Chapter 2 — Part 3: of “Transforming healthcare with AI”
EIT Health — European Institute of Innovation and Technology
March 3, 2020
This is an excerpt of the report “ Transforming healthcare with AI. Impact on the workforce and organisations”, published in 2020 by EIT Health. Survey of 175 healthcare professionals, health investors and AI start-up founders and executives
Executive Summary
by Joaquim Cardoso MSc.
Chief Editor of “The Health Revolution” Institute
AI Health Unit @ Digital Health Institute
March 25, 2022
We cannot make predictions for exactly how AI applications in healthcare will evolve, but three distinct phases of development are starting to emerge.
Near term
In the first phase — the near term — we expect AI solutions to pick up pace in areas with low-hanging fruit — i.e., a need for support in completing certain tasks, where the technology is available and where practitioners are willing to adopt the solutions.
- These are likely to be administrative, repetitive and operational tasks, which will have an impact on a wide range of healthcare practitioners, especially nurses and support staff.
- Medical specialties leading the way with AI solutions that have already received regulatory approval and are expected to continue developing AI solutions include radiology, pathology, ophthalmology
Medium term
In the second phase — the midterm — we expect to see more AI solutions embedded into clinical workflows.
- In particular, these will support the shift from hospital-based to home-based or remote healthcare, and more applications that help patients take ownership of their own health, at different levels of complexity (e.g., from noncomplex acute discharge patients to oncology patients).
- With machine learning, a patient with a cancer diagnosis can be matched with a personalised treatment or clinical trial, for example
- NLP solutions may be increasingly applied in a variety of applications, such as medical notetaking and information retrieval, both in the hospital as well as the home.
- In this second phase, we would expect to see an increase in AI-related clinical trials and in regulatory approvals of AI solutions, paving the way for the development of reimbursement models.
- Specialties likely to become more involved with AI during this second phase will probably be clinical disciplines such as oncology, cardiology or neurology, where advances are already being made.
- We would also expect the continued scaling up in diagnostics-focused specialties, focusing on the development and evaluations in the areas of diagnostics and CDS.
- Solutions empowering patients and releasing pressure on practitioners, such as virtual assistants and administrative workflow tools could also become more commonplace in this phase.
- This scaling up of AI deployment would be fuelled by a combination of technological advancements (e.g., in deep learning, NLP, connectivity etc.) and cultural change and capability building within organisations.
Long term
In the longer term, we would expect to see more AI solutions in clinical practice that drive improvements in quality of care, with approaches demonstrated in clinical trials.
- These will increasingly use longitudinal data sets, providing insights across episodes of treatment and settings of care, and incorporating new types of data from wearables and sensors.
- There will be increasing focus on improved and scaled CDS tools in a sector that has learned how to implement AI into clinical practice and has adapted its mindset, culture and skills.
- New applications will include the ability of AI-based mathematical formulas to determine the correct dosage of immunosuppressant drugs to administer to organ-transplant patients
- As data integration allows us to develop the “digital twin” of the patient — i.e., a comprehensive data record of an individual — …
Preconditions
An important precondition for AI to deliver its full promise in European healthcare will be
- the integration of broader datasets — and robust governance on the use of algorithms across partitioned datasets and organisations —
- alongside increasing organisational,
- user and patient confidence in AI and in their own ability to harness its potential to improve care.
Use cases
The main AI applications we see today are in diagnostics, clinical decision making and data management and operational management, …
but over the next five to ten years, they expect other areas to grow, such as self-care, prevention and wellness, triage and diagnosis, or applications linked to care delivery, in and outside the hospital (Exhibit 2.10).
Exhibit 2.10 — AI will become increasingly important for clinical decision making
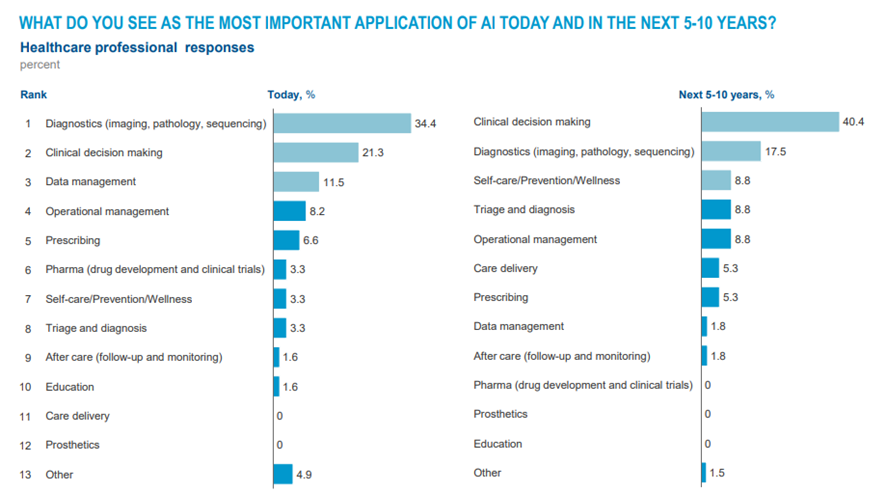
SOURCE: Survey of healthcare professionals, healthcare investors and startup executives across European countries, conducted in December 2019 and January 2020
This direction of travel is also reflected in the solutions startups are developing.
AI startups and healthcare investors agree that the US will continue to be a major growth market.
ORIGINAL PUBLICATION (long version)
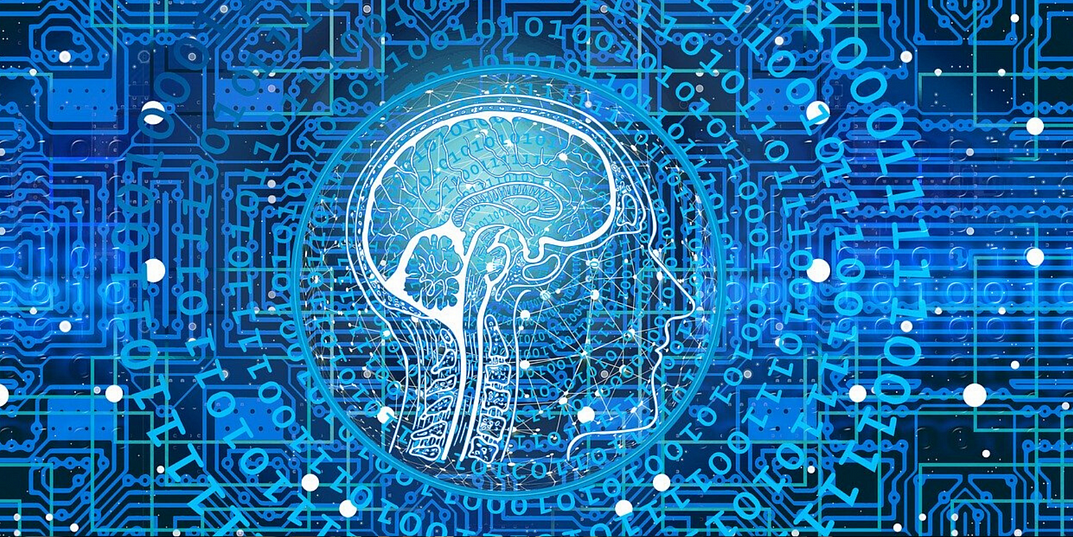
Introduction
We cannot make predictions for exactly how AI applications in healthcare will evolve, but three distinct phases of development are starting to emerge.
· Phase 1: near term
· Phase 2: midterm
· Phase 3: longer term
Phase 1: near term
In the first phase — the near term — we expect AI solutions to pick up pace in areas with low-hanging fruit — i.e., a need for support in completing certain tasks, where the technology is available and where practitioners are willing to adopt the solutions.
These are likely to be administrative, repetitive and operational tasks, which will have an impact on a wide range of healthcare practitioners, especially nurses and support staff.
Medical specialties leading the way with AI solutions that have already received regulatory approval and are expected to continue developing AI solutions include radiology, pathology, ophthalmology and dermatology.
“The low-hanging fruits of AI in healthcare will come with image recognition, triage and helping people with chronic diseases to manage independently and live well.”
Stefan Vlachos, Karolinska University Hospital
Phase 2: midterm
In the second phase — the midterm — we expect to see more AI solutions embedded into clinical workflows.
In particular, these will support the shift from hospital-based to home-based or remote healthcare, and more applications that help patients take ownership of their own health, at different levels of complexity (e.g., from noncomplex acute discharge patients to oncology patients).
As Hiyam Nadel from Massachusetts General Hospital says, “With machine learning, a patient with a cancer diagnosis can be matched with a personalised treatment or clinical trial; acute patients who urgently need to check symptoms at home appreciate such technologies; as do providers who see greater opportunities for predictive analytics.”
NLP solutions may be increasingly applied in a variety of applications, such as medical notetaking and information retrieval, both in the hospital as well as the home.
In this second phase, we would expect to see an increase in AI-related clinical trials and in regulatory approvals of AI solutions, paving the way for the development of reimbursement models.
Specialties likely to become more involved with AI during this second phase will probably be clinical disciplines such as oncology, cardiology or neurology, where advances are already being made.
We would also expect the continued scaling up in diagnostics-focused specialties, focusing on the development and evaluations in the areas of diagnostics and CDS.
Solutions empowering patients and releasing pressure on practitioners, such as virtual assistants and administrative workflow tools could also become more commonplace in this phase.
This scaling up of AI deployment would be fuelled by a combination of technological advancements (e.g., in deep learning, NLP, connectivity etc.) and cultural change and capability building within organisations.
“The most promising area of technology is how to optimise patients going home and having the right alert systems in place. Machine learning is helping define the right trigger points for alerts. We cannot serve all these people in hospital anymore.”
Monique van Dijk, Erasmus MC
Phase 3: longer term
In the longer term, we would expect to see more AI solutions in clinical practice that drive improvements in quality of care, with approaches demonstrated in clinical trials.
These will increasingly use longitudinal data sets, providing insights across episodes of treatment and settings of care, and incorporating new types of data from wearables and sensors.
There will be increasing focus on improved and scaled CDS tools in a sector that has learned how to implement AI into clinical practice and has adapted its mindset, culture and skills.
New applications will include the ability of AI-based mathematical formulas to determine the correct dosage of immunosuppressant drugs to administer to organ-transplant patients — a task previously done through a combination of guidelines and educated guesswork.
With dosing errors accounting for more than 35 percent of preventable medical errors, these would be powerful examples of significant quality and outcome improvements for patients with complex histories.[1]73
While we ultimately expect to see the development of AI solutions in all medical specialties, some — such as obstetrics — are likely to take longer.
“The future will bring further integration of data, including the relevant context of the patient, which will help to create an enriched “digital twin” of the patient that will help decide on the optimal therapy outcome for each specific patient — and maybe help to predict the risk of developing a disease or complication.”
Hans Hofstraat, Philips Chief Technology Office
As data integration allows us to develop the “digital twin” of the patient — i.e., a comprehensive data record of an individual — AI may also open new routes to delivering better, faster and more cost-effective care, many of which we may find hard to imagine at this early stages of AI’s evolution, and may have a greater focus on prevention and promoting wellness.
An important precondition for AI to deliver its full promise in European healthcare will be the integration of broader datasets — and robust governance on the use of algorithms across partitioned datasets and organisations — alongside increasing organisational, user and patient confidence in AI and in their own ability to harness its potential to improve care.
This progression from routine tasks to more complex applications was echoed in our survey of healthcare practitioners.
The main AI applications they see today are in diagnostics, clinical decision making and data management and operational management, but over the next five to ten years, they expect other areas to grow, such as self-care, prevention and wellness, triage and diagnosis, or applications linked to care delivery, in and outside the hospital (Exhibit 2.10).
This direction of travel is also reflected in the solutions startups are developing.
Exhibit 2.10 — AI will become increasingly important for clinical decision making
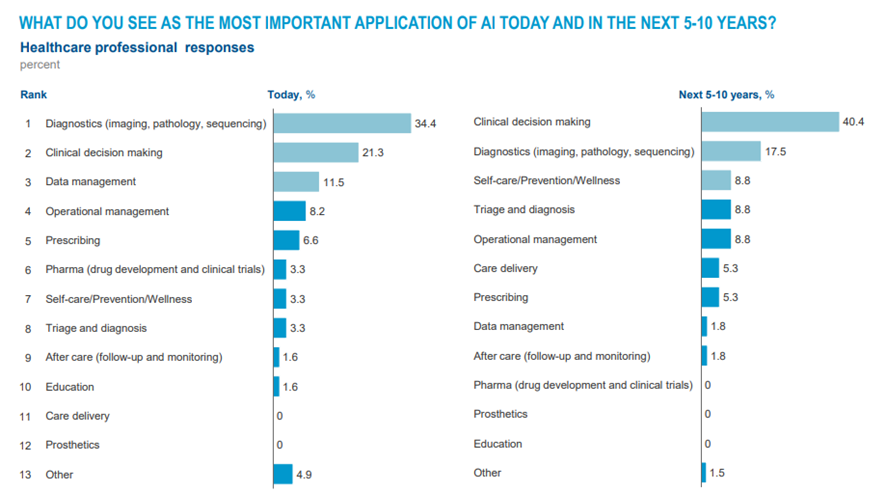
SOURCE: Survey of healthcare professionals, healthcare investors and startup executives across European countries, conducted in December 2019 and January 2020
AI startups and healthcare investors agree that the US will continue to be a major growth market.
Startups seem bullish about the prospects of the European market, seeing it on a par with the US (Exhibit 2.11), while investors see the US dominating, with China as a growing new market.
Exhibit 2.11 Startups and investors agree on the importance of the US AI healthcare market, but have differing views on Europe and China
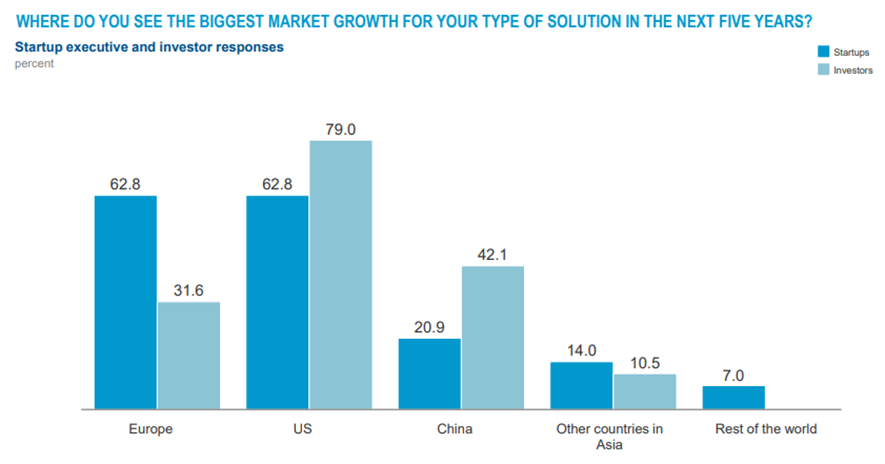
SOURCE: Survey of healthcare professionals, healthcare investors and startup executives across European countries, conducted in December 2019 and January 2020; multiple choices
All survey respondent segments were equally bullish, however, about the potential of AI in European healthcare and internationally.
Despite scepticism around the maturity or effectiveness of specific solutions available today, healthcare professionals, health investors and AI startups are increasingly excited about growing AI in European healthcare and are increasingly ready to contemplate the changes it could bring to their day-to-day life and work.
This is an excerpt of the report “ Transforming healthcare with AI. Impact on the workforce and organisations; EIT Health and McKinsey & Company, (2020). Survey of 175 healthcare professionals, health investors and AI start-up founders and executives
References
[1] Kalis, B. et al., “10 promising applications of AI in healthcare”, Harvard Business Review, May 10, 2018, https://hbr.org/2018/05/10-promising-ai-applications-in-health-care.