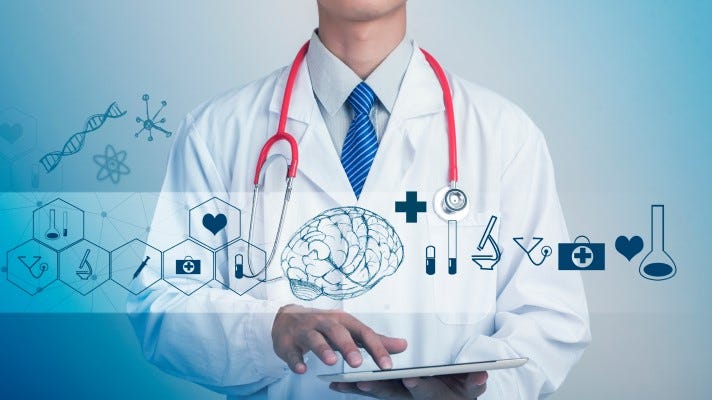
What Is a Learning Healthcare System?
A learning healthcare system has been defined3 as a system in which, “science, informatics, incentives, and culture are aligned for continuous improvement and innovation, with best practices seamlessly embedded in the delivery process and new knowledge captured as an integral by-product of the delivery experience. [… Such systems …] explicitly use technical and social approaches to learn and improve with every patient who is treated.”
We have added the emphasis in the above quotations to highlight the notion that everyday healthcare delivery and knowledge generation are intricately linked — not activities separated by an intended or unintended firewall, as is the case with most patient encounters today.
For a high-level overview of what a learning system based on feedback loops could look like in the pharmaceutical ecosystem and what kind of actionable information it might yield, please refer to Figure 1 .
Figure 1
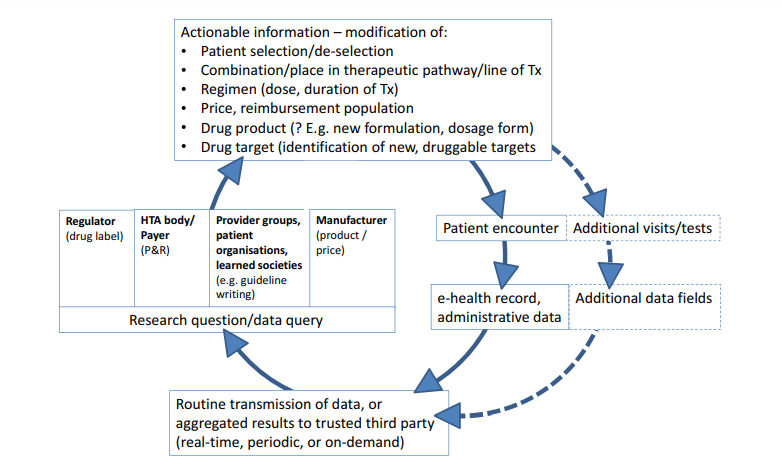
The learning healthcare system applied to pharmaceuticals.
The graph depicts the vision of repeated cycles of “learn and improve” that are expected to make the drug development and use chain more effective and efficient.
The clockwise cycle starts at the right-hand side with a patient encountering a healthcare professional.
This could happen in a hospital, office, or any other setting; the encounter will generate entries in the patient’s eHR, electronic prescribing system, and/or other administrative databases that are transmitted to a third party (bottom of graph).
We assume that a third party may likely be necessary in many healthcare systems to ensure trust in the overall process, but specifically to guarantee patient data protection and anonymity as well as gatekeeper and custodian functions and, where needed, to ensure linkage across different data sources (e.g., linking hospital and primary care data).
Where individual patient data cannot be transmitted and need to remain behind local firewalls (e.g., for legal reasons), a distributed data approach will be required.
In such cases, the third party could act as a conduit to distribute queries in the form of standardized computer programs to local database owners who run the queries and return aggregated results to the third party (“share answers not data”).
Depending on the country and healthcare system, the third party may be a new dedicated organization, a national regulator or health service, or another existing entity.
Following agreed procedures, data or aggregated results will be made available to vested stakeholders to answer research questions or other data queries (left-hand side of graph).
Relevant research questions may be raised by a drug regulator and relate to on-market benefit–risk assessment in various patient populations or by HTA bodies/healthcare payers about on-market drug use, value, relative effectiveness, cost-effectiveness, coverage, or pay-for-performance and risk-sharing schemes.
Alternatively, provider groups, patient organizations, or learned societies could initiate data queries for research projects or to inform clinical guideline development. Last, the data (or aggregated results) can be used by drug manufacturers for postlicensing monitoring, risk management, or even postlicensing or de novo drug development.
The kind of actionable information that could be generated from the data is illustrated by examples at the top of the graph:
information on favorable or unfavorable treatment outcomes might help select or deselect patients with defined (phenotypic or genotypic) characteristics; in turn, this will be reflected in an optimized treatment-eligible population in the regulatory label and for payer coverage; the information can also inform the value assessment, providing a basis for price negotiations; results of secondary data analysis may provide guidance on the best use of a drug, e.g., on best combinations, place in the therapeutic pathway (e.g., second- vs. first-line treatment), start–stop criteria for treatment or dose selection; perhaps less frequently, information may be translated into new drug dosage forms or formulations (e.g., for special populations, like elderly people) or support hypothesis generation on novel treatment targets.
Further downstream (right-hand side of graph, dotted lines), the information may trigger a change of the routine practice of medicine, i.e., of future patient encounters:
additional patient visits (or longer intervals), the incorporation of new or different tests, and, ultimately, the modification of the eHR itself (by way of adding new data fields).
At that stage, the learning healthcare system would have achieved its goal and a new cycle of “learn and improve” starts.
As a drug moves along its developmental and on-market phases, the nature of research questions asked is expected to change.
Although the focus during the R&D phase is expected to be on, e.g., quantification of unmet medical need, description of the natural history of a patient (sub-) population, or elucidation of standard of care, the focus during the on-market phase is likely on optimization of the treatment population, quantification of effectiveness in clinical practice, and safety, along with available therapeutic options.
The scope of RWD-based knowledge generation is broader than classical “phase IV” studies. eHR, electronic health record; HTA, health technology assessment; P&R, pricing and reimbursement; R&D, research and development. RWD, real-world data; Tx, treatment.
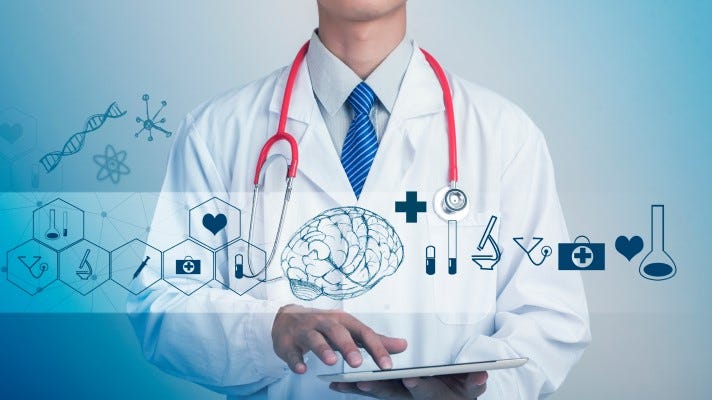
Why Do We Need a Learning Healthcare System That Harnesses RWD?
Under the traditional paradigm, assessment of the benefits and harms of any new drug takes place in dedicated research settings.
Data are generated prospectively (i.e., according to preplanned and vetted protocols), studies require preauthorization, and patients are asked to actively enroll in a research project.
In terms of research method, the (double-blind) RCT became the standard for demonstrating efficacy to support marketing authorizations, HTA, reimbursement decisions, and individual treatment decisions.
Regulators, often in collaboration with academia and industry, already make use of RWD in pharmacovigilance processes.
These aim to broaden the funnel of incoming data, primarily for the monitoring of drug safety and, in particular, for the assessment of rare and/or late-onset safety issues.4–6 Preplanned and vetted study protocols (e.g., by regulators in the framework of scientific advice) have now become standard for many RWD studies. Although these initiatives have been largely successful for the purpose of monitoring safety, we argue that scientific developments — some of which are briefly described below and in Box 1 — necessitate much broader use of RWD and the implementation of all components of the learning healthcare system.
Box 1. Beyond the research setting: highlighting the need for a learning healthcare system
Case study 1. Oncology treatment combinations
Pharmacologic treatment of hemato-oncologic diseases and cancer is now progressing fast, but 20th-century hopes for “the one” magic bullet will not likely materialize anytime soon.
Combination therapy offers the potential for antitumor activity in the greatest number of patients.7
The many potential molecules and pathways to target include immune modulators and other classes of drugs as well as other treatment modalities.
This abundance creates “combinatorial complexity.”8
The variables to be studied include dose selection, drug combination partners (to maximize synergy of effect and minimize toxicity), sequence of treatment (-combinations), washout periods, changing tumor characteristics over time, and a myriad of potential patient- and tumor-related biomarkers for treatment stratification.
It is impossible to study even a fraction of the permutations of treatment options in a prospective research setting, especially given rapidly changing treatment options.
Although innovative research and development strategies8 can help expedite the delivery of more effective therapies to some patients, it is unlikely that the optimal use of new agents can be fully characterized before licensing.
A learning healthcare system — with rapid uptake in clinical practice of novel developments (e.g., biomarkers) and well-documented individual treatment experiences linked to patient and tumor characteristics, fed back to drug developers and other stakeholder groups — will be the only hope to come to grips with “combinatorial complexity.”
Case study 2. Duration of anticoagulant therapy
Patients with unprovoked venous thromboembolism (VTE) have a recurrence risk of 5–10% per year after postevent anticoagulation is stopped.
A challenge is to stratify such patients according to risk of recurrence VTE, to distinguish patients who would derive a net clinical benefit from indefinite anticoagulant therapy from those in whom long-term anticoagulation would not be justified. Predicting VTE recurrence was previously not feasible.
The Vienna Prediction Model for assessment of recurrence risk to guide duration of anticoagulant treatment integrates clinical and laboratory risk factors. The model is based on a prospective cohort study9 that took ≈17 years from planning to completion and cost ≈12 to 15 million Euros. This was subsequently validated using an independent patient-level data set pooled from several other cohort studies that assessed, in part, the clinical utility of D-dimer (a laboratory test) in predicting recurrent VTE.10 The validation study required ≈6 months and <100,000 Euros.
Could the highly relevant research question (“how long should a patient be treated?”) have been answered retrospectively from electronic health records (eHRs), only faster and cheaper?
The answer is both yes and no: the most important predictors of recurrent VTE, male sex and site of VTE, could probably have been gleaned from eHRs in many healthcare environments. However, at the time when the study was initiated, many laboratory variables, such as gain-of-function mutations, F VIII (another laboratory test), and D-dimer, were not determined on a routine basis and could not have been incorporated in the initial development of the model. Their routine use and recording in the eHR became more widespread over time. Hence, these parameters could have been incorporated in a subsequent iteration of the prediction model, illustrating the need and opportunities for repeated assessments of research questions as health care evolves.
Case study 3. Gene therapy for thalassemia
Beta thalassemias are severely debilitating, life-shortening, rare, inherited blood disorders.
Gene therapy holds the promise of fixing a patient’s own bone marrow cells by transferring the normal gene into hematopoietic stem cells to permanently produce normal red blood cells.
One such product, LentiGlobin BB305, has a current development plan for once-only administration, and a conditional approval route is conceivable in the European Union.11 This would provide the initial basis for labelling and the value proposition. However, long-term follow-up will be needed to establish the duration of the effect and long-term safety. One safety concern over vector-based gene therapies is insertional mutagenesis leading to oncogenesis; it can take up to several years for tumors to develop.12 Because the risk level is likely to be low, follow-up will need to incorporate almost all exposed patients. Follow-up over decades (or even lifelong) in the interventional research setting is unrealistic and may not be necessary if robust information could be gleaned from eHRs. The learning healthcare system would be a key enabler for the development and safe use of gene therapies.
The “research-setting” paradigm with data generation tailored to the researchers’ needs and mostly protocol rather than care driven, and the RCT as the workhorse for knowledge generation, has served stakeholders well, but at least two major limitations have long been recognized.
First, the cost, complexity, and duration of prospective data generation: Current (regulatory) RCTs usually deliver robust results.
However, there is a price to pay: studies take place in a dedicated infrastructure, which needs to be set up by a study sponsor, comprising clinical investigators, staff, monitors, standard operating procedures, and other processes. The cost and operational complexity are considerable. The planning, approval, and run-up phase to the start of a prospective trial usually takes months to a few years. A direct consequence of this practical limitation is that the number of trials — and therefore the number of research questions answered — about a particular product will always be wanting.
This point was highlighted by recent experience by EUnetHTA, a European collaborative of HTA organizations.
The group wanted to conduct a relative effectiveness assessment of a new antidiabetic drug, canagliflozin, around the time of market launch. The authors identified a total of 18 relevant comparison scenarios. Unsurprisingly, head-to-head RCTs were unavailable for most comparisons.13 Given the timelines and resource requirements, it is unrealistic to expect that even the most critical of the required relative effectiveness information will become available from RCTs. It follows that HTA and payer organizations, as well as prescribing physicians and their patients, will have to either make choices in the absence of information or obtain information from other sources, such as RWD. (For more examples about the logistic limitations of conducting prospective clinical trials, see Box 1 .)
Second, the inability to detect rare treatment effects: RCTs (or any other interventional trials) are rarely large enough to accurately measure infrequent outcomes.
Consider an event with a probability of occurring in 1 of 1,000 patients; although this would not be considered rare, the probability of seeing one or more such events in a study of 1,000 exposed patients (which would typically make it a 2,000-patient RCT) is only 0.63. Note that just seeing one (or a few) events does not allow for estimation of the underlying probability with any accuracy, so this would constitute a low evidence standard of benefit–risk or value. Benoxaprofen (Opren), for example, a drug launched in 1980, had to be withdrawn 2 years after its launch because of reports of serious adverse effects, including 61 deaths.14 This was despite preceding clinical trials involving >3,000 patients.
The difficulty of detecting a modest increase in some event against a high natural background rate is perhaps an even greater challenge.
A few extra myocardial infarctions in a trial of elderly people can easily go unnoticed because common events usually do not arouse suspicion. Large observational data sets are the only practical means of acquiring such vital information.
These and other15, 16 shortcomings of RCTs have highlighted the need to complement RCTs with information from outside the clinical trial setting.
An area where the need for additional data sources and methods has been apparent for some time is new, highly promising medicines that are being fast tracked, and for which continued close monitoring and postapproval benefit–risk (re-) assessment is paramount. These (often highly priced) products also need monitoring to judge if the expected outcome is realized in everyday clinical practice, and to decide if a high price is still justified.
In addition, recent developments in the life-science area will likely make greater use of RWD a necessity in generating evidence.
Long-term outcomes
Over the next decade, more new medicinal products will likely be gene or cell therapies.
These products come with their own challenges for evidence generation: some of them are expected to be administered only once in a lifetime, but the effect size and duration as well as late-onset adverse drug reactions can only be ascertained after prolonged periods.
For some chronic diseases, like Alzheimer’s disease, treatment initiation at the time when symptoms develop may be too late to change the patient outcome.
Early disease interception in people with to-be-defined biomarkers is likely required, which means treating patients years or perhaps decades before they develop clinical symptoms.
Assessing the clinical efficacy of such treatments in an RCT (or other research settings) is impractical because patients will drop out over the years, rendering trial results uninformative.
Information on long-term clinical outcomes in these scenarios can only be gleaned from RWD that follow the lifelong treatment experiences of patients in routine practice.
Learning from interindividual variance
The trend toward precision medicine dictates that another aspect of evidence generation needs to be reconsidered.
In the past, interindividual variance has often been considered “noise,” and a high level of noise reduces the signal/noise ratio. Hence, most research trials were designed to minimize variance to maximize the chances of demonstrating a treatment effect. Yet, as understanding of the biological basis of variance and its implications for therapy selection improves (e.g., because of multiple different sets of mutations or other biomarkers), the interpretation of variance changes from a nuisance to be minimized to being the focus of research. The research question changes from: “Is A better than B in a defined group of patients?” to: “Given that compound A has been shown to modulate target X (i.e., it has shown pharmacodynamic activity), (how) can we identify patient subgroups who will benefit from A, rather than B?”17
Consider the case of abacavir (Ziagen), used to treat HIV infection: it was only the detection of a genetic biomarker that enabled prescribers to preidentify patients who are at high risk of developing a hypersensitivity reaction to abacavir. Screening out those likely to develop the reaction allowed for most patients to continue benefitting from the drug. This is remarkable because, in the past, often most patients were denied the potential benefits of a treatment to protect a few patients who might have experienced a serious adverse event and could not be identified beforehand.
Understanding the reasons for interindividual variance will be the key to getting the right treatments to the right patient.
Unfortunately, any realistic RCTs (or other research studies) will be hopelessly underpowered to address the complexity presented by large numbers of low-frequency biomarkers that drive interindividual variance. It would be unrealistic to assume that RWD analysis could provide an instant answer to all questions. An obvious caveat is that newly characterized biomarkers are not in routine use for some time and, once introduced, may not be sufficiently standardized to enable robust conclusions. Examples are given in Box 1 (case studies 1 and 2). There will be a time lag before they can be used for knowledge generation, dependent on the speed of adoption of new scientific developments in clinical practice.
Notwithstanding this limitation, we agree with Klauschen et al.8 who argue that “the requirement of the classical clinical trials that patients should be similar and groups should be homogeneous […] is irreconcilable with the molecular diversity and diverse therapeutic options of the future personalized medicine approaches […].”; if the current separation between learning and clinical use is maintained, we will not be able to keep researching benefits and small risks in ever smaller groups over longer periods.
Clearly, progress in the life-science area offers the potential for better patient outcomes.
But progress in science is outrunning our ability to generate the knowledge required to efficiently translate science into next-generation treatments, regulate drugs, or make informed reimbursement and treatment decisions. We argue that a truly learning healthcare system that harnesses RWD will be the only route to continued success in the pharmaceutical ecosystem.
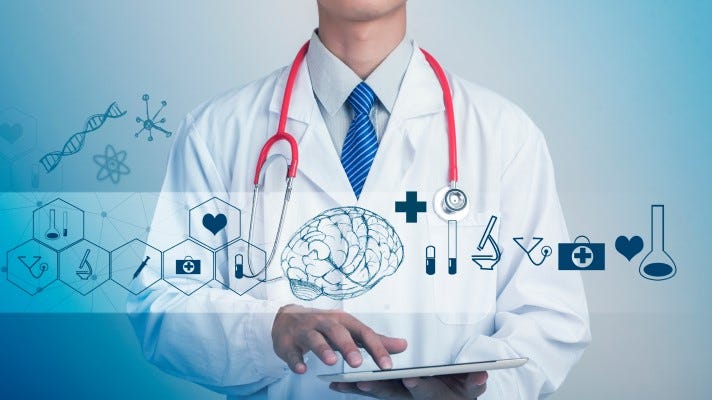
Can We Overcome the Current Research vs. Practice Divide?
Before addressing the question, let us contextualize the issue of a learning system and consider how data support both the use and the development of products in other high-technology sectors.18
For example, aircraft manufacturers evaluate and manage systems on board their aircraft as well as manage electronic controls and mechanical features.
They can analyze in real time sensor information received from planes that are in the air to support safe operation.
However, integrated with their own historical data on aircraft performance and maintenance, the analyses also support the development of new services or products.
In agriculture, farmers generate data that are used by companies producing machinery, such as tractors or harvesters.
For example, sensors in some of the latest equipment can help farmers manage their fleet of vehicles, reduce tractor downtime, and save resource consumption.
Geocoded maps of fields and real-time monitoring of every activity, from seeding to harvesting, are used to raise agricultural productivity.
The same sensor data can then be reused and linked with historical and current data on weather patterns, soil conditions, fertilizer use, and crop features, to predict and optimize production.
At the same time as cultivation methods are improved and the know-how of skilled farmers is made widely available, these data support R&D into next-generation products.
Although comparison of these industries with health care has obvious limitations, the examples illustrate how everyday use and R&D are not deemed two separate activities in these sectors; …
… the concepts of future learning and rapid feedback loops between use and R&D are built into the system, which is often referred to as data-driven innovation.
Contrast this with the traditional way pharmaceuticals are developed and used: based on a series of high-profile and disturbing experiences, legislation, policy, and mindsets have evolved to establish a separation of research (“learning”) from everyday practice (“using”).
With a focus on potentially high-risk interventional trials, the aim of this separation was primarily to protect patients against harm and allow them to give or refuse fully informed consent to enroll in studies.
In the research setting, researchers have offered explicit guarantees to trial patients that their data will not be widely shared.
At the time when the current systems of ethics and regulatory approval processes for research were created, limited thought was given to the learning opportunities that could arise from secondary analysis of data gathered in everyday practice.
This is unsurprising given that the state of record keeping (hand-written, scattered, and nonlinkable in physician’s offices or hospitals) did not provide much practical opportunity for secondary analysis.
Yet, the (unintended) consequence was an effective firewall between learning and using.
… the state of record keeping (hand-written, scattered, and nonlinkable in physician’s offices or hospitals) did not provide much practical opportunity for secondary analysis.
The world has changed. The 2016 survey1 of OECD countries showed that the use of eHRs is increasing fast.
Although some countries are still lagging, several indicated that 100% of primary care physician offices, specialist offices, and hospitals use eHRs.
Many others are following closely.
In a few years, the vast majority of patient encounters in clinical practice in most developed economies will be recorded in digital form.
Although this means that the first building block of learning healthcare systems has been laid, these systems have been built to enable daily practice.
In most cases, learning is not an explicit goal and the other building blocks are not yet in place.2, 18
Can we learn from the other industries?
Are there possible analogies to e.g., the aircraft or agricultural industries, or is health care too different and are the issues too sensitive?
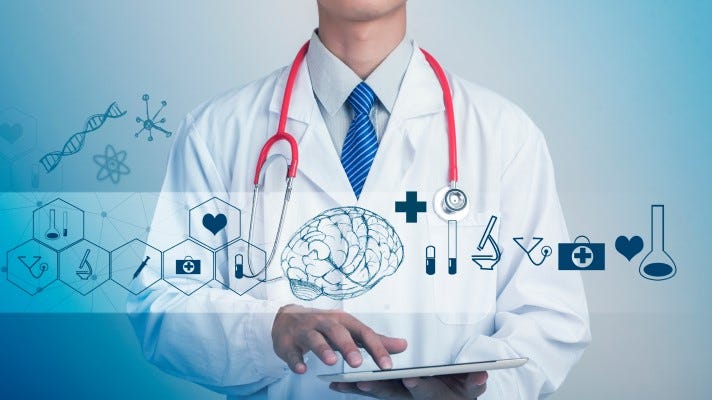
Consent, Data Protection, and the Patient Position
Consent and personal data protection are frequently cited reasons why health care is different from other sectors (and why purportedly we cannot learn from aircraft manufacturers or agriculture).
In a truly learning healthcare system, eHRs (in conjunction with other patient data) are used to generate new knowledge by answering research questions (Figure 1 ).
In many situations, the benefits will be accrued by future generations of patients, not primarily by the patients who contributed to the databases.
In so doing, patients become part of noninterventional research that usually cannot be preplanned because the research questions evolve over time and may not become apparent until long after the patient encounter or even after the patient’s death.
It follows that governance exigencies, including patient data protection, consent, ethics, and data access, are paramount — and far more demanding than in any other sector.
Some jurisdictions are taking steps toward best practices in data sharing, with appropriate protection of individuals’ data privacy and respect for the ability (or inability) to consent (see below).
However, it bears reminding that patients are the single most important stakeholder group regarding the learning healthcare system.
They are both the donors of personal clinical data and the ultimate beneficiaries from the knowledge gained. What are their views?
The European Patient Forum (EPF), an umbrella organization of specific chronic disease groups or national coalitions of patients at the European Union (EU) level, actively supported the “datasaveslives” petition at a time when EU institutions debated the final text of the EU Data Protection Regulation.
Patient groups lobbied to achieve sound protection of their privacy, and allowing data processing to continue for healthcare, public health, and research purposes.
Moreover, EPF’s position was that, although informed consent is a fundamental right and should be the rule, exemptions are needed in cases when it is practically impossible to seek consent or reconsent from research participants.19
In the United States, patient representatives have contributed or supported the Institute of Medicine recommendations to facilitate the conduct of health research.20
Some patient advocacy groups (e.g., Friends of Cancer Research) have taken an active role in exploring and supporting the use of “real-world evidence” in drug development and drug regulation.21
Protecting personal data while facilitating research is even more challenging in rare diseases because patients are much easier to identify (e.g., through crosscutting of databases).22
Nonetheless, a survey of patients with rare diseases revealed that they are positively disposed toward (genomics) research and toward allowing data and even biosamples to be shared internationally.23
It is encouraging to observe that those who have most at stake generally come out in favor of secondary use of their data.
We would assume that patients would overwhelmingly welcome and support the learning healthcare system, provided there are adequate safeguards for personal data protection.
Maybe we can learn from other sectors, after all.
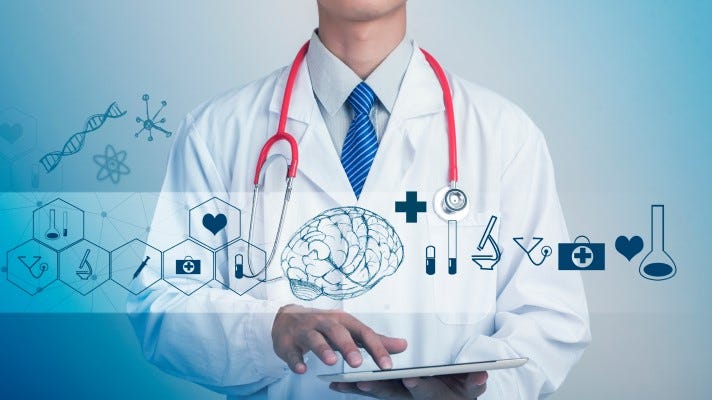
Are We Ready to Implement the Learning Healthcare System?
In the learning healthcare system, the way in which patient data are generated, stored, and used in the eHR is at the heart of the system (Figure 1 ).
Requirements for data standardization, quality, connectivity, and usability of eHRs will far exceed those of the routine use of eHRs.
For example, many future research questions will require linkage of longitudinal phenotype data and treatment outcomes (adverse events and efficacy outcomes) to “omics” data sets.
For pharmaceuticals, integrated data from different sources will be particularly helpful.
Hence, linkage of eHR data to shared RCT data will be key.
It is in this respect that the inadequacies of current eHRs used in the various healthcare systems constitute the biggest technical bottleneck to the learning healthcare system.
Since 2010, an OECD working group has been repeatedly surveying member countries regarding the development of national health data assets and their use to improve health, healthcare quality, and health system performance.
In these studies, an eHR was defined as “the longitudinal electronic record of an individual patient that contains or virtually links records together from multiple Electronic Medical Records, which can then be shared across healthcare settings.
It aims to contain a history of contact with the healthcare system for individual patients.”
The surveys aimed to assess the following:
(i) the technical and operational readiness, including data quality challenges; and
(ii) health data governance readiness.
Participating countries received a score for each dimension.
Results and survey items are shown in Figure 2 and briefly summarized below.1, 2
Figure 2
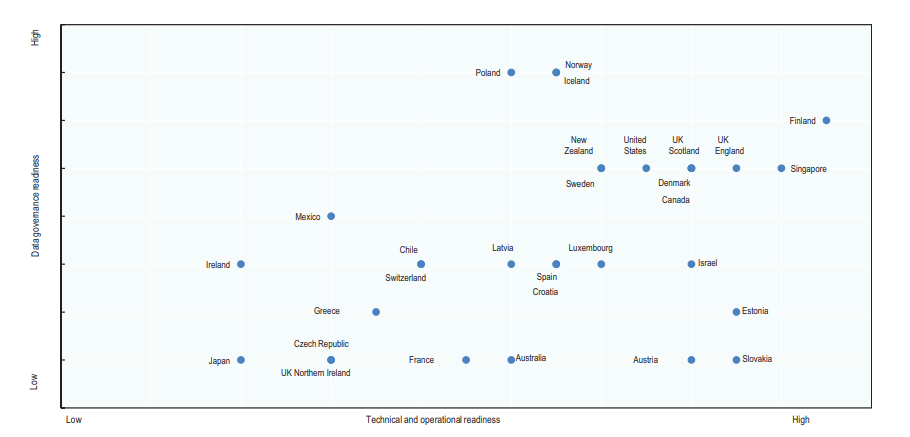
Data governance and technical/operational readiness to develop national information from eHRs in countries surveyed, in 2016.
Technical and operational readiness (horizontal axis) is the cumulative score of nine factors (electronic medical record coverage, information sharing among physicians and hospitals, a defined minimum data set, use of structured data, unique record identification, national standardization of terminology and electronic messaging, legal requirements for adoption, software vendor certification, and incentives for adoption) supporting the development of eHR systems that will contain high-quality data suitable for national monitoring and for research.
Data governance readiness (vertical axis) is the cumulative score of four factors (a national plan or priority for secondary data use; contribution of eHR data to data set creation; contribution of eHR data to monitoring and research, which are each valued at one point; and legal issues impeding data set creation) supporting the use of data held within eHR systems to fulfil national health information and research objectives.
For a full description of methods, refer to ref. 1. Modified from Oderkirk1 with permission.
- With regard to technical and operational readiness, considerable progress between 2012 and 2016 has been made in most healthcare systems. Some, but not all, countries aim to establish one country-wide eHR system with comprehensive sharing of records from multiple providers, “one-patient, one-record.” Most countries have defined minimum data sets, and the use of international standards for data elements is high for diagnoses, medicines, and laboratory and imaging results but not for surgical procedures. In general, eHRs are currently not structured to capture patient-reported outcomes or patient experiences of care; some countries reported that they were planning to capture such data. Although most OECD countries already use or are implementing a unique patient identification number in eHRs, some (large) countries remain without a unique patient identifier at the national level, notably Japan and the United States. This makes longitudinal tracking of outcomes for most patients challenging, especially in an environment, like the United States, with high patient mobility from one payer and provider to another.
- Concerns exist with the quality, completeness, and standardization of data within eHRs that limit their application to monitoring or research. Some countries report that they are mapping clinical terminologies to a consistent standard, but mapping exercises were not always successful. Several policy levers were used by some countries to encourage or enforce the adoption of advanced eHRs that meet national standards, but not all are successful, and lack of resources is a frequently cited constraint.
- With regard to key indicators of the readiness of national health data governance, results also showed a high level of variation. Most countries reported that their national plan or policy for eHR implementation included secondary uses of the data. Yet, many referred to legal constraints that limit the ability to use eHRs for monitoring or research, because eHR systems are only legally authorized to share data for medical treatment purposes (i.e., direct patient care).
- Another key health data governance factor is ensuring that the legal framework that protects patient privacy and data security within eHR systems does so in a manner that still allows data to be extracted for approved research purposes. Many of the top tier countries have specific legislation that authorizes the creation of data sets from data extracted from the eHR system. However, even in the top tier countries, legislative challenges remain.
- Last, the lack of an appropriate gatekeeper function, both procedural and institutional, prevents clarity as to who may get lawful access to query the data and for what purposes. Uncertainty in the implementation of well-intended laws and policies impedes secondary data use. Some, but not all, countries are addressing these governance issues.
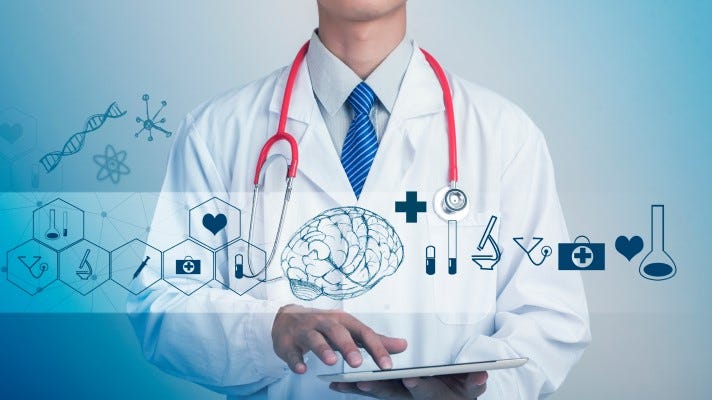
Proposals for Action
Given the challenges described above, how can we accelerate the implementation of a learning healthcare system? We believe that progress can be supported on at least three levels:
Politics, policy, and public debate
A starting point is the OECD Council Recommendation on Health Data Governance, which provides the basic building blocks of the learning healthcare system and was welcomed by all OECD Health Ministers in January 2017.24
The document sets out with a recommendation “that governments establish and implement a national health data governance framework to encourage the availability and use of personal health data to serve health-related public interest purposes while promoting the protection of privacy, personal health data and data security.”
More technically, the document “encourage[s] common data elements and formats; quality assurance; and data interoperability standards; [… as well as …] common policies and procedures that minimise barriers to sharing data for health system management, statistics, research and other health-related purposes that serve the public interest while protecting privacy and data security.”
Several other public institutions and groups, including the US Institute of Medicine, have also proposed recommendations to facilitate the conduct of health research while maintaining or strengthening the privacy protections of personal health information.20, 25
These and other initiatives are encouraging, but they may not be widely known.
The scientific community could support them in public debate by emphasizing at least two points.
First, by reinforcing the urgency that opportunities for patients are lost because science progresses faster than the “system,” impeding the development and best use of novel treatment options.
It falls on members of the scientific community and vested stakeholders to shift the debate from “Using personal health data is a risk to individuals” to “Not using personal health data is a risk to individuals, health systems and societies.”
Second, some myth busting may be called for: contrary to often-heard beliefs, patient–data protection and secondary data use are, in fact, not a tradeoff; both can be achieved at the same time.
Although there have been highly publicized security breaches of health databases in several jurisdictions, these were related to criminal hacking episodes or other incidences perpetrated for different motives or accidentally.
Appropriate data governance practices, as described in the OECD Council Recommendation26, 27 and based on best practices in OECD countries,2 allow the benefits from health data uses to be maximized and the risks to privacy and data security to be minimized.
Appropriate governance must also protect against undesirable uses of data, such as commercialization that may not be in patients’ best interests.
Emphasizing those points may help reduce barriers to implementing a learning healthcare system that stem from perceived risks to the general public or individual patients.
However, they will likely require a higher level of public engagement and more transparency than in the past.
Many healthcare systems and countries are now striving to improve usability of their own healthcare data.
Yet, transnational exchange or integration of data (or at least combining answers from local or national analyses) would greatly enhance their scientific value, not only in the obvious case of rare diseases.
Sharing of data or answers should be anticipated and built into these endeavors.
Last, it bears reminding that physicians are currently paid to take care of patients, not to generate high-quality data for future use.
If eHRs are to be considered a valuable resource to “serve health-related public interest purposes,”24 future policies will need to find ways to minimize the record-keeping burden and incentivize the data generators; this point has recently been highlighted in Portugal in the context of a new National Oncology Register.
Highlighting best practices
Highlighting best practices may help to establish a virtuous circle of mutual learning from experiences in other healthcare systems.
This approach has often been used by the OECD, to good effect.
The OECD report on readiness of eHR systems1 and the recently published OECD Council Recommendation Implementation Toolkit26 highlight remarkable steps taken by member countries.
Although progress is patchy and each of those actions and achievements address only one (small) building block of the learning healthcare system, their combination would become a game changer if they were adopted more widely.
- Public engagement about eHR data use. New Zealand held a public consultation in 2015 to develop a new Health Strategy, including a roadmap to achieve a smarter health system. Results have led to a national eHR to present existing health information in a single longitudinal view accessible to patients, health care professionals, and decision makers. It will support precision medicine, personal wellness information, and appropriate linkages to non–health data across the social sector.1, 28 Australia is consulting the public regarding research and statistical and other secondary uses of data within its national eHR system.29
- Policies to support high-quality eHR data. Adequate data quality and integrity are key, if regulatory or other decisions are to be based on eHR data. Seven countries have high population coverage of high-quality eHRs as a result of laws or regulations that require healthcare providers to adopt and use eHR systems that adhere to national standards for both clinical terminology (content) and electronic messaging (record sharing) — Austria, Denmark, Estonia, Finland, Luxembourg, Poland, and Slovakia.1 Australia has recently improved population coverage through legislation that adjusts the national eHR from an opt-in to an opt-out patient consent model.1 An opt-out consent model is also applied in the United Kingdom (England).2 Certification encourages software vendors to offer eHR systems that meet national standards in 14 countries (Australia, Canada, Croatia, Finland, France, Ireland, Luxembourg, Mexico, Singapore, Slovakia, Sweden, United Kingdom (England and Scotland), and the United States). Thirteen countries offer financial incentives to encourage healthcare providers to adopt and maintain eHRs that conform to national standards (Australia, Austria, Canada, Chile, Estonia, Finland, Israel, Norway, Singapore, Spain, United Kingdom (England and Scotland), and the United States). Nine countries further ensure that the data quality is high by auditing the clinical content (Australia, Estonia, Iceland, Israel, New Zealand, Norway, Singapore, Spain, and United Kingdom (England)).
- eHRs are part of national health statistics. In Iceland, Finland, Portugal, and the United States, the legal framework and government policy have enabled eHR data to be extracted to contribute to national health data. The Directorate of Health in Iceland builds many national data sets that rely on data extracted from the eHR system, including the Cancer Registry, Birth Registry, Registry of Contacts With Primary Health-Care Centres, Hospital Registry, Pharmaceutical Database, Communicable Disease Registry, Adverse Events Database, Database on Accidents, and the Cardio-Vascular Disease Database.1 In Finland, a Primary Health Care Registry is populated by extracting data from the national eHR system and a project is underway to enable eHR data to populate the national hospital registry.1 In the United States, the National Center for Health Statistics is developing national healthcare surveys by requesting data submissions from the eHR systems of healthcare providers and hospitals.1
- eHRs are linked with other key data for research. Denmark has national databases and registries where data are collected as extractions from eHR systems. These data are used for clinical trials, such as when testing new pharmaceutical products or for phase IV testing, and in local surveillance programs.1 The Farr Institute for Health Informatics Research in the United Kingdom is a collaboration of research centers that fosters research involving the linkage of eHR data and other research and routinely collected health data.2 Farr has work streams on data governance, capacity building, public engagement, infrastructure, and research to strengthen cross-border linkage projects within the United Kingdom. Israel is launching a new “big data” strategy that will be considering how to better govern, integrate, and benefit from large volumes of current, deidentified, personal health data from multiple sources for research, including the data within the national health information exchange.1 In Portugal, the electronic medical prescription tool is integrated with the eHR already available to manage the medication path from prescription to dispensing; this is linked to quality monitoring and improvement initiatives relating to patient records.30
- Cooperation between the private pharmaceutical sector and healthcare systems. This is another critical aspect of the learning healthcare system. The European Network of Centres for Pharmacoepidemiology and Pharmacovigilance (ENCePP) collaboration coordinated by the European Medicines Agency (EMA) has elaborated a Code of Conduct31 and other guidance32 aiming to enable public–private collaboration by directly addressing and managing potential conflict of interest issues.
Implementation
We recall the apocryphal quote: “People Who Say It Cannot Be Done Should Not Interrupt Those Who Are Doing It.” We are impressed by several successful examples of what an end-to-end learning healthcare system could do.
For example, Kaiser Permanente, a large US health maintenance organization, has capitalized on its integrated eHR system and applied Big Data analytics to improving healthcare outcomes.33
This wide array of work includes analyzing years of maternal and neonatal data to develop an algorithm to predict the likelihood of sepsis in newborns, which has helped to reduce unnecessary use of antibiotics; using data from years of acute episodes to predict the likelihood of in-hospital deterioration, transfer to intensive care, and death, to reduce the risk of deterioration and improve bedside care; and analyzing data on screening adherence and glycemic and blood pressure control among diabetic patients to support improvements in disease management.2, 26
The EMA commissioned a study to quantify the risk of metformin use in patients with renal impairment to assess the relevance of a contraindication in the label because of a concern of lactic acidosis.
The study results, based on routine registries and databases, showed a much lower risk of lactic acidosis than previously estimated,34 and the contraindication was modified in the product label.
The US Food and Drug Administration (FDA) Sentinel initiative has enabled the FDA to successfully evaluate and address drug safety concerns, using hundreds of millions of person-years of data and ≈200 million people for faster identification of safety signals related to medicines and medical devices.35
One of the reasons behind the success of Sentinel is the principle “share answers not data”; individual patient data do not leave their environment, only aggregated results do.
These and other successful applications illustrate an aspect of the learning healthcare system that we have not so far touched on: even the best real-world data do not necessarily translate into useful real-world evidence in the absence of robust algorithms and statistical methods to extract, analyze, and interpret them.
Although these are in place and broadly acceptable for several research questions, one important exception remains: conducting relative effectiveness studies on the basis of eHR data is controversial because of the inherent risk of confounding attributable to the nonrandomized nature of the intergroup comparison. On several occasions, nonrandomized real-world studies found results that differed from those in RCTs; for some studies, it was not clear to what extent study protocols were preplanned and vetted, all of which has caused skepticism about observational comparative studies.
Detailed discussion of the methodological issues of observational studies is outside the scope of this article.
Yet, we note that researchers are now starting to address the inherent limitations of this study type.36–39 Recent work by Fralick et al.40 assessed whether retrospective analysis of healthcare data could be used to confirm results from an RCT for an approved medication (the Ongoing Telmisartan Alone and in Combination With Ramipril Global Endpoint Trial (ONTARGET)). To compare the effectiveness and safety of two antihypertensive agents, the study mirrored the patient selection criteria of the RCT and used propensity score matching to address confounding by a large range of patient characteristics. While highlighting some limitations of retrospective analyses, results were almost identical to those of the RCT. The authors noted “In contrast to ONTARGET, which took approximately 7 years to complete and cost tens of millions of dollars, our study took approximately 12 weeks to implement for less than a hundredth of the cost.”
Clearly, many more pilot studies and demonstration projects will be needed to gain experience and trust before a tipping point will occur16 and relative effectiveness questions can be reliably answered — and acted on — on the basis of routine healthcare data.
A proposal for action is, therefore, to ensure adequate funding and support for these key enablers of the learning healthcare system.
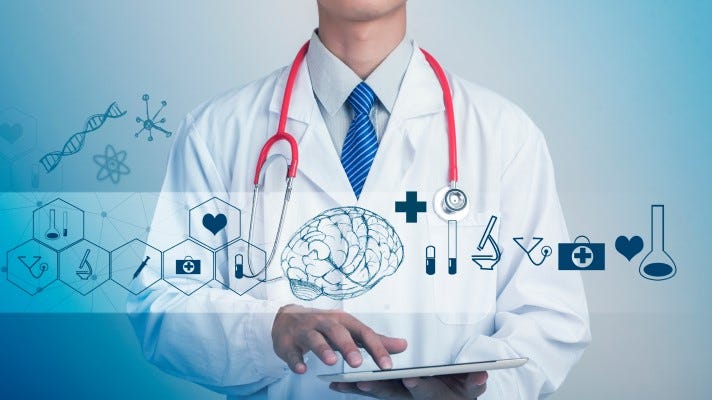
Who Stands to Benefit From the Learning Healthcare System?
We are optimistic that a concerted effort and the necessary upfront investments can be made because this is not a zero-sum game, quite the contrary; all players in the pharmaceutical ecosystem stand to gain.
Patients and the public are the primary beneficiaries, because more effective and efficient learning will lead to more beneficial treatments and more information on optimal use.
Healthcare professionals are enablers and will profit for the same reasons.
The learning healthcare system allows them to better target the right treatments to individual patients, which will mean increased effect sizes and fewer therapeutic misadventures.
We acknowledge, however, that eHR systems need to continue to evolve to improve their utility and minimize the burden on healthcare professionals.
Regulators have long recognized and leveraged the potential of routine data as a source of information about drugs, mostly in the area of safety.41, 42
Given their role for public health and ample experience in pharmacovigilance, which could be considered a focused form of the learning healthcare system, they are uniquely positioned to drive and catalyze the next steps in the evolution of learning.
The obvious benefit for regulators is better information for decision making about safety and benefits of drugs that are on the market and the ability to do rapid cycle analyses of data, avoiding highly publicized safety scares and the ensuing public distrust.
HTA bodies and payers have similar interests in a learning healthcare system: better identification of value, relative effectiveness, cost-effectiveness, more cost-effective product use, and better tools to manage expenditures, including stopping-rules and pay-for-performance schemes. Given their role in the health systems, they are key beneficiaries.
Last, the research-based industry will gain along some of the lines described for other stakeholders: better definition of an appropriate target population, better management of safety, value demonstration, and the tools for managed entry agreements. Although much of the benefit for drug developers will accrue downstream (i.e., during the on-market phase of drug product), it is expected that more information from routine health care will enable upstream drug development (e.g., by way of better quantification of unmet need; disease and biomarker prevalence; and, perhaps, even identification of novel (genomic) drug targets).
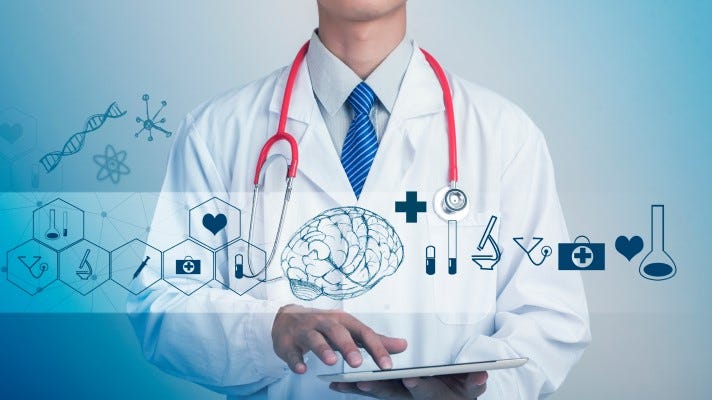
Conclusions
Perpetuating the 20th-century model of the dedicated research setting and relying (almost exclusively) on RCTs will not allow for translating the current pace of progress in the life sciences into new and better treatments for patients. It will also not enable decision makers to navigate complex scenarios in the future, be they related to investment, regulatory, financing, or patient-level decisions.
We have discussed key aspects of the future learning healthcare system as it could apply to pharmaceuticals and complement RCT information. Although the vision described above and in Figure 1 may appear ambitious, we have no doubt that the learning healthcare system is achievable, with a concerted effort on the part of key players.
There are additional caveats. We have limited the scope of this article to data that are routinely collected throughout the delivery of health care. However, even with “perfect” eHRs, other elements and data sources would be essential for a true learning health system.43 These are expected to include omics data, data from wearable sensors, clinical trials, registries, and others. To grapple with such a broader, and messier, range of elements may also require advancements in computing power, data analytics, and data security protections, to advance governance and operational readiness within and between healthcare systems.44
Many stakeholders would likely agree on the potential benefits and on what should be in place; all the basic technology is available; there are many steps taken in the right direction, yet progress remains patchy. A coordinated effort from all stakeholders and international cooperation will be required to reduce resource requirements for individual actors; reduce uncertainty and associated concerns about the adoption of new approaches and methods; and help sharing failures and success. On the upside, this will increase the speed of implementation of the learning healthcare system, to everybody’s benefit.
References and additional information:
See the original publication https://ascpt.onlinelibrary.wiley.com
About the authors & affiliations:
Hans-Georg Eichler 1,
Brigitte Bloechl-Daum 2,
Karl Broich 3,
Paul Alexander Kyrle 2,
Jillian Oderkirk 4,
Guido Rasi 1,
Rui Santos Ivo 5,
Ad Schuurman 6,
Thomas Senderovitz 7,
Luke Slawomirski 4,
Martin Wenzl 4,
Valerie Paris 4
- 1European Medicines Agency, London, UK.
- 2Medical University of Vienna, Vienna, Austria.
- 3Federal Institute for Drugs and Medical Devices, Bonn, Germany.
- 4Organisation for Economic Co-operation and Development, Paris, France.
- 5National Authority of Medicines and Health Products, Lisbon, Portugal.
- 6National Health Care Institute, Diemen, The Netherlands.
- 7Danish Medicines Agency, Copenhagen, Denmark.