9.Connected Governance
10.AI Risk Management
11.Vendor and Regional Ecosystems
12.Expansion to the Edge
This is an excerpt of the publication below, with the title above, focusing on the topic(s) in question. For the full version of the article, please, refer to the original publication.
Gartner
Top Trends in Data and Analytics, 2022
By Rita Sallam, Ted Friedman, and 37 more
11 March 2022
Excerpt by
Joaquim Cardoso MSc.
The Health Revolution
Multidisciplinary Institute for Better Health for All
May 27, 2022
Top Trends in Data and Analytics
The top D&A trends represent business, market and technology dynamics that you cannot afford to ignore
They have the potential to transform your enterprise, and will accelerate in their adoption over the next three years.
You should decide whether to proactively monitor, experiment with or aggressively invest in key trends based on their urgency and alignment to strategic priorities.
The top D&A trends and technologies do not exist in isolation; they build on and reinforce one another.
We have selected our trends for 2022 in part based on their combined effects. Taken together, our top data and analytics technology trends for 2022 will help you meet your organization’s top strategic priorities to anticipate, adapt and scale value.
This, in turn, will enable you to encourage innovation and put in place success metrics and incentives that emphasize learning and reward innovation.
Top Trends in Data and Analytics
- Group 1: Activate Dynamism and Diversity
- Group 2: Augment People and Decisions
- Group 3: Institutionalize Trust
Group 3: Institutionalize Trust
9.Connected Governance
10.AI Risk Management
11.Vendor and Regional Ecosystems
12.Expansion to the Edge
Key prognosis
- By 2026, 20% of high-performing organizations will use connected governance to scale and execute on their digital ambitions.
- By 2026 organizations that develop trustworthy purpose-driven AI will see over 75% of AI innovations succeed, compared to 40% among those that don’t.
- By 2024, 50% of new system deployments in the cloud will be based on a cohesive cloud data ecosystem, rather than on manually integrated point solutions.
- By 2025, more than 50% of enterprise-critical data will be created and processed outside the data center or cloud.
By 2025, more than 50% of enterprise-critical data will be created and processed outside the data center or cloud.
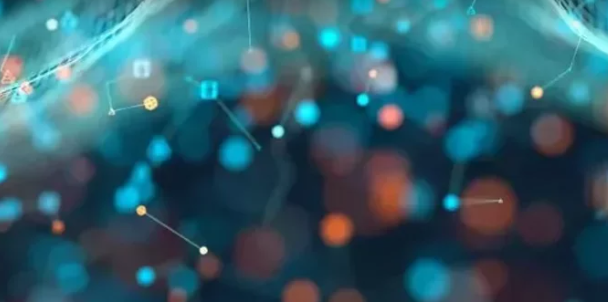
9.Connected Governance
Analysis by: Andrew White, Saul Judah, Ted Friedman, Guido De Simoni
SPA:
By 2026, 20% of high-performing organizations will use connected governance to scale and execute on their digital ambitions.
By 2026, 20% of high-performing organizations will use connected governance to scale and execute on their digital ambitions.
Description:
Connected governance is a framework for establishing a virtual D&A governance layer across organizations, business functions and geographies, to achieve cross-enterprise governance outcomes. Connected governance provides a means to connect disparate governance efforts, including D&A governance, across different organizations, both physical and virtual, as well as geographies. This approach is similar to virtualized governance efforts, but with at least one difference: virtualized governance organizations are new and discrete teams with an independent objective. Connected governance does not exactly create a new team, but helps connect those that are already established. It also provides a means to align and link efforts so that complex outcomes shared by the organizations and geographies can be achieved.
Why Trending:
- As the anticipated transformation across most industries materializes, organizations that are unable to address cross-organizational, cross-geographical challenges in a flexible and agile way will be left vulnerable to competition or fail to meet political pressures in the public sector.
- The accelerating pace and complexity of digitalization is putting pressure on senior leaders across multiple business functions to respond to business and mission demands. Similarly, governments are consolidating organizations that are often driven by a need to provide more effective and comprehensive services or regulatory regimes.
- Organizations need effective governance at all levels that not only addresses their existing operational challenges, but is also flexible, scalable and highly responsive to changing market dynamics and strategic organizational challenges.
Implications:
- New demands for cross-enterprise responses and initiatives will require accountability from multiple executive leaders. This will require knowledge of local governance accountability and how decision rights are implemented.
- Connected governance will be a mechanism to bring together and coordinate diverse business areas to avoid time-consuming, watered down or even conflicted decisions and mediocre action and performance.
Recommendations:
Data and analytics leaders should:
- Identify the business scenarios and outcomes that are most difficult to govern in the ecosystem because of, for example, geographic and organizational diversity, complexity and autonomy.
- Build trust in data-driven decision making by setting up connected governance of all data assets in the enterprise.
- Consider how connected governance can help to address these challenges more effectively.
Build trust in data-driven decision making by setting up connected governance of all data assets in the enterprise.
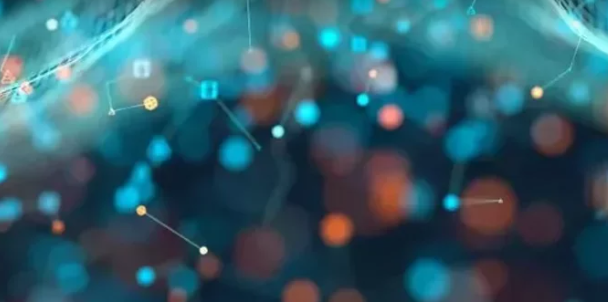
10.AI Risk Management
Analysis by: Avivah Litan, Bart Willemsen, Svetlana Sicular, Sumit Agarwal, Farhan Choudhary
SPA:
By 2026 organizations that develop trustworthy purpose-driven AI will see over 75% of AI innovations succeed, compared to 40% among those that don’t.
By 2026 organizations that develop trustworthy purpose-driven AI will see over 75% of AI innovations succeed, compared to 40% among those that don’t.
Description:
The speed of AI innovation is increasing pressure to keep pace while keeping businesses operating, tempting organizations to cut corners on AI trust, risk and security management (TRiSM). This will lead to potentially harmful outcomes. Organizations must spend time and resources now on supporting AI TRiSM. Those that do will see improved AI outcomes in terms of adoption, achieved business goals and both internal and external user acceptance.
Why Trending:
- Almost half of AI models developed by experienced organizations do not make it into production, and users cite security and privacy as a primary reason for this negative outcome (see Survey Analysis: Moving AI Projects From Prototype to Production).
- AI is becoming more pervasive, yet most organizations cannot interpret or explain what their models are doing. They struggle to ensure trust and transparency in their models.
- Most organizations developing AI:
- Are not precise on what they want to achieve when developing models; and often expand the scope when they see what is possible, making operationalization almost impossible.
- Have no processes, tools or measurements to govern and manage model trust, risk and security.
- Tend to gather AI training data without deliberate data selection goals. Data is often biased and inadequate for training models.
- Are driven mainly by regulatory compliance when it comes to model governance. However, compliance does not necessarily lead to trustworthy models.
- The increasing dependence on, and scale of, AI escalates the impact of misperforming AI models with severely negative consequences.
- AI regulations are proliferating across the globe, mandating certain auditable practices that ensure trust, transparency and consumer protection.
- Organizations are not prepared to manage the risks of fast-moving AI innovation and are inclined to cut corners around model governance.
- Organizations that properly and continuously govern their models have much improved AI model outcomes.
Implications:
- Increased focus on AI TRiSM will lead to:
- Controlled and stable implementation and operationalization of AI models.
- Far fewer AI failures, including incomplete AI projects, and a reduction in unintended or negative outcomes.
- Positive societal impact if AI is trustworthy and has a legitimate purpose, leading to reduced discrimination, more fairness and protection of human autonomy and privacy.
- In contrast, AI models that are not trustworthy or transparent and do not have a legitimate purpose can eventually lead to severely negative consequences.
Recommendations:
Data and analytics leaders should:
- Engage all internal and external stakeholders from the start to foster transparency and trust.
- Define in full the primary purpose of AI, and use AI engineering and governance to develop metrics to continuously assess the intended impacts of the models.
- Account for the parameters of the entire ecosystem in which change is to be made, in addition to focusing on the primary purpose of the AI model itself.
- Make sure the right amount of the right data is available to train the model to achieve balance, improve accuracy and mitigate bias.
- Consider tools such as synthetic data to generate more useful training data when trusted sources do not suffice.
- Reach beyond compliance by involving the ethics board or other interested parties in creating purposeful unbiased models.
Engage all internal and external stakeholders from the start to foster transparency and trust.
Make sure the right amount of the right data is available to train the model to achieve balance, improve accuracy and mitigate bias.
Consider tools such as synthetic data to generate more useful training data when trusted sources do not suffice.
Changes Since Last Year
The rising interest in privacy-enhancing computation (PEC) techniques (see Top Strategic Technology Trends for 2022: Privacy-Enhancing Computation for an introduction) is a promising support factor for this trend. Particularly, the expedient adoption of generative AI-based synthetic data (see Hype Cycle for Privacy, 2021) will aid in providing non-identifiable, privacy risk-free data to train AI models on.
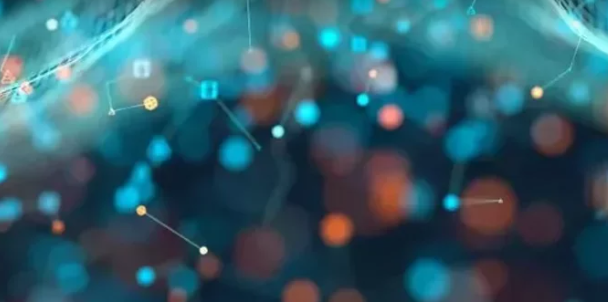
11.Vendor and Region Ecosystems
Analysis by: Rita Sallam, Julian Sun, Adam Ronthal, Carlie Idoine, Sumit Agarwal
SPA:
By 2024, 50% of new system deployments in the cloud will be based on a cohesive cloud data ecosystem, rather than on manually integrated point solutions.
By 2024, 50% of new system deployments in the cloud will be based on a cohesive cloud data ecosystem, rather than on manually integrated point solutions.
Description:
D&A ecosystems consisting of increasingly comprehensive D&A capabilities are growing in availability and capabilities. Both CSPs and ISVs are offering less costly, less complex architecture requiring little or no integration of products and cloud services. At the same time, many global organizations are assessing the implications of building parallel regional D&A ecosystems to comply with local regulation. Data sovereignty, financial governance and orchestration across components are key ecosystem considerations.
Why Trending:
- CSPs increasingly view compute- and data-intensive D&A workloads as an attractive vehicle for overall cloud services revenue growth.
- Most CSPs and ISVs are using varying degrees of workflow integration with the promise of composability, AI-driven automation and capabilities convergence across D&A categories. The aim is to deliver lower cost, shorter time to deployment and agility as a key competitive strategy to respond to buyer needs.
- Cloud data ecosystems redefine the best-of-breed and best-fit engineering versus suite debate. By resolving disparate and increasingly siloed D&A, they can alleviate some of the challenges of multicloud, intercloud and hybrid deployments. Unlike with the on-premises stacks of the past, with cloud ecosystems, the vendor lock-in challenges of using a single vendor may be outweighed by the potential benefits.
- Regional ecosystem pull is accelerated by regional data security laws, which intensify data gravity and require better regional composability between vendors. This pushes global organizations to consider migrating and duplicating some or all parts of their D&A stack within specific regions.
Implications:
- Over the past several years, many once-separate D&A software markets have been converging, driven by increased adoption in the cloud and buyer demands for faster deployments and lower cost. Greater workflow integration between previously separate products has enabled this trend.
- Although there is a need for third-party tools to fill in elements of the D&A ecosystem, this should diminish over time via innovation in the D&A ecosystem and through acquisitions and cross-category organic development.
- Unlike stack-centric market swings in the past, innovation in the market will thrive, but not just to the benefit of small, innovative vendors. CSPs might not be the main drivers of innovation; because of cloud agility and composability, they are fast followers and acquirers of innovation introduced into the market by smaller, nimble vendors.
- Most large organizations will, by design or by default, have to manage a multicloud and multivendor strategy. However, because it requires additional skills, time and cost to build and manage applications built from multiple vendor product components, multicloud and intercloud tooling will evolve to make it far easier to deal with.
- Regions not able to create or sustain their own D&A ecosystems will have no choice but to leverage capabilities created in other regions (hyperscalers) and resort to legislation and regulation to maintain some level of control and sovereignty.
Recommendations:
Data and analytics leaders should:
- Begin by evaluating the extensibility and broader ecosystem offerings for vendor solutions already in use and consider aligning with them.
- Lower costs and improve organizational resilience by evaluating D&A vendors on the availability, strength and — importantly — the integration of their broader ecosystem of D&A capabilities.
- Evaluate not only extensibility, cost, agility and speed, but also coherence and consistent ease of use and workflow across the CSP-based D&A ecosystem.
- Reevaluate policies favoring a best-of-breed or best-fit strategy for end-to-end D&A capabilities in the new cloud world by weighing the benefits of a single vendor ecosystem in terms of cost, agility and speed.
- Consider regional composability while assessing the global deployment of D&A solutions by understanding their technology partnerships and integration with regional D&A ecosystems.
Lower costs and improve organizational resilience by evaluating D&A vendors on the availability, strength and — importantly — the integration of their broader ecosystem of D&A capabilities.
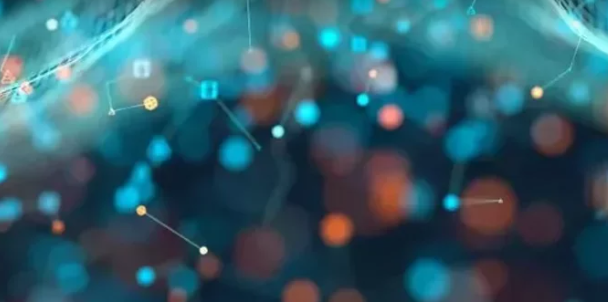
12.Expansion to the Edge
Analysis by: Ted Friedman, Pieter den Hamer, W. Roy Schulte, Paul DeBeasi
SPA:
By 2025, more than 50% of enterprise-critical data will be created and processed outside the data center or cloud.
By 2025, more than 50% of enterprise-critical data will be created and processed outside the data center or cloud.
Description:
D&A activities are increasingly executed in distributed devices, servers or gateways located outside data centers and public cloud infrastructure, closer to where the data and decisions of interest are created and executed.
For example, the memory, storage and compute capacities of hardware built into various types of endpoint devices continue to expand, making larger, more sophisticated workloads possible.
Despite these advances, edge environments bring substantial constraints on resources and flexibility, and the functionality they offer for D&A workloads is different from that offered by data centers or cloud environments.
This means D&A leaders and their teams must enhance their skills and rebalance their architectures.
D&A activities are increasingly executed in distributed devices, servers or gateways located outside data centers and public cloud infrastructure, closer to where the data and decisions of interest are created and executed.
This means D&A leaders and their teams must enhance their skills and rebalance their architectures.
Why Trending:
- Data, analytics and the technologies supporting them increasingly reside in edge computing environments, closer to assets in the physical world and outside IT’s traditional purview.
- A diversity of use cases is driving the interest in edge capabilities for D&A, ranging from supporting real-time event analytics to enabling autonomous behavior of “things.”
- Edge-generated data is growing dramatically in terms of volume and diversity. Since it often won’t be desirable or possible to collect and process this data centrally, organizations need to support distributed data processing and persistence models.
- Digital business solutions increasingly demand faster data distribution and reduced latency, requiring a shift of data and data processing away from the cloud and traditional data center environments.
- Data sovereignty and solution reliability concerns are generating interest for data to be stored “locally” in edge environments.
Implications:
- By placing data, analytic workloads and AI capabilities at optimal points ranging out to endpoint devices, interesting new applications of analytics and AI are coming into focus for D&A leaders and their teams, including more real-time use cases.
- By using distributed computing resources and spreading the load across the ecosystem, D&A teams can more broadly scale their capabilities and extend their impact into more areas of the business.
- Pushing D&A capabilities toward edge environments can also bring benefits in the form of greater fault tolerance, remote monitoring, remote operations and autonomous behavior.
- With the distribution and complexity of edge environments comes a greater challenge from a D&A governance perspective — ensuring quality, security, privacy and consistency definitions/models are all more difficult.
Recommendations:
Data and analytics leaders should:
- Evolve their strategies and practices to de-emphasize centralized approaches and architectures, while developing new capabilities that can be deployed, executed and administered in various locations along the cloud-to-device continuum.
- Develop skills and experience in edge environments, including complex solutions and governance. Communicate and collaborate with stakeholders and teams that have historically been outside the sphere of influence of traditional D&A, e.g., operational technology (OT) teams.
- Provide support for data persistence in edge environments by including edge-resident IT-oriented technologies (relational and nonrelational database management systems), as well as small-footprint embedded databases for the storage and processing of data closer to the device edge.
- Optimize distributed data architectures for their use cases by balancing the latency requirements against the need for data consistency (between cloud/data center and edge, as well as across edge environments).
- Use edge topology to address data sovereignty and availability requirements while minimizing risk by extending D&A governance capabilities to edge environments and providing visibility through active metadata.
Changes Since Last Year
The main change in this trend compared to last year is the degree of acceleration. Deployment of edge D&A solutions has continued to grow due to two main forces: the demand for automation and control of remote environments to mitigate health, safety and resourcing constraints, and the increasingly complex regulatory landscape emphasizing data sovereignty.
Evolve their strategies and practices to de-emphasize centralized approaches and architectures, while developing new capabilities that can be deployed, executed and administered in various locations along the cloud-to-device continuum.
Originally published at https://www.gartner.com
About the authors
By Rita Sallam, Ted Friedman, Erick Brethenoux, Donald Feinberg, Soyeb Barot, Lydia Clougherty Jones, Malcolm Hawker, Eric Hunter, Jason Medd, Robert Thanaraj, Melody Chien, Mark Beyer, Ehtisham Zaidi, Mayank Talwar, Pieter den Hamer, W. Roy Schulte, Paul DeBeasi, David Pidsley, Sumit Pal, Joe Maguire, Yefim Natis, Shaurya Rana, Guido De Simoni, Afraz Jaffri, Alan D. Duncan, Julian Sun, Gareth Herschel, Avivah Litan, Bart Willemsen, Svetlana Sicular, Farhan Choudhary, Sumit Agarwal, Mike Fang, Adam Ronthal, Andrew White, Carlie Idoine, Jorgen Heizenberg, Peter Krensky, Sally Parker