The major barrier to AI in Health Care is not AI, is data
Excerpt of Chapter 4.3 of the report: “Transforming healthcare with AI”
EIT Health — European Institute of Innovation and Technology
March 3, 2020
This is an excerpt of the report “ Transforming healthcare with AI. Impact on the workforce and organisations”, published in 2020 by EIT Health. Survey of 175 healthcare professionals, health investors and AI start-up founders and executives
Executive Summary
by Joaquim Cardoso MSc.
Digital Health Platform — Institute
AI Powered Health Care Unit
March 28, 2022
What are the prerequisites for AI?
- A key first step for the effective introduction of AI in healthcare is adopting digitised and automated processes.
- Systematising digital data collection, linking datasets between systems and ensuring data cleansing is performed routinely, are all prerequisites for introducing AI solutions.
- However, there is often a discrepancy between the aspiration for complete clean data, and the reality that most healthcare organisations deal with.
What are the issues, particularly in Europe? What is the situation of digitalisation in Europe?
When the survey participants were asked what the major barriers were to introducing or scaling AI in healthcare, data cropped up again and again … reflecting concerns around: (1) data quality and security, the (2) interoperability of systems and (3) the governance and ownership around data
- Healthcare is among the least digitised sectors in Europe …
- One of the biggest barriers for AI in healthcare is that while enough data are often available across a national system, they are not connected or interoperable.
- Implementing electronic data collection and automating operational and clinical tasks is still in development in many EU countries and will require significant changes to workflow practices and a shift in workforce skills.
What are the challenges for digitising health and collecting the right data?
- This lack of digital records and processes poses significant challenges for adopting AI solutions not just because data are needed to feed the algorithms, but also because staff frustration about the inadequacies of existing digital systems can lead to a reluctance to adopt more innovative and futuristic AI solutions.
- Either way, it is critical to get the basic digitisation of systems and data and the development of digital skills in place before launching AI efforts.
Ensuring strong data governance within healthcare organisations
- Data Security — Healthcare providers are used to dealing with vast amounts of clinical data, much of which is highly sensitive and confidential and they have well-tested policies for managing data security; for example asking patients to consent to their data being shared, including for the purposes of clinical research.
- However, as more healthcare is delivered using new digital technologies, public concerns about how healthcare data are being used have grown.
- Healthcare organisations should have robust and compliant data-sharing policies, which support the improvements in care that AI offers while providing the right safeguards. Healthcare providers need to communicate clearly with patients as to how their data will be used.
- There are well-developed data security laws in Europe, the US, Canada and other parts of the world, so one could argue that patients can be reassured about their data security.
What is the situation of data governance in Europe?
- Both healthcare as a sector and Europe as a region have significant advantages in terms of data governance. Of course these policies need to be updated as changes occur.
- Processes around data anonymisation also need to be updated to be made fit-for-purpose for the fast-evolving healthcare sector (but not restrict access to data)
- Clear guidelines within healthcare organisations and systems on the required levels of pseudonymisation or anonymisation, linking and de-linking of data records needed for particular applications would help both AI developers and clinicians looking to implement their solutions — in particular if they helped simplify and streamline the process of anonymisation.
- The process of anonymisation data, in the absence of clear guidelines could have significant cost implications and render diagnostic algorithms prohibitively expensive.
Data interoperability and building bigger datasets
- Policy makers, funding bodies and nonprofit organisations need to support efforts to sufficiently anonymise or link data and, where sensible, build federated networks of datasets that are interoperable and can be queried as needed (with the right security and governance protocols), or help create scaled-up databases that can be accessed only by stakeholders with the appropriate safeguards.
- Recent examples of such data sources include The European Nucleotide Archive; the Danish Reference Genome Project or the 100,000 Genomes Project by Genomics England.
- The Israeli government’s recent initiative to combine national digital medical records in a unified system is another example of centralised efforts to spearhead and support the development of national datasets for healthcare, including for applications of AI. [4]118
However, we must acknowledge that healthcare data can be notoriously complex.
- They are often gathered by proprietary software and compiled in siloed databases that are part of largely incompatible systems — at times, even within the same hospital.
- This makes a large amount of the data in existence virtually useless in their current state.
- This is a key consideration for healthcare providers, who need to develop policies to promote interoperability within their own proprietary systems, as well as with those of external suppliers and other providers.
The need for data infrastructure and data standards
In order to make the most of the rich data available, healthcare systems need an interconnected data infrastructure, as well as standards for data formats, be they Fast Interoperability Resources (FHIR) or open EHRs, data exchange and semantic interoperability of medical terminologies to reduce noise in the data.[5]119
- Such efforts have been successful in the past, for example in gene- and protein-naming conventions, in data-format standards for next-generation DNA sequencing data and the International Classification of Diseases codes.[6]120
- They have often developed organically, proposed by groups of researchers or international committees of larger organisations and could offer an example of a potential path for similar efforts on AI.
What needs to change to encourage the introduction and scaling of AI in healthcare?
It is critical to get the basic digitisation of systems and data in place before embarking on AI deployments — not least because the frustrations staff have with basic digitisation could spill over to the wider introduction of AI.
Healthcare organisations should have robust and compliant data-sharing policies that support the improvements in care that AI offers while providing the right safeguards in a cost-efficient way.
The final data challenge is getting datasets to talk to each other.
- Policy makers, funding bodies and nonprofit organisations need to support efforts to sufficiently anonymise and link data and, where sensible, to build databases that can be accessed by stakeholders with the appropriate safeguards.
- In order to make the most of the rich data that is available, healthcare systems need an interconnected data infrastructure.
- This is an area where Europe, as mentioned, could have a significant advantage, … in … providing a prototype for the creation of centres of excellence for AI in healthcare.
Exhibit 4.2 — Data issues dominate the perceived barriers to growing AI in healthcare
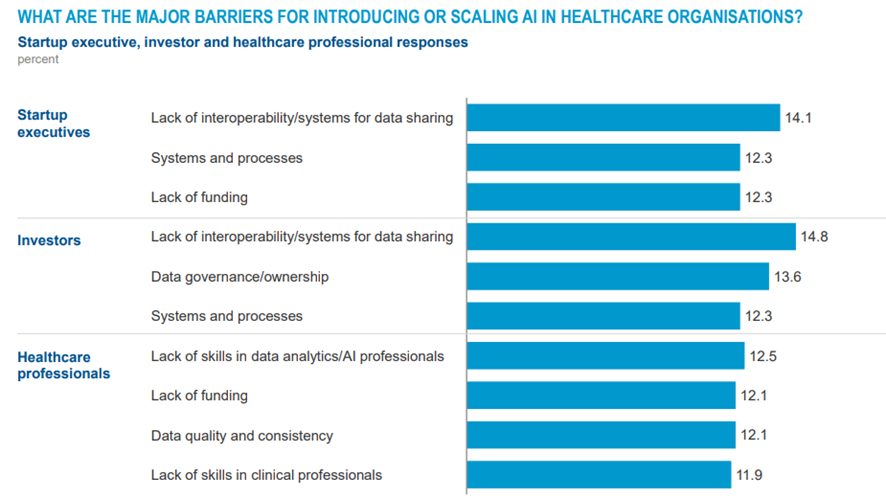
EXCERPTED VERSION
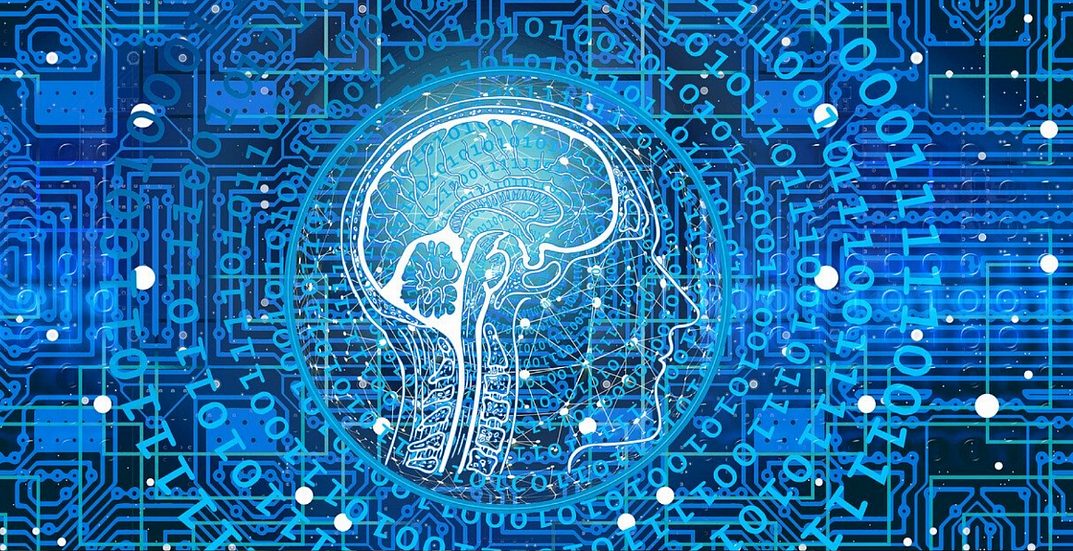
Data quality, governance, security and interoperability
Chapter 4.3: “Transforming healthcare with AI”
EIT Health — European Institute of Innovation and Technology
March 3, 2020
This is an excerpt of the report “ Transforming healthcare with AI. Impact on the workforce and organisations”, published in 2020 by EIT Health. Survey of 175 healthcare professionals, health investors and AI start-up founders and executives
Introduction
When we asked our survey participants what the major barriers were to introducing or scaling AI in healthcare, data cropped up again and again.
It was at the heart of the two most popular answers for investors, the most popular for the startups themselves and a top-three answer for the healthcare professionals, reflecting concerns around data quality and security, the interoperability of systems and the governance and ownership around data (Exhibit 4.2).
Exhibit 4.2 — Data issues dominate the perceived barriers to growing AI in healthcare
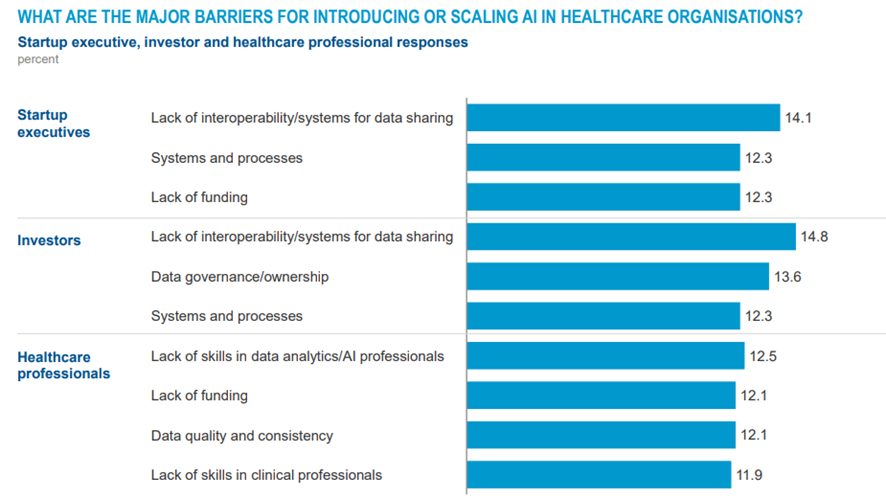
SOURCE: Survey of healthcare professionals, healthcare investors and startup executives across European countries, conducted in December 2019 and January 2020
In the following sections we will explore the data challenge focusing on issues around digitising health to generate data, collecting the data and setting up the governance around data management.
Digitising health and collecting the right data
A key first step for the effective introduction of AI in healthcare is adopting digitised and automated processes.
Healthcare providers have made advances in using EHRs, but there are still many examples of frontline staff recording data manually and of independent clinical systems that cannot communicate with each other or fully integrate all the information relating to a patient.
As new digital solutions are introduced, such fragmentation of data increases.
Interfaces that would seem imperative, such as digital data on patients’ vital signs integrating with EHRs, are often still not standard and the levels of interoperability within and across hospital systems and other care providers are variable at best.
A key first step for the effective introduction of AI in healthcare is adopting digitised and automated processes.
Implementing electronic data collection and automating operational and clinical tasks is still in development in many EU countries and will require significant changes to workflow practices and a shift in workforce skills.
Healthcare is among the least digitised sectors in Europe, lagging behind others in digital business processes, digital spend per worker, digital capital deepening and digitisation of work and processes.
Implementing electronic data collection and automating operational and clinical tasks is still in development in many EU countries and will require significant changes to workflow practices and a shift in workforce skills.
Healthcare is among the least digitised sectors in Europe …
This lack of digital records and processes poses significant challenges for adopting AI solutions not just because data are needed to feed the algorithms, but also because staff frustration about the inadequacies of existing digital systems can lead to a reluctance to adopt more innovative and futuristic AI solutions.
Either way, it is critical to get the basic digitisation of systems and data and the development of digital skills in place before launching AI efforts.
This lack of digital records and processes poses significant challenges for adopting AI solutions …
Systematising digital data collection, linking datasets between systems and ensuring data cleansing is performed routinely, are all prerequisites for introducing AI solutions.
However, there is often a discrepancy between the aspiration for complete clean data, and the reality that most healthcare organisations deal with.
… there is often a discrepancy between the aspiration for complete clean data, and the reality that most healthcare organisations deal with.
Ensuring strong data governance within healthcare organisations
Healthcare providers are used to dealing with vast amounts of clinical data, much of which is highly sensitive and confidential and they have well-tested policies for managing data security; for example asking patients to consent to their data being shared, including for the purposes of clinical research.
However, as more healthcare is delivered using new digital technologies, public concerns about how healthcare data are being used have grown.
There are well-developed data security laws in Europe, the US, Canada and other parts of the world, so one could argue that patients can be reassured about their data security.
However, as more healthcare is delivered using new digital technologies, public concerns about how healthcare data are being used have grown.
Despite this, there have been high-profile cases where AI companies and healthcare providers have been deemed not to have communicated clearly with patients as to how their data would be used, or have appeared to have accessed data without the necessary consents.
In the UK, for example, the Information Commissioner stated in 2017 that the Royal Free NHS Foundation Trust’s sharing of 1.6 million patient records with Google DeepMind failed to comply with the UK Data Protection Act, as “patients would not have reasonably expected their information to have been used in this way, and the Trust could and should have been far more transparent with patients as to what was happening.”[1]115
Healthcare organisations should have robust and compliant data-sharing policies, which support the improvements in care that AI offers while providing the right safeguards.
Our interviewees emphasised that both healthcare as a sector and Europe as a region have significant advantages in terms of data governance.
First, both healthcare organisations and health systems are used to dealing with sensitive data through well-structured data governance and risk-management processes.
In some cases, healthcare could lead the way for other sectors seeking to put such measures in place.
Secondly, Europe benefits from national health systems with extensive datasets, often shared within integrated care systems, offering a set of systems and processes to build on that could also serve as examples to other regions.
… both healthcare as a sector and Europe as a region have significant advantages in terms of data governance.
Of course, as ever more solutions are introduced, these policies need to keep pace with technological advances and organisations and health systems will need to have rapid processes for approving data-sharing agreements with new suppliers.
Of course, as ever more solutions are introduced, these policies need to keep pace with technological advances and organisations and health systems will need to have rapid processes for approving data-sharing agreements with new suppliers.
Processes around data anonymisation also need to be updated to be made fit-for-purpose for the fast-evolving healthcare sector. “We should anonymise data, but not restrict access to data”, said Petia Radeva from the University of Barcelona.
“We should anonymise data, but not restrict access to data”, said Petia Radeva from the University of Barcelona.
Clear guidelines within healthcare organisations and systems on the required levels of pseudonymisation or anonymisation, linking and de-linking of data records needed for particular applications would help both AI developers and clinicians looking to implement their solutions — in particular if they helped simplify and streamline the process of anonymisation.
Physicians we interviewed emphasised that, given the volume of data required for AI, the process of anonymisation in the absence of clear guidelines could have significant cost implications and render diagnostic algorithms prohibitively expensive.
… given the volume of data required for AI, the process of anonymisation in the absence of clear guidelines could have significant cost implications and render diagnostic algorithms prohibitively expensive.
Data interoperability and building bigger datasets
One of the biggest barriers for AI in healthcare is that while enough data are often available across a national system, they are not connected or interoperable.
Policy makers, funding bodies and nonprofit organisations need to support efforts to sufficiently anonymise or link data and, where sensible, build federated networks of datasets that are interoperable and can be queried as needed (with the right security and governance protocols), or help create scaled-up databases that can be accessed only by stakeholders with the appropriate safeguards.
One of the biggest barriers for AI in healthcare is that while enough data are often available across a national system, they are not connected or interoperable.
“People call data the oil of the 21st century. They should be calling it the crude oil of the century. It has huge potential, but it is not trivial to use.”
Dr. med. Dominik Pförringer, TUM Hospital
Recent examples of such data sources include The European Nucleotide Archive, which allows free unrestricted access to genomic data associated with academic life science research.[2]116
More sensitive data, such as those generated by the Danish Reference Genome Project or the 100,000 Genomes Project by Genomics England, are also available for research purposes, but de-identified and with strict access policies.
For instance, the Genomics England dataset is hosted in a secure environment within the datacentre and analytical tools are available inside this environment, but data cannot be moved outside or otherwise downloaded.[3]117
The Israeli government’s recent initiative to combine national digital medical records in a unified system is another example of centralised efforts to spearhead and support the development of national datasets for healthcare, including for applications of AI. [4]118
The Israeli government’s recent initiative to combine national digital medical records in a unified system is another example of centralised efforts to spearhead and support the development of national datasets for healthcare, including for applications of AI.
However, we must acknowledge that healthcare data can be notoriously complex.
They are often gathered by proprietary software and compiled in siloed databases that are part of largely incompatible systems — at times, even within the same hospital.
This makes a large amount of the data in existence virtually useless in their current state.
This is a key consideration for healthcare providers, who need to develop policies to promote interoperability within their own proprietary systems, as well as with those of external suppliers and other providers.
However, we must acknowledge that healthcare data can be notoriously complex.
… a large amount of the data in existence is virtually useless in their current state
… some healthcare providers, need to develop policies to promote interoperability within their own proprietary systems, as well as with those of external suppliers and other providers.
In order to make the most of the rich data available, healthcare systems need an interconnected data infrastructure, as well as standards for data formats, be they Fast Interoperability Resources or open EHRs, data exchange and semantic interoperability of medical terminologies to reduce noise in the data.[5]119
Such efforts have been successful in the past, for example in gene- and protein-naming conventions, in data-format standards for next-generation DNA sequencing data and the International Classification of Diseases codes.[6]120
They have often developed organically, proposed by groups of researchers or international committees of larger organisations and could offer an example of a potential path for similar efforts on AI.
In order to make the most of the rich data available, healthcare systems need an interconnected data infrastructure, as well as standards for data formats, be they Fast Interoperability Resources (FHIR) or open EHRs, data exchange and semantic interoperability of medical terminologies to reduce noise in the data.
What needs to change to encourage the introduction and scaling of AI in healthcare?
Section 3: Strengthening data quality, governance, security and interoperability.
This is an excerpt from the Executive Summary.
Both interviewees and survey respondents emphasised that data access, quality and availability were potential roadblocks.
The data challenge breaks down into digitising health to generate the data, collecting the data, and setting up the governance around data management.
MGI analyses show that healthcare is among the least digitised sectors in Europe, lagging behind in digital business processes, digital spend per worker, digital capital deepening and the digitisation of work and processes.
It is critical to get the basic digitisation of systems and data in place before embarking on AI deployments — not least because the frustrations staff have with basic digitisation could spill over to the wider introduction of AI.
It is critical to get the basic digitisation of systems and data in place before embarking on AI deployments — not least because the frustrations staff have with basic digitisation could spill over to the wider introduction of AI.
In addition, as more healthcare is delivered using new digital technologies, public concerns about how healthcare data are used have grown.
Healthcare organisations should have robust and compliant data-sharing policies that support the improvements in care that AI offers while providing the right safeguards in a cost-efficient way.
Physicians we interviewed emphasised that, given the volume of data required for AI, a poorly thought out process of anonymisation could be a major cost, making diagnostic algorithms prohibitively expensive.
Healthcare organisations should have robust and compliant data-sharing policies that support the improvements in care that AI offers while providing the right safeguards in a cost-efficient way.
Interviewees also emphasised, however, that both healthcare as a sector and Europe as a region have significant advantages.
First, both healthcare organisations and health systems are used to dealing with sensitive data through well-structured data governance and risk management processes.
In some cases, healthcare could lead the way for other sectors seeking to put such measures in place.
Secondly, Europe benefits from national health systems with extensive datasets, often shared within integrated care systems, offering a set of systems and processes to build on that could also serve as examples to other regions.
Interviewees also emphasised, however, that both healthcare as a sector and Europe as a region have significant advantages.
The final data challenge is getting datasets to talk to each other.
Policy makers, funding bodies and nonprofit organisations need to support efforts to sufficiently anonymise and link data and, where sensible, to build databases that can be accessed by stakeholders with the appropriate safeguards.
In order to make the most of the rich data that is available, healthcare systems need an interconnected data infrastructure.
This is an area where Europe, as mentioned, could have a significant advantage, in terms of its extensive national datasets and its networks of innovations clusters or hubs and pan-European collaborations with academia and industry, providing a prototype for the creation of centres of excellence for AI in healthcare.
Policy makers, funding bodies and nonprofit organisations need to support efforts to sufficiently anonymise and link data and, where sensible, to build databases that can be accessed by stakeholders with the appropriate safeguards.
In order to make the most of the rich data that is available, healthcare systems need an interconnected data infrastructure.
This is an excerpt of the report “ Transforming healthcare with AI. Impact on the workforce and organisations; EIT Health and McKinsey & Company, (2020). Survey of 175 healthcare professionals, health investors and AI start-up founders and executives
References
See the original publication
TAGS: Data Driven Health Care; Interoperability; Data Governance; Data Management; Data Skills; Barriers; Issues; Use Cases; Digital Health Systems;