National Bureau Of Economic Research
Working Paper 30857
Nikhil Sahni, George Stein, Rodney Zemmel and David M. Cutler
January 2023
The paper represents the views of the authors and not any entity with which they may be affiliated. The views expressed herein are those of the authors and do not necessarily reflect the views of the National Bureau of Economic Research.
NBER working papers are circulated for discussion and comment purposes. They have not been peer-reviewed or been subject to the review by the NBER Board of Directors that accompanies official NBER publications.
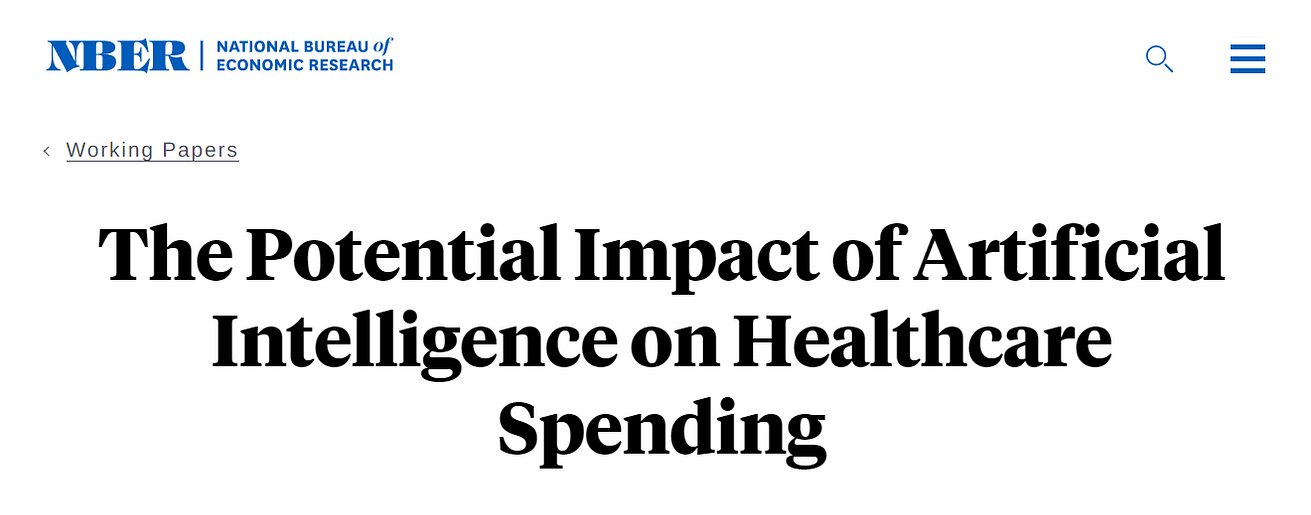
EXECUTIVE SUMMARY [by Joaquim Cardoso MSc]
ABSTRACT
- The potential of artificial intelligence (AI) to simplify existing healthcare processes and create new, more efficient ones is a major topic of discussion in the industry. Yet healthcare lags other industries in AI adoption.
- In this paper, the authors estimate that wider adoption of AI could lead to savings of 5 to 10 percent in US healthcare spending — roughly $200 billion to $360 billion annually in 2019 dollars.
- These estimates are based on specific AI-enabled use cases that employ today’s technologies, are attainable within the next five years, and would not sacrifice quality or access.
- These opportunities could also lead to non-financial benefits such as improved healthcare quality, increased access, better patient experience, and greater clinician satisfaction.
- We further present case studies and discuss how to overcome the challenges to AI deployments.
- We conclude with a review of recent market trends that may shift the AI adoption trajectory toward a more rapid pace.
In this paper, the authors estimate that wider adoption of AI could lead to savings of 5 to 10 percent in US healthcare spending — roughly $200 billion to $360 billion annually in 2019 dollars.
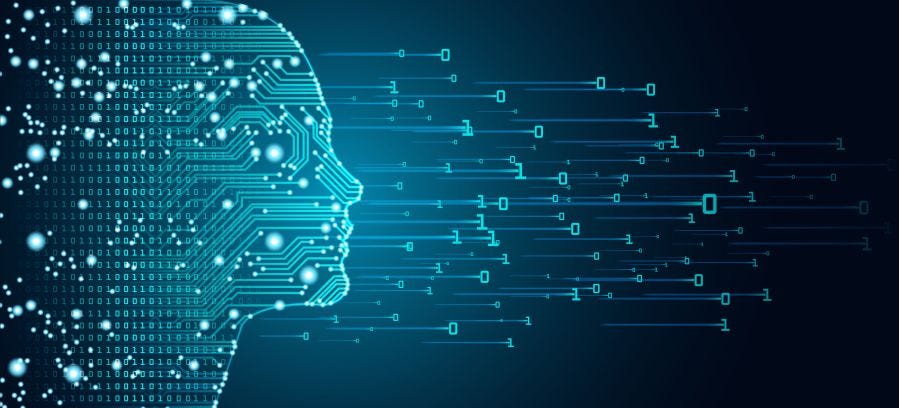
OVERVIEW:
Can artificial intelligence (AI) improve productivity in healthcare? That is a central question in the United States and the focus of this paper.
In the United States, healthcare is considered too expensive for what it delivers. Businesses are looking to manage their costs, and healthcare spending is a large and growing expense.
As healthcare spending grows in the public sector, it crowds out other governmental budget priorities.
Previous research has found that healthcare in the United States could be more productive — both costing less and delivering better care (Berwick et al., 2012[1]; Sahni et al., 2019[2]). AI is likely to be part of the solution.
The improvement in US healthcare productivity could manifest in several ways.
1.Reducing administrative costs is one example.
Administrative costs are estimated to account for nearly 25 percent of all US healthcare spending (Sahni et al., 2021)[3]; AI could reduce this burden.
2.Harnessing clinical knowledge to improve patient health is a second way.
Medical knowledge is growing so rapidly that only 6 percent of what the average new physician is taught at medical school today will be relevant in ten years (Rajkomar et al., 2019)[4].
Technology such as AI could provide valuable clinical data to the clinician at the time of diagnosis.
3.Improving clinical operations is still another example.
Operating rooms (ORs) are one of hospitals’ most critical assets.
Yet inefficient operations can result in wasted hours, leading to excessive building of space, hindering patient access, degrading the patient experience, and reducing hospitals’ financial margins.
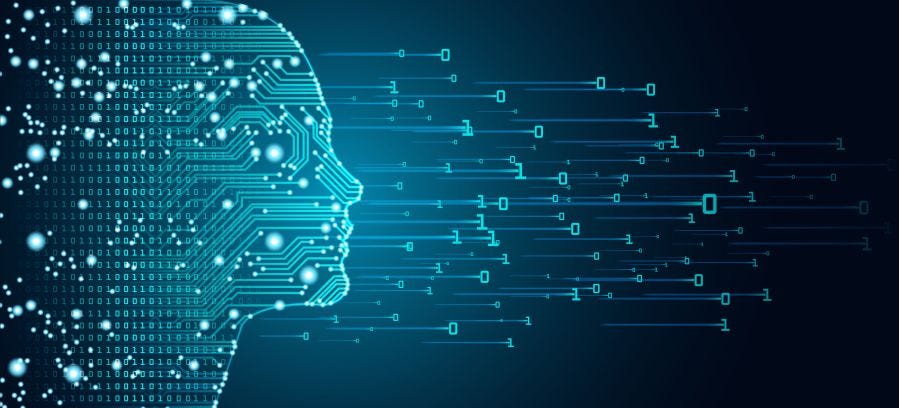
In this paper the authors focus on two questions about AI.
1.First, how much might be saved by wider adoption of AI in healthcare?
To answer this, we estimate potential savings by considering how AI might affect processes for three stakeholder groups — hospitals, physician groups, and private payers.
For each stakeholder group, we illustrate AI-enabled use cases across both medical and administrative costs and review case studies.
Using national healthcare spending data, we then scale the estimates to the entire US healthcare industry.
We find that AI adoption within the next five years using today’s technologies could result in savings of 5 to 10 percent of healthcare spending, or $200 billion to $360 billion annually in 2019 dollars, without sacrificing quality and access.[5]
We find that AI adoption within the next five years using today’s technologies could result in savings of 5 to 10 percent of healthcare spending, or $200 billion to $360 billion annually in 2019 dollars, without sacrificing quality and access.[5]
For hospitals, the savings come largely from use cases that improve clinical operations (for example, operating room optimization) and quality and safety (for example, condition deterioration management or adverse event detection).
For physician groups, the savings also mostly come from use cases that improve clinical operations (for example, capacity management) and continuity of care (for example, referral management).
For private payers, the savings come largely from use cases that improve claims management (for example, auto-adjudication or prior authorization), healthcare management (for example, tailored care management or avoidable readmissions), and provider relationship management (for example, network design or provider directory management).
While we only quantify cost savings in this paper, there are additional non-financial benefits from the adoption of AI, …
… including improved healthcare quality, increased access, better patient experience, and greater clinician satisfaction.
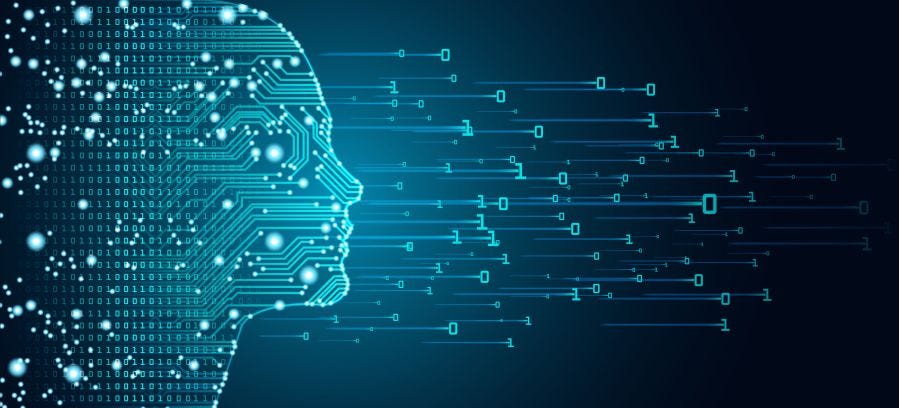
2.The magnitude of these savings raises a second question: if AI in healthcare can be so valuable, why is it not in greater use?
At the organizational level, in our experience, there are six factors for successful AI adoption.
AI’s limited uptake can be partly explained by the difficulty of addressing these factors, such as the failure to create “digital trust” with patients.
In addition, we discuss industry-level challenges such as data heterogeneity, lack of patient confidence, and misaligned incentives.
Recent market trends, such as increasing venture capital and private equity investments, may increase the rate of AI adoption in the near future.
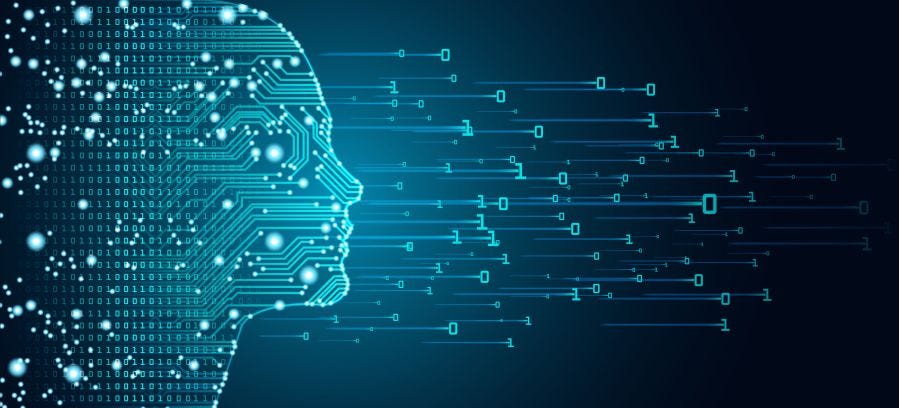
The paper is organized as follows.
- Section I outlines the scope of potential uses of AI in healthcare.
- Section II lays out how AI might be used for the three specific stakeholder groups — hospitals, physician groups, and private payers — and presents case studies as examples.
- Section III estimates the annual net savings that might result from adopting AI across all of US healthcare.
- Section IV considers the challenges to greater adoption of AI, and
- Section V discusses how market trends may change the decisions that organizations make about adopting AI.
- The final section offers concluding thoughts.
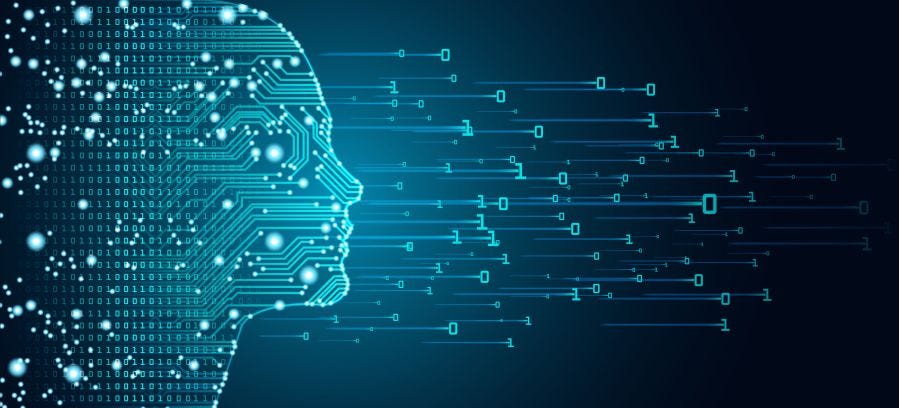
Conclusions:
The promise of AI in healthcare has been a topic of discussion in the industry for more than a decade. But its potential has not been quantified systematically, and adoption has been lacking.
The authors estimate that AI in healthcare offers a $200 billion to $360 billion annual run-rate net savings opportunity that can be achieved within the next five years using today’s technologies without sacrificing quality or access.
- These opportunities could also result in non-financial benefits such as improved healthcare quality, increased access, better patient experience, and greater clinician satisfaction.
- As our case studies highlight, both the challenges to adoption and actionable solutions are becoming better understood as more organizations pilot AI.
- Recent market trends also suggest that AI in healthcare may be at a tipping point.
Still, taking full advantage of the savings opportunity will require the deployment of many AI-enabled use cases across multiple domains.
Ongoing research and validation of these use cases is needed.
- This could include conducting randomized control trials to prove the impact of AI in clinical domains to increase confidence for broader deployment.
- However, given that these studies will likely require a long timeline, additional work focused on case studies of successful deployments may provide greater evidence for organizations to overcome internal inertia in the near term.
- Finally, an independent third party could create a central data repository of AI deployments — both successful and unsuccessful — which would allow for more robust econometric analyses to inform rapid scaling.
As other industries have shown, AI as a technology could have an outsized financial and non-financial impact in healthcare, enabling patients to receive better care at a lower cost.
The next few years will determine whether this promise becomes a reality.
INFOGRAPHIC
Figure 6. Breakdown of overall AI net savings opportunity within next five years using today’s technology without sacrificing quality or access
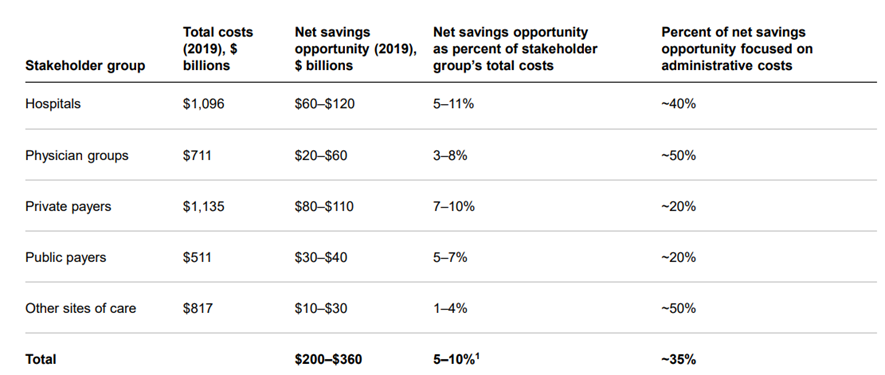
Figure 2. Hospital AI domains and example use cases
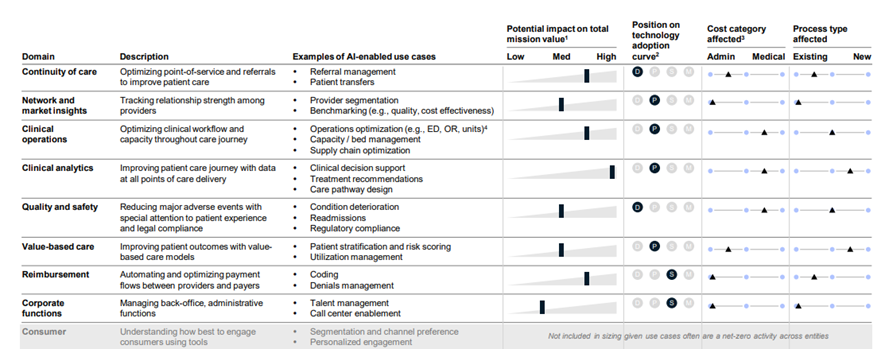
Figure 3. Physician group AI domains and example use cases
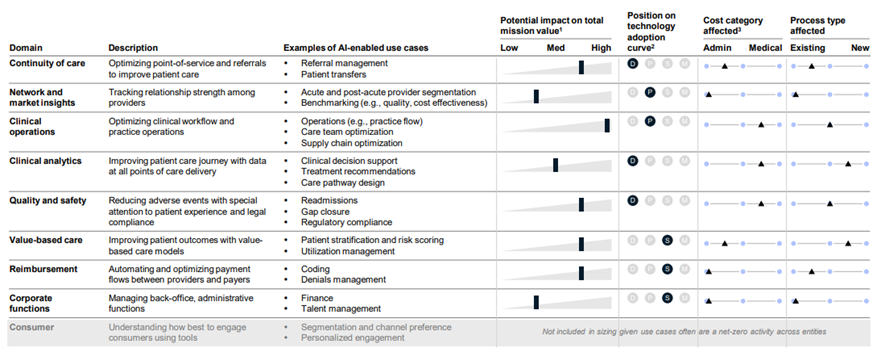
Figure 4. Private payer AI domains and example use cases
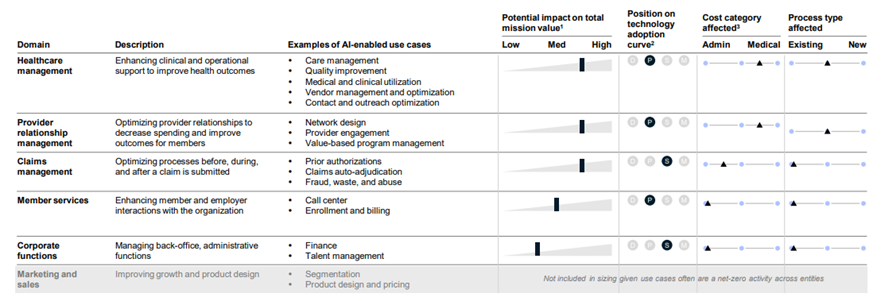