By 2026, applying automated trust metrics across internal and external data ecosystems will replace most outside intermediaries, reducing data sharing risk by 50%.
D&A leaders at high-performing organizations promote data sharing or increase access to the right data aligned to the business case.
This is an excerpt of the publication below, with the title above, focusing on the topic in question. For the full version of the article, please, refer to the original publication.
Gartner
Top Trends in Data and Analytics, 2022
By Rita Sallam, Ted Friedman, and 37 more
11 March 2022
Excerpt by
Joaquim Cardoso MSc.
The Health Revolution
Multidisciplinary Institute for Better Health for All
May 27, 2022
Top Trends in Data and Analytics
The top D&A trends represent business, market and technology dynamics that you cannot afford to ignore
They have the potential to transform your enterprise, and will accelerate in their adoption over the next three years.
You should decide whether to proactively monitor, experiment with or aggressively invest in key trends based on their urgency and alignment to strategic priorities.
The top D&A trends and technologies do not exist in isolation; they build on and reinforce one another.
We have selected our trends for 2022 in part based on their combined effects. Taken together, our top data and analytics technology trends for 2022 will help you meet your organization’s top strategic priorities to anticipate, adapt and scale value.
This, in turn, will enable you to encourage innovation and put in place success metrics and incentives that emphasize learning and reward innovation.
Top Trends in Data and Analytics
- Group 1: Activate Dynamism and Diversity
- Group 2: Augment People and Decisions
- Group 3: Institutionalize Trust
Group 1: Activate Dynamism and Diversity
- Adaptive AI Systems
- Data-Centric AI
- Metadata-Driven Data Fabric
- Always Share Data
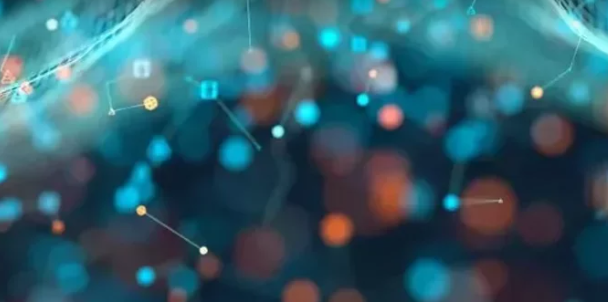
4.Always Share Data
Analysis by: Lydia Clougherty Jones, Eric Hunter, Malcolm Hawker, Jason Medd
SPA:
By 2026, applying automated trust metrics across internal and external data ecosystems will replace most outside intermediaries, reducing data sharing risk by 50%.
By 2026, applying automated trust metrics across internal and external data ecosystems will replace most outside intermediaries, reducing data sharing risk by 50%.
Description:
Data sharing includes sharing data internally (between or among departments or across commonly owned and controlled parties such as subsidiaries or sister companies) and externally (between or among parties outside the ownership and control of your organization).
The longstanding calculus that data sharing is not worth the risk of data misuse is obsolete.
We observe that a risk of failure now inures to those organizations that do not share data automatically, or “always share data,” unless there is a vetted reason not to.
Data sharing is a business-facing key performance indicator that an organization is achieving effective stakeholder engagement and providing enterprise value.2
D&A leaders at high-performing organizations promote data sharing or increase access to the right data aligned to the business case.3
D&A leaders at high-performing organizations promote data sharing or increase access to the right data aligned to the business case.
Why Trending:
- Organizations need more and more singular types and complex combinations of data to feed their increasingly voracious use of data and analytics in order to drive business value.
This can often be found in silos within the organization and increasingly, external to it. - D&A leaders responsible for building a data-driven enterprise also require expansive data sharing, yet they struggle to identify and then locate the right data for their business case.
They also face internal data hoarding, external data hijacking and privacy shaming. - The global COVID-19 pandemic created urgency to share data in order to accelerate independent and interrelated public and commercial digital business value, as well as improvements to surrounding agility and resilience.
- Global data strategies highlight data sharing as a key priority for increasing government efficiency and generating public value.
They also encourage industry data sharing with the purpose of producing market growth. - External data has an increased level of relevance for D&A leaders in support of predictive models, as models trained exclusively with internal or first-party data have seen model drift due to phase shifts in customer behaviors.
- There is a lack of relevant available data for AI training, as well as sustainability and cost pressures for processing large amounts of AI training data.
- We observe increased demand for more robust predictive analytics generated from more diverse data sources to drive relevant, unique or otherwise unknowable insights and data-driven innovation.
The global COVID-19 pandemic created urgency to share data in order to accelerate independent and interrelated public and commercial digital business value, as well as improvements to surrounding agility and resilience.
There is a lack of relevant available data for AI training, as well as sustainability and cost pressures for processing large amounts of AI training data.
Implications:
- D&A leaders know that data sharing is a key digital transformation capability, but they lack the “know how” to share data at scale and with trust.
- &A leaders will change their investment priorities to automate the identification and acquisition of the most relevant data, whether from big, small, personal, synthetic or yet to be created data, to match their business case.
- Remedying interoperability challenges and adopting a data fabric design will become a priority, contributing to environmental sustainability through data centralization, reuse and resharing, while meeting or exceeding stakeholder value and business outcomes, including composable business objectives.
- Automation and open-data programs ease the investment burden; machine-readable metadata provides automatic discovery of datasets and services, and open standards for metadata lower the barriers for their discoverability, reuse and resharing.
Recommendations:
Data and analytics leaders should:
- Collaborate across business and industry lines, promoting data sharing to create individual and aggregate stakeholder value.
This will accelerate buy-in for increased budget authority and investment in data sharing. - Establish trust in internal and external data, metadata, data sources, data sharing technologies, and downstream reuse and resharing ecosystems through automated mechanisms and metrics from active metadata insights, augmented data catalogs and automated data quality metrics
- Overcome data sharing hurdles by focusing on, and then quantifying, the risk of not sharing data, including business failure.
- Consider adopting data fabric design to enable a single architecture for data sharing across heterogeneous internal and external data sources.
Changes Since Last Year
As part of the Top Data and Analytics Trends, 2021, we introduced a trend called “D&A as a Core Business Function,” which noted that smarter data sharing increasingly plays a key business role.
Smarter data sharing has evolved from “don’t share data unless” to “share data always,” unless there is a well vetted reason not to.
Smarter data sharing has evolved from “don’t share data unless” to “share data always,” unless there is a well vetted reason not to.
Originally published at https://www.gartner.com