5.Context-Enriched Analysis;
6.Business-Composed D&A;
7.Decision-Centric D&A and
8.Skills and Literacy Shortfall
This is an excerpt of the publication below, with the title above, focusing on the topic(s) in question. For the full version of the article, please, refer to the original publication.
Gartner
Top Trends in Data and Analytics, 2022
By Rita Sallam, Ted Friedman, and 37 more
11 March 2022
Excerpt by
Joaquim Cardoso MSc.
The Health Revolution
Multidisciplinary Institute for Better Health for All
May 27, 2022
Top Trends in Data and Analytics
The top D&A trends represent business, market and technology dynamics that you cannot afford to ignore
They have the potential to transform your enterprise, and will accelerate in their adoption over the next three years.
You should decide whether to proactively monitor, experiment with or aggressively invest in key trends based on their urgency and alignment to strategic priorities.
The top D&A trends and technologies do not exist in isolation; they build on and reinforce one another.
We have selected our trends for 2022 in part based on their combined effects. Taken together, our top data and analytics technology trends for 2022 will help you meet your organization’s top strategic priorities to anticipate, adapt and scale value.
This, in turn, will enable you to encourage innovation and put in place success metrics and incentives that emphasize learning and reward innovation.
Top Trends in Data and Analytics
- Group 1: Activate Dynamism and Diversity
- Group 2: Augment People and Decisions
- Group 3: Institutionalize Trust
Group 2: Augment People and Decisions
5.Context-Enriched Analysis
6.Business-Composed D&A
7.Decision-Centric D&A
8.Skills and Literacy Shortfall
Key prognosis:
- By 2025, context-driven analytics and AI models will replace 60% of existing models built on traditional data.
- By 2025, 50% of embedded analytics content will be developed by business users leveraging a low-code/no-code modular assembly experience.
- By 2023, more than 33% of large organizations will have analysts practicing decision intelligence, including decision modeling.
- Through 2025, the majority of CDOs will have failed to foster the necessary data literacy within the workforce to achieve their stated strategic data-driven business goals.
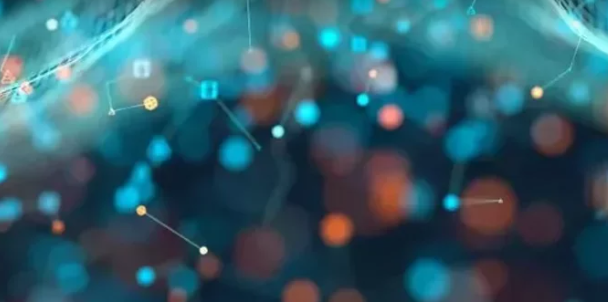
5.Context-Enriched Analysis
Analysis by: Afraz Jaffri, David Pidsley, Sumit Pal
SPA:
By 2025, context-driven analytics and AI models will replace 60% of existing models built on traditional data.
By 2025, context-driven analytics and AI models will replace 60% of existing models built on traditional data.
Description:
Contextual data originates in multiple sources, including image, text, audio, log, sensor and associated metadata. It is needed to build a richer knowledge-based-model of business entities and relationships. Holding this information in a graph structure enables deeper analysis utilizing the relationships between data points as much as the data points themselves. Graph analytics exploits this structure to identify and create further context based on similarities, constraints, paths and communities. This forms the basis for the utilization of active metadata that drive data fabrics, personalization of automated insights and data stories, powerful feature sets for machine learning models and a wider field of vision for improved decision making.
Why Trending:
- In an ever-changing world full of uncertainty, context is a necessity, not just “nice to have.” Utilizing contextual data enables building a richer knowledge-based model of business entities and relationships to meet the challenge of accelerated internal and external business dynamics.
- Analytics and data science techniques are applied to data where the properties of each data item are taken in isolation. Adding contextual information gathered from the relationships between entities uncovers new patterns of behavior that reveal facts and better insights and generate more predictive power.
- Advances in techniques for audio, image and video recognition, natural language processing (NLP) and speech-to-text are increasing the accessibility of a wider set of data for use in advanced analytics and decision intelligence.
- Augmented analytics tools capture contextual information about the user, environment, datasets and analytics outputs to generate automated insights and data stories, as well as personalized news feeds in social-media-style timelines.
- There is an overload of analytics content and insights. Preserving context enables targeted insights to be provided on demand, enabling consumers to concentrate on core insights that require exploration and action.
- Graph data models naturally accommodate the context of data through relationships and graph structural properties. Data is represented in a form that is easily understood by business users and domain experts.
- Increasing the usage of data fabrics within data management exposes metadata that captures the context and usage of data assets.
Implications:
- Capturing, storing and utilizing contextual data demands capabilities and skills in building data pipelines, X analytics techniques and AI cloud services that can process different data types and provisioning use cases that include this data for end-user applications.
- Automated insight generation using greater contextual insights enables analysts who produce BI reports and dashboards to focus on the more complex needs of business users. It also enables them to answer critical business questions and improve insights that are used in decision making. This will shift the traditional skills required for analysts from technology-centric to business-focused, and give rise to the importance of business translators who can identify decision-making points and priorities.
- Identifying and accessing contextual data that lies outside an organization requires the enablement of data sharing services and exchanges and the creation of policies that promote and regulate internal and external data sharing.
Recommendations:
Data and analytics leaders should:
- Identify multiple data sources and formats that can be used to augment data that is already used for analytics, data science and AI, and enable the extraction of key entities and relationships.
- Utilize the power and flexibility of graph data models to connect data that exists across the organization into graph structures that can be fed into downstream data management, analytics and AI processes.
- Augment existing machine learning models with features extracted from graph representation using X analytics, graph analytics and data science techniques.
- Identify key personas that can benefit from shorter time to insight and assess analytics and BI platforms that deliver contextual insights.
Changes Since Last Year
The “Context-Enriched Analytics” trend builds on previous top D&A trends on graph technologies as a key enabler for data and analytics innovation. This year, the focus is on bridging the gap between structured, unstructured and metadata with graphs and the utilization of graph analytics for an increasing number of business use cases, both stand-alone and within packaged applications.
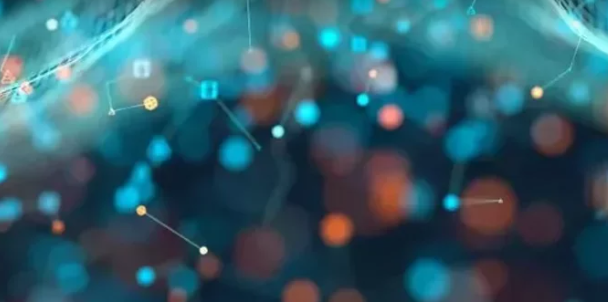
6.Business-Composed D&A
Analysis by: Julian Sun, Yefim Natis, Shaurya Rana
SPA:
By 2025, 50% of embedded analytics content will be developed by business users leveraging a low-code/no-code modular assembly experience.
By 2025, 50% of embedded analytics content will be developed by business users leveraging a low-code/no-code modular assembly experience.
Description:
Business-composed D&A refers to the active building of D&A capabilities by business technologists using low-code composition technology along with collaboration tools to assemble the modular and rich capabilities of organization-level value streams. “Business-composed D&A” shifts application development power to the business users or business technologists, enabling them to craft business-driven D&A capabilities collaboratively.
Why Trending:
- Historically, building custom analytics applications or embedding data and analytics in a workflow or business process required traditional IT-led embedded D&A capabilities, such as APIs and developer software development kits (SDKs). This is slow and expensive, and requires a high level of technical skills.
- Per a recent Gartner Building Digital Platforms survey, D&A capabilities are the most common and fastest growing capabilities to be integrated with digital business platforms within an enterprise, enabling agile decision-making.4
- IT-led embedded D&A is time consuming to deploy and lacks business impact. Business users expect to close the loop of D&A and build the last mile of it by turning insights into business actions.
- Fully decentralized D&A causes siloed insights that achieve local goals at the expense of achieving organizationwide goals.
Implications:
- D&A leaders will increasingly foster and build collaborative D&A experiences and processes with cross-functional teams.
- The adoption of business-composed D&A, facilitated by fusion teams, will bridge the insights with actions and decrease business outcome challenges, which wasn’t possible with fragmented embedded D&A deployed by IT.
- Organizations will develop a business-driven data and analytics architecture by composing packaged business capabilities (PBCs) aligned with value streams.
- The D&A catalog will evolve as business technologists create PBCs as reusable D&A assets for others to easily compose and recompose, beyond traditional datasets, reports or dashboards that need slow and expensive integration.
- The expanded capabilities would bring more complex governance concerns for both D&A and application development, requiring more Ops practices to manage production. A more comprehensive Citizen-X program might emerge within the community to share skills, best practices and governance rules and processes
Recommendations:
Data and analytics leaders should:
- Prepare for growth in the number of citizen developers and business technologists using low-code/no-code technology to broaden their use of self-service analytics by providing training in basic software development practices.
- Evaluate your existing analytics and data science tools and innovative startups offering low-code enabled composition experience to build rich analytics-infused applications within the business process.
- Foster collaboration and build more PBCs by creating cross-functional fusion teams of both centralized and decentralized D&A users that are aligned with the corporate-level value streams.
Changes Since Last Year
As part of the Top Data and Analytics Trends, 2021, we introduced a trend called “Composable Data and Analytics.” Business-composed D&A builds on previous trends in low-code-enabled composition from modular ABI and DSML capabilities as a key enabler for D&A applications. This year, the focus is on the people side, which is shifting from IT to business, composing D&A capabilities that are aligned with value stream management and powered by automation workflow.
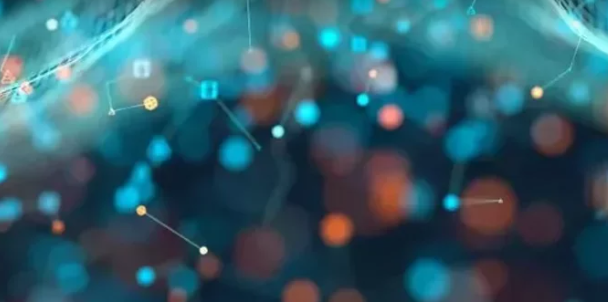
7.Decision-Centric D&A
Analysis by: Gareth Herschel, W. Roy Schulte
SPA:
By 2023, more than 33% of large organizations will have analysts practicing decision intelligence, including decision modeling.
By 2023, more than 33% of large organizations will have analysts practicing decision intelligence, including decision modeling.
Description:
Careful consideration of how decisions should be made (the discipline of decision intelligence — see Note 1) is causing organizations to rethink their investments in D&A capabilities. The application of D&A to organizational decisions requires contextual understanding that this application can take several forms, such as decision support, augmentation or automation. The initial focus of decision-centric D&A is on the insight that decision makers need to govern D&A investments, rather than on the available data or the analysis that can be carried out. This is a sign of increasing maturity of D&A strategies and organizations’ ability to reengineer decisions to drive business outcomes.
Why Trending:
- Access to a growing variety of data, combined with a continually increasing variety of analytical techniques, means organizations have an overwhelming permutation of decisions to make about the analysis they could carry out. Relevance to organizational decisions is an easily identifiable filter for these options.
- Forty-seven percent of organizations believe that the decisions they face will be more complex, increasing demand for connected, continuous and contextual D&A and explainable decision processes.5 Decisions are increasingly interconnected and based on continuously changing data about the business context. Without D&A, the growing complexity of decisions is impossible for organizations to manage.
- Only 58% of organizations report that they consistently and formally define decision ownership. Decisions are made in several different ways. Personal decision making based on experience, training or intuition is the most common, but also the most difficult to combine with D&A.
- Improving the quality of decisions is not a new goal, but organizations are not good at building the systems and processes needed for it to be possible. This is the intent behind growing interest in the discipline of decision intelligence.
Implications:
- Decision-centricity requires a deep understanding of human psychology and behavior. Embedding D&A into collaborative or individual decisions is most likely to be successful when supported by NLP or data visualization capabilities.
- Decision-centric D&A needs to be aligned with enterprise architecture and technical development teams. D&A can be embedded into decisions in several different ways. For example, embedding analytic decision models (e.g., machine learning) into business processes requires technical integration with business applications, as described in the Business-Composed D&A trend.
- Time and complexity are the main criteria for determining which decisions are most suited to automation with D&A. Simple problems that can be confronted quickly are the most appropriate candidates for automation, while highly complex problems that need time to solve require decision support and augmentation, rather than automation.
Recommendations:
Data and analytics leaders should:
- Approach D&A projects by considering which decisions you are seeking to influence, rather than what data you possess or how to analyze it.
- Create new decision-making habits by training decision makers to apply best practices, such as critical thinking, trade-off analysis, recognizing bias and listening to opposing views.
- Consider creating a role for decision engineers by hiring or upskilling experts who can work with decision makers to identify opportunities that would benefit from the rigor of decision intelligence practices.
Approach D&A projects by considering which decisions you are seeking to influence, rather than what data you possess or how to analyze it.
Changes Since Last Year
The application of D&A to decisions has often been an add-on; we have the data and analysis, then we think about how to accelerate its deployment to real time, or contextualize it for a collaborative decision. We are seeing a switch to a decision-first approach: using decision intelligence disciplines to design the best decision, and then delivering the required inputs (possibly including D&A). This flip from D&A-driven decisions to decision-driven D&A is subtle, but fundamental.
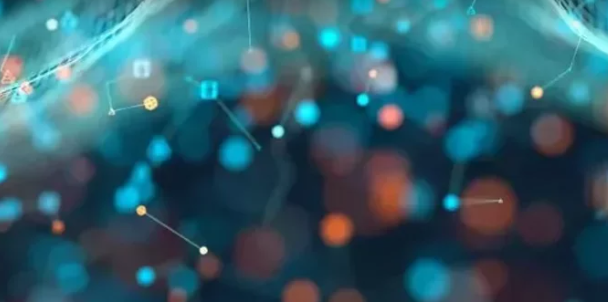
8.Skills and Literacy Shortfall
Analysis by: Alan D. Duncan, Eric Hunter, Jorgen Heizenberg, Peter Krensky, Joe Maguire, Sally Parker
SPA:
Through 2025, the majority of CDOs will have failed to foster the necessary data literacy within the workforce to achieve their stated strategic data-driven business goals.
Through 2025, the majority of CDOs will have failed to foster the necessary data literacy within the workforce to achieve their stated strategic data-driven business goals.
Description:
The 2021 Gartner CDO Survey shows that organizations that deal with the human elements of D&A are more successful than organizations that only consider technology. With a human focus, the mission of D&A is to foster broader data literacy and digital learning, rather than simply delivering core platforms, datasets and tools. Organizations (and society) will need to learn how to learn, and they will need a lot of help to do so.
Why Trending:
- Virtual workplaces and the heightened competition for D&A talent have exposed weaknesses in organizations lacking content-neutral and enterprise-specific data literacy strategies
- Increasing talent acquisition efforts require D&A leaders to take an increasingly agile approach to data literacy and upskilling investments. New hires are increasingly dependent on this investment to accelerate their contribution of critical delivery and support roles across the organization.
- Cultural aversion to change is a prevailing and recurring roadblock to the success of D&A programs. Expecting data literacy in the workforce as a default is a false presumption.
- Momentum is being driven by vendors addressing customer enablement, advocacy and adoption as part of their go-to-market (e.g., Tableau, Qlik, Alteryx, Collibra). More and more providers are offering learning solutions for D&A. These solutions include not just technical learning, but also the “soft” skills for curiosity, critical thinking and communication (e.g., Pluralsight, Skillsoft, The Center for Applied Data Science, Udacity).
Implications:
- CDOs must distinguish transferrable/domain-neutral skills from domain-specific knowledge and experience of the organization’s own processes. Those who are carrying out succession planning are not referring to it as such.
- Data literacy and technical upskilling strategies will increasingly embrace the concept of “just-in-time” channels and options for the provision of key skills and concepts as talent becomes increasingly fluid (new hires, contingent workers, etc.).
- D&A leaders will reduce their selectivity criteria for new hires as competition for talent intensifies, but will compensate for this through diverse upskilling strategies to complement new hires’ potential.
- The cost of investing in data literacy and employee upskilling will lead employers to insert “claw-back” or “payback” clauses into contracts with new hires in order to recover costs in the event that an employee departs the organization.
- Traditional succession planning discussions will incorporate data literacy performance.
- Platform and technology selections will increasingly prioritize capabilities for prebuilt content and customer literacy training and assessment as a part of key workflows and platform usage
Recommendations:
Data and analytics leaders should:
- Take the lead in creating a narrative that sets a strong vision for the desired end state and business outcomes, particularly with respect to innovation opportunities and use cases that have not been identified by others.
- Work with line-of-business leaders to trace measurable business outcomes back to supporting analytics output and underlying data.
- Monitor the effects of improved data literacy among the workforce by using data literacy assessments and measuring associated improvements to data-driven business outcomes.
- Distinguish between competition for people who already have D&A skills and educating/training those who currently do not.
Develop a multispeed approach: different approaches for the most enthusiastic, the slower on the uptake and the outright resisters. - Collaborate with HR and business leaders to run data literacy pilot projects in business areas where there is a high likelihood of achieving measurable business outcomes.
Use quick wins to build momentum and incentivize staff to use data in their interactions.
Using workers’ stated pain points as stimuli can get them to identify the changes necessary to address those pain points. - Go beyond vendor product training to focus on people’s roles.
Use a mix of training delivery methods by considering the times, locations, roles and skills differences to improve overall learning effectiveness and experiences for new analytics capabilities. - Formulate a succession plan for key D&A roles both inside and outside IT. Budget for 25% turnover.
Incentivize teaching colleagues what you know, rather than engaging in self-interest-based protectionism. - Coordinate data literacy initiatives with overall data governance programs.
Take the lead in creating a narrative that sets a strong vision for the desired end state and business outcomes, particularly with respect to innovation opportunities and use cases that have not been identified by others.
Formulate a succession plan for key D&A roles both inside and outside IT. Budget for 25% turnover.
Changes Since Last Year
The limited pool of D&A talent has become acute, with competition for skilled staff escalating amid the “great resignation.” There is an increasing recognition that this cannot be solved by reorganization, but by development of the workforce.
Originally published at https://www.gartner.com