At Johns Hopkins Hospital, these algorithms provided ready access to state-of-the-art Covid-19 care for hospitalized patients, enabling clinicians to expertly manage the new illness in the midst of a crisis.
Harvard Business Review
by J. Hunter Young, Kyle Richardville, Bradley Staats, Brian J. Miller
May 06, 2022
Summary
Automated clinical algorithms promise to transform primary care.
Clinical algorithms specify the steps of a clinical process, leading from inputs such as a patient’s diagnosis to outputs such as recommendations for medication, further diagnostic testing, imaging, or even specialty referrals.
Once automated, they direct the care process without real-time clinician input.
Automated clinical algorithms can be as simple as age-based rules that trigger a scheduling call for a preventive vaccination or as complex as an automated clinical pathway specifying a series of tests and treatments for chronic conditions such as high blood pressure.
As has been demonstrated during the Covid-19 pandemic, done well, they enhance operational efficiency and maximize clinical quality.
But tapping their full potential will require adherence to principles developed over 75 years for automating the clinical trial — which we will discuss in this article.
Six core principles are discussed, to automate Medical Systems
1.First, do no harm: Safety must be a primary principle guiding the utilization of an automated clinical process.
2.Choice: Patients who meet the inclusion criteria for a specific automated process should be able to opt out.
3. Disclosure: To facilitate patients’ and clinicians’ choices, the automated nature of the decision-making process needs to be disclosed to them.
4. Personalization: There should be an opportunity for patients to “tell the algorithm” their personal preferences for treatment.
5. Degrees of automation: A clinical process may be fully or partially automated.
6. A learning health care system: Automated primary care will become a critical component of a learning and continuously adapting health care system.
As has been demonstrated during the Covid-19 pandemic, done well, (algorithms) enhance operational efficiency and maximize clinical quality.
But tapping their full potential will require adherence to principles developed over 75 years for automating the clinical trial — which we will discuss in this article.
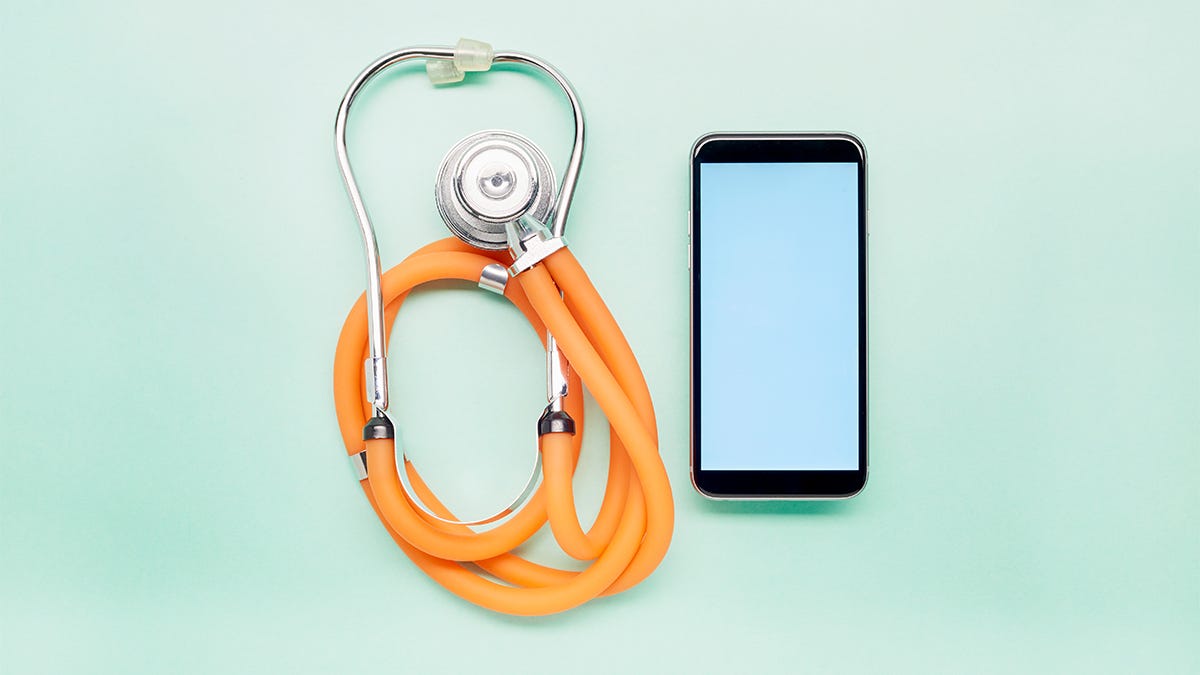
Structure of the paper
- Automated Algorithms for Covid-19 Care
- How to Automate Primary Care
- Six core principles, to automate Medical Systems
1. First, do no harm.
2. Choice.
3. Disclosure.
4. Personalization.
5. Degrees of automation.
6. A learning health care system
- Conclusion
Automated Algorithms for Covid-19 Care
Overwhelmed by the volume of Covid-19 patients in their communities, health systems have deployed online and phone-based automated triage tools to rapidly differentiate between those who can safely remain quarantined at home from those who may need more focused attention.
One approach included automated daily check-ins by phone or text message with patients at home and escalating care to a triage nurse as symptoms warranted.
The addition of a home-based blood-oxygen-measurement device improved the quality and actionability of data for sicker patients.
These automated solutions vastly increased the health system’s capacity to handle the surge of Covid-19 outpatients.
On the inpatient side, hospitals were overwhelmed with critically ill Covid-19 patients.
Exhausted clinicians, unable to continuously evaluate the surge of research studies examining Covid-19 and its treatment, needed help. In response, many institutions convened local experts to review the evidence and to generate recommendations for Covid-19 management.
In many cases, these recommendations were codified into algorithms, embedded into electronic health records systems, and automated through standardized order sets (a set of requests, such as lab tests and prescriptions, applied to a particular clinical situation).
One place that did this is the Johns Hopkins Hospital, the home institution of several of us.
These algorithms provided ready access to state-of-the-art Covid-19 care for hospitalized patients, enabling clinicians to expertly manage the new illness in the midst of a crisis.
At Johns Hopkins Hospital, the home institution of several of us … These algorithms provided ready access to state-of-the-art Covid-19 care for hospitalized patients, enabling clinicians to expertly manage the new illness in the midst of a crisis.
The use of automated algorithms to assist with Covid-19 follows a tradition of successful incorporation of decision-support algorithms for conditions such as heart failure, diabetes, and anticoagulation, which require ongoing monitoring and frequent medication-dose adjustment.
The prescribing clinician sets a therapeutic goal and prescribes a set of steps to achieve that goal.
That process is subsequently managed by other clinicians such as a nurses and pharmacists without real-time input from the prescribing physician.
These programs are effective due to their clinical focus and well-established guidelines and often reduce cost while maintaining or improving quality.
The use of automated algorithms to assist with Covid-19 follows a tradition of successful incorporation of decision-support algorithms for conditions
How to Automate Primary Care
Primary care, too, can be transformed through the broad application of automated algorithms, but this requires building automated clinical processes that are safe and effective.
Fortunately, the medical world has lots of experience with automated clinical processes in the form of clinical trials.
In trials, the intervention is specified in the form of an algorithm that guides the clinical process.
Primary care, too, can be transformed through the broad application of automated algorithms, but this requires building automated clinical processes that are safe and effective.
In trials, the intervention is specified in the form of an algorithm that guides the clinical process.
For example, in a trial of a new drug for high blood pressure, the trial’s algorithm will specify the frequency of blood pressure checks and the adjustment of the study drug’s dose based on the blood pressure level.
Despite the clinician’s lack of input, trial participants achieve their therapeutic goal safely, more quickly, and more consistently than patients treated in a traditional practice.
Automated primary care systems also employ algorithms that guide the process of care.
They codify the logic of a clinical process through specification of the steps leading from inputs such as patient factors, including diagnoses and biomarkers, to outputs such as recommended medications.
Automated primary care systems also employ algorithms that guide the process of care.
At the core of the approach to generating algorithms is a systematic evaluation and synthesis of clinical evidence.
Since medical knowledge evolves as evidence accumulates, algorithms must be updated as the evidence and experience with an automated process accumulates.
In addition, algorithms may need to consider feasibility of a range of treatment options.
For example, a recommended medication may be too expensive for the patient and therefore other less-expensive options can also be offered.
Of course, the trade-offs of selecting an inexpensive option, such as less convenience (e.g., once-daily versus twice-daily dosing), should be specified.
Finally, the process of algorithm development and modification requires independent oversight that’s focused on ensuring quality, safety, feasibility, and transparency.
For example, a committee comprised of an institution’s clinical experts, administrators, and patient representatives might review an algorithm’s impact on patient safety and satisfaction, clinical outcomes, and costs.
Delivery systems may also directly partner with software developers, whose algorithms may “crawl” existing medical literature in real time to update guidelines.
In turn, software developers should seek out medical professional specialty societies, which are often well-versed in the limitations of existing research.
Finally, the U.S. Food and Drug Administration (FDA), as the nation’s medical device regulator, has a role to play.
It already exerts flexible, focused oversight of “ software as a medical device” — software with an intended medical use that functions independent of a hardware medical device.
Finally, the U.S. Food and Drug Administration (FDA), as the nation’s medical device regulator, has a role to play.
It already exerts flexible, focused oversight of “ software as a medical device” — software with an intended medical use that functions independent of a hardware medical device.
While medical devices are traditionally subject to the FDA’s risk-based regulatory framework, software developers will soon have an alternative pathway to obtaining regulatory approval:
the FDA’s voluntary digital health precertification pathway. Introduced in 2017 with nine companies as part of a pilot, it provides oversight of clinical algorithms and artificial intelligence and it can decrease “time to market.”
- It utilizes an upfront, total-product-lifecycle approach to medical software oversight that focuses on both the developer’s processes — such as risk management, configuration management to keep the process in the desired state,
- and change management (altering the process for new goals and objectives) — and product-specific analyses (e.g., to verify claims about the product’s performance).
This approach means low-risk products could be marketed without additional FDA review, speeding patient access to improved products.
Furthermore, high-risk products would undergo streamlined FDA review, reducing preparation and review time and cost.
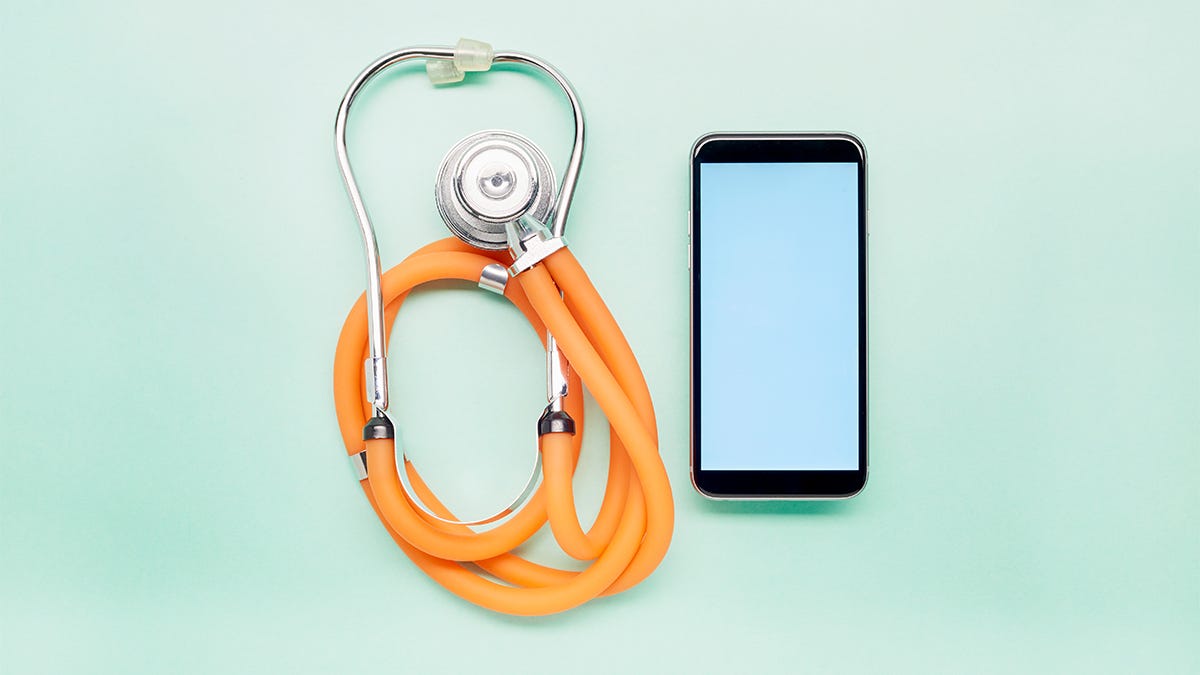
Six core principles, to automate Medical Systems
Irrespective of how oversight and accountability are achieved, it is crucial that the development and application of the automated medical system adhere to six core principles, which are derived from organizations’ extensive experience in automation through clinical trials.
1. First, do no harm.
Safety must be a primary principle guiding the utilization of an automated clinical process.
Strict inclusion and exclusion criteria are an important mechanism for ensuring safety.
Here’s an example: Participants in clinical trials usually represent a small subset of the types of patients who may benefit from the therapy under investigation.
A trial’s strict inclusion and exclusion criteria are used to limit participants to those who are most likely to benefit and least likely to be harmed.
Similarly, participation in an automated process should be limited to maximize safety.
Patients with uncomplicated conditions will be more likely to qualify for inclusion in a given automated process, while those with more complex needs will be managed through traditional clinician-intensive pathways.
This distinction is key as it enables clinicians to spend more time with complex patients who need the additional attention, thus allowing clinicians to practice closer to the top of their license.
As evidence accumulates, the safety of including a wider range of increasingly complex patients can be evaluated and the scope of the automated process expanded.
2. Choice.
Patients who meet the inclusion criteria for a specific automated process should be able to opt out.
In practice, a common output of an automated primary care process will be a treatment recommendation.
Therefore, the patient, with the care provider’s support, should make the final decision regarding whether to follow the recommendation.
Similarly, clinicians should be able to opt out of a given automated process for their patients.
The potential consequences of opting out, such as delays in care and higher cost, should be clearly communicated, and health care systems should follow up with those who opt out to better understand the reasons — information they can use to improve the design of the clinical process.
The potential consequences of opting out, such as delays in care and higher cost, should be clearly communicated, and health care systems should follow up …
3. Disclosure.
To facilitate patients’ and clinicians’ choices, the automated nature of the decision-making process needs to be disclosed to them.
The logic and evidence used to determine the recommendation should be clearly communicated.
For example, a recommendation to prescribe a particular medication for hypertension would be based on risks of not taking the medication, such as suffering a future stroke, versus the risk of taking the medication, such as potential side effects.
An important challenge will be communicating complex factors such as probabilities of clinical outcomes and the timing and severity of those outcomes.
4. Personalization.
There should be an opportunity for patients to “tell the algorithm” their personal preferences for treatment.
For example, some patients prefer to use lifestyle approaches to manage chronic conditions (e.g., managing their weight and their weight-related conditions by exercise and diet); others would rather take medication for high cholesterol than modify their diet.
These preferences can impact the algorithm’s set of recommendations.
5. Degrees of automation.
A clinical process may be fully or partially automated.
For example, the automated phone-based tool for triaging Covid-19 patients described above was fully automated and required no clinician input.
In contrast, a clinical process may be only partially automated — for example, when a treatment recommendation is the output but the patients and their providers decide whether to take it.
Partially automated processes can provide robust support to help clinicians make decisions, can reduce the variability in the decisions that they make, and can help minimize their deviation from evidence-based guidelines.
Key branch points in an algorithm may require a decision by the patient or clinician.
For example, the clinician may specify a treatment goal and engage an automated process to achieve that goal.
In this case, the clinician is essentially prescribing a process of care rather than a specific medication and dose.
Examples include algorithms dedicated to the management of heart failure, diabetes, and anticoagulation, where non-prescribers adjust medications, provide patient education, and clinically assess patients within the confines of an already diagnosed condition.
In these cases, clinician involvement in the process may remain important for safety and patient acceptance.
In this case, the clinician is essentially prescribing a process of care rather than a specific medication and dose.
Key branch points in an algorithm may require a decision by the patient or clinician.
Many patients believe that their medical needs are unique and cannot be adequately addressed by algorithms, which can be viewed as inflexible.
In reality, algorithms may be able to deliver more individualized care due to their capacity to account for more factors than a clinician can consider.
Many patients believe that their medical needs are unique and cannot be adequately addressed by algorithms, which can be viewed as inflexible.
In reality, algorithms may be able to deliver more individualized care due to their capacity to account for more factors than a clinician can consider.
6. A learning health care system.
Automated primary care will become a critical component of a learning and continuously adapting health care system.
Through measurement of predictors and outcomes, combined with rigorous approaches to structured implementation, automated systems could evaluate the impact of novel therapies on clinical outcomes, patient satisfaction, and costs.
These evaluations will need to be thoughtfully designed to minimize the impact of biases.
Subsequent modifications of the algorithms based on the accumulating evidence can be manual or automated.
But as the degree of automation increases, the degree of local oversight by users and health systems must also increase.
Through measurement of predictors and outcomes, combined with rigorous approaches to structured implementation, automated systems could evaluate the impact of novel therapies on clinical outcomes, patient satisfaction, and costs.
These evaluations will need to be thoughtfully designed to minimize the impact of biases.
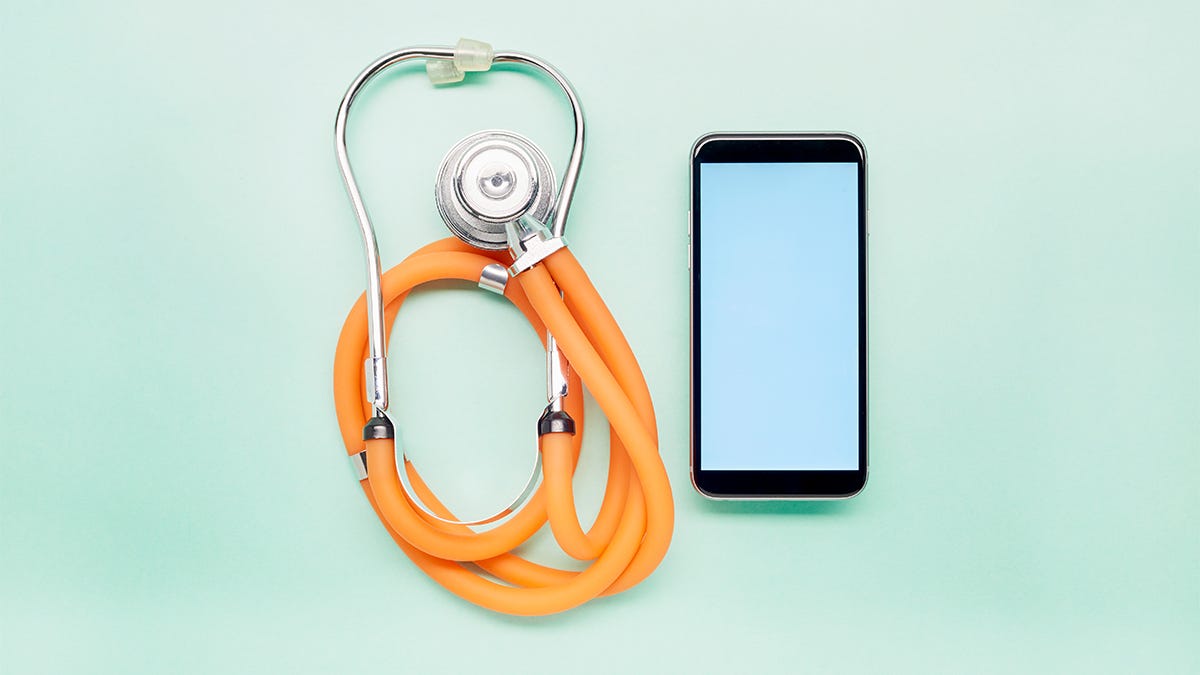
Conclusion
Accelerated by the Covid-19 pandemic, automation has arrived in primary care.
Once they’re built following the principles derived from our long experience with clinical trials, automated algorithms will ensure clinical quality, enhance operational efficiency, and accelerate the pace of innovation.
Done well, automation promises to play a crucial role in the next transformation of health care.
Originally published at https://hbr.org on May 6, 2022.