Healthcare transformation to a digitally connected care ecosystem cannot happen without expert data capabilities.
Gartner
Laura Craft, VP Analyst
12 August 2020
Edited by (this site version)
Joaquim Cardoso MSc
Health Revolution — Foundation
Data and Analytics — Institute
June 24, 2022
OVERVIEW
Key Challenges
·
- Most healthcare organizations are mired in traditional data integration processes and governance that are weighing down real-time response and nimbleness.
Yet across providers, payers and life science companies, the value of healthcare data is increasing as more powerful digital and analytics capabilities are leveraged.
Your legacy data integration and governance processes will become roadblocks to progress if they are not updated for adaptability and improved agility.
- COVID-19 exposed serious flaws and gaps in data nimbleness and the ability to use data to help manage the crisis on the local, regional and national levels.
Examining shortcomings and closing gaps are critical for effective ongoing surveillance, response and recovery.
- Healthcare’s adoption of AI and automation capabilities is becoming pervasive.
AI can create competitive advantage and value in terms of cost optimization and improvements in clinical safety, experience and other outcomes.
However, as more AI implementations move from the lab and into workflows, frontline usability is still a struggle.
Artificial intelligence demands the careful blend of the science behind complex processing of massive data, and the art of engendering trust on the front lines in the integrity and accuracy of the algorithm.
Recommendations
As a healthcare CIO or analytics leader responsible for advancing digital innovation and transformation, you should:
- Reengineer your data services layer by investing in next-generation smart data technologies.
Develop a pipeline of data tools and enablers across your digital architecture. - Move AI from the lab to value realization by focusing on the human elements of culture and frontline adoption.
Democratize ownership by engaging end users early and continuously. - Break down and reconstruct your data governance processes and models.
Drive improved enterprise data agility and collaboration by using adaptive data governance and DataOps approaches.
ORIGINAL PUBLICATION (long version)
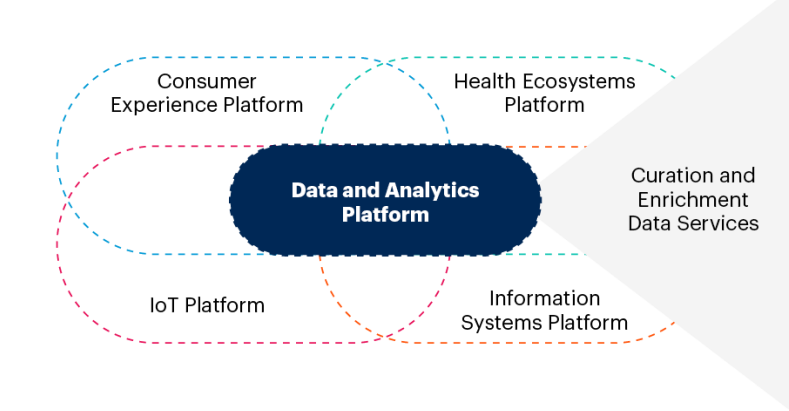
Best Practices to Reset Healthcare Organizations’ Data and Analytics Strategy
Gartner
Laura Craft, VP Analyst
12 August 2020
INTRODUCTION
Reliable, trustworthy and accessible data is crucial to any healthcare organization’s ongoing ability to successfully orchestrate and pay for care, conduct research and deliver value in the expanding healthcare ecosystem, tackle another COVID-like disruption, and extract value from AI investments.
Yet persistent challenges with data across the entire data pipeline from inception to value stifle providers’ ability to persistently leverage data as a strategic asset.
CIOs must make 2020 the year of data primacy by building new institutional alignment around data and renewed urgency in reigniting data and analytics strategies.
During a spring 2020 Gartner webinar of payer and provider CIO attendees, Gartner polled the participants and found that the №1 area for increased investment in data and analytics in 2020 is data integration and curation tools (see Figure 1).
Figure 1: Top Areas for Increased Data and Analytics Investment 2020
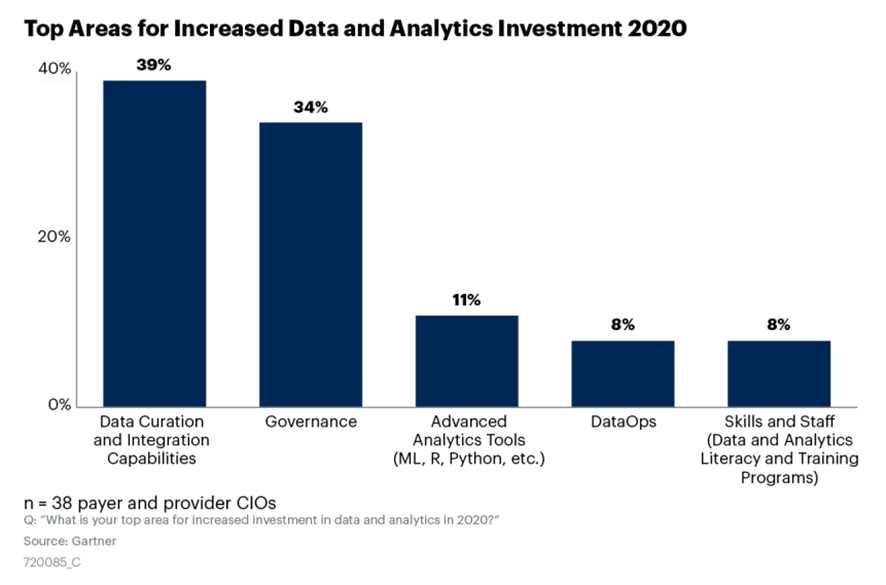
ANALYSIS
- Reengineer Your Data Services Layer by Investing in Next-Generation Smart Data Technologies
- Move AI From the Lab to Value Realization by Focusing on the Human Elements of Culture and Adoption
- Drive Improved Enterprise Agility by Implementing Adaptive Data Governance and DataOps
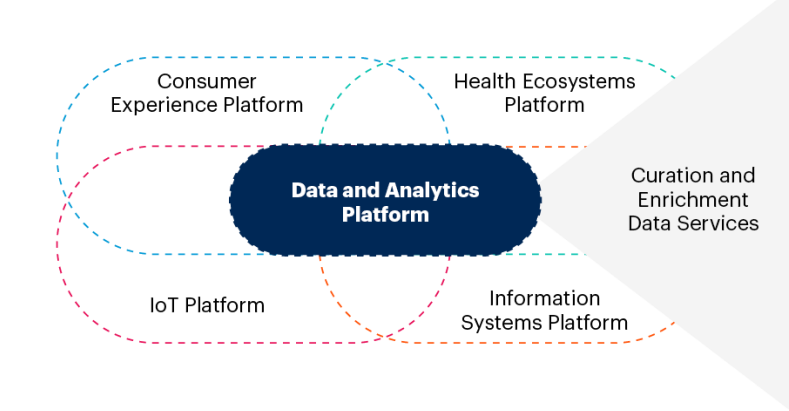
1.Reengineer Your Data Services Layer by Investing in Next-Generation Smart Data Technologies
Organizations that are leading in the digital transformation era are reengineering their enterprise architecture to include a layer of data and analytics microservices.
These services enable the kind of massive data orchestration and real-time delivery of insights that is needed to optimize and transform provider operating models (see Figure 2).
Figure 2: Data Services for the Digital Ecosystem
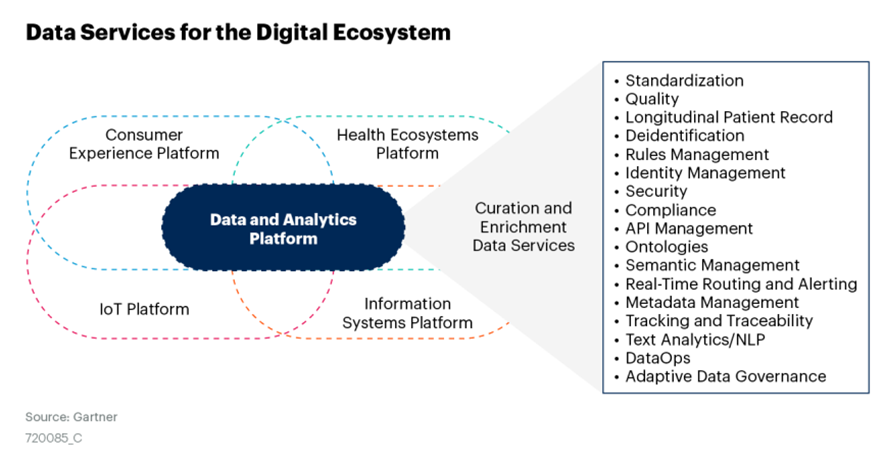
Specific services and capabilities to support both optimization and transformation include:
· Automating the ingestion, normalization and standardization of data from multiple data sources and EHRs, across all types and formats common to healthcare data (for example, unstructured, unstructured, CDA and HL7)
· Providing agile data governance capabilities, including data quality management (some leveraging AI), metadata management, lineage tracking and traceability
· Creating the longitudinal patient record for population health management, patient journey tracking, consumer health and wellness management
· Providing deidentification routines for sensitive data that may be used for research or study purposes or otherwise to maintain consumer privacy
· Coordinating access, identity management, security and compliance rules
· Building an API and rule management library to support the increasing use of APIs, real-time routing and alerting to deliver insights back into the clinical, operational, consumer engagement and administrative workstreams
· Building a layer of semantic management that enables use of the appropriate ontologies and taxonomies
Gartner calls this collection of services a data curation and enrichment hub (see “Healthcare’s Digital Data Dexterity Demands a Data Curation and Enrichment Hub”).
You will evolve your hub overtime, using the services of one or several vendors, depending on your needs and scope.
This is why for data architects it is an endgame design point as you modernize your entire analytics core to fit in the enterprise architecture.
These hubs progressively replace legacy ways of moving data from source to target and enable digital transformation and AI.
As with all tiers of the architecture, the model should be flexible (composable) so new tools and capabilities can be added as needed.
The movement toward healthcare data curation and enrichment hubs is active, with essentially three approaches emerging among healthcare providers:
· The first approach comes in the form of upgrades and new releases from existing full-service, analytics and population health platform players that historically have been the integrators of bringing disparate data together.
Gartner observes that some of the large, well-established vendors like Arcadia, Cerner, Dimensional Insight, Health Catalyst and Philips are retooling their platforms to have more powerful ingestion and data management strengths.
Some are even moving in the direction of Gartner’s connected care vision (see “Create Connected Care Pathways That Bridge Consumer and Healthcare Provider Activities”).
These architectures are positioned to deliver adaptable building blocks of application and analytics products and services.
· The second approach is instantiated by an emerging class of vendors.
Their primary focus is delivering the broad array of data services essential to a hub.
Example vendors in this category include Clearsense, InterSystems and Verinovum.
Many of these vendors have interoperability as their backbone strength and are expanding the services and capabilities of their platforms to meet the growing demands and challenges of moving health data across the ecosystem.
· An interesting third emerging vendor approach is leveraging knowledge graph technology to pull together data from anywhere and create connected and cross-referenced datasets.
While the market is nascent, it is still worth considering how vendors like AllegroGraph or TigerGraph might fit into your preferred architecture approach.
To make sure that data doesn’t become a barrier to progress and innovation, and can support rapid action and response, healthcare CIOs should take the following steps:
· Proactively assess what the data integration demands across your healthcare organization will be over the next three to five years.
Cull insight from your organization’s strategic plan and through other deliberate short- and long-term visioning exercises.
Determine if today’s data integration strategies will be sufficient in three years.
· Create requirements by mapping out the patient, provider and administrative journeys, and document the ideal movement of data across the enterprise.
Update the enterprise and information architectures to reflect the future state. Develop your five-year roadmap.
· Evaluate your existing vendors (including the EHR, population health, analytics and/or integration vendors) to determine if they have a roadmap and strategy to meet your demands.
Understand the specialty vendors in the market and track their evolution.
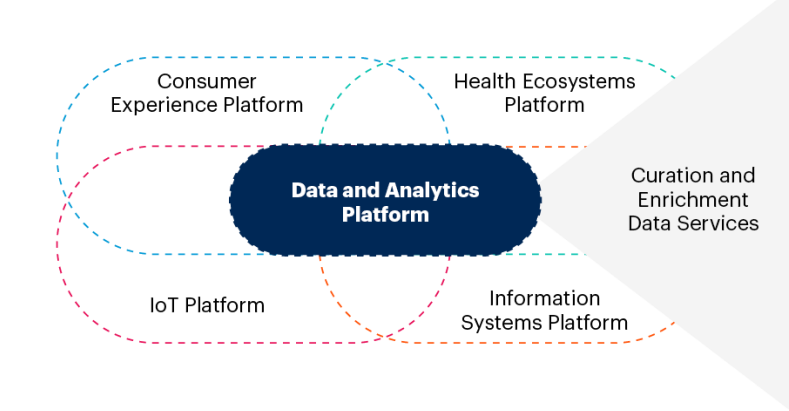
2.Move AI From the Lab to Value Realization by Focusing on the Human Elements of Culture and Adoption
While success with AI absolutely depends on having lots of clean and accurate available data, flexible database management tools, and advanced skills, there is an equally important component — the human element.
Good AI/data governance also accounts for human risk factors associated with adoption, such as cultivating trust, using AI with confidence and successfully blending the data science of AI with the art of how to use AI successfully in practice.
Consider the three dominant approaches to applying AI in healthcare, and the combination of data art and data science that is needed to apply them successfully:
· Augmented intelligence — The use of this term emphasizes the assistive role of devices and algorithms in diagnosing and treating patients, and that the AI isn’t intended to replace clinicians but to make care delivery more accurate and efficient.
The AI might provide amplified insights and/or suggest/recommend pathways, diagnosis or the next best action.
Clinicians need to be literate in understanding how to use the information to make better decisions by complementing their own training, knowledge and even intuition.
Noga Leviner, CEO of PicnicHealth, said, “It’s only going to become more obvious that doctors using their brains alone aren’t going to be as good as brains combined with algorithms.”[1]
“It’s only going to become more obvious that doctors using their brains alone aren’t going to be as good as brains combined with algorithms.
But this will not happen without elevated data literacy and training on how to incorporate AI into the decision-making process.
Indeed, the American Medical Association (AMA) has added augmented intelligence to its recommended training.[2]
Indeed, the American Medical Association (AMA) has added augmented intelligence to its recommended training
An AMA board member noted, “To realize the benefits for patient care, physicians must have the skills to work comfortably with augmented intelligence in healthcare … Educating physicians to work effectively with AI systems, or more narrowly, the AI algorithms that can inform clinical care decisions, will be critical to the future of AI in healthcare.”[3]
“To realize the benefits for patient care, physicians must have the skills to work comfortably with augmented intelligence in healthcare …
… Educating physicians to work effectively with AI systems, or more narrowly, the AI algorithms that can inform clinical care decisions, will be critical to the future of AI in healthcare.
· Actionable intelligence — Sometimes AI algorithms trigger an alert or warning that providers need to act on immediately.
This could be for a clinical condition such as sepsis, or an operational issue such as capacity strain.
In either case, the science of the algorithm is typically well-vetted and verified by the data scientists as well as the end users, so that the users can trust in the alerts the algorithm signals.
There is typically a set of prescribed protocols to follow once the alert is triggered, and in the case of sepsis any delay in following established protocols directly affects the likelihood of sepsis mortality.
The term “actionable intelligence” implies immediate action, and the algorithm has to be trusted explicitly — the accuracy and precision unquestionable.
This requires the science of quality data, rigorous testing, and ongoing validation and verification, eliminating as many false positives as possible.
It also requires the art of cultivating confidence and trust in its use by engaging end users in the end-to-end process, from development through deployment. Healthcare organizations advanced in their adoption of AI incorporate this culture into the deployment of their AI initiatives.
Consider the following quotes from peers at leading healthcare organizations regarding (successful) AI implementations:
· “To gain acceptance at the bedside, we didn’t just say, ‘The computer sees sepsis, start treating it.’ We said, ‘This is what the computer sees; do you agree?,’” said Jonathan Perlin, M.D., Ph.D., president of clinical services and CMO at a Nashville-based health system, on its implementation of its sepsis algorithm Sepsis Prediction and Optimization of Therapy (SPOT).[4]
· “A primary focus of Ochsner’s success is cultivating a cultural change among physicians,” said Richard Milani, M.D., chief clinical transformation officer, on Ochsner Medical Center’s implementation of an early warning system for clinical deterioration.[5]
· “Do not overestimate the level of knowledge your audience has on statistics and ML. It is a learning experience for all; you need to approach it with care and cooperation,” said Michael Thompson, executive director, enterprise data intelligence, Cedars-Sinai on its implementation of a capacity strain predictive model.[6]
· Automated intelligence — There are an abundance of processes for which providers are using AI to automate and eliminate simple and repetitive tasks by orchestrating robotic process automation (RPA) bots.
From clinical documentation to insurance preauthorizations, supply chain and claims processing, AI is changing the way people work.
This use of AI is the heart and the pulse of digital transformation.
There is, however, an immediate and even profound impact on the workforce that is vulnerable to replacement and therefore potentially resistant. Here you must master change management to make the workforce a party to the new norm.
You and your peers must be prepared to answer staff questions, including:
· How does AI automation fit into and/or change my workflow?
· How am I expected to modify my work?
· What are my new measures of success?
· Do I need new skills?
· Who gets blamed if the AI makes a mistake?
· What do you want me to do instead now that AI is making those decisions?
Ultimately, user adoption is dependent on the trustworthiness of the science, along with sensitivity and attention to the human element — how the introduction of AI will alter today’s way of doing something.
The more attention that you pay to explaining AI and bringing the end users into the process of deployment, the more likely it is that end users will see the value and benefit.
This is how value from AI is achieved across organizations leading in their use of and gleaning ROI from AI.
One recent media headline stated: “AI Adoption Growing Rapidly Among Health Industry IT Leaders.” This is very true. Another recent headline stated: “Adopting AI in Healthcare Will be Slow and Difficult.” This could also be true, but it doesn’t have to be the case.
CIOs can ensure they are extracting maximum value from AI investment by using an effective AI governance model to create a safe culture for introducing game-changing technologies into the workforce.
Figure 3 is from Gartner’s “Healthcare Provider CIOs: Get Ahead of AI Innovation WIth Strong AI Governance” research.
There are four essential components to the model:
1. Ethical evaluation and usage guidelines to vet appropriate use
2. Legal, regulatory and compliance reviews to deploy safely
3. Clinical and/or operational valuation of the AI, and data science verification of the model
4. The human aspect of deploying AI: organizational and cultural change management (the component emphasized in this document).
Figure 3: The Human Element of AI Governance
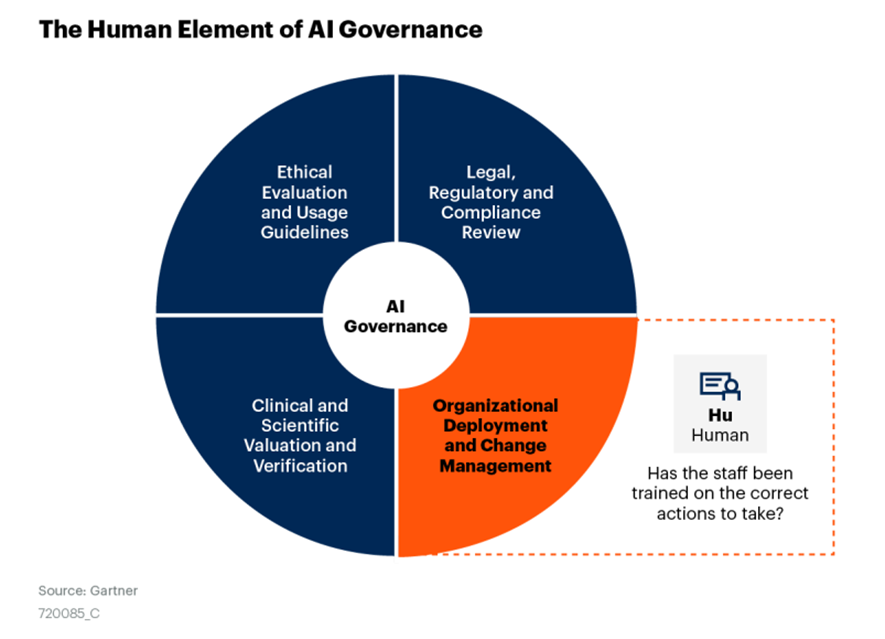
3.Drive Improved Enterprise Agility by Implementing Adaptive Data Governance and DataOps
CIOs and enterprise analytics leaders are delivering value along a spectrum of maturity and needs, and solving many different business problems.
A one-size governance model does not fit this diversity; governance models demand adaptable and flexible processes (see Table 1 and “Demystifying the Analytics and BI Space”). [See the original publication]
As healthcare analytics becomes more real-time and more critical to supporting more diverse, complex and mission-critical business and clinical processes, the traditional decision-support business and governance models fall short.
Pressure to deliver faster, with higher quality and with resiliency in the face of constant change (even more so in light of COVID-19), is causing CIOs and data and analytics leaders to rethink how their teams are organized and how they work.
Healthcare provider CIOs need to adopt two core governance best practices to be successful:
· Adaptive data governance — Many healthcare organizations are already using data and analytics to drive value, and CEOs have growing expectations that AI and advanced automation will augment, enrich and scale their digital health success.
However, if success is to be delivered and sustained, AI and data and analytics initiatives need governance and oversight from CIOs and analytics leaders that can adapt to the full range of business scenarios and requirements.
Flexibility is a core characteristic of adaptive governance — accepting that different business situations require varying degrees of liberty, autonomy, control and intervention.
As business requirements evolve, adaptive governance will help you keep up with change (see “Data and Analytics Leaders Must Use Adaptive Governance to Succeed in Digital Business”).
· DataOps — DataOps is a collaborative data management practice focused on improving the communication, integration and automation of data flows among data managers and data consumers across the organization.
Coupled with adaptive governance, DataOps techniques can address the challenges of multiple complex and differing demands through a more agile, collaborative and change-friendly approach to building and managing data pipelines (see “Your Data Culture Is Changing — Do You Need DataOps?”).
It’s essential to understand the current mix within your analytics portfolio and your ambition for the future portfolio mix to be able to balance your resources and align your governance and processes.
Many health systems are stuck in the world of compliance and quality reporting, with most information delivered via reports and dashboards.
Healthcare is very used to formal data models, structured sources of truth and the strict processes to deliver repeatable, precise quality measures and outcome reports.
These processes and structures are still vitally important for clinical, quality and compliance reporting.
But more advanced analytics and AI are applied to problems that are more complex, abstract and demanding than traditional reporting, and the data and analytics fundamentals are also very different.
At this tier of maturity, when advanced analytics and data science is being used, you are no longer supporting a one-size-fits-all analytics environment.
Along with new skills, talents and in some cases new organizational delivery models, you need to modernize your supporting governance and operational principles as well. Some benefits of making this change include:
· Making sure AI and advanced analytics projects don’t churn and fall into cycles of disappointment
· Providing better collaboration and creation across clinical, business and IT units
· Shifting to rapid and continuous delivery of new functionality, enabling the organization to keep pace with digital change, innovation and transformation
· Ensuring the ability to respond to unexpected events and/or emergencies
To succeed with AI, you must initiate a systematic review of your data governance processes.
Even if you have been doing data governance well, it is likely that your organization needs a modernization overhaul.
Make sure to align this effort with the reengineering of your data services; by doing so, you will realize many synergies.
Conclusion
2020 is the year that healthcare CIOs have a clear imperative to reinvent their data strategies, processes and governance.
Without a solid command of your data assets, your organization will fall short in delivering on your CEO’s digital transformation goals.
The spotlight that COVID-19 has put on the need for immediate access to real-time data and analytics (and how poorly many providers have performed) is the fuel for reigniting a serious commitment to a data-is-essential mindset.
Originally published at https://www.gartner.com