Harvard Business Review
by Vijay D’Silva, Bruce Lawler
February 28, 2022
Executive Summary
by Joaquim Cardoso MSc.
Chief Editor of “The Health Strategy Blog”
March 9, 2022
What is the context?
- Companies in a wide range of industries are trying to integrate analytics and data to improve their operations
- Not all companies have been as successful.
- In fact, top performers can have more than twice the impact in half the time compared to the average company implementing machine intelligence.
Why do some companies do so much better than others?
- By looking at 21 performance indicators across nine categories — strategy, opportunity focus, governance, deployment, partnerships, people, data execution, budget, and results — we were able to divide the 100 companies into four categories:leaders, planners, executors, and emerging organizations to identify the relationships between actions taken and investments made, and tangible and sustainable outcomes.
What are The Secrets Behind Smart Operators?
- The race to leverage data and analytics could be won with multiple coordinated actions rather than any single bold move.
Not everyone should strive to be a leader immediately; they should instead strive to move to the next better state.
- Leaders are the highest performers and comprise about 15% of the sample.
- Planners comprise about a quarter of the data set (25%)
- Executors, approximately a third of the respondents (33%) , tap into the ever-increasing pool of expertise and work with partners to create specific solutions directed at the most promising opportunities.
- Emerging companies, about a quarter of the pool, have the lowest level of maturity and have seen the smallest gains; many are just getting started.
Leaders are the highest performers and comprise about 15% of the sample.
- By investing in the right places, they have captured the largest gains from advanced digital technologies.
- Leaders are much more likely to have a defined process for the assessment and implementation of digital innovation.
- They are also more likely to follow that process regularly and to update it continually.
As a result, they have achieved significantly larger improvements than the rest in 20 of the 21 key performance indicators evaluated and were in the top 25% in all nine performance categories.
What are the Five Ways to Get Smart About Machine Intelligence?
- In general, we found that companies that succeeded in the deployment of advanced digital technologies did an honest assessment of where they were in terms of the nine performance indicators.
- On that basis, they were able to form a vision of where they wanted to be in three or four years.
- At the same time, they identified a few promising use cases to rack up quick wins.
- More specifically, the research identified five areas where the top performers stand out.
(1) Governance; (2) Deployment; (3) Partnerships; (4) People and (5) Data availability.
What are the Building Blocks for Digital Transformation?
- We found that the five areas — governance, deployment, partnerships, people, and data — were most effective when integrated into a playbook, often coordinated by a center of excellence.
- But first, companies need an honest assessment of their starting point across the nine dimensions.
- From there, a transition plan can start to take shape.
- While the ambition can be boundless, the steps cannot be too small — most leaders started with using data and simple tools to make decisions, then moved to more advanced techniques as they built maturity and familiarity with their data.
What is the size of the opportunity?
- Despite the recent and significant advances in MI, the full scale of the opportunity is just beginning to unfold. And that brings us to one more important difference between the leaders and the rest: money.
- The leaders spent 30 to 60% more and they expected to increase their budgets 10 to 15%, while the others reported little or no rises. That means the gap between the leaders and the rest could actually widen.
- Depending on its starting point, each company’s path will be different. But in terms of what works, the leaders are showing the way.
ORIGINAL PUBLICATION (full version)
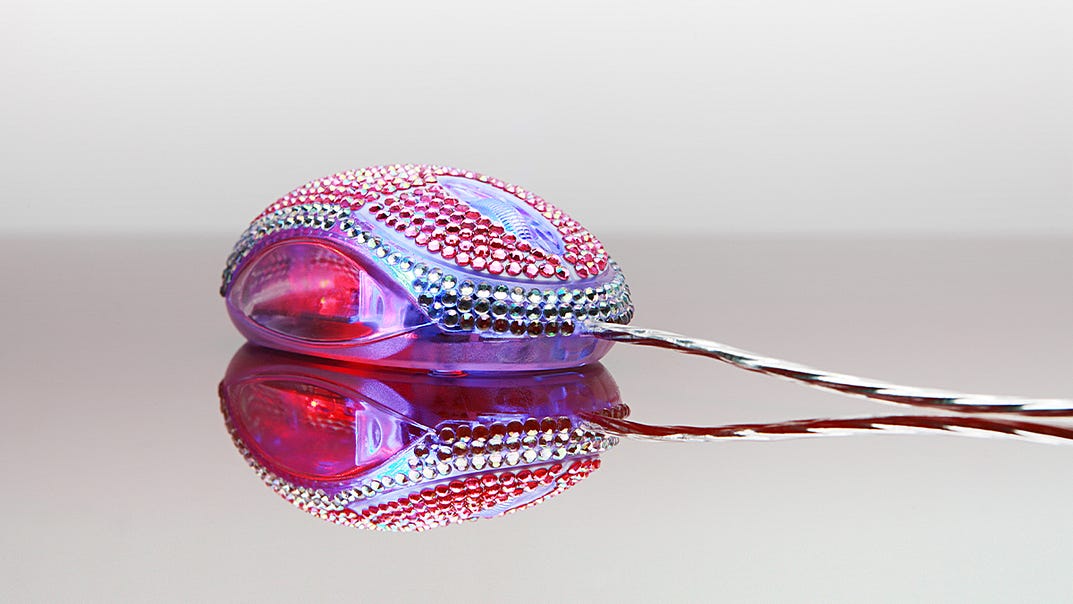
What Makes a Company Successful at Using AI?
Harvard Business Review
by Vijay D’Silva, Bruce Lawler
February 28, 2022
Summary
Companies in a wide range of industries are trying to integrate analytics and data to improve their operations, with decidedly mixed results. What are top performers doing differently — and better — than others?
In general, leading companies did an honest assessment of where they were, formed a vision of where they wanted to be in three or four years, and looked for ways to rack up quick wins.
More specifically, they outperformed in five areas: governance, deployment, partnerships, people, and data availability.
Vistra, a major U.S. power producer, had a problem. For its plants to operate efficiently, workers had to continuously monitor hundreds of different indicators, tracking temperatures, pressures, oxygen levels, and pump and fan speeds — and they had to make adjustments in real time. The process involved a huge amount of complexity, and it was too much for even the most skilled operator to get right all the time.
To address this challenge, the plant installed an AI-powered tool — a heat-rate optimizer — that analyzed hundreds of inputs and generated recommendations every 30 minutes.
Result: a 1% increase in efficiency. That may not sound like much, but it translates into millions in savings as well as lower greenhouse gas emissions.
Companies in a wide range of industries are trying to integrate analytics and data to improve their operations.
Wayfair, the e-commerce company, was an early mover in shifting its data to the cloud and investing in machine learning. When Covid-19 hit, and rapid changes to consumer demand followed, it was able to optimize container ship logistics, continually adjusting what goods were sent to which ports.
Result: an astonishing 7.5% reduction in inbound logistics costs.
Not all companies have been as successful as Wayfair, however. In fact, top performers can have more than twice the impact in half the time compared to the average company implementing machine intelligence.
Why do some companies do so much better than others?
… top performers can have more than twice the impact in half the time compared to the average company implementing machine intelligence.
To answer that question, McKinsey and MIT’s Machine Intelligence for Manufacturing and Operations (MIMO) studied 100 businesses in sectors from automotive to mining.
Through interviews, research, and a survey, we sought to get a sense of how they used digital, data analytics, and machine intelligence (MI) technologies; what they wanted to achieve; and how they kept track of their progress.
By looking at 21 performance indicators across nine categories — strategy, opportunity focus, governance, deployment, partnerships, people, data execution, budget, and results — we were able to divide the 100 companies into four categories:
leaders, planners, executors, and emerging organizations to identify the relationships between actions taken and investments made, and tangible and sustainable outcomes.
Any company with ambitions to gain from advanced digital technologies has the opportunity learn from best practice approaches, whether it is a planner, an executor, or an emerging company today.
We take a look beyond the top-level numbers to explore the underlying drivers of success.
The Secrets Behind Smart Operators
The race to leverage data and analytics could be won with multiple coordinated actions rather than any single bold move.
All four segments — leaders, planners, executers, and emerging companies — are operating in a dynamic space where the bar is rising and the number of machine learning use cases will continue to increase and embed themselves into business-as-usual.
Not everyone should strive to be a leader immediately; they should instead strive to move to the next better state.
- Leaders are the highest performers and comprise about 15% of the sample.
- Planners comprise about a quarter of the data set (25%)
- Executors, approximately a third of the respondents (33%) , tap into the ever-increasing pool of expertise and work with partners to create specific solutions directed at the most promising opportunities.
- Emerging companies, about a quarter of the pool, have the lowest level of maturity and have seen the smallest gains; many are just getting started.
Leaders are the highest performers and comprise about 15% of the sample.
- By investing in the right places, they have captured the largest gains from advanced digital technologies.
- Leaders are much more likely to have a defined process for the assessment and implementation of digital innovation.
- They are also more likely to follow that process regularly and to update it continually.
As a result, they have achieved significantly larger improvements than the rest in 20 of the 21 key performance indicators evaluated and were in the top 25% in all nine performance categories.
Planners comprise about a quarter of the data set.
- Planners often have strong people skills and considerable data execution expertise;
- they are methodical and focused on making the right investments.
- In many cases, though, these haven’t paid off yet, though a few are on the cusp of joining the leaders.
- While some planners are able to point to successful implementations, several have been unable to crack the code on scaling the use cases that really count.
- Others are struggling to escape from the” pilot purgatory “ McKinsey described in 2018.
Executors, approximately a third of the respondents, tap into the ever-increasing pool of expertise and work with partners to create specific solutions directed at the most promising opportunities.
- Then they implement these solutions as broadly as they can.
- Executors are results-oriented.
- They can and have achieved significant gains, despite building less infrastructure than the leaders or planners.
- On the other hand, they sometimes find it difficult to knit together disparate efforts into company-wide performance.
Emerging companies, about a quarter of the pool, have the lowest level of maturity and have seen the smallest gains; many are just getting started.
- Some emerging companies report moderate success with select use cases, but others are finding it difficult even to figure out where to invest.
- Few have the strategy, skills or infrastructure in place to go much further.
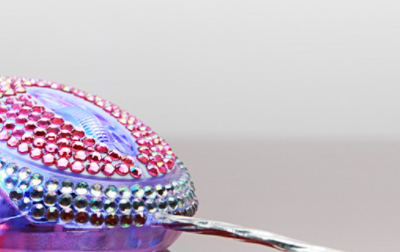
Five Ways to Get Smart About Machine Intelligence
In general, we found that companies that succeeded in the deployment of advanced digital technologies did an honest assessment of where they were in terms of the nine performance indicators.
On that basis, they were able to form a vision of where they wanted to be in three or four years.
At the same time, they identified a few promising use cases to rack up quick wins.
More specifically, the research identified five areas where the top performers stand out.
- Governance
- Deployment.
- Partnerships.
- People
- Data availability
More specifically, the research identified five areas where the top performers stand out.
Governance.
Machine intelligence is a strategic priority for leading companies. Many have built dedicated centers of excellence to support their implementation efforts, either within business units or as a centralized function to support the entire organization, ensure standards, and accelerate deployment. A dedicated and centralized support function also helps keep their digital programs on track and documents how their portfolio is progressing. Leaders are much more likely than lower-performing companies to have a defined process for the assessment of and implementation of digital innovation. For example, the pharmaceutical firm Bayer uses a well-documented governance process to deploy multiple applications at one plant, which it then rolled out across its network, resulting in a revenue lift.
However, leaders also recognize that change is inevitable in this fast-moving space. Most of the leaders in our data set continually refine and improve their processes, whereas executors and planners in our data set often get stuck, which limits the ability to scale successfully.
Deployment.
Leading organizations apply MI more widely and use more sophisticated approaches. For example, every single leader implemented MI in forecasting, maintenance optimization, and logistics and transportation. The leaders are also much more likely to adopt advanced approaches, such as the application of machine vision to product quality assurance. One biopharma player, Amgen, found that visual inspection system operations posed great opportunities to automate and leverage AI technologies. Amgen is developing a fully validated visual inspection system using AI that will boost particle detection 70% and cut false rejects by 60%.
While applications like these can have tremendous impact, these firms also realize that any long-term impact requires pulling multiple levers in concert, and that broad, enterprise-wide deployment is key.
Partnerships.
Partnerships are common, often with academia, start-ups, existing technology vendors, and external consultants. Leaders, however, worked with a wider range of partners, and more intensively, in order to maximize speed and learning. For example, Colgate-Palmolive and Pepsico/Frito-lay, two consumer product companies worked with a systems vendor, Augury, deployed AI-driven machine health diagnostics on their production lines; in one case, this prevented an eight-day outage. Analog Devices, a semiconductor firm, collaborated with MIT to develop a novel MI quality-control that allowed it to identify which production runs and tools might have a fault. This meant that company engineers only had to review 5% of the process data they had to before.
Leaders, despite their higher capabilities, actually relied more on external partners to further accelerate their learning and time to impact.
People.
Leading companies take steps to ensure that as many stakeholders as possible have the skills and resources they need to employ advanced digital approaches, rather than keeping this expertise the preserve of specialists. More than half train their front-line personnel in MI fundamentals, for example, compared to only 4% of other companies. McDonald’s, a global quick-service restaurant, used MI to improve a wide range of operational tasks, from predicting customer response to forecasting real time footfall. The company adopted a hybrid approach to do this: its corporate center of excellence tests and develops new approaches before packaging them into easy-to-use tools that are made widely available. This system helps team members in the field understand the importance of good data and hone their problem identification skills.
It became clear that leaders view the use of data and analytics as deeply embedded to how they operate, rather than keeping it siloed and restricted to a few employees.
Data availability.
Leaders make data accessible. All of the leaders in our research give frontline staff access to data, compared to 62% of the rest. The leaders also all acquire data from customers and suppliers, and 89% share their own data back. Leading companies are almost twice as likely as others to enable remote access to data and to store a significant fraction of their data in the cloud. In short, the democratization of data is a critical aspect to the effective use of analytics. A good example comes from Cooper Standard, an automotive supplier. It requires teams to address data strategy early in the development process for new MI applications; this ensures that all uses cases are built on robust, well-managed data. This democratization of data stands in stark contrast to many firms where information is power and zealously guarded.
Building Blocks for Digital Transformation
We found that the five areas — governance, deployment, partnerships, people, and data — were most effective when integrated into a playbook, often coordinated by a center of excellence.
But first, companies need an honest assessment of their starting point across the nine dimensions.
From there, a transition plan can start to take shape. Even if it’s rough, it assigns realist medium-term targets that account for the barriers to change — skilled talent, investment capacity, and critical infrastructure such as the migration of data from legacy systems to the cloud.
While the ambition can be boundless, the steps cannot be too small — most leaders started with using data and simple tools to make decisions, then moved to more advanced techniques as they built maturity and familiarity with their data.
Despite the recent and significant advances in MI, the full scale of the opportunity is just beginning to unfold.
And that brings us to one more important difference between the leaders and the rest: money.
The leaders spent 30 to 60% more and they expected to increase their budgets 10 to 15%, while the others reported little or no rises. That means the gap between the leaders and the rest could actually widen.
Depending on its starting point, each company’s path will be different. But in terms of what works, the leaders are showing the way.
The authors would like to thank Duane Boning, Erez Kaminski, Pete Kimball, Retsef Levi, Ingrid Millan, and Aaron Wang, along with MIT’s LGO program, for their contributions to this research and article.
Originally published at https://hbr.org on February 28, 2022.