What is the message?
Exploring the vast potential of big data across key economic sectors and its transformative impact on productivity, quality of care, and industry competitiveness, particularly in US healthcare, with an estimated annual value creation exceeding $300 billion and significant implications for stakeholders across the sector
Excerpt from Manyika, J., et al. (2011) Big Data: The Next Frontier for Innovation, Competition, and Productivity, Chapter 3 — The transformative potential of big data in five domains, San Francisco, MGI, CA, USA.
EXECUTIVE SUMMARY
What are the key points?
Domains Studied: Healthcare in the US, public sector administration in the EU, retail in the US, global manufacturing, and global personal location data.
Economic Impact: These domains represented close to 40% of global GDP in 2010.
Data Utilization: Each sector varies in sophistication and maturity in using big data.
Healthcare Focus: Multiple stakeholders in the US healthcare system generate fragmented pools of data.
Public Sector: Governments have large pools of data but have underutilized them.
Retail: Sector has been using big data for customer segmentation and supply chain management, with significant potential for expansion.
Manufacturing: Complex value chains and substantial data availability provide opportunities across the product lifecycle.
Personal Location Data: Rapid growth due to smartphone adoption, with potential for significant consumer surplus.
What are the key examples?
US Department of Veterans Affairs (VA): Demonstrated success with health IT and remote patient monitoring, outperforming the private sector in adherence to clinical guidelines and drug therapy.
Kaiser Permanente: Early integration of clinical and cost data led to the discovery of Vioxx’s adverse effects.
UK National Health Service (NICE): Uses large clinical datasets to assess the cost-effectiveness of new drugs.
Italian Medicines Agency: Analyzes clinical data for cost-effectiveness, imposing conditional reimbursement on new drugs.
What are the key statistics?
Value Creation in US Healthcare: Over $300 billion annually, with two-thirds from reduced national healthcare expenditure.
Potential Productivity Increase: 0.7% annual productivity growth in the US healthcare sector.
Data Fragmentation: As much as 30% of clinical data in the US is not yet digitized.
Sector-Wide Data: The majority of clinical data are still held by individual providers and not shared.
Conclusion
Big data has the transformative potential to significantly enhance productivity, quality of care, and competitiveness across various economic sectors.
In US healthcare alone, the effective use of big data could generate over $300 billion annually, primarily through cost reductions and efficiency gains.
The realization of this potential depends on the alignment of incentives, policy support, and overcoming structural barriers.
Early success stories from organizations like the VA and Kaiser Permanente illustrate the tangible benefits of leveraging big data, underscoring the need for broader adoption and integration across the industry.
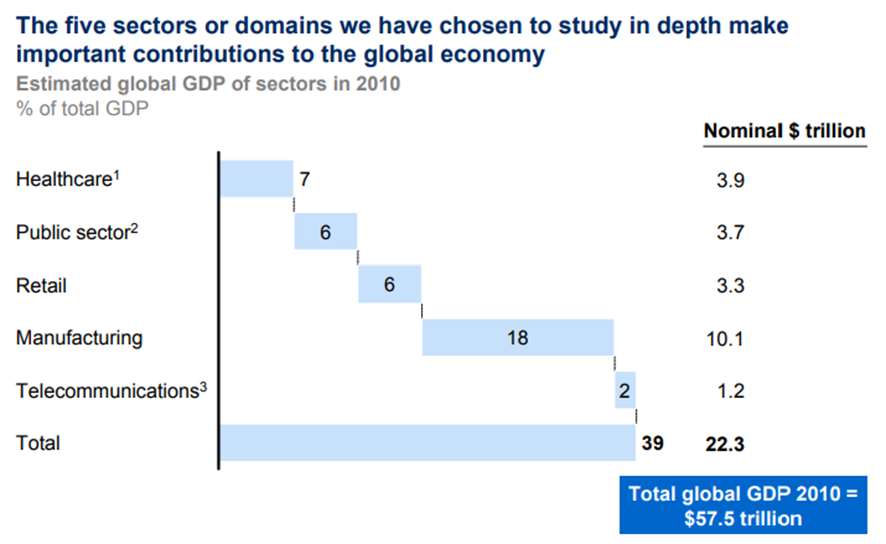
SOURCE: Global Insight; McKinsey Global Institute analysis
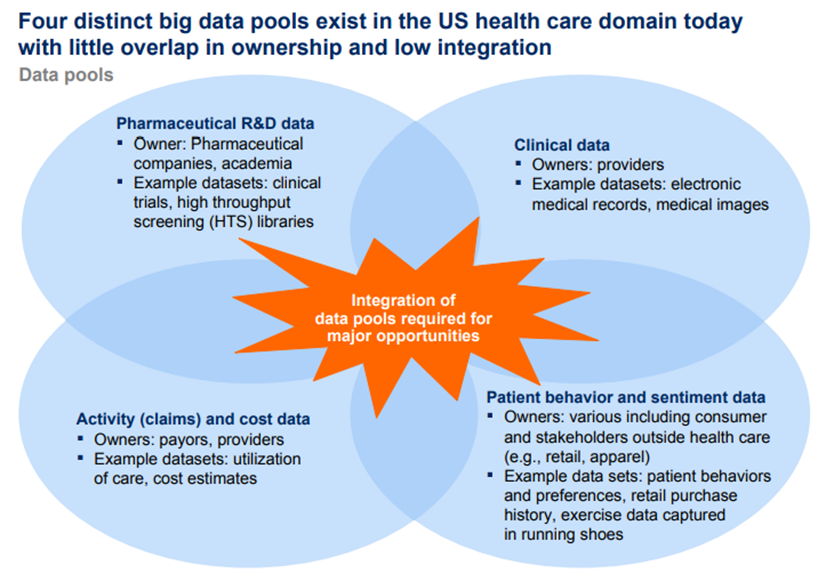
SOURCE: McKinsey Global Institute analysis
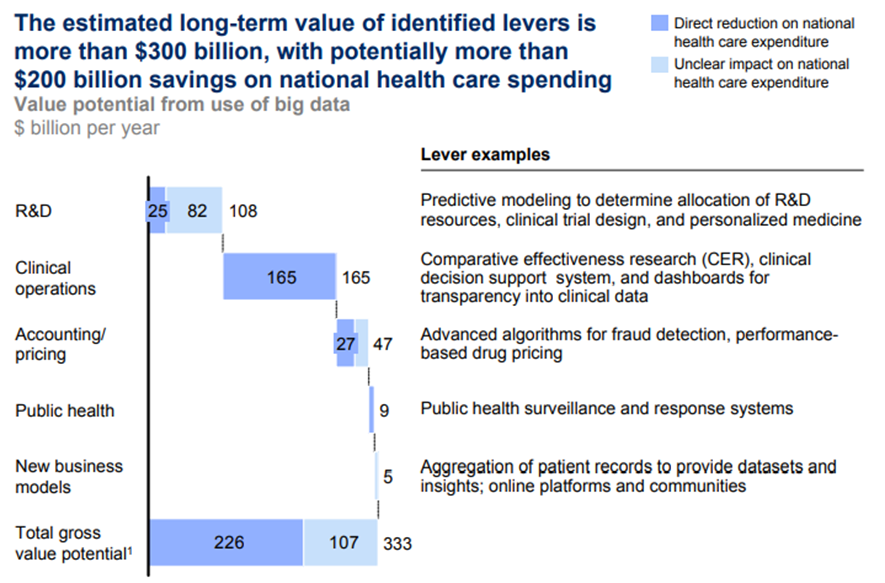
SOURCE: Expert interviews; press and literature search; McKinsey Global Institute analysis
DEEP DIVE
Health care (United States)
Reforming the US health care system to reduce the rate at which costs have been increasing while sustaining its current strengths is critical to the United States both as a society and as an economy.
Health care, one of the largest sectors of the US economy, accounts for slightly more than 17 percent of GDP and employs an estimated 11 percent of the country’s workers.
It is becoming clear that the historic rate of growth of US health care expenditures, increasing annually by nearly 5 percent in real terms over the last decade, is unsustainable and is a major contributor to the high national debt levels projected to develop over the next two decades.
An aging US population and the emergence of new, more expensive treatments will amplify this trend.
Thus far, health care has lagged behind other industries in improving operational performance and adopting technology-enabled process improvements. The magnitude of the problem and potentially long timelines for implementing change make it imperative that decisive measures aimed at increasing productivity begin in the near term to ease escalating cost pressures.
It is possible to address these challenges by emulating and implementing best practices in health care, pioneered in the United States and in other countries. Doing so will often require the analysis of large datasets.
MGI studied the health care sector in the United States, where we took an expansive view to include the provider, payor, and pharmaceutical and medical products (PMP) subsectors to understand how big data can help to improve the effectiveness and efficiency of health care as an entire system. Some of the actions that can help stem the rising costs of US health care while improving its quality don’t necessarily require big data.
These include, for example, tackling major underlying issues such as the high incidence and costs of lifestyle and behavior-induced disease, minimizing any economic distortion between consumers and providers, and reducing the administrative complexity in payors.[2]
However, the use of large datasets underlies another set of levers that have the potential to play a major role in more effective and cost-saving care initiatives, the emergence of better products and services, and the creation of new business models in health care and its associated industries.
But deploying big data in these areas would need to be accompanied by a range of enablers, some of which would require a substantial rethinking of the way health care is provided and funded.
Our estimates of the potential value that big data can create in health care are therefore not predictions of what will happen but our view on the full economic potential, assuming that required IT and dataset investments, analytical capabilities, privacy protections, and appropriate economic incentives are put in place.
With this caveat, we estimate that in about ten years, there is an opportunity to capture more than $300 billion annually in new value, with two-thirds of that in the form of reductions to national health care expenditure — about 8 percent of estimated health care spending at 2010 levels.
US HEALTH CARE COSTS ARE OUTPACING ECONOMIC GROWTH
The United States spends more per person on health care than any other nation in the world — without obvious evidence of better outcomes. Over the next decade, average annual health spending growth is expected to outpace average annual growth in GDP by almost 2 percentage points.[3]
Available evidence suggests that a substantial share of US spending on health care contributes little to better health outcomes. Multiple studies have found that the United States spends about 30 percent more on care than the average Organisation for Economic Co-operation and Development (OECD) country when adjusted for per capita GDP and relative wealth.[4]
Yet the United States still falls below OECD averages on such health care parameters as average life expectancy and infant mortality. The additional spending above OECD trends totals an estimated $750 billion a year out of a national health budget in 2007 of $2.24 trillion — that’s about $2,500 per person per year (Exhibit 13). Age, disease burden, and health outcomes cannot account for the significant difference.
Exhibit 13
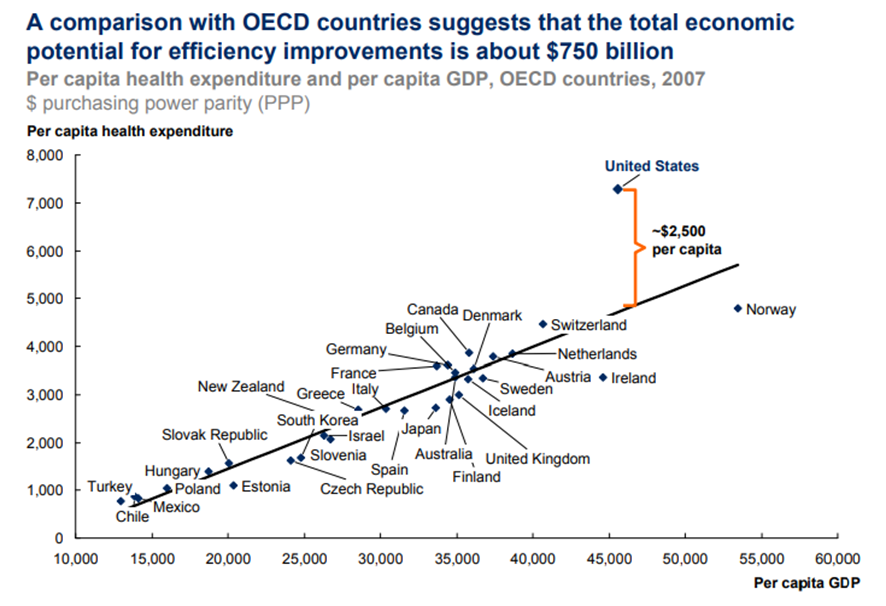
SOURCE: Organisation for Economic Co-operation and Development (OECD)
The current reimbursement system does not create incentives for doctors, hospitals, and other providers of health care — or even their patients — to optimize efficiency or control costs.
As currently constructed, the system generally pays for procedures without regard to their effectiveness and necessity.
Significantly slowing the growth of health care spending will require fundamental changes in today’s incentives.
Examples of integrated care models in the United States and beyond demonstrate that, when incentives are aligned and the necessary enablers are in place, the impact of leveraging big data can be very significant (see Box 6, “Health care systems in the United States and beyond have shown early success in their use of big data”).
US HEALTH CARE HAS FOUR MAIN POOLS OF DATA
The US health care system has four major pools of data within health care, each primarily held by a different constituency. Data are highly fragmented in this domain.
The four pools are provider clinical data, payor activity (claims) and cost data, pharmaceutical and medical products R&D data, and patient behavior and sentiment data (Exhibit 14).
The amount of data that is available, collected, and analyzed varies widely within the sector.
For instance, health providers usually have extensively digitized financial and administrative data, including accounting and basic patient information.
In general, however, providers are still at an early stage in digitizing and aggregating clinical data covering such areas as the progress and outcomes of treatments.
Exhibit 14
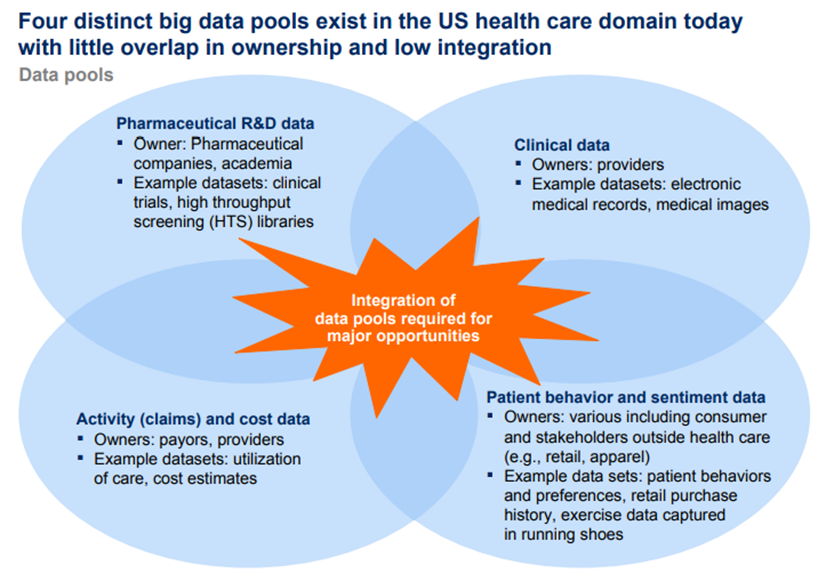
SOURCE: McKinsey Global Institute analysis
Depending on the care setting, we estimate that as much as 30 percent of clinical text/numerical data in the United States, including medical records, bills, and laboratory and surgery reports, is still not generated electronically.
Even when clinical data are in digital form, they are usually held by an individual provider and rarely shared.
Indeed, the majority of clinical data actually generated are in the form of video and monitor feeds, which are used in real time and not stored.
There is a strong political push in the United States to deploy electronic health records (EHR) — sometimes referred to as electronic medical records (EMR) — more widely in provider settings.
The American Recovery and Reinvestment Act of 2009 included $20 billion in stimulus funding over five years to encourage the meaningful use of EHR by physicians and hospitals.
Outcomes-based reimbursement plans could also encourage the deployment of EHR because they would require accurate and complete databases and analytical tools to measure outcomes.
Payors, meanwhile, have been capturing activity (claims) and cost data digitally for many years. Nevertheless, the information is not generally in a form that payors can use for the kind of advanced analysis necessary to generate real insights because it is rarely standardized, often fragmented, or generated in legacy IT systems with incompatible formats.
The PMP subsector is arguably the most advanced in the digitization and use of data in the health care sector. PMP captures R&D data digitally and already analyzes them extensively.
Additional opportunities could come from combining PMP data with other datasets such as genomics or proteomics data for personal medicine, or clinical datasets from providers to identify expanded applications and adverse effects.
In addition to clinical, activity (claims) cost data, and pharmaceutical R&D datasets, there is an emerging pool of data related to patient behavior (e.g., propensity to change lifestyle behavior) and sentiment (e.g., from social media) that is potentially valuable but is not held by the health care sector.
Patient behavior and sentiment data could be used to influence adherence to treatment regimes, affect lifestyle factors, and influence a broad range of wellness activities.
Many of the levers we identify in the next section involve the integration of multiple data pools.
It will be imperative for organizations, and possibly policy makers, to figure out how to align economic incentives and overcome technology barriers to enable the sharing of data.
US HEALTH CARE CAN TAP MULTIPLE BIG DATA LEVERS
We have identified a set of 15 levers in five broad categories that have the potential to improve the efficiency and effectiveness of the health care sector by exploiting the tremendous amount of electronic information that is, and could become, available throughout the US health care sector.
Where possible, we estimate the financial potential of deploying these levers in the form of cost savings, increased efficiencies, improved treatment effectiveness, and productivity enhancement.
We have arrived at these estimates by referring to international and US best practices (see the appendix for more details).
Assuming that the US health care system removes structural barriers and puts the right incentives in place for different stakeholders, we estimate that big data can help to unlock more than $300 billion a year in additional value throughout the sector.
The amount that the sector will capture in reality will depend on the collective actions of health care organizations and policy makers in overcoming structural and other barriers.
We focus on levers that require the analysis of large datasets that relate primarily to the development and provision of care, rather than all HIT levers such as automation in claims processing.
Our estimates do not aim to capture the entirety of the value generated by HIT (e.g., we exclude value that does not fundamentally require the analysis of large datasets, such as the time saved by doctors and nurses in transcribing notes through the use of EMR or the efficiency savings and increased access to care through mobile health).
To validate our findings, we have compared our estimates with those made by other researchers and found them to be comparable (see the appendix).
We divide the levers into five broad categories: clinical operations, payment/pricing, R&D, new business models, and public health. We now discuss each in turn:
BIG DATA CAN ENABLE MORE THAN $300 BILLION A YEAR IN VALUE CREATION IN US HEALTH CARE
All the big-data-enabled levers that we have described can play a substantial part in overhauling the productivity of the US health care system, improving the quality of care and treatment, enhancing patients’ experience, boosting industry competitiveness, and creating a range of fresh business models and services.
In total, we estimate that US health care could capture more than $300 billion in value every year, with two-thirds of that in the form of reductions to national health care expenditure of around 8 percent.
Holding health care outcomes constant (a conservative assumption considering that many of the levers will improve health care quality, which is difficult to quantify), accounting for annual operating costs and assuming that the value potential of each big data lever grows linearly with health care expenditure, this would mean that the annual productivity of the US health care sector could grow by an additional 0.7 percent.
This productivity boost assumes that the industry realizes all of the potential benefits from the use of big data over the next ten years (Exhibit 15).43 As we have said, the actual amount of value that the sector will capture will depend on the collective actions of health care organizations and policy makers in overcoming structural and other barriers.
The benefits of these dramatic potential improvements would flow to patients, providers, payors, and the PMP sector. However, using these levers would also redistribute value among players throughout the industry as revenue and profits shift and new, imaginative leaders emerge.
Exhibit 15
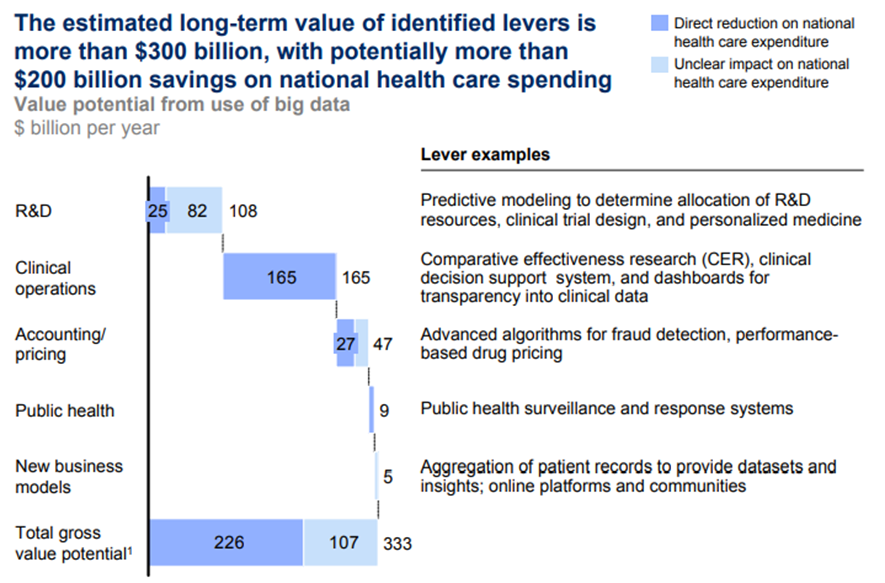
SOURCE: Expert interviews; press and literature search; McKinsey Global Institute analysis
A.Clinical operations
Within clinical operations are five big data levers that mainly affect the way providers, payors, and PMP provide clinical care.
We estimate that, if fully employed, these five levers could reduce national health care expenditure by up to $165 billion a year from a base of $2.5 trillion in 2009.
· 1.Comparative effectiveness research
· 2.Clinical decision support systems.
· 3.Transparency about medical data
· 4.Remote patient monitoring.
· 5.Advanced analytics applied to patient profiles.
1.Comparative effectiveness research.
Outcomes-based research determines which treatments will work best for specific patients (“optimal treatment pathways”) by analyzing comprehensive patient and outcome data to compare the effectiveness of various interventions.
This research includes what is known as comparative effectiveness research (CER). Many studies have shown that wide variations exist in health care practices, outcomes, and costs across different providers, geographies, and patients.
Critically analyzing large datasets that include patient characteristics and the cost and outcomes of treatments can help to identify the most clinically effective and cost-effective treatments to apply.
If the health care system implements CER, there is potential to reduce incidences of overtreatment — i.e., interventions that do more harm than good — and undertreatment — cases in which a specific therapy should have been prescribed but was not.
Both overtreatment and undertreatment result in worse patient outcomes and higher health care costs in the long run.
Around the world, agencies such as NICE in the United Kingdom, the Institut für Qualität und Wirtschaftlichkeit im Gesundheitswesen (Institute for Quality and Efficiency in Health Care, or IQWIG) in Germany, the Common Drug Review in Canada, and Australia’s Pharmaceutical Benefits Scheme have begun CER programs with successful results.
The United States took a first step in this direction through the American Recovery and Reinvestment Act of 2009. The law created the Federal Coordinating Council for Comparative Effectiveness Research, which, as its name implies, coordinates comparative effectiveness research across the federal government and makes recommendations for the $400 million allocated for CER.
If this lever is to achieve systemwide scale in the United States, it needs to overcome some significant barriers. Comprehensive and consistent clinical and claims datasets need to be captured, integrated, and made available to researchers, and a number of potential issues need to be negotiated.
For example, in the current rush to deploy EHR, a potential lack of standards and interoperability could make it difficult to combine datasets.
Another concern is how to ensure patient privacy while still providing sufficiently detailed data to allow effective analyses.
Having identified optimal treatment pathways, payors will need to be allowed to tie reimbursement decisions and the design of benefits to the results of this research.
However, current US law prohibits the Centers for Medicare and Medicaid Services from using the cost/ benefit ratio for reimbursement decisions.
Disseminating knowledge about the most effective treatments to medical professionals will require the introduction or upgrade of tools, including clinical decision support systems (see the next lever), so that physicians can receive recommendations of best practices at the point of actual decision making about treatments.
2.Clinical decision support systems.
The second lever is deploying clinical decision support systems for enhancing the efficiency and quality of operations.
These systems include computerized physician order-entry capabilities.
The current generation of such systems analyzes physician entries and compares them against medical guidelines to alert for potential errors such as adverse drug reactions or events.
By deploying these systems, providers can reduce adverse reactions and lower treatment error rates and liability claims, especially those arising from clinical mistakes.
In one particularly powerful study conducted at a pediatric critical care unit in a major US metropolitan area, a clinical decision support system tool cut adverse drug reactions and events by 40 percent in just two months.[5]
In the future, big data systems such as these can become substantially more intelligent by including modules that use image analysis and recognition in databases of medical images (X-ray, CT, MRI) for prediagnosis or that automatically mine medical literature to create a medical expertise database capable of suggesting treatment options to physicians based on patients’ medical records.
In addition, clinical decision support systems can enable a larger portion of work to flow to nurse practitioners and physician assistants by automating and facilitating the physician advisory role and thereby improving the efficiency of patient care.
3.Transparency about medical data.
The third clinical big data lever is analyzing data on medical procedures and creating transparency around those data both to identify performance opportunities for medical professionals, processes, and institutions and to help patients shop for the care that offers the best value.
Operational and performance datasets from provider settings can be analyzed to create process maps and dashboards enabling information transparency.
The goal is to identify and analyze sources of variability and waste in clinical processes and then optimize processes. Mapping processes and physical flows as well as “patient journeys” within an organization can help to reduce delays in the system.
Simply publishing cost, quality, and performance data, even without a tangible financial reward, often creates the competition that drives improvements in performance.
The operational streamlining resulting from these analyses can produce reduced costs through lean processes, additional revenue potential from freed-up capacity, more efficient staffing that matches demand, improved quality of care, and better patient experiences.
The Centers for Medicare and Medicaid Services is testing dashboards as part of an initiative to implement open government principles of transparency, public participation, and collaboration.
In the same spirit, the Centers for Disease Control and Prevention has begun publishing health data in an interactive format and providing advanced features for manipulating its pretabulated data.
Publishing quality and performance data can also help patients make more informed health care decisions compared with the situation today in which differences in cost and quality are largely opaque to them.[6]
Transparency about the data on cost and quality, along with appropriate reimbursement schemes (e.g., where patients’ out-of-pocket expenses are tied to the actual costs charged by the providers) will encourage patients to take a more value-conscious approach to consuming health care, which in turn will help make providers more competitive and ultimately improve the overall performance of the sector.
4.Remote patient monitoring.
The fourth clinical big data lever is collecting data from remote patient monitoring for chronically ill patients and analyzing the resulting data to monitor adherence (determining if patients are actually doing what was prescribed) and to improve future drug and treatment options.
An estimated 150 million patients in the United States in 2010 were chronically ill with diseases such as diabetes, congestive heart failure, and hypertension, and they accounted for more than 80 percent of health system costs that year.
Remote patient monitoring systems can be highly useful for treating such patients.
The systems include devices that monitor heart conditions, send information about blood-sugar levels, transmit feedback from caregivers, and even include “chipon-a-pill” technology — pharmaceuticals that act as instruments to report when they are ingested by a patient — that feeds data in near real time to electronic medical record databases.
Simply alerting a physician that a congestive heart failure patient is gaining weight because of water retention can prevent an emergency hospitalization.
More generally, the use of data from remote monitoring systems can reduce patient in-hospital bed days, cut emergency department visits, improve the targeting of nursing home care and outpatient physician appointments, and reduce long-term health complications.
5.Advanced analytics applied to patient profiles.
A fifth clinical operations big data lever is applying advanced analytics to patient profiles (e.g., segmentation and predictive modeling) to identify individuals who would benefit from proactive care or lifestyle changes.
For instance, these approaches can help identify patients who are at high risk of developing a specific disease (e.g., diabetes) and would benefit from a preventive care program.
These approaches can also enable the better selection of patients with a preexisting condition for inclusion in a disease-management program that best matches their needs. And, of course, patient data can provide an enhanced ability to measure the success of these programs, an exercise that poses a major challenge for many current preventive care programs.
B.Payment / Pricing
The two levers in this category mainly involve improving health care payment and pricing, and they focus primarily on payors’ operations.
Together, they have the potential to create $50 billion in value, half of which would result in cost savings to national health care expenditure.
· 1.Automated systems
· 2.Health Economics and Outcomes Research and performance-based pricing plans
1.Automated systems.
The first lever is implementing automated systems (e.g., machine learning techniques such as neural networks) for fraud detection and checking the accuracy and consistency of payors’ claims.
The US payor industry estimates that 2 to 4 percent of annual claims are fraudulent or unjustified; official estimates for Medicare and Medicaid range up to a 10 percent share.
Savings can be achieved through a comprehensive and consistent claims database (e.g., the proposed all-payors claims database) and trained algorithms to process and check claims for accuracy and to detect cases with a high likelihood of fraud, defects, or inaccuracy either retroactively or in real time.
When used in near real time, these automated systems can identify overpayments before payouts are made, recouping significant costs.
2.Health Economics and Outcomes Research and performance-based pricing plans.
The second lever is utilizing Health Economics and Outcomes Research and performance-based pricing plans based on real-world patient outcomes data to arrive at fair economic compensation, from drug prices paid to pharmaceutical companies to reimbursements paid to providers by payors.
In the case of drug pricing, pharmaceutical companies would share part of the therapeutic risk.
For payors, a key benefit is that cost- and risk-sharing schemes for new drugs enable controls or a cap on a significant part of health care spending.
At the same time, PMP companies could gain better market access in the presence of strong efforts to contain health care costs.
PMP companies can also secure potentially higher revenue from more efficient drug use through innovative pricing schemes.
Patients would obtain improved health outcomes with a value-based formulary and gain access to innovative drugs at reasonable costs.
To achieve maximum value for the health care system, the United States would need to allow collective bargaining by payors.
Several pharmaceutical pricing pilot programs based on Health Economics and Outcomes Research are in place, primarily in Europe.
Novartis, for example, agreed with German health insurers to cover costs in excess of €315 million ($468 million) per year for Lucentis, its drug for treating age-related macular degeneration.
Some payors are also measuring the costs and quality of providers and negotiating reimbursements based on the data.
For example, payors may exclude providers whose costs to treat different diseases are out of line after adjusting for comorbidities (the presence of one or more diseases in addition to a primary disease).
Alternatively, they may negotiate innovative pricing plans, such as outcome-based payments, if providers achieve specific quality and outcomes benchmarks.
C.R&D
Five big data levers could improve R&D productivity in the PMP subsector.
Together, these levers could create more than $100 billion in value, about $25 billion of which could be in the form of lower national health care expenditure.
· 1.Predictive modeling
· 2.Statistical tools and algorithms to improve clinical trial design.
· 3.Analyzing clinical trials data.
· 4.Personalized medicine.
· 5,Analyzing disease patterns.
1.Predictive modeling.
The first lever is the aggregation of research data so that PMP companies can perform predictive modeling for new drugs and determine the most efficient and cost-effective allocation of R&D resources.
This “rational drug design” means using simulations and modeling based on preclinical or early clinical datasets along the R&D value chain to predict clinical outcomes as promptly as possible.
The evaluation factors can include product safety, efficacy, potential side effects, and overall trial outcomes.
This predictive modeling can reduce costs by suspending research and expensive clinical trials on suboptimal compounds earlier in the research cycle.
The benefits of this lever for the PMP sector include lower R&D costs and earlier revenue from a leaner, faster, and more targeted R&D pipeline.
The lever helps to bring drugs to market faster and produce more targeted compounds with a higher potential market and therapeutic success rate.
Predictive modeling can shave 3 to 5 years off the approximately 13 years it can take to bring a new compound to market.
2.Statistical tools and algorithms to improve clinical trial design.
Another lever is using statistical tools and algorithms to improve the design of clinical trials and the targeting of patient recruitment in the clinical phases of the R&D process.
This lever includes mining patient data to expedite clinical trials by assessing patient recruitment feasibility, recommending more effective protocol designs, and suggesting trial sites with large numbers of potentially eligible patients and strong track records.
The techniques that can be employed include performing scenario simulations and modeling to optimize label size (the range of indications applicable to a given drug) to increase the probability of trial success rates.
Algorithms can combine R&D and trial data with commercial modeling and historic regulatory data to find the optimal trade-off between the size and characteristics of a targeted patient population for trials and the chances of regulatory approval of the new compound.
Analyses can also improve the process of selecting investigators by targeting those with proven performance records.
3.Analyzing clinical trials data.
A third R&D-related lever is analyzing clinical trials data and patient records to identify additional indications and discover adverse effects.
Drug repositioning, or marketing for additional indications, may be possible after the statistical analysis of large outcome datasets to detect signals of additional benefits.
Analyzing the (near) real-time collection of adverse case reports enables pharmacovigilance, surfacing safety signals too rare to appear in a typical clinical trial or, in some cases, identifying events that were hinted at in the clinical trials but that did not have sufficient statistical power.
These analytical programs can be particularly important in the current context in which annual drug withdrawals hit an all-time high in 2008 and the overall number of new drug approvals has been declining. Drug withdrawals are often very publicly damaging to a company.
The 2004 removal of the painkiller Vioxx from the market resulted in around $7 billion in legal and claims costs for Merck and a 33 percent drop in shareholder value within just a few days.
4.Personalized medicine.
Another promising big data innovation that could produce value in the R&D arena is the analysis of emerging large datasets (e.g., genome data) to improve R&D productivity and develop personalized medicine.
The objective of this lever is to examine the relationships among genetic variation, predisposition for specific diseases, and specific drug responses and then to account for the genetic variability of individuals in the drug development process.
Personalized medicine holds the promise of improving health care in three main ways: offering early detection and diagnosis before a patient develops disease symptoms; more effective therapies because patients with the same diagnosis can be segmented according to molecular signature matching (i.e., patients with the same disease often don’t respond in the same way to the same therapy, partly because of genetic variation); and the adjustment of drug dosages according to a patient’s molecular profile to minimize side effects and maximize response.
Personalized medicine is in the early stages of development. Impressive initial successes have been reported, particularly in the early detection of breast cancer, in prenatal gene testing, and with dosage testing in the treatment of leukemia and colorectal cancers.
We estimate that the potential for cost savings by reducing the prescription of drugs to which individual patients do not respond could be 30 to 70 percent of total cost in some cases.
Likewise, earlier detection and treatment could significantly lower the burden of lung cancer on health systems, given that early-stage surgery costs are approximately half those of late-stage treatment.
5.Analyzing disease patterns.
The fifth R&D-related big data value creation lever is analyzing disease patterns and trends to model future demand and costs and make strategic R&D investment decisions.
This analysis can help PMP companies optimize the focus of their R&D as well as the allocation of resources including equipment and staff.
D.New business models
The proliferation of digital health care data, from clinical to claims information, is creating business models that can complement, or compete with, existing ones and their levers. We highlight two potential new business models:
· 1.Aggregating and synthesizing patient clinical records and claims datasets
· 2.Online platforms and communities.
1.Aggregating and synthesizing patient clinical records and claims datasets.
The first type of new business model is one that aggregates and analyzes patient records to provide data and services to third parties. Companies could build robust clinical datasets that would enable a number of adjacent businesses.
These might include licensing and analyzing clinical outcomes data for payors and regulators to improve clinical decision making, leveraging patient databases to help PMP companies identify patients meeting certain criteria for inclusion in a clinical trial, or providing access to clinical databases for the discovery of biomarkers that help guide the selection of treatments.
An adjacent market that is developing not only provides services based on patient clinical records but also integrates claims datasets to provide services to the PMP sector for R&D and commercial modeling.
Clinical and claims data and services markets are just beginning to develop and grow — the rate of their expansion will depend on how rapidly the health care industry implements electronic medical records and evidence-based medicine.
2.Online platforms and communities.
Another potential new business model enabled by big data is that of online platforms and communities, which are already generating valuable data.
Examples of this business model in practice include Web sites such as PatientsLikeMe.com, where individuals can share their experience as patients in the system; Sermo.com, a forum for physicians to share their medical insights; and Participatorymedicine.org, a Web site made available by a nonprofit organization that encourages patient activism.
These online platforms could become a valuable source of data. For example, Sermo charges the PMP sector for access to its member physicians and information from their interactions on the site.
E.Public health
The use of big data can improve public health surveillance and response. By using a nationwide patient and treatment database, public health officials can ensure the rapid, coordinated detection of infectious diseases and a comprehensive outbreak surveillance and response through an Integrated Disease Surveillance and Response program.
This lever offers numerous benefits, including a smaller number of claims and payouts, thanks to a timely public health response that would result in a lower incidence of infection.
The United States would also be better prepared — in terms of laboratory capacity, for instance — for emerging diseases and outbreaks.
There would also be a greater public awareness of the health risks related to infectious diseases, which, in turn, would lower the chance of infections thanks to accurate and timely public health advisories. Taken together, all these components would help to produce a better quality of life.
References
[1] For more details on the methodology that we used in our case studies, see the appendix.
[2] Paul D. Mango and Vivian E. Riefberg, “Three imperatives for improving US health care,” McKinsey Quarterly December 2008.
[3] Centers for Medicare and Medicaid Services, National Health Expenditure Projections 2009–2019, September 2010.
[4] These studies adjust for relative health using purchasing power parity. For more detail, see Accounting for the cost of US health care: A new look at why Americans spend more, McKinsey Global Institute, December 2008 (www.mckinsey.com/mgi); Chris L. Peterson and Rachel Burton, US health care spending: Comparison with other OECD countries, Congressional Research Service, September 2007; Mark Pearson, OECD Health Division, Written statement to Senate Special Committee on Aging, September 2009.
[5] Amy L. Potts, Frederick E. Barr, David F. Gregory, Lorianne Wright, and Neal R. Patel, “Computerized physician order entry and medication errors in a pediatric critical care unit,” Pediatrics 113(1), 2004: 59–63.
[6] Cost differences can be quite substantial. For example, the cost of a colonoscopy can vary by a factor of six within the San Francisco area.