The Health Strategist
institute for
continuous health transformation
and digital health
Joaquim Cardoso MSc
Chief Research and Strategy Officer (CRSO)
May 12, 2023
EXECUTIVE SUMMARY:
Generative AI is a rapidly evolving technology that has the potential to transform businesses and improve existing workflows.
- Generative AI, exemplified by tools like ChatGPT, Bard, Claude, and Midjourney, has gained significant attention and popularity.
- It stands out from previous AI technologies due to its accessibility and democratization.
- Users do not require extensive machine learning knowledge to interact with and derive value from generative AI tools.
- The technology’s versatility enables a wide range of applications, making it suitable for diverse audiences worldwide.
Generative AI is powered by foundation models, expansive neural networks trained on vast amounts of unstructured data.
- Unlike previous AI models, which were narrow in scope, foundation models can perform multiple tasks across various domains.
- For instance, a single foundation model can create executive summaries, draft business strategies, and generate recipes.
- However, the versatility of generative AI can sometimes result in less accurate outcomes, necessitating careful attention to AI risk management.
By implementing proper guardrails, generative AI can enhance and accelerate existing business processes.
- For instance, during a sales call, generative AI can analyze real-time conversations to suggest upselling opportunities, drawing from various data sources.
- It can also generate initial sales pitches for personalization.
- The potential benefits extend to knowledge workers across different roles, with generative AI integrated into everyday tools, thereby increasing productivity.
CEOs face the decision of whether to act swiftly and seize the transformative potential of generative AI or adopt a more cautious approach with limited investments.
- Factors to consider include technical expertise, technology infrastructure, data architecture, operating models, and risk management processes required for successful generative AI implementation.
The potential of generative AI is undeniable, and executives are eager to leverage this technology strategically.
The field of generative AI is rapidly evolving, and a comprehensive value chain is emerging to support its training and application.
- Specialized hardware provides the necessary computational power, while cloud platforms enable access to this hardware.
- MLOps and model hub providers offer the tools and technologies needed to adapt and deploy foundation models.
- Many companies are entering the market to offer applications built on top of foundation models, catering to specific tasks and customer needs.
Previously, developing foundation models required substantial investment and resources, limiting their development to a few tech giants, well-funded startups, and open-source research collectives.
However, advancements are being made in developing smaller and more efficient models, which could open the market to more entrants.
- Startups such as Cohere, Anthropic, and AI21 Labs have successfully developed and trained their own large language models.
Generative AI has the potential to create value across various use cases. CEOs should view exploration of generative AI as essential to stay competitive.
- It can transform multiple aspects of a company, from research and development to marketing and customer operations.
- Organizations can choose to start small and scale later or take a transformative approach from the beginning.
- Technical pathways exist to execute the strategy based on the specific use case.
Generative AI can enhance productivity for knowledge workers by integrating its features into existing software systems.
- Email systems can generate drafts,
- productivity applications can create presentations,
- financial software can generate financial reports, and
- customer-relationship-management systems can suggest customer interaction methods.
This technology can also be transformative in specific use cases.
CEOs can learn valuable lessons from Generative AI examples:
- Transformative use cases exist across sectors, and companies can start small or large based on their aspirations.
- Costs of pursuing generative AI vary depending on the use case and required resources.
- Building a basic business case before starting can help navigate the generative AI journey effectively.
Considerations for getting started with generative AI:
- Organize cross-functional teams to identify high-value use cases and enable coordinated implementation across the organization.
- Reimagine end-to-end domains rather than focusing on sporadic use cases, aligning with the organization’s overall strategy.
- Ensure a fully loaded technology stack with the necessary computing resources, data systems, and tools for generative AI.
- Build a “lighthouse” by showcasing internal applications of generative AI, fostering enthusiasm, and refining business cases.
- Balance risk and value creation by establishing ethical guidelines, understanding risks associated with each use case, and staying updated on generative AI regulations.
- Apply an ecosystem approach by partnering with generative AI vendors and experts to accelerate execution and prevent vendor lock-in.
- Focus on building the required talent and skills within the organization through hiring, upskilling, and ongoing education and training.
Generative AI represents a leap forward with new possibilities.
Businesses should start their generative AI journey based on their aspirations and risk appetite, learning through practical implementation and experimentation.
DEEP DIVE
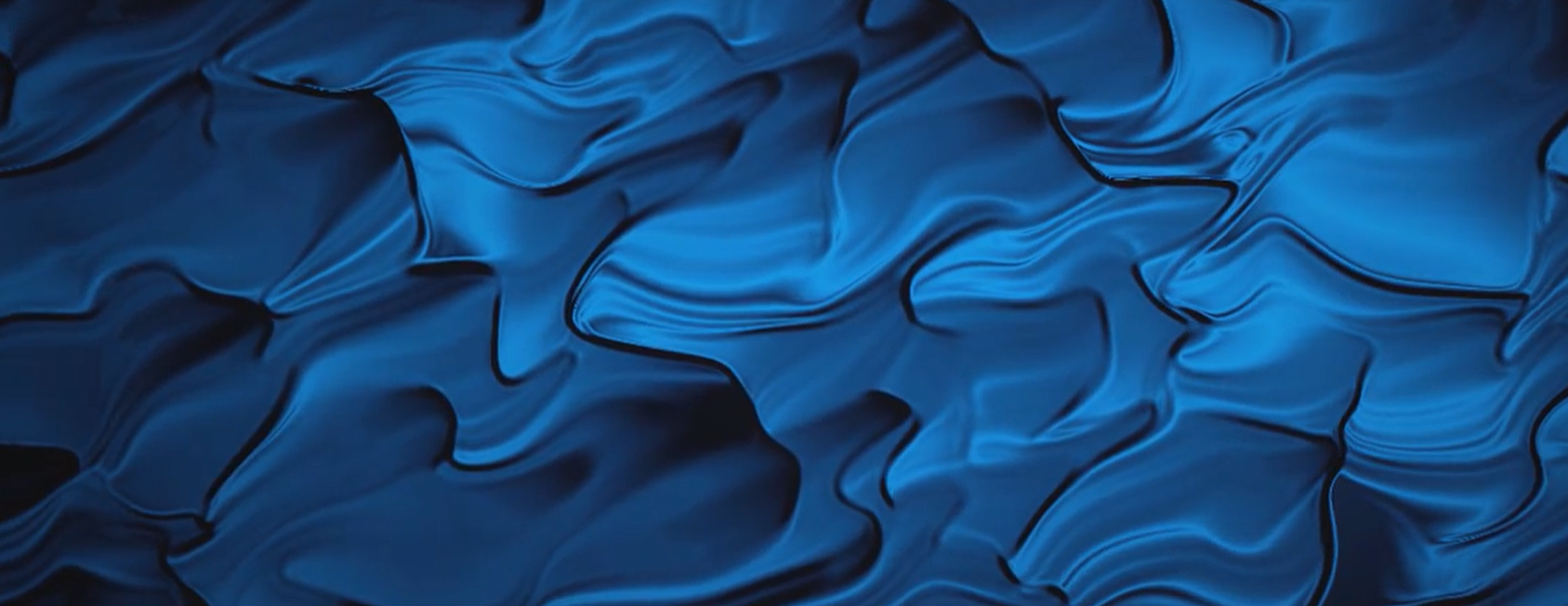
What every CEO should know about generative AI
Generative AI is evolving at record speed while CEOs are still learning the technology’s business value and risks. Here, we offer some of the generative AI essentials.
Michael Chui, Roger Roberts, Tanya Rodchenko, Alex Singla, Alex Sukharevsky, Lareina Yee, and Delphine Zurkiya,
May 12, 2023
Amid the excitement surrounding generative AI since the release of ChatGPT, Bard, Claude, Midjourney, and other content-creating tools, CEOs are understandably wondering: Is this tech hype, or a game-changing opportunity? And if it is the latter, what is the value to my business?
The public-facing version of ChatGPT reached 100 million users in just two months. It democratized AI in a manner not previously seen while becoming by far the fastest-growing app ever. Its out-of-the-box accessibility makes generative AI different from all AI that came before it. Users don’t need a degree in machine learning to interact with or derive value from it; nearly anyone who can ask questions can use it. And, as with other breakthrough technologies such as the personal computer or iPhone, one generative AI platform can give rise to many applications for audiences of any age or education level and in any location with internet access.
All of this is possible because generative AI chatbots are powered by foundation models, which are expansive neural networks trained on vast quantities of unstructured, unlabeled data in a variety of formats, such as text and audio. Foundation models can be used for a wide range of tasks. In contrast, previous generations of AI models were often “narrow,” meaning they could perform just one task, such as predicting customer churn. One foundation model, for example, can create an executive summary for a 20,000-word technical report on quantum computing, draft a go-to-market strategy for a tree-trimming business, and provide five different recipes for the ten ingredients in someone’s refrigerator. The downside to such versatility is that, for now, generative AI can sometimes provide less accurate results, placing renewed attention on AI risk management.
With proper guardrails in place, generative AI can not only unlock novel use cases for businesses but also speed up, scale, or otherwise improve existing ones. Imagine a customer sales call, for example. A specially trained AI model could suggest upselling opportunities to a salesperson, but until now those were usually based only on static customer data obtained before the start of the call, such as demographics and purchasing patterns. A generative AI tool might suggest upselling opportunities to the salesperson in real time based on the actual content of the conversation, drawing from internal customer data, external market trends, and social media influencer data. At the same time, generative AI could offer a first draft of a sales pitch for the salesperson to adapt and personalize.
The preceding example demonstrates the implications of the technology on one job role. But nearly every knowledge worker can likely benefit from teaming up with generative AI. In fact, while generative AI may eventually be used to automate some tasks, much of its value could derive from how software vendors embed the technology into everyday tools (for example, email or word-processing software) used by knowledge workers. Such upgraded tools could substantially increase productivity.
CEOs want to know if they should act now-and, if so, how to start. Some may see an opportunity to leapfrog the competition by reimagining how humans get work done with generative AI applications at their side. Others may want to exercise caution, experimenting with a few use cases and learning more before making any large investments. Companies will also have to assess whether they have the necessary technical expertise, technology and data architecture, operating model, and risk management processes that some of the more transformative implementations of generative AI will require.
The goal of this article is to help CEOs and their teams reflect on the value creation case for generative AI and how to start their journey. First, we offer a generative AI primer to help executives better understand the fast-evolving state of AI and the technical options available. The next section looks at how companies can participate in generative AI through four example cases targeted toward improving organizational effectiveness. These cases reflect what we are seeing among early adopters and shed light on the array of options across the technology, cost, and operating model requirements. Finally, we address the CEO’s vital role in positioning an organization for success with generative AI.
Excitement around generative AI is palpable, and C-suite executives rightfully want to move ahead with thoughtful and intentional speed. We hope this article offers business leaders a balanced introduction into the promising world of generative AI.
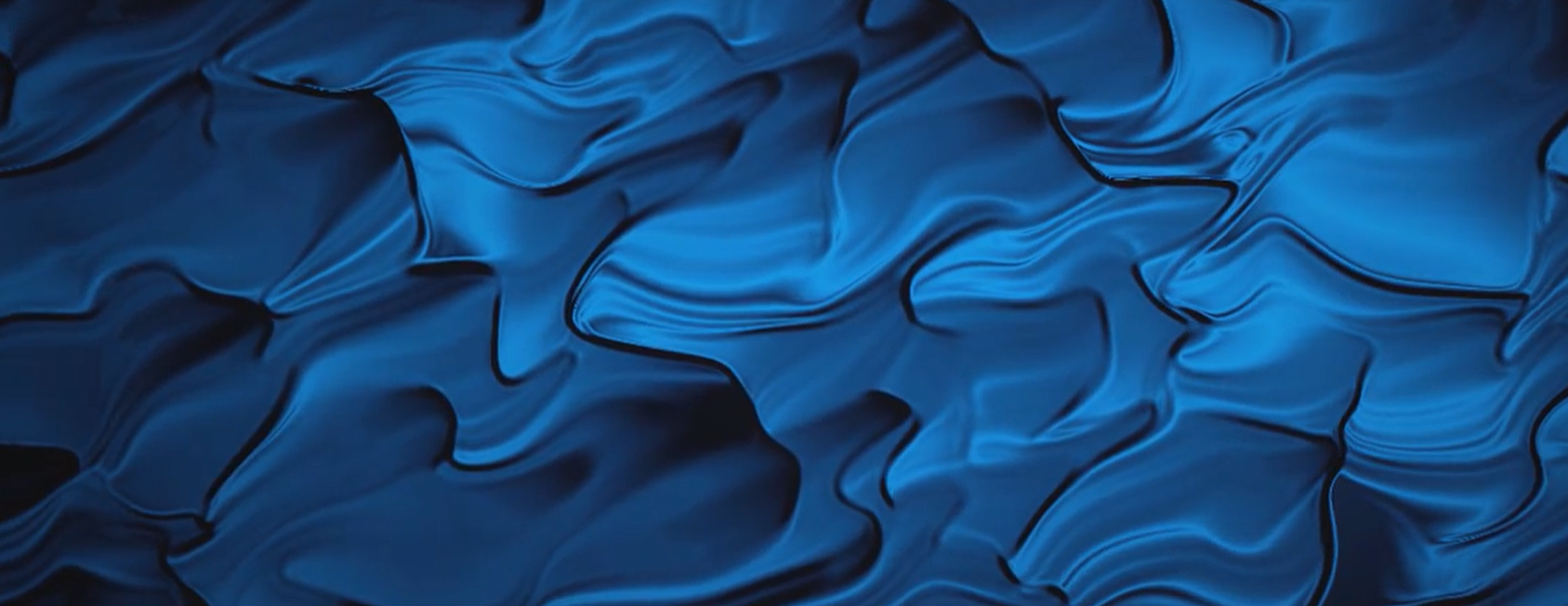
A generative AI primer
Generative AI technology is advancing quickly (Exhibit 1). The release cycle, number of start-ups, and rapid integration into existing software applications are remarkable. In this section, we will discuss the breadth of generative AI applications and provide a brief explanation of the technology, including how it differs from traditional AI.
More than a chatbot
Generative AI can be used to automate, augment, and accelerate work. For the purposes of this article, we focus on ways generative AI can enhance work rather than on how it can replace the role of humans.
While text-generating chatbots such as ChatGPT have been receiving outsize attention, generative AI can enable capabilities across a broad range of content, including images, video, audio, and computer code. And it can perform several functions in organizations, including classifying, editing, summarizing, answering questions, and drafting new content. Each of these actions has the potential to create value by changing how work gets done at the activity level across business functions and workflows. Following are some examples.
Classify
- A fraud-detection analyst can input transaction descriptions and customer documents into a generative AI tool and ask it to identify fraudulent transactions.
- A customer-care manager can use generative AI to categorize audio files of customer calls based on caller satisfaction levels.
Edit
- A copywriter can use generative AI to correct grammar and convert an article to match a client’s brand voice.
- A graphic designer can remove an outdated logo from an image.
Summarize
- A production assistant can create a highlight video based on hours of event footage.
- A business analyst can create a Venn diagram that summarizes key points from an executive’s presentation.
Answer questions
- Employees of a manufacturing company can ask a generative AI-based “virtual expert” technical questions about operating procedures.
- A consumer can ask a chatbot questions about how to assemble a new piece of furniture.
Draft
- A software developer can prompt generative AI to create entire lines of code or suggest ways to complete partial lines of existing code.
- A marketing manager can use generative AI to draft various versions of campaign messaging.
As the technology evolves and matures, these kinds of generative AI can be increasingly integrated into enterprise workflows to automate tasks and directly perform specific actions (for example, automatically sending summary notes at the end of meetings). We already see tools emerging in this area.
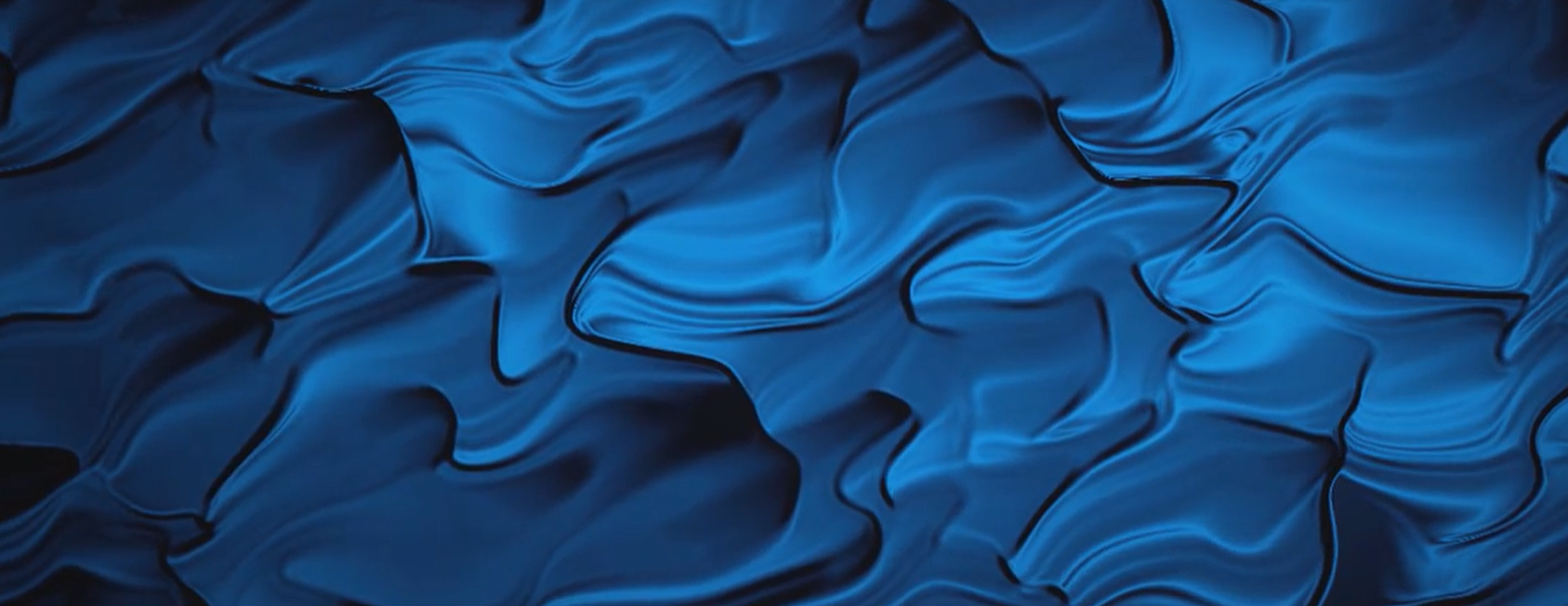
How generative AI differs from other kinds of AI
As the name suggests, the primary way in which generative AI differs from previous forms of AI or analytics is that it can generate new content, often in “unstructured” forms (for example, written text or images) that aren’t naturally represented in tables with rows and columns (see sidebar “Glossary” for a list of terms associated with generative AI).
The underlying technology that enables generative AI to work is a class of artificial neural networks called foundation models. Artificial neural networks are inspired by the billions of neurons that are connected in the human brain. They are trained using deep learning, a term that alludes to the many (deep) layers within neural networks. Deep learning has powered many of the recent advances in AI.
However, some characteristics set foundation models apart from previous generations of deep learning models. To start, they can be trained on extremely large and varied sets of unstructured data. For example, a type of foundation model called a large language model can be trained on vast amounts of text that is publicly available on the internet and covers many different topics. While other deep learning models can operate on sizable amounts of unstructured data, they are usually trained on a more specific data set. For example, a model might be trained on a specific set of images to enable it to recognize certain objects in photographs.
In fact, other deep learning models often can perform only one such task. They can, for example, either classify objects in a photo or perform another function such as making a prediction. In contrast, one foundation model can perform both of these functions and generate content as well. Foundation models amass these capabilities by learning patterns and relationships from the broad training data they ingest, which, for example, enables them to predict the next word in a sentence. That’s how ChatGPT can answer questions about varied topics and how DALL·E 2 and Stable Diffusion can produce images based on a description.
Given the versatility of a foundation model, companies can use the same one to implement multiple business use cases, something rarely achieved using earlier deep learning models. A foundation model that has incorporated information about a company’s products could potentially be used both for answering customers’ questions and for supporting engineers in developing updated versions of the products. As a result, companies can stand up applications and realize their benefits much faster.
However, because of the way current foundation models work, they aren’t naturally suited to all applications. For example, large language models can be prone to “hallucination,” or answering questions with plausible but untrue assertions (see sidebar “Using generative AI responsibly”). Additionally, the underlying reasoning or sources for a response are not always provided. This means companies should be careful of integrating generative AI without human oversight in applications where errors can cause harm or where explainability is needed. Generative AI is also currently unsuited for directly analyzing large amounts of tabular data or solving advanced numerical-optimization problems. Researchers are working hard to address these limitations.
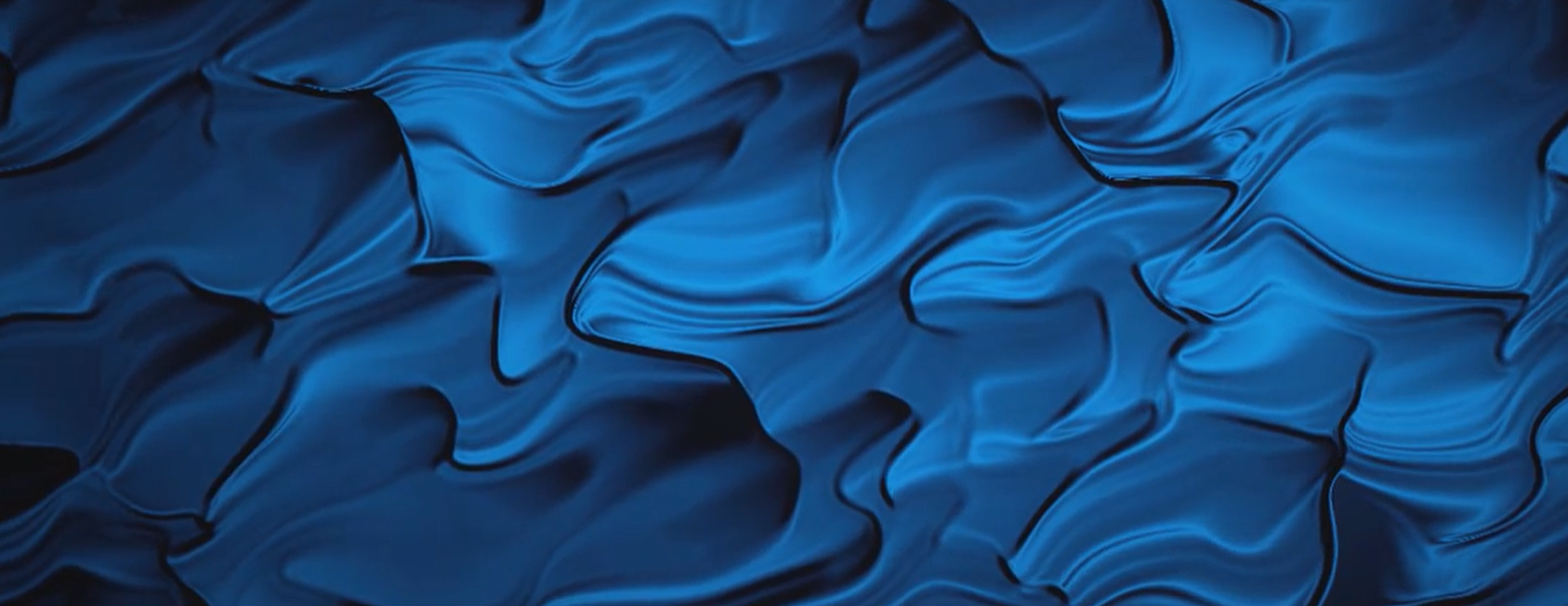
The emerging generative AI ecosystem
While foundation models serve as the “brain” of generative AI, an entire value chain is emerging to support the training and use of this technology (Exhibit 2). Specialized hardware provides the extensive compute power needed to train the models. Cloud platforms offer the ability to tap this hardware. MLOps and model hub providers offer the tools, technologies, and practices an organization needs to adapt a foundation model and deploy it within its end-user applications. Many companies are entering the market to offer applications built on top of foundation models that enable them to perform a specific task, such as helping a company’s customers with service issues.
The first foundation models required high levels of investment to develop, given the substantial computational resources required to train them and the human effort required to refine them. As a result, they were developed primarily by a few tech giants, start-ups backed by significant investment, and some open-source research collectives (for example, BigScience). However, work is under way on both smaller models that can deliver effective results for some tasks and training that’s more efficient. This could eventually open the market to more entrants. Some start-ups have already succeeded in developing their own models-for example, Cohere, Anthropic, and AI21 Labs build and train their own large language models.
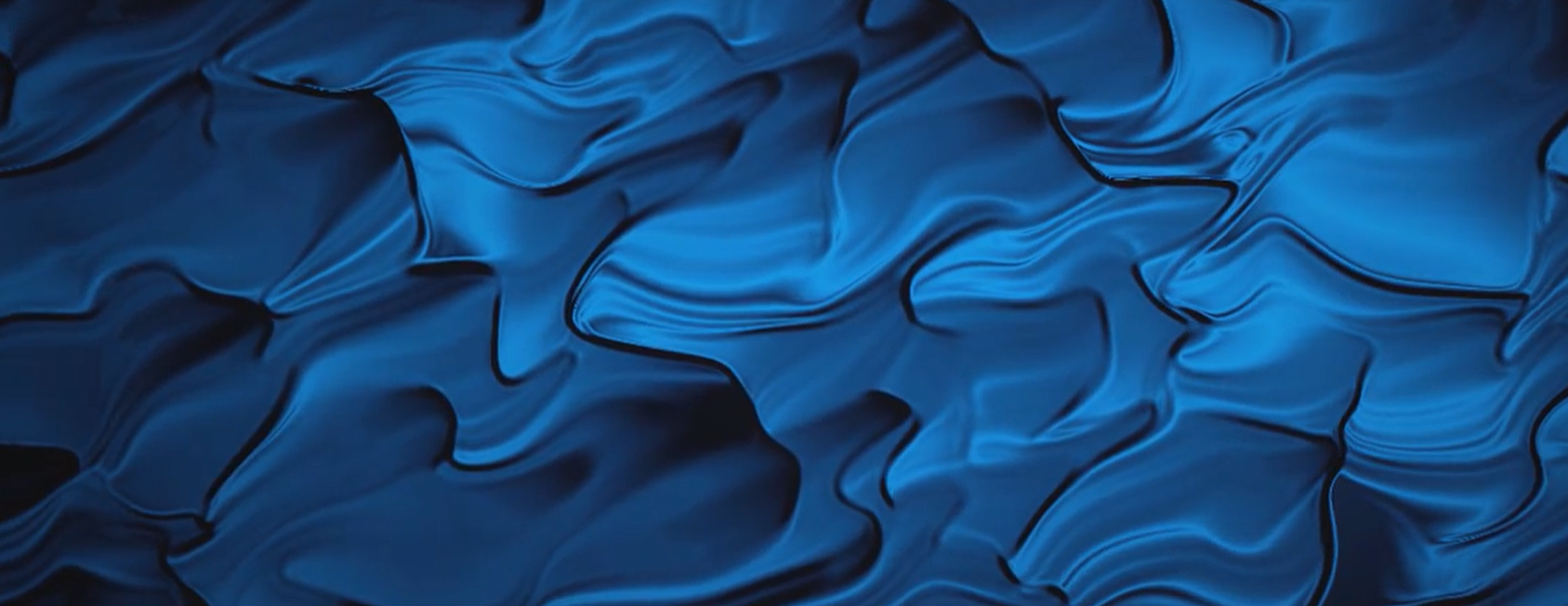
Putting generative AI to work
CEOs should consider exploration of generative AI a must, not a maybe. Generative AI can create value in a wide range of use cases. The economics and technical requirements to start are not prohibitive, while the downside of inaction could be quickly falling behind competitors. Each CEO should work with the executive team to reflect on where and how to play. Some CEOs may decide that generative AI presents a transformative opportunity for their companies, offering a chance to reimagine everything from research and development to marketing and sales to customer operations. Others may choose to start small and scale later. Once the decision is made, there are technical pathways that AI experts can follow to execute the strategy, depending on the use case.
Much of the use (although not necessarily all of the value) from generative AI in an organization will come from workers employing features embedded in the software they already have. Email systems will provide an option to write the first drafts of messages. Productivity applications will create the first draft of a presentation based on a description. Financial software will generate a prose description of the notable features in a financial report. Customer-relationship-management systems will suggest ways to interact with customers. These features could accelerate the productivity of every knowledge worker.
But generative AI can also be more transformative in certain use cases. Following, we look at four examples of how companies in different industries are using generative AI today to reshape how work is done within their organization. The examples range from those requiring minimal resources to resource-intensive undertakings. (For a quick comparison of these examples and more technical detail, see Exhibit 3.)
Exhibit 3
The organizational requirements for generative AI range from low to high, depending on the use case.
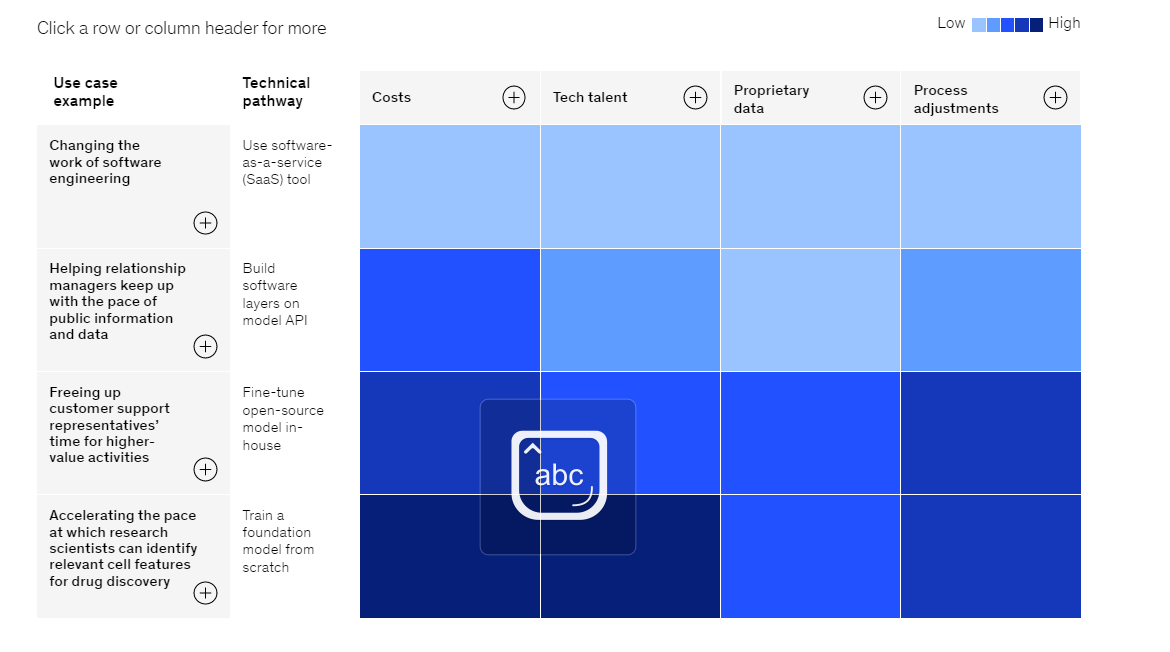
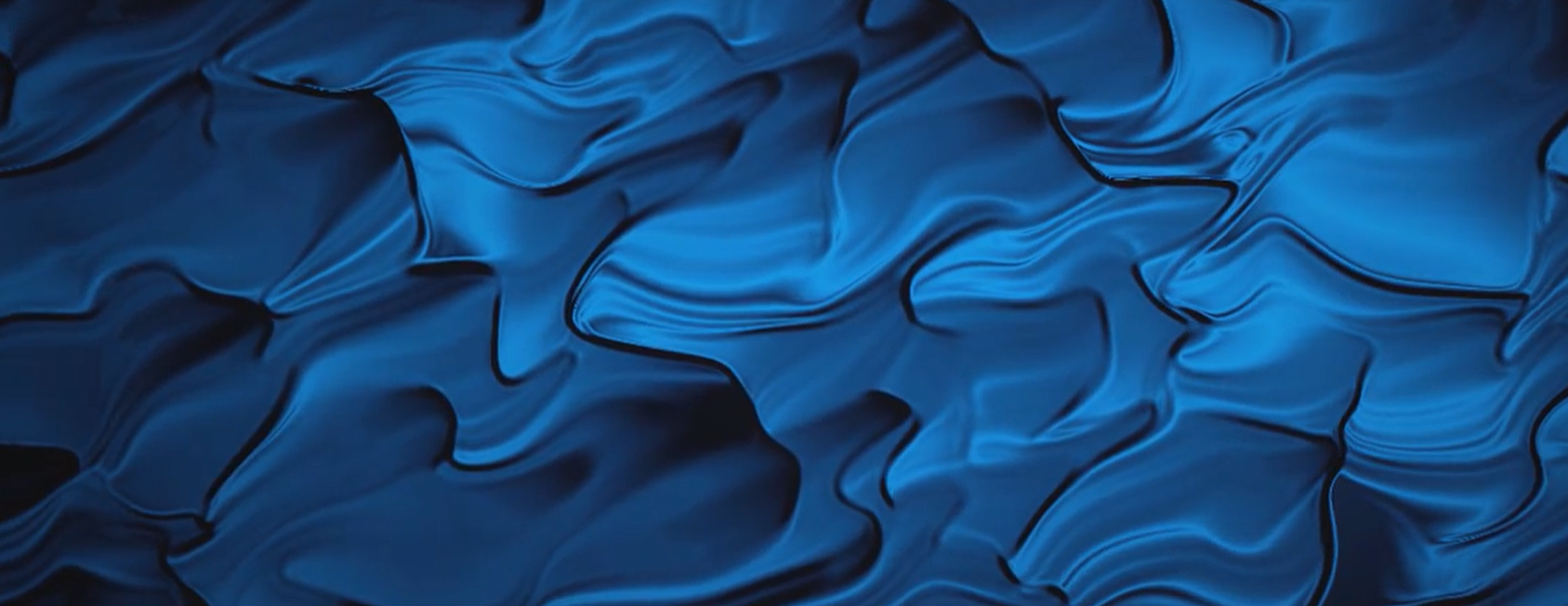
Use Cases (see the full version of the publication)
- Changing the work of software engineering
- Helping relationship managers keep up with the pace of public information and data
- Freeing up customer support representatives for higher-value activities
- Accelerating drug discovery
Lessons CEOs can take away from these examples
The use cases outlined here offer powerful takeaways for CEOs as they embark on the generative AI journey:
- Transformative use cases that offer practical benefits for jobs and the workplace already exist. Companies across sectors, from pharmaceuticals to banking to retail, are standing up a range of use cases to capture value creation potential. Organizations can start small or large, depending on their aspiration.
- Costs of pursuing generative AI vary widely, depending on the use case and the data required for software, cloud infrastructure, technical expertise, and risk mitigation. Companies must take into account risk issues, regardless of use case, and some will require more resources than others.
- While there is merit to getting started fast, building a basic business case first will help companies better navigate their generative AI journeys.
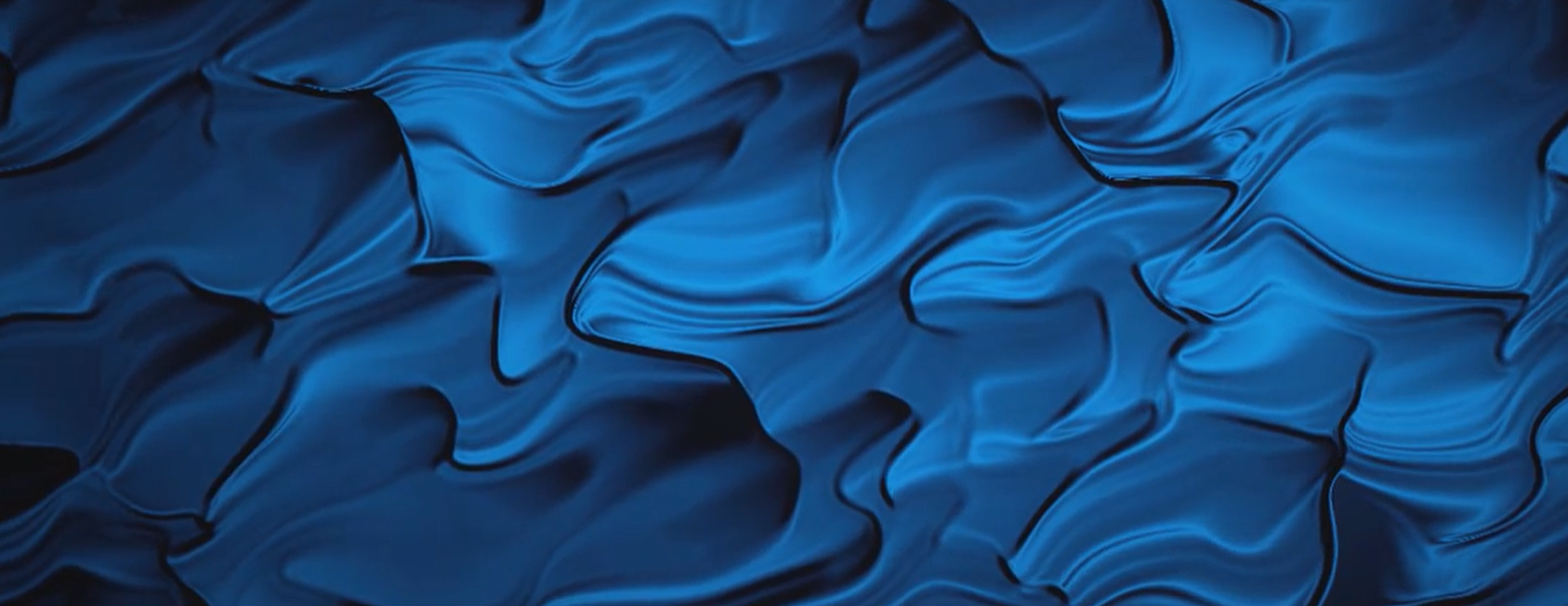
Considerations for getting started
The CEO has a crucial role to play in catalyzing a company’s focus on generative AI. In this closing section, we discuss strategies that CEOs will want to keep in mind as they begin their journey. Many of them echo the responses of senior executives to previous waves of new technology. However, generative AI presents its own challenges, including managing a technology moving at a speed not seen in previous technology transitions.
1.Organizing for generative AI
Many organizations began exploring the possibilities for traditional AI through siloed experiments. Generative AI requires a more deliberate and coordinated approach given its unique risk considerations and the ability of foundation models to underpin multiple use cases across an organization. For example, a model fine-tuned using proprietary material to reflect the enterprise’s brand identity could be deployed across several use cases (for example, generating personalized marketing campaigns and product descriptions) and business functions, such as product development and marketing.
To that end, we recommend convening a cross-functional group of the company’s leaders (for example, representing data science, engineering, legal, cybersecurity, marketing, design, and other business functions). Such a group can not only help identify and prioritize the highest-value use cases but also enable coordinated and safe implementation across the organization.
2.Reimagining end-to-end domains versus focusing on use cases
Generative AI is a powerful tool that can transform how organizations operate, with particular impact in certain business domains within the value chain (for example, marketing for a retailer or operations for a manufacturer). The ease of deploying generative AI can tempt organizations to apply it to sporadic use cases across the business. It is important to have a perspective on the family of use cases by domain that will have the most transformative potential across business functions. Organizations are reimagining the target state enabled by generative AI working in sync with other traditional AI applications, along with new ways of working that may not have been possible before.
3.Enabling a fully loaded technology stack
A modern data and tech stack is key to nearly any successful approach to generative AI. CEOs should look to their chief technology officers to determine whether the company has the required technical capabilities in terms of computing resources, data systems, tools, and access to models (open source via model hubs or commercial via APIs).
For example, the lifeblood of generative AI is fluid access to data honed for a specific business context or problem. Companies that have not yet found ways to effectively harmonize and provide ready access to their data will be unable to fine-tune generative AI to unlock more of its potentially transformative uses. Equally important is to design a scalable data architecture that includes data governance and security procedures. Depending on the use case, the existing computing and tooling infrastructure (which can be sourced via a cloud provider or set up in-house) might also need upgrading. A clear data and infrastructure strategy anchored on the business value and competitive advantage derived from generative AI will be critical.
4.Building a ‘lighthouse’
CEOs will want to avoid getting stuck in the planning stages. New models and applications are being developed and released rapidly. GPT-4, for example, was released in March 2023, following the release of ChatGPT (GPT-3.5) in November 2022 and GPT-3 in 2020. In the world of business, time is of the essence, and the fast-paced nature of generative AI technology demands that companies move quickly to take advantage of it. There are a few ways executives can keep moving at a steady clip.
Although generative AI is still in the early days, it’s important to showcase internally how it can affect a company’s operating model, perhaps through a “lighthouse approach.” For example, one way forward is building a “virtual expert” that enables frontline workers to tap proprietary sources of knowledge and offer the most relevant content to customers. This has the potential to increase productivity, create enthusiasm, and enable an organization to test generative AI internally before scaling to customer-facing applications.
As with other waves of technical innovation, there will be proof-of-concept fatigue and many examples of companies stuck in “pilot purgatory.” But encouraging a proof of concept is still the best way to quickly test and refine a valuable business case before scaling to adjacent use cases. By focusing on early wins that deliver meaningful results, companies can build momentum and then scale out and up, leveraging the multipurpose nature of generative AI. This approach could enable companies to promote broader AI adoption and create the culture of innovation that is essential to maintaining a competitive edge. As outlined above, the cross-functional leadership team will want to make sure such proofs of concept are deliberate and coordinated.
5.Balancing risk and value creation
As our four detailed use cases demonstrate, business leaders must balance value creation opportunities with the risks involved in generative AI. According to our recent Global AI Survey, most organizations don’t mitigate most of the risks associated with traditional AI, even though more than half of organizations have already adopted the technology. Generative AI brings renewed attention to many of these same risks, such as the potential to perpetuate bias hidden in training data, while presenting new ones, such as its propensity to hallucinate.
As a result, the cross-functional leadership team will want to not only establish overarching ethical principles and guidelines for generative AI use but also develop a thorough understanding of the risks presented by each potential use case. It will be important to look for initial use cases that both align with the organization’s overall risk tolerance and have structures in place to mitigate consequential risk. For example, a retail organization might prioritize a use case that has slightly lower value but also lower risk-such as creating initial drafts of marketing content and other tasks that keep a human in the loop. At the same time, the company might set aside a higher-value, high-risk use case such as a tool that automatically drafts and sends hyperpersonalized marketing emails. Such risk-forward practices can enable organizations to establish the controls necessary to properly manage generative AI and maintain compliance.
CEOs and their teams will also want to stay current with the latest developments in generative AI regulation, including rules related to consumer data protection and intellectual property rights, to protect the company from liability issues. Countries may take varying approaches to regulation, as they often already do with AI and data. Organizations may need to adapt their working approach to calibrate process management, culture, and talent management in a way that ensures they can handle the rapidly evolving regulatory environment and risks of generative AI at scale.
6.Applying an ecosystem approach to partnerships
Business leaders should focus on building and maintaining a balanced set of alliances. A company’s acquisitions and alliances strategy should continue to concentrate on building an ecosystem of partners tuned to different contexts and addressing what generative AI requires at all levels of the tech stack, while being careful to prevent vendor lock-in.
Partnering with the right companies can help accelerate execution. Organizations do not have to build out all applications or foundation models themselves. Instead, they can partner with generative AI vendors and experts to move more quickly. For instance, they can team up with model providers to customize models for a specific sector, or partner with infrastructure providers that offer support capabilities such as scalable cloud computing.
Companies can use the expertise of others and move quickly to take advantage of the latest generative AI technology. But generative AI models are just the tip of the spear: multiple additional elements are required for value creation.
7.Focusing on required talent and skills
To effectively apply generative AI for business value, companies need to build their technical capabilities and upskill their current workforce. This requires a concerted effort by leadership to identify the required capabilities based on the company’s prioritized use cases, which will likely extend beyond technical roles to include a talent mix across engineering, data, design, risk, product, and other business functions.
As demonstrated in the use cases highlighted above, technical and talent needs vary widely depending on the nature of a given implementation-from using off-the-shelf solutions to building a foundation model from scratch. For example, to build a generative model, a company may need PhD-level machine learning experts; on the other hand, to develop generative AI tools using existing models and SaaS offerings, a data engineer and a software engineer may be sufficient to lead the effort.
In addition to hiring the right talent, companies will want to train and educate their existing workforces. Prompt-based conversational user interfaces can make generative AI applications easy to use. But users still need to optimize their prompts, understand the technology’s limitations, and know where and when they can acceptably integrate the application into their workflows. Leadership should provide clear guidelines on the use of generative AI tools and offer ongoing education and training to keep employees apprised of their risks. Fostering a culture of self-driven research and experimentation can also encourage employees to innovate processes and products that effectively incorporate these tools.
Conclusion
Businesses have been pursuing AI ambitions for years, and many have realized new revenue streams, product improvements, and operational efficiencies. Much of the successes in these areas have stemmed from AI technologies that remain the best tool for a particular job, and businesses should continue scaling such efforts. However, generative AI represents another promising leap forward and a world of new possibilities. While the technology’s operational and risk scaffolding is still being built, business leaders know they should embark on the generative AI journey. But where and how should they start? The answer will vary from company to company as well as within an organization. Some will start big; others may undertake smaller experiments. The best approach will depend on a company’s aspiration and risk appetite. Whatever the ambition, the key is to get under way and learn by doing.
Originally published at https://www.mckinsey.com on May 12, 2023.
APPENDIX
Using generative AI responsibly
Generative AI poses a variety of risks. CEOs will want to design their teams and processes to mitigate those risks from the start — not only to meet fast-evolving regulatory requirements but also to protect their business and earn consumers’ digital trust (we offer recommendations on how to do so later in this article).1
Fairness: Models may generate algorithmic bias due to imperfect training data or decisions made by the engineers developing the models.
Intellectual property (IP): Training data and model outputs can generate significant IP risks, including infringing on copyrighted, trademarked, patented, or otherwise legally protected materials. Even when using a provider’s generative AI tool, organizations will need to understand what data went into training and how it’s used in tool outputs.
Privacy: Privacy concerns could arise if users input information that later ends up in model outputs in a form that makes individuals identifiable. Generative AI could also be used to create and disseminate malicious content such as disinformation, deepfakes, and hate speech.
Security: Generative AI may be used by bad actors to accelerate the sophistication and speed of cyberattacks. It also can be manipulated to provide malicious outputs. For example, through a technique called prompt injection, a third party gives a model new instructions that trick the model into delivering an output unintended by the model producer and end user.
Explainability: Generative AI relies on neural networks with billions of parameters, challenging our ability to explain how any given answer is produced.
Reliability: Models can produce different answers to the same prompts, impeding the user’s ability to assess the accuracy and reliability of outputs.
Organizational impact: Generative AI may significantly affect the workforce, and the impact on specific groups and local communities could be disproportionately negative.
Social and environmental impact: The development and training of foundation models may lead to detrimental social and environmental consequences, including an increase in carbon emissions (for example, training one large language model can emit about 315 tons of carbon dioxide).2
NAMES MENTIONED
Cohere, Anthropic, and AI21 Labs