the health strategist
research and strategy institute — for continuous transformation
in value based health, care, cost and tech
Joaquim Cardoso MSc
Chief Researcher & Editor of the Site
March 30, 2023
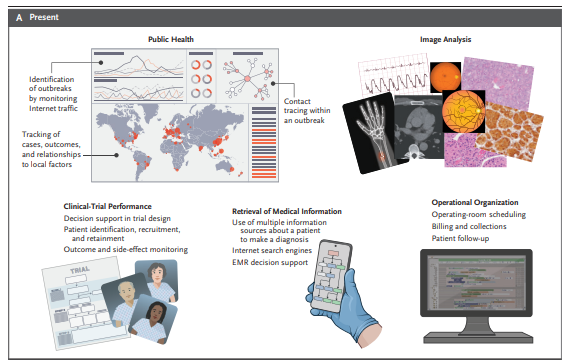
EXECUTIVE SUMMARY
The article, explores the role of artificial intelligence (AI) and machine learning (ML) in clinical medicine.
- As computers and the concept of artificial intelligence (AI) were almost simultaneously developed in the 1940s and 1950s, the field of medicine was quick to see their potential relevance and benefit.1,2
- However, by the late 1970s, there was disappointment that the two main approaches to computing in medicine — rule-based systems and matching, or pattern recognition, systems — had not been as successful in practice as one had hoped.
- While initial expectations for rule-based and matching systems were not met, incorporating pathophysiological knowledge improved performance.
- However, computers in the 1980s were still too slow for practical use in clinical medicine.
- The authors note that progress in data science has changed this, as dense circuits, increased storage capacity, and faster computing have allowed for the identification and processing of unstructured data.
- As a result, AI and ML can now provide actionable insights into human behavior, with neural networks and deep learning models offering sophisticated functional real-time output to data queries.
- The article highlights the potential for AI and ML to transform clinical medicine, but also raises ethical and societal concerns about data privacy and the use of AI in decision-making.
The second part of the article discusses the use of AI and machine learning in medicine, including image processing, outbreak detection, and hospital business operations.
- However, the article highlights the need to establish norms for AI and machine learning in medicine and address the bias in algorithms to ensure that the results obtained reflect the real problems faced by health professionals.
- The article also raises questions about the role of AI and machine learning in clinical practice and the standards to which AI and machine learning-based interventional research should be held.
- The evaluation of progress in AI and machine learning has its own set of problems, and the medical community needs to develop a unique set of standards for this type of intervention to ensure that the outcome is beneficial for all patients under consideration.
The authors firmly believe that the introduction of AI and machine learning in medicine has helped health professionals improve the quality of care that they can deliver and has the promise to improve it even more in the near future and beyond.
- Just as computer acquisition of radiographic images did away with the x-ray file room and lost images, AI and machine learning can transform medicine.
- Health professionals will figure out how to work with AI and machine learning as we grow along with the technology.
- AI and machine learning will not put health professionals out of business; rather, they will make it possible for health professionals to do their jobs better and leave time for the human–human interactions that make medicine the rewarding profession we all value.
SELECTED IMAGE(S)
Figure 1. Improvements over 50 Years in the Ability of Computers to Store and Process Data.
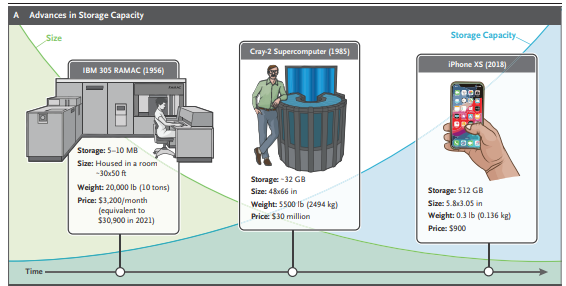
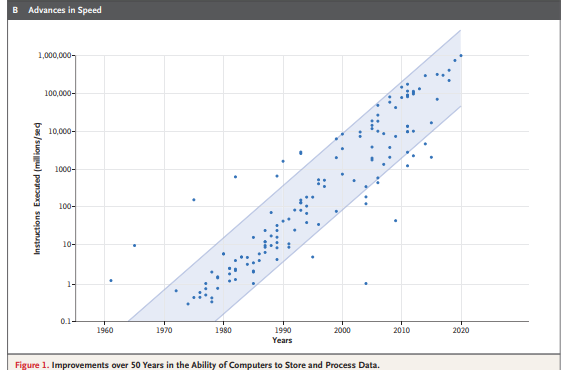
Figure 2. Spectrum of Artificial Intelligence (AI) in Medicine.
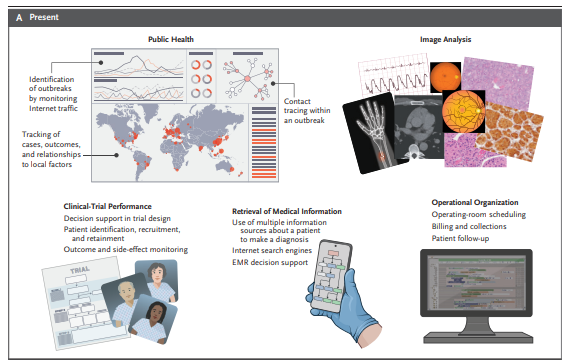
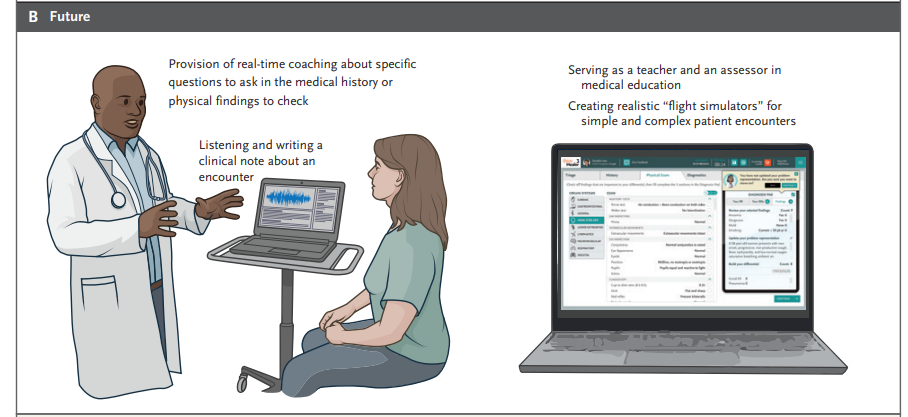
Figure 3. Chatbots in Medicine.
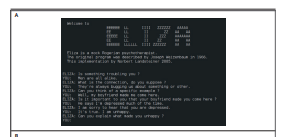
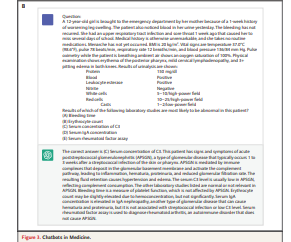
DEEP DIVE
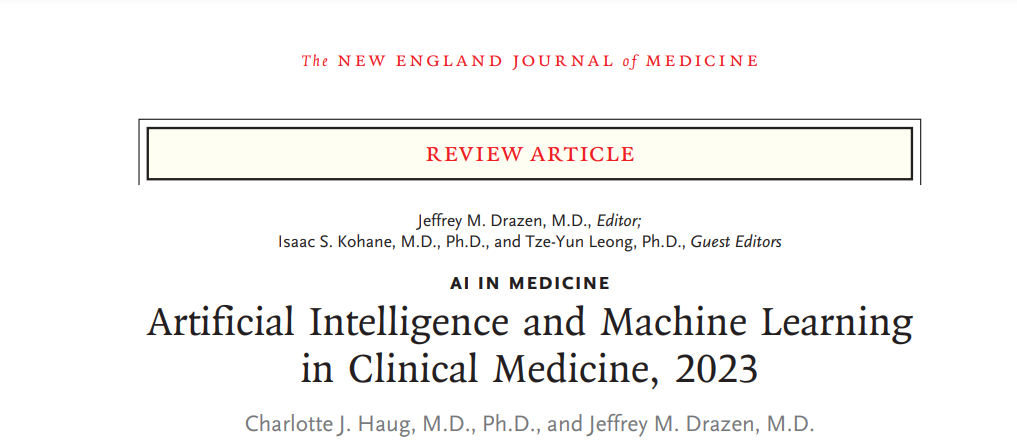
Artificial Intelligence and Machine Learning in Clinical Medicine, 2023
NEJM
Charlotte J. Haug, M.D., Ph.D., and Jeffrey M. Drazen, M.D.
As computers and the concept of artificial intelligence (AI) were almost simultaneously developed in the 1940s and 1950s, the field of medicine was quick to see their potential relevance and benefit.1,2
In 1959, Keeve Brodman and colleagues claimed that “the making of correct diagnostic interpretations of symptoms can be a process in all aspects logical and so completely defined that it can be carried out by a machine.”3 Eleven years later, William B. Schwartz wrote in the Journal, “Computing science will probably exert its major effects by augmenting and, in some cases, largely replacing the intellectual functions of the physician.”4 He predicted that by the year 2000, computers would have an entirely new role in medicine, acting as a powerful extension of the physician’s intellect.
However, by the late 1970s, there was disappointment that the two main approaches to computing in medicine — rule-based systems and matching, or pattern recognition, systems — had not been as successful in practice as one had hoped.
The rule-based systems were built on the hypothesis that expert knowledge consists of many independent, situation-specific rules and that computers can simulate expert reasoning by stringing these rules together in chains of deduction. The matching strategies tried to match a patient’s clinical characteristics with a bank of “stored profiles,” which we now refer to as “illness scripts,”5 of the findings in a given disease. More effort was put into understanding the clinical decision-making process itself.6 It became clear that the key deficiencies in most previous programs stemmed from their lack of pathophysiological knowledge. When such knowledge was incorporated, the performance greatly improved.
Nevertheless, in the 1980s, computers were not up to the task. The rule-based systems had by 1987 proved useful in a variety of commercial tasks but had not worked in clinical medicine.
Indeed, Schwartz and colleagues noted that “the process is so slow that it is impractical even with modern high-speed computers.”7 They continued: “After hearing for several decades that computers will soon be able to assist with difficult diagnoses, the practicing physician may well wonder why the revolution has not occurred.”7
Progress in Data Science
Figure 1. Improvements over 50 Years in the Ability of Computers to Store and Process Data.
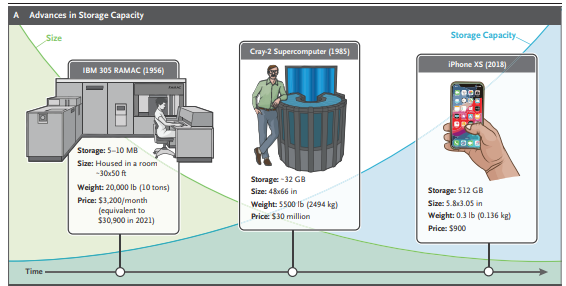
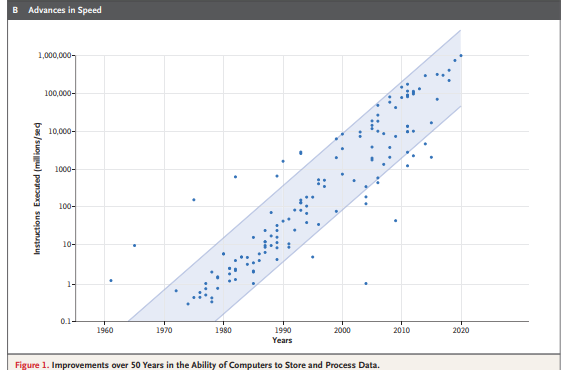
In the 1950s, computers were large and slow. The first hard-disk drive was the IBM Model 350 Disk File, introduced in 1956.
It had a total storage capacity of 5 million characters (just under 5 MB). The first hard drive to have more than 1 GB in capacity was the IBM 3380, introduced in 1980. It was the size of a refrigerator and weighed 550 lb (250 kg); the price was $100,000. But integrated-circuit technology was improving. In 1965, Gordon Moore, cofounder of Fairchild Semiconductor and Intel, predicted that the number of transistors in an integrated circuit, and, hence, its potential computing power, would double every 2 years. His prediction was right; this change in semiconductor density is known as Moore’s law. However, Moore’s law tells us more than the number of transistors per square centimeter, since other aspects of technological progress, such as processing speed and the price of electronic products, are strongly linked to Moore’s law. With more dense circuits, computer memory and computing speeds increased, and today, pocket-sized devices that are more powerful than the 1980s supercomputers, which took up entire rooms, are common and available at a fraction of the price (Figure 1).
Progress in data science is not simply a matter of increased performance, speed, and storage.
In addition to the type of information found in libraries, data generated in organizations, and established systems designed to gather and codify data, new forms of technology can use data that are both people-generated and machine-generated. These data are often chaotic and unstructured. Data now come from many additional sources, including social networks, blogs, chat rooms, product-review sites, communities, website pages, email, documents, images, videos, and music, along with wearable and environmental sensors. Many people open aspects of their medical records and personal genetic data for online access by anyone. Storage capacity is so great that vast portions of the corpus of recorded human knowledge and activity can be stored and readily accessed.
Once we had the data, we needed more than data; we needed ways to identify and process the data.
Google became the leader in online searching by harnessing the searches performed by others to identify what people wanted to know. This required a second revolution, mathematical algorithms that could rapidly, and with reasonable reliability, track this behavior and aid the end user in finding particular information. More dense information storage and faster computing allowed for practical, real-time solutions of mathematical expressions that could be used to find relationships in the data that were previously unknowable. As a result, data science could flourish and flex its muscles in a way that was previously impossible.
We are now able to use unstructured data to identify untold relationships among elements in the data, allowing the use of dynamic data and data with multiple contexts that, when approached and analyzed in nontraditional ways, provide actionable insights into human behavior.
Neural networks became more sophisticated as the computing power allowed functional real-time output to data queries. Transformers (i.e., deep-learning models that differentially weigh the importance of each part of the input data) made natural-language processing possible. With this approach, the complexities of the underlying computer models, and the corpus of data from which those models could draw, grew and became more powerful. The goal of a computer that could emulate certain aspects of human interaction went from an impossible dream to a reality.
The connectedness allowed by data science is driving a new kind of discovery.
People are using social networks to draw their own connections between friends, things, events, likes, dislikes, places, ideas, and emotions. Governments are analyzing social networks to stop terrorist acts. Businesses are mining social and transactional information for connections that will help them discover new opportunities. Scientists are building massive grids of connected data to tease out new findings, using AI and machine learning. As addressed in more detail below, these advances have allowed the emergence of computers that can help you perform tasks that previously had been tedious. The Star Wars character C-3PO was a crude version of the AI-based virtual assistants (e.g., Apple’s Siri, Google’s Assistant, and Amazon’s Alexa) that have become part of our daily life and can help us perform defined tasks. Anyone who has used one of these devices has experienced their convenience (e.g., instructing the virtual assistant to “set the oven timer for 20 minutes” so that food is properly cooked) but also the annoyance of having the assistant break into a conversation with some unrelated facts. AI and machine learning constitute the driving force behind these devices.
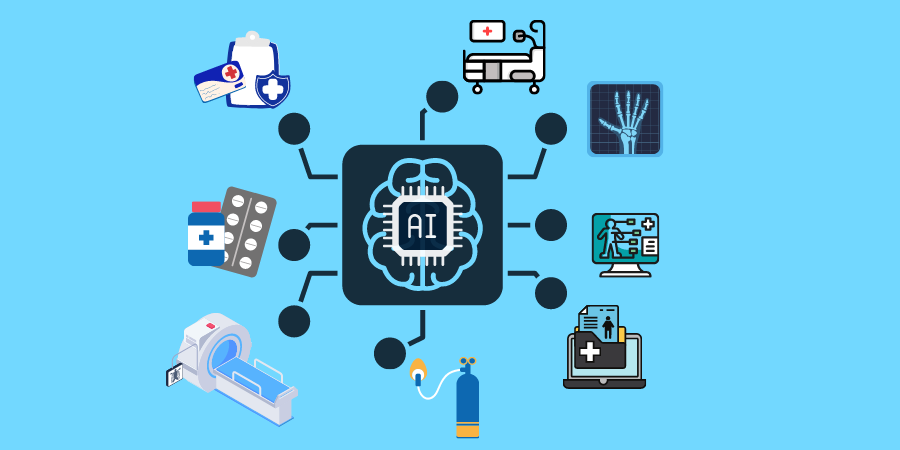
AI and Machine Learning in Medicine
In the 1990s and into the early 2000s, even with slow computers and limited memory, the problem of having machines successfully perform certain medical tasks that were repetitive, and therefore prone to human error, was being solved.
Through a substantial investment of money and intellectual effort, computer reading of electrocardiograms (ECGs) and white-cell differential counts, analysis of retinal photographs and cutaneous lesions, and other image-processing tasks has become a reality. Many of these machine-learning–aided tasks have been largely accepted and incorporated into the everyday practice of medicine. The performance of these machine tasks is not perfect and often requires a skilled person to oversee the process, but in many cases, it is good enough, given the need for relatively rapid interpretation of images and the lack of local expertise.
Figure 2. Spectrum of Artificial Intelligence (AI) in Medicine.
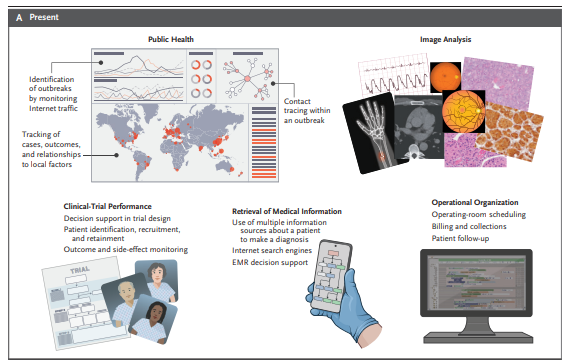
However, the use of AI and machine learning in medicine has expanded beyond the reading of medical images.
AI and machine-learning programs have entered medicine in many ways, including, but not limited to, helping to identify outbreaks of infectious diseases that may have an impact on public health; combining clinical, genetic, and many other laboratory outputs to identify rare and common conditions that might otherwise have escaped detection; and aiding in hospital business operations (Figure 2). In the months to come, the Journal will publish other review articles that take a selective look at AI and machine learning in medicine in 2023. But before the first article appears, in about a month’s time, it is important to consider the overriding issues that need to be considered as we learn to work hand in hand with machines.
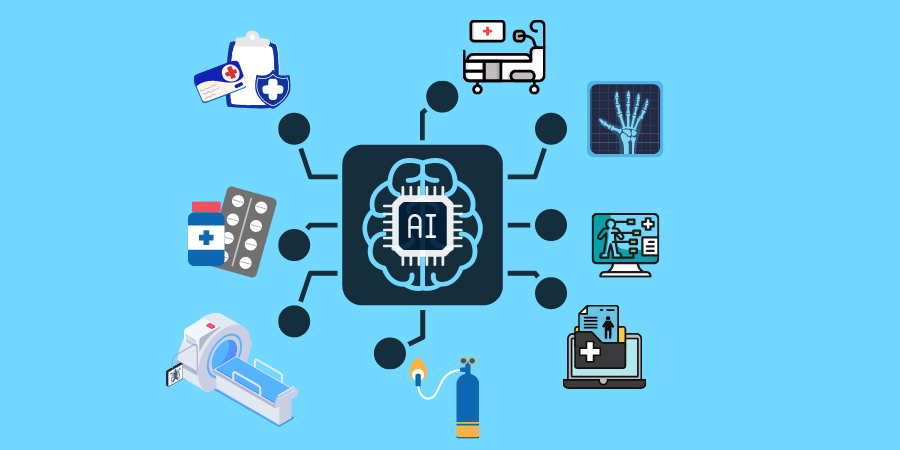
Unresolved Issues in AI and Machine Learning in Medicine
ESTABLISHING NORMS
As noted above, the use of AI and machine learning has already become accepted medical practice in the interpretation of some types of medical images, such as ECGs, plain radiographs, computed tomographic (CT) and magnetic resonance imaging (MRI) scans, skin images, and retinal photographs. For these applications, AI and machine learning have been shown to help the health care provider by flagging aspects of images that deviate from the norm.
This suggests a key question: what is the norm? This simple question shows one of the weaknesses of the use of AI and machine learning in medicine as it is largely applied today. How does bias in the way AI and machine-learning algorithms were “taught” influence how they function when applied in the real world? How do we interject human values into AI and machine-learning algorithms so that the results obtained reflect the real problems faced by health professionals? What issues must regulators address to ensure that AI and machine-learning applications perform as advertised in multiple-use settings? How should classic approaches in statistical inference be modified, if at all, for interventions that rely on AI and machine learning? These are but a few of the problems that confront us; the “AI in Medicine” series will address some of these matters.
ROLE OF AI AND MACHINE LEARNING IN CLINICAL PRACTICE
Pitfalls aside, there is much promise. If AI and machine-learning algorithms can be reduced to clinically useful “apps,” will they be able to weed their way through mountains of clinical, genomic, metabolomic, and environmental data to aid in precision diagnosis? Can AI and machine-learning–driven apps become your personal scribe and free up your time spent on documentation so that you can spend more time with patients? Can the apps prompt you to ask a key question that could help in the differential diagnosis? Can they outwit the AI and machine-learning algorithms, used by insurance companies, that make it difficult for you to order a positron-emission tomographic–CT scan or collect reimbursement for the time you spent with a patient and the patient’s family? In each area, progress has been made. Is it good enough?
CLINICAL RESEARCH ON AI AND MACHINE-LEARNING APPLICATIONS
The evaluation of progress has its own set of problems. In traditional clinical research, when progress takes the form of a new drug for a definable condition, the standards for testing and accepting the drug as an advance are well established. When the intervention is an AI and machine-learning algorithm rather than a drug, the medical community expects the same level of surety, but the standards for describing and testing AI and machine-learning interventions are far from clear.
What are the standards to which AI and machine learning–based interventional research should be held, if an app is going to be accepted as the standard that will shape, reform, and improve clinical practice? That research has three components. First, the research must be structured to answer a clinically meaningful question in a way that can influence the behavior of the health professional and lead to an improvement in outcomes for a patient. Second, the intervention must be definable, scalable, and applicable to the problem at hand. It must not be influenced by factors outside the domain of the problem and must yield outcomes that can be applied to similar clinical problems across a wide range of populations and disease prevalences. Can AI and machine learning–driven care meet these standards — ones that we demand from a novel therapeutic intervention or laboratory-based diagnostic test — or do we need to have a unique set of standards for this type of intervention? Third, when the results of the research are applied in such a way as to influence practice, the outcome must be beneficial for all patients under consideration, not just those who are similar to the ones with characteristics and findings on which the algorithm was trained. This raises the question of whether such algorithms should include consideration of public health (i.e., the use of scarce resources) when diagnostic or treatment recommendations are being made and the extent to which such considerations are part of the decision-making process of the algorithm. Such ethical considerations have engaged health professionals and the public for centuries.8
USE OF AI AND MACHINE-LEARNING APPLICATIONS IN CONDUCTING CLINICAL RESEARCH
AI and machine learning have the potential to improve and possibly simplify and speed up clinical trials through both more efficient recruitment and matching of study participants and more comprehensive analyses of the data. In addition, it may be possible to create synthetic control groups by matching historical data to target trial enrollment criteria. AI and machine learning may also be used to better predict and understand possible adverse events and patient subpopulations. It seems possible that AI could generate “synthetic patients” in order to simulate diagnostic or therapeutic outcomes. But the use of AI and machine-learning applications and interventions introduces a set of uncertainties that must be dealt with both in protocols and in reporting of clinical trials.9,10
In this AI in Medicine series, we plan to cover progress, pitfalls, promise, and promulgation at the interface of AI and medicine. It is important to understand that this is a fast-moving field, so to some extent, what we publish may have the resolution of a snapshot of the landscape taken from a bullet train. Specifically, things happening in close temporal proximity to publication may be blurred because they are changing quickly, but the distant background will be in reasonably good focus. One area of substantial progress in AI and machine learning (i.e., in the foreground, in our snapshot analogy) is the appearance of sophisticated chatbots that are available for use by the general public. Although chatbots have only recently been introduced at a level of sophistication that could have an impact on daily medical practice, we believe that their potential to influence how medicine is practiced is substantial and that we would be remiss not to address that potential as well as possible problems related to their use.
Chatbots in Medicine
Figure 3. Chatbots in Medicine.
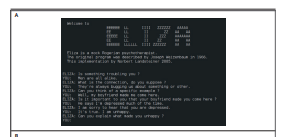
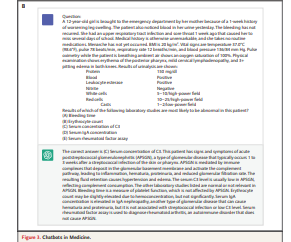
In this issue of the Journal, an article by Lee et al.11 introduces the GPT-4 chatbot and its medical applications. The article was written by a team of researchers who work for the entities that created GPT-4, a chatbot with a broad education that includes medical knowledge. Before we see the future, a quick look at the past will be helpful. A chatbot is a computer program that uses AI and natural-language processing to understand questions and automate responses to them, simulating human conversation. A very early medical chatbot, ELIZA, was developed between 1964 and 1966 by Joseph Weizenbaum at the Artificial Intelligence Laboratory of the Massachusetts Institute of Technology (Figure 3).
Chatbot technology is now almost everywhere, from customer service to personal virtual assistants, as noted above. With the powerful computers available today, language models have hundreds of billions of parameters, which can be used to generate new text. This ability, combined with an almost infinite amount of available (Internet) data with which to train the network, means that language models can do more and more, as shown by the Chat Generative Pre-trained Transformer, or ChatGPT.
ChatGPT is a language model trained by OpenAI. It was introduced publicly in November 2022 (https://openai.com/blog/chatgpt. opens in new tab) and has demonstrated a new way in which AI-driven machines can interact with people. The new-generation chatbots hold the promise of being a scribe and coach, but with some key caveats. Many of these caveats were described by the developers of ChatGPT at its launch but warrant special consideration when used in medicine, as detailed by Lee et al.11 In their current iteration, the new generation of chatbots can help with the medical documentation problem and answer key questions that could help in the differential diagnosis, as noted above. But it is difficult to know whether the answers provided are grounded in appropriate fact. The onus would be on clinicians to proofread the work of the chatbot, just as clinicians need to proofread clinical notes that they dictate. The difficulty is that such proofreading may be beyond the expertise of the user. Proofreading a note on a patient visit is likely to be well within the range of the provider’s expertise, but if the chatbot is asked a question as a “curbside consult,” the veracity of the answer may be much harder to determine.
The application of greatest potential and concern is the use of chatbots to make diagnoses or recommend treatment. A user without clinical experience could have trouble differentiating fact from fiction. Both these issues are addressed in the article by Lee and colleagues,11 who point out the strengths and weaknesses of using chatbots in medicine. Since the authors have created one such entity, bias is likely.
Nevertheless, we think that chatbots will become important tools in the practice of medicine. Like any good tool, they can help us do our job better, but if not used properly, they have the potential to do damage. Since the tools are new and hard to test with the use of the traditional methods noted above, the medical community will be learning how to use them, but learn we must. There is no question that the chatbots will also learn from their users. Thus, we anticipate a period of adaptation by both the user and the tool.
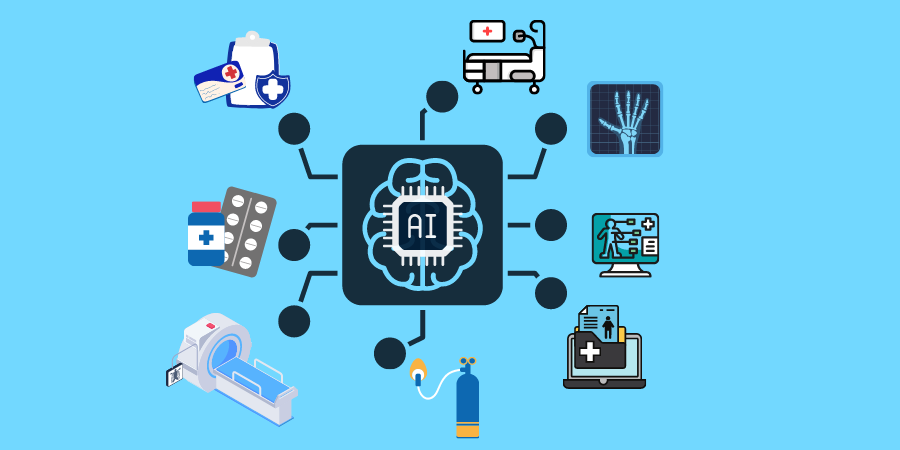
Conclusions
We firmly believe that the introduction of AI and machine learning in medicine has helped health professionals improve the quality of care that they can deliver and has the promise to improve it even more in the near future and beyond.
- Just as computer acquisition of radiographic images did away with the x-ray file room and lost images, AI and machine learning can transform medicine. Health professionals will figure out how to work with AI and machine learning as we grow along with the technology.
- AI and machine learning will not put health professionals out of business; rather, they will make it possible for health professionals to do their jobs better and leave time for the human–human interactions that make medicine the rewarding profession we all value.
References
1.Turing AM. Computing machinery and intelligence. Mind 1950;59:433–460.
2.Yu K-H, Beam AL, Kohane IS. Artificial intelligence in healthcare. Nat Biomed Eng 2018;2:719–731.
3.Brodman K, Van Woerkom AJ, Erdmann AJ Jr, Goldstein LS. Interpretation of symptoms with a data-processing machine. AMA Arch Intern Med 1959;103:776–782.
4.Schwartz WB. Medicine and the computer — the promise and problems of change. N Engl J Med 1970;283:1257–1264.
5.Bowen JL. Educational strategies to promote clinical diagnostic reasoning. N Engl J Med 2006;355:2217–2225.
6.Pauker SG, Gorry GA, Kassirer JP, Schwartz WB. Towards the simulation of clinical cognition: taking a present illness by computer. Am J Med 1976;60:981–996.
7.Schwartz WB, Patil RS, Szolovits P. Artificial intelligence in medicine. Where do we stand? N Engl J Med 1987;316:685–688.
8.Rosenbaum L. Trolleyology and the dengue vaccine dilemma. N Engl J Med 2018;379:305–307.
9.Liu X, Cruz Rivera S, Moher D, Calvert MJ, Denniston AK; SPIRIT-AI and CONSORT-AI Working Group. Reporting guidelines for clinical trial reports for interventions involving artificial intelligence: the CONSORT-AI extension. Nat Med 2020;26:1364–1374.
10.Cruz Rivera S, Liu X, Chan A-W, Denniston AK, Calvert MJ; SPIRIT-AI and CONSORT-AI Working Group. Guidelines for clinical trial protocols for interventions involving artificial intelligence: the SPIRIT-AI extension. Lancet Digit Health 2020;2(10):e549-e560.
11.Lee P, Bubeck S, Petro J. Benefits, limits, and risks of GPT-4 as an AI chatbot for medicine. N Engl J Med 2023;388:1233–1239.