Wiley Online Library
Advanced Intelligent Systems
Mathias Egermark, Agata Blasiak, Alexandria Remus, Yoann Sapanel, Dean Ho
26 May 2022, pwcch
Abstract
- Worldwide, digital medicine technologies are being developed at a rapid rate.
- While these digital medicine technologies offer the potential to transform and revolutionize health care, many are at risk of stalling and remaining in the pilot stage, known as “pilotitis,” thus never reaching true potential.
- Therefore, overcoming “pilotitis” to increase the uptake of digital medicine technologies is a global concern.
- To date, several authors have proposed solutions to overcome various barriers owing to the “pilotitis” of digital medicine technologies, such as
– regulatory frameworks and
– patients’ data ownership;
however, many areas require further consideration. - This perspective piece identifies three barriers to the adoption and implementation of digital medicine technologies and proposes approaches for how to overcome them.
- Addressing such barriers may provide a pathway to success for digital medicine technologies to improve patient outcomes and increase the efficiency of healthcare delivery.
Structure of the publication
- Introduction
- Over-Reliance on Big Data Approaches
- Lack of Robust Clinical Evidence Base
- Lack of Reimbursement and Other Funding Channels for Digital Health Tools
- Conclusions
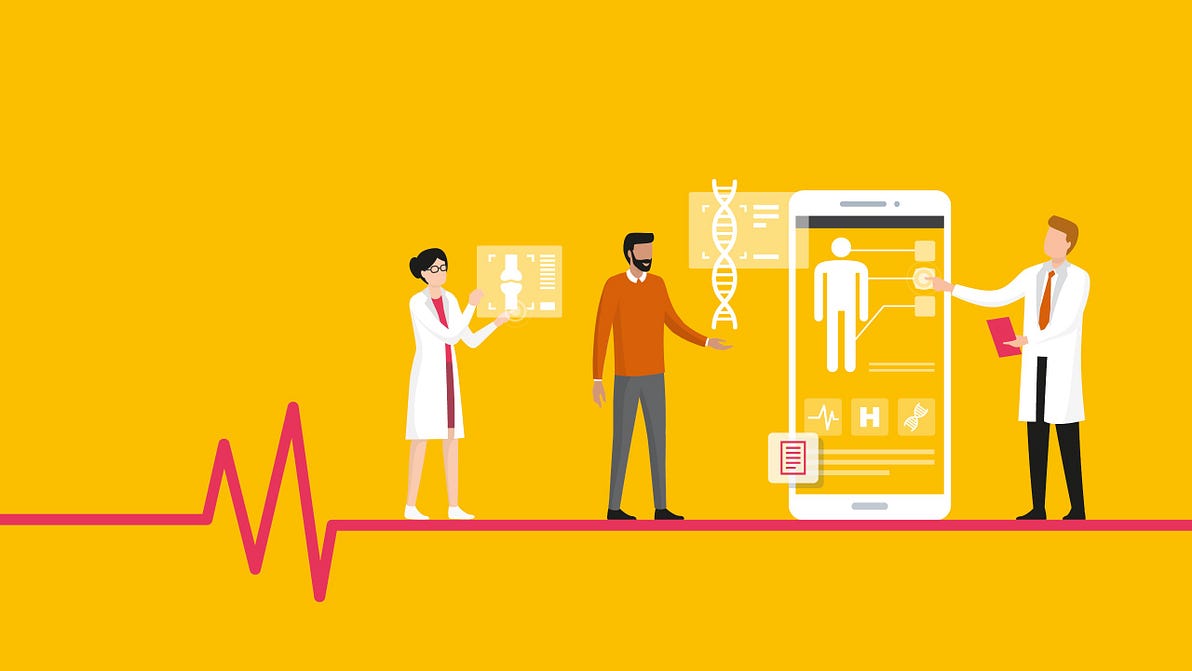
1 Introduction
The number of digital medicine technologies, a category of tools that use software and algorithmically driven products to measure or intervene to improve human health,[1] has expanded enormously in recent years, further accelerated by the need for remote care delivery during the COVID-19 pandemic, and now ranges from telemedicine to serious gaming, wearable devices, AI-supported diagnostics, and clinical decision support systems (CDSSs), among others.
Building on the experiences during the pandemic, there is a real potential for the digital transformation of health care to the benefit of patients worldwide.
However, the promising developments to date are at risk of stalling due to inherent limitations in the way digital medicine and especially medical artificial intelligence (AI) technologies are being developed and implemented today.[2]
Already in 2017, the number of digital medicine technologies on the worldwide market exceeded 318 000, with the mobile application segment growing by over 200 daily.[3]
There are widespread expectations that the surge will lead to improvement of health care globally,[4] not least evidenced by the WHO declaration on digital health,[5] and by the rapid growth of private and public investment in this field.[6, 7]
The advent of digital medicine bears special promise for low- and middle-income countries (LMIC), where applications such as telemedicine and CDSS may enable these countries to “leapfrog” from today’s often insufficient healthcare systems into more efficient, accessible, and patient-centric models,[8] similar to what has been seen in Africa’s finance sector.[9]
The level of improvement possible would correspond to the digital transformation seen in other sectors of society, such as media and retail industries, with the digitalization of processes and service models, beyond the mere digitization of traditional healthcare practices seen so far, e.g., the conversion from paper-based patient records to electronic medical records.[10]
Developments have indeed accelerated with the COVID-19 pandemic, as societies worldwide have rushed to employ digital health technology to maintain healthcare services while enforcing lockdowns and social distancing measures.[11, 12]
However, beyond COVID-induced increases in teleconsultations, examples of broad, practice-changing implementation of novel digital medicine technologies in routine clinical practice are still few, and the initial surge of AI-based technologies for diagnosing and managing COVID-19 has thus far been unable to produce tools that can be widely used in clinical practice.[13]
In spite of large expectations, the pattern of lower rates of digitalization in health care compared to other sectors[14] remains seemingly unchanged.
In fact, much of the innovation in digital health might be doomed to remain in the pilot stage, a phenomenon sometimes referred to as “pilotitis”, unless a number of limitations in today’s environment are systematically addressed.[15, 16]
Several authors have pointed out and proposed solutions to limiting factors that need to be addressed regarding, e.g., regulatory frameworks,[17] equity in patients’ access to, and control of, their medical data,[18] as well as economic and social sustainability of digital health applications.[18–21]
Here, we propose three areas that have not been discussed extensively elsewhere, but which we believe warrant increased recognition in the public discourse on how to enable wider uptake of digital tools in health care, namely:
- the current overreliance on big data approaches in CDSSs and other areas of medical AI,
- insufficient scope and scale of clinical evidence generation across digital health technologies,
- lack of economic incentives and funding structures for clinical implementation of evidence-based digital health.
Unless these factors are addressed by the digital medicine development community and health care policymakers, there is a real and prominent risk that the potential to improve health care with digital medicine technologies will become a missed opportunity, with digital medicine remaining a fragmented landscape of scattered pilot projects without the critical mass needed for substantial evidence generation, and therefore unable to support broad and sustainable implementation.
To date, digital medicine has provided the possibility to improve patient engagement in their care and the quality of care delivered.
Digital medicine also has shown the potential to improve patient access and cost-efficiency of healthcare systems for the benefit of societies worldwide, not least in LMICs, a critically important consideration which continued studies have sought to further evaluate.[22–25]
Realizing such improvements will require not just digitization, such as replacing physical patient records with Electronic Health Records, but digital transformation in the sense of changing clinical practice and healthcare provision.[10]
Examples of technologies to support such transformation include the use of CDSS tools as well as remote patient monitoring.[26–31]
Significant technological progress has been made in the field, but more efforts are needed to establish cost-effective approaches that support global adoption. Failure to successfully harness the opportunity of digital healthcare transformation risks markedly limiting access to this developing state-of-the-art in health care — particularly in LMICs, and also risks reducing patient and clinician trust in digital healthcare technologies, potentially leading to a prolonged dearth of healthcare improvements and a sector-specific “AI winter.”
This is an outcome that healthcare systems can ill afford, especially considering the high need to tackle cost containment and reduction of wasteful spending in the face of the world’s aging population, after decades of healthcare costs outstripping economic growth.[32]
Overcoming pilotitis in digital medicine should, therefore, be a key priority for societies worldwide.
In the following sections, we describe three “pathogens” that appear causative of pilotitis and propose approaches that the digital health developer community could consider deploying toward its prevention:
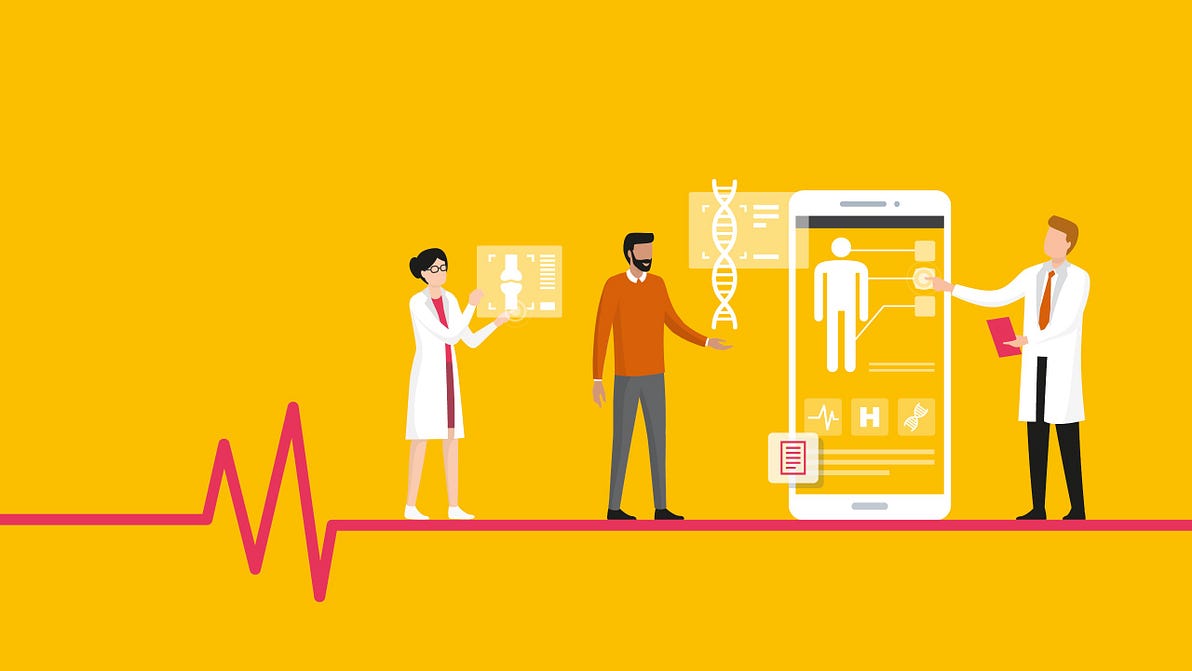
2 Over-Reliance on Big Data Approaches
It is time to consider N-of-1 approaches in CDSSs based on AI that do not require the use of big data.
2.1 Challenge
AI in medicine to date has to a large extent been based on machine learning (ML) approaches using big datasets, successfully applied, for example, in the development of image recognition used in radiology and digital pathology.[2, 33, 34]
In principle, the more comprehensive the data set, the more tailored information it can yield to personalize patient treatment.
However, when applying AI to clinical decision support, there are large challenges associated with the use of big data.
First and foremost, the amount of data points required for the algorithmic training inevitably requires reliance on real-world data (RWD).
This approach can encounter practical limitations such as siloed data structures and a lack of interoperability and common data standards across electronic medical record systems.
Similarly, there is no one, worldwide accepted standard in the data structure collected directly by the patients.
Other challenges include patient privacy concerns and medico-legal access barriers to data, lack of standardization of clinical terminology, and high variability in the quality of the clinical documentation itself, as well as fundamental methodological problems such as the risk of reproducing racial, social, and demographic biases present in the underlying datasets.
Also, big data approaches based on large populations face the inherent challenge of lacking longitudinal intra-patient data series, and their feasibility in clinical practice is often limited by the large efforts required for data collection and curation.
Other challenges include the risk of incorporation bias and overfitting, as well as insufficient study size and handling of missing data.[35, 36]
While there has been significant progress in developing ML models that can account for some of the common issues with medical datasets, this approach can only provide limited improvements and there is a lack of broadly accepted standards that can guide the selection of the most appropriate model to use.
Finally, it has been broadly recognized that for digital health tools to be the most advantageous to society as a whole, they should be deployed in ways that address widespread and growing concerns about data privacy and they need to be equitable, accessible, and inclusive.[37]
However, if no alternatives are offered to big-data-based digital health tools, patients with rare conditions may be underserved in the digital health revolution as the generation of sufficiently large datasets for reliable ML may take too long or even be practically impossible.
In the absence of overcoming the requirement for big data, the field of digital medicine may deepen the divide instead of closing the gap for patients with rare diseases.
2.2 Proposed Approaches
As ML developers in areas outside medicine are increasingly noticing, the next step in the development of clinically deployable AI will require a more data-centric approach than the hitherto dominating model-centric approach, where lack of data quality is sought to be compensated for by increased volumes of data.[38, 39]
The capability of modern ML models to process complex data and generate actionable decision support should be increasingly applied to developing N-of-1 medicine — personalized medicine, where only a patient’s own data is used as the basis for clinical decision support, for example, in the optimization of treatment regimens.[40, 41]
The capability of modern ML models to process complex data and generate actionable decision support should be increasingly applied to developing N-of-1 medicine — personalized medicine, where only a patient’s own data is used as the basis for clinical decision support, for example, in the optimization of treatment regimens
This approach could be advantageous in developing patient-specific therapies, where the drug and the doses are selected specifically for the given patient. One example of such an area is adaptive and evolutionary chemotherapy.[42, 43]
Another example of a clinically validated, AI-derived system is phenotypic personalized medicine (PPM), where prospectively generated, pre-designed, small, but high-quality datasets are used to personalize drug combinations and dose selection.[44–50]
In one use case, CURATE.AI, an AI-derived platform from the PPM family, was used to prospectively optimize chemotherapy doses taken by a patient with advanced cancer (metastatic castration-resistant prostate cancer), which led to halting the disease progression at the doses lower than the maximum tolerated doses (MTD) — a standard of care in cancer treatment. Importantly, only the treated patient data were used by CURATE.AI to recommend doses for that patient.[46] This approach can be extended to nonpharmacological interventions like physical and cognitive training,[51] currently undergoing testing in clinical trials (ClinicalTrials.gov registration numbers: NCT04357691 and NCT0484935).
These strategies illustrate that data-driven patient intervention may need to rely less on the amount of data needed, but rather focus more on how the data is acquired.
Using only carefully selected, prospectively collected data points from the individual patient can avoid the potential pitfalls of big-data-dependent approaches.
Addressing the need to facilitate data acquisition and curation, with the advent of wearables and digitally enabled self-reporting tools (e.g., smartphone-based apps), data acquisition and curation have become greatly simplified and automated.
These approaches need to be deployed more extensively to enable the creation of sufficient datasets for model generation.
Importantly, these technologies will also be important to avoid inconsistencies in data collection from untrained individuals.
Additionally, to address the growing concerns surrounding data privacy related to wearables and other digital tools, increased emphasis and effort need to be placed on developing reliable infrastructures that enable patients to collect, control, and share their individual health data.[52]
As a result, many healthcare systems will need to state patients’ right to control of their data more clearly and explicitly, and greatly facilitate patients’ access to their own data, in all of its forms, including lab reports, imaging results, and electronic health records.
This will be required not only from a patients’ rights point-of-view, but also to enable the development of N-of-1 CDSS and ultimately patient-controlled N-of-1 applications in clinical practice.
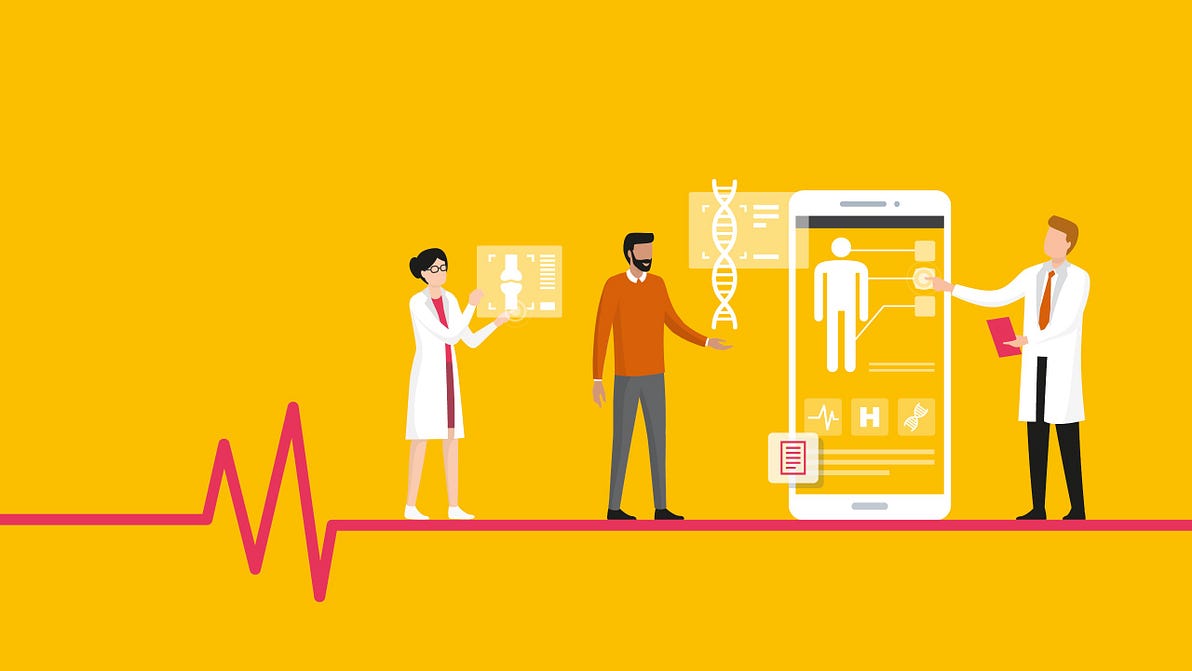
3 Lack of Robust Clinical Evidence Base
3.1 Challenge
Recent commentary suggests that there is distrust in digital health applications in general[53] and in medical AI in particular[54, 55] across patients, physicians, and healthcare systems.
The reasons for distrust are many and often well-founded in a situation where an emerging technology is still often immature and healthcare systems’ infrastructure is not yet fully in place.
Many proposals have been put forward to increase trust and improve adoption, including addressing stakeholder involvement, greater usability focus, etc.[53–55]
Based on interviews with clinicians and a review of the available landscape of published evidence, we conclude that another key underlying factor behind low adoption of digital health solutions is lack of robust clinical evidence of their efficacy and safety.
Recent findings in clinical practice strongly reinforce this learning.[13, 56]
Unlike the situation for pharmaceutical drugs, in vitro diagnostics (IVD), and medical devices, many digital medicine technologies, particularly in the mobile applications and even software-as-medical device (SaMD) segments (classified in the USA as medical device software (MDSW) under the EU’s Medical Device Regulation (MDR)) do not have sufficient clinical evidence for efficacy and safety to make clinicians and healthcare organizations confident in implementing them in clinical practice.
Evidence is often only developed for technical validity, with insufficient proof of validated usability and only rarely is clinical and health economic evidence of benefit generated.[57]
Also, the issue of reporting bias, which is recognized as pervasive in clinical development in general, is likely common also in clinical development of digital medicine, leading to under-reporting of negative evaluations and consequently holding back progress as developers recreate previously unsuccessful models, contributing to projects stalling in the pilot phase.[58–60]
3.2 Proposed Approaches
3.2.1 Mindset Shift from Digital Product Development to Clinical Management System Development
To realize the promise of digital transformation of health care, a fundamental mindset shift is needed.
More specifically, this will mean a shift from:
- a singular emphasis on the “move-fast-and-break-things” approach of rapid iterative on-market software development, to an approach, where time and resource are also devoted to robust clinical evidence generation as supported by emerging regulatory frameworks (see point (Section 3.2.2) below), and
- from a siloed approach in product development and data accumulation, to an open, collaborative, solutions development approach characterized by interoperability.
Many digital health technologies can be shown to improve clinical practice in single-site study settings but will require open connectivity with a multitude of different systems to be able to scale into clinical practice.
For example, an algorithm for personalized optimization of drug dosing for individual patients might require data to be pulled from multiple sources such as home-based patient wearables, and its output may need to be transmitted directly into hospital EMRs or electronic prescription systems for clinician sign-off.
Only when these interfaces are safely and effectively integrated will such an algorithm be likely to be time-saving and convenient enough for healthcare staff and patients to be useful and economically efficient in clinical practice.
Developers of individual health tech products, therefore, need to form collaborations across disciplines spanning the entire healthcare workflow to create integrated end-to-end solutions that solve medical problems, and provide solid evidence for the economic benefits (cost-savings and/or cost-effectiveness) of the overall solution as a clinical management system.
3.2.2 Investment in Rigorous Clinical Evidence Generation
Public and private investors as well as developers in startups, academia, and industry, need to realize that digital medicine technologies will require levels of evidence of efficacy and safety closer to the standards used for pharmaceutical drugs than to consumer technology apps, and be prepared to invest accordingly in clinical development programs based on sufficiently powered randomized controlled trials (RCTs).
Regulatory requirements may drive increasing demands for evidence of efficacy and safety for higher risk digital applications, but adoption by healthcare providers will require higher evidence levels across low to high-risk classification levels, as seen for example in recent experiences of the implementation of prescribable low-risk digital health applications in Germany.[61, 62]
To address the issue of time and cost of large-scale clinical development, digital medicine developers are advised to take on rigorously validated biomarkers as surrogate endpoints to enable early readouts and cost-efficient study designs, e.g., by the use of NT-proBNP as the gold standard biomarker for heart failure[63] hemoglobinA1c for diabetes mellitus, blood pressure for cardiovascular events; or HIV-RNA for HIV drug efficacy.[64]
Although seemingly straightforward in theory, designing clinical study programs with biomarkers as a core element of efficacy and safety outcomes will require that developers of digital medicine build up extensive knowledge in the fields of diagnostics expertise, e.g., IVD, electrophysiology, and radiology, consequently take on the roles and responsibilities of medical technology manufacturers, and use established validation frameworks to ensure quality and reliability of products.[64–67]
Innovative approaches such as “clinical simulations” should be considered as methods to de-risk and speed up clinical evidence generation, especially in the earlier stage of development before investment in full-scale RCTs.[68]
The field of digital medicine has hitherto emphasized the development of new tools, models, and algorithms; we propose that, going forward, academic researchers and developers put increasing effort into development of innovative new approaches to clinical trial design and robust evidence generation.
3.2.3 Build Consensus Frameworks and International Study Collaborations to Counter Not-Invented-Here Syndrome
The high study population sizes and costs associated with rigorous clinical evidence generation will require more multi-site, multi-national clinical trials. Such measures will also be needed to address another major hurdle against clinical uptake of digital health: the not-invented-here syndrome.[69]
Although historically associated primarily with attitudes toward product development teams in industrial R&D, the not-invented-here syndrome can be observed in many settings, not least among clinical practitioners.[70, 71]
Since hurdles to entry are fairly low in the technical development of digital health applications, the number of locally developed solutions is high, as indicated by the continuous increase of products in this space.[3]
We observe how healthcare organizers, clinicians, and other stakeholders often express a strong preference for locally developed products, e.g., due to stated intentions to support local developers and perceptions that local needs are “unique.”
However, with the clinical development investments needed to turn digital health into evidence-based medical practice, consolidation will be necessary.
To ensure trust and perceived local relevance in study results for digital health applications, multinational study site allocation will be needed, as well as third-party verification, validation, and accreditation of results, similar to what is done by regulatory bodies for pharmaceuticals today.
Several nongovernmental bodies already provide such services already[57] and provide a base to build on.
However, a substantial enhancement in the capacity and scope of such bodies will be needed to provide broad and deep international recognition, and instill confidence in findings, and implementation of recommendations by the worldwide clinical practitioner community. In this sense, global governing bodies, such as the WHO and UN, should provide the foundation for such initiatives, but the lead must be driven by societies and organizations of multinational stakeholders and experts (i.e., Digital Medicine Society (DiMe), Digital Therapeutics Alliance (DTA), Decentralized Trials & Research Alliance (DTRA), IMPACT, etc.) for widespread implementation and adoption of digital medicine technologies to occur.
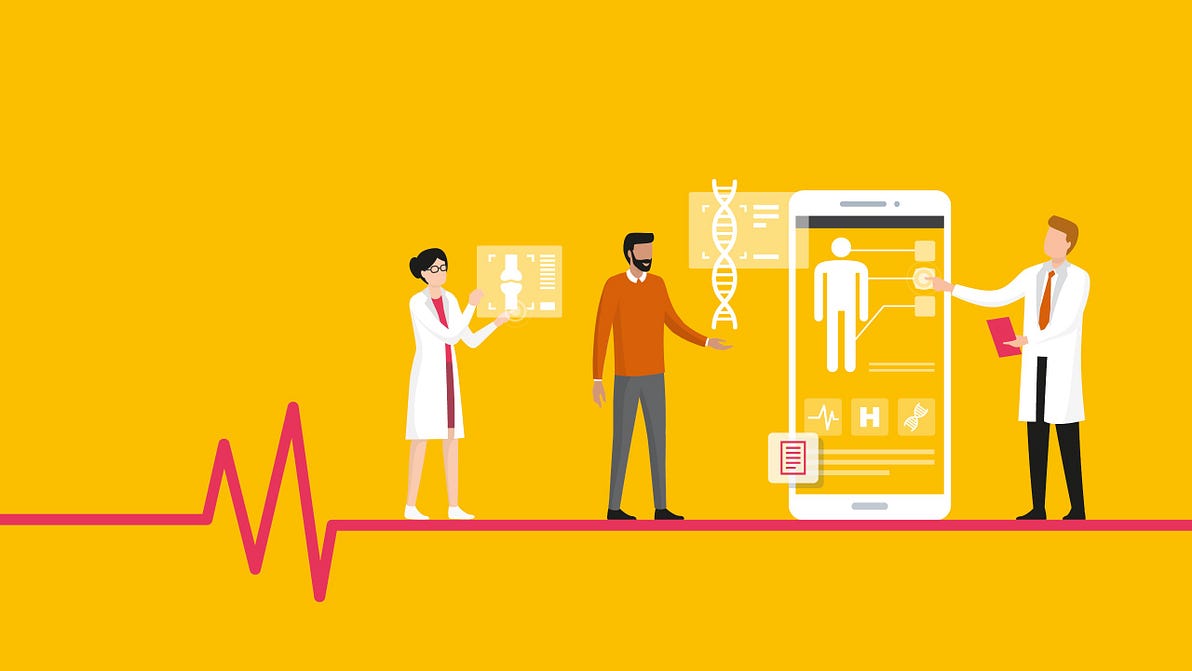
4 Lack of Reimbursement and Other Funding Channels for Digital Health Tools
It is time to start generating substantial proof of the health and economic benefits of AI in digital medicine.
This is needed to provide a basis for payers to construct reimbursement systems that incentivize investment in medical AI by healthcare providers, and investment in solid clinical evidence generation by developers.
In other words, solving this funding challenge will be a prerequisite to building a robust clinical evidence base in digital medicine.
4.1 Challenge
Digital medicine technologies have the potential to add value by enabling better coordination between healthcare providers and different levels of care, and by reducing the need for patient visits to hospitals and clinics.
Therefore, healthcare insurance based on fee-for-service reimbursement, which rewards visits to doctors and clinics may disincentivize investment in digital health technologies.
Furthermore, even though digital health tools may be cost-saving over time, initial investments needed to implement digital health solutions are often high, e.g, in procuring wearable devices for patients, and incurring costs for integrating novel software into hospitals’ legacy IT systems.
4.2 Proposed Approaches
Funding models are needed to enable digital health transitions, which means that not only do insurance-based healthcare financing systems need to consider reimbursement for digital health technologies, but tax-financed, single-payer healthcare financing systems need to consider allocating dedicated funding for digital transformation.
To motivate the substantial investments needed, developers of novel digital health tools need to provide proof of economic benefit in the form of cost savings or cost-effective improvements in care quality and patient outcomes.
This will require not only more openness in enabling interoperability and data sharing capabilities in digital product design, but a new level of willingness to invest in collaborative health economic data generation from developers and their financial backers.
Such investments, however, will also require payers to make clear upfront that reimbursement and investment funds will be made available for digital health applications for which cost-effective clinical impact or healthcare cost-savings are robustly demonstrated.
Hence, it will be increasingly important for payer organizations and health technology assessment (HTA) bodies worldwide to increase efforts to define funding models for digital health applications and develop value-based reimbursement schemes that will sufficiently reward innovation and health economic evidence generation.
Experience from the pharmaceutical sector clearly shows how economic incentives can generate a massive innovative effort and private investment into R&D.
A similar development could be possible for digital health and medicine, where investments could help bring innovations to market that not only improve patient health, but also enable cost-containment and savings in health care, e.g., by enabling more efficient care delivery, home-based care through telemedicine applications, more effective use of available medicines through individualized dose-optimization, etc.
A number of novel funding mechanisms have been proposed and even piloted in certain countries.
Examples include early health technology assessments[72] and bundled payments based on performance metrics in so-called value-based healthcare models.[73, 74]
Transitioning these mechanisms from theory and piloting toward standard policy will be essential.
A leading example of such a transition is the recent development of healthcare digitization in Germany.
Driven by strong political interest and initiative, within a few years Germany has transitioned from having one of the lowest digitization levels among developed countries to instead positioning itself at the frontier of digital health innovation.[75]
With the Digital Healthcare Act (Digitale-Versorgung-Gesetz or “DVG”) adopted by parliament in November 2019, and the Digital Health Applications Ordinance (Digitale Gesundheitsanwendungen-Verordnung — DiGAV) enacted in April 2020, Germany introduced a national reimbursement framework which makes digital health applications available on prescription and reimbursed through public health insurance providers, contingent on demonstration of value.[76–79]
The learnings from the first years of DiGA implementation should be considered by more countries.
As can be seen in Table 1, there are many encouraging examples of digital medical products being reimbursed by payers, although outside of Germany these examples have mainly been seen in the private sector, with slow uptake in the public health sector.
These observations serve as encouragement that reimbursable status is indeed possible to achieve for digital medicine applications, but more effort is needed to make these technologies widely available to the general population.
Table 1. Examples of initial funding and reimbursement successes for digital health[82–88]
See the original publication.
Taking stock of the learnings from the initial experience in Germany it seems clear that most physicians require more evidence, education, and information regarding digital applications before prescribing them than what is available today.[61, 62]
It will be crucial to increase clarity upfront on payer organizations’ requirements for evidence of clinical effectiveness and safety as well as health economic benefits, to incentivize digital health companies and their backers to invest in cross-technology collaborations and health economic data generation.
The community of digital medicine developers will need to engage more proactively with payers, to highlight the need for clarity on reimbursement and funding policies for digital medicine.
Developers should also engage in dialogue with payer organizations earlier in the development process than today, to make use of the increasing openness to dialogue among HTA agencies.
Ultimately, digital medicine technologies must demonstrate their value and cost-effectivenes (and thus returns) to payer organizations, such as Government agencies, commercial insurance companies, and employers.
Developers are therefore well-advised to seek early health technology assessment (HTA) for development projects,[72, 80] design studies as “strategy trials,” where digital medicine products are tested as part of an overall patient management approach compared to standard of care rather than as an isolated intervention, and ensure that studies capture outcome measures that allow assessment not only of clinical efficacy but also health economic aspects such as cost-effectiveness of the tested strategies.
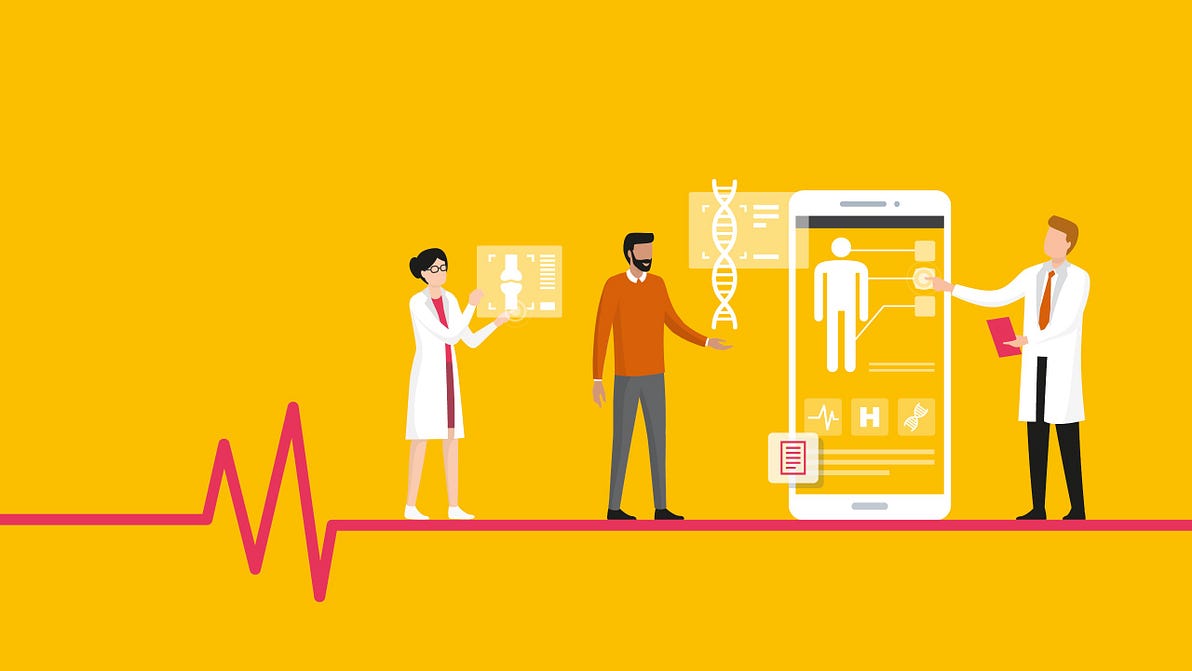
5 Conclusions
Digital transformation of health care bears the promise of dramatically improving health access and outcomes for populations worldwide compared to traditional healthcare approaches.
The hurdles are manifold, as is to be expected when a transformation of health care rather than an incremental technology implementation is afoot, and previous authors have prudently pointed out the need to address critical topics such as the requirement for new regulatory frameworks for digital medical technology,[17] measures to ensure equitable access and data privacy,[18] and systematic approaches guided by insights from implementation science, complexity studies, and social science to spread and scale up the use of new technologies in clinical practice.[81]
However, in addition to what previous authors have pointed out, we find that several systemic aspects of how digital medicine technologies are developed and financed need to be given more attention and be more fundamentally addressed to avoid such technologies getting caught in the “valley of death” between proof-of-concept and clinical adoption, languishing in the pilot phase.
Therefore, we propose that such changes need to take place in the following three ways:
- Increased application of N-of-1 approaches to overcome the ever-increasingly obvious limitations of big data approaches in medicine.
- The paradigm shift from a focus on technically focused product development to the development of clinical management systems with significant, long-term investment in robust, prospective clinical and health economic evidence generation.
- Clear commitments and early health technology assessment guidance from payers in general, and public payers, in particular, regarding funding for digital medicine applications in clinical practice, to incentivize investment in clinical and health-economic evidence generation.
Collectively, these three proposed changes are interconnected, and each must be addressed, either one by one or in parallel, to enable the digital transformation that needs to take place for societies worldwide to successfully overcome the ever-increasing growth in need, demand, and cost of health care.
About the authors & Biographies
- Mathias Egermark is the inaugural Executive Visiting Fellow at the National University of Singapore, currently engaged in the clinical development of digital platforms for therapy optimization at The N.1 Institute for Health and The Institute for Digital Medicine (WisDM). He is a clinical practitioner by training, life science industry executive by practice, and an employee of Roche Diagnostics.
- Agata Blasiak is Head of Digital Health Innovation and Senior Research Fellow affiliated with The N.1 Institute of Health (N.1), The Institute for Digital Medicine (WisDM), and the Department of Biomedical Engineering at the National University of Singapore. She aims to develop new digital health and medtech solutions ready for implementation and scale-up by design.
- Alexandria Remus is a research fellow at the N.1 Institute for Health and the Department of Biomedical Engineering at the National University of Singapore. Her research pertains to the development and implementation of digital therapeutics, wearable technologies, and digital health applications to optimize human performance across the lifespan.
- Yoann Sapanel is Head of Health Innovation at The Institute for Digital Medicine (WisDM) at the National University of Singapore. He aims to foster and accelerate cross-industry, public–private collaborations to validate, implement, and scale first-of-its-kind pharmacological and digital interventional technology into the clinic.
- Dean Ho is currently Provost’s Chair Professor, Director of The N.1 Institute for Health (N.1), Director of The Institute for Digital Medicine (WisDM), and Head of the Department of Biomedical Engineering at the National University of Singapore. His research pertains to the use of digital medicine platforms to optimize N-of-1 patient therapy and drug development.
References and additional information
See the original publication