The potential for automation and AI in health care
This is an excerpt of the paper “Switched on” — How do we get the best out of automation and AI in health care?”, focused on Chapter 2 of the report.
The Health Foundation
Tom Hardie, Tim Horton, Matt Willis, Will Warburton
June, 2021
Index
- 2.1 Types of task amenable to automation
- 2.2 Application to administrative tasks in health care
- 2.3 Application in clinical services
2.1 Types of task amenable to automation
There are different ways of analysing and understanding tasks when considering what kinds of work can be automated and what cannot.
One influential approach, by economist David Autor, focuses on the broad nature of tasks and views the degree of routine involved in a task as a key determinant of automatability.
Autor categorised tasks based on two properties: routine versus non-routine, and manual versus cognitive. Routine manual tasks require what Autor calls a ‘methodical repetition of an unwavering procedure’, such as picking and sorting items and repetitive assembly — the kind of repetition that could be explicitly programmed and performed by machines. Non-routine manual tasks, on the other hand, such as caretaker work and truck driving, are considered to have more limited scope for automation in Autor’s model. Routine cognitive tasks include record keeping and repetitive customer services like bank clerk work, and these are thought to offer substantial room for automation. Finally, non-routine cognitive tasks, which include medical diagnosis, legal writing and sales, are not seen to be candidates for automation and are viewed as more likely to remain (at least partially) in the domain of human performance.
Autor’s analysis has shone a light on labour market trends such as the ‘disappearing middle’, whereby — contrary to earlier predictions — many manual, non-routine jobs have remained resistant to automation, while other ‘white collar’ jobs, such as clerical work, have been automated. In these cases, it has been routine work that has been susceptible to automation, whether manual or cognitive, while non-routine work has proved harder to automate.
Autor’s analysis has been influential over many years, though technological advancements have led to certain non-routine tasks becoming easier to automate — truck driving, for example. Due to these advancements, some argue that automation can be extended to any non-routine task that is not subject to any ‘engineering bottlenecks’, which we discuss in more detail in Chapter 3.
This way of looking at tasks suggests that automation will tend to have most impact when applied to tasks that are frequent or time consuming, important and repetitive (though there can sometimes be benefits to applying automation to infrequent tasks too — for example, when a drug is rarely prescribed — as it is often these types of task where mistakes are most likely to occur).
Such repetitive, rule-based tasks frequently lend themselves to a form of automation known as robotic process automation (RPA), which uses software to enable transaction processing, data manipulation and communication across multiple IT systems.
RPA has successfully been deployed in many sectors to process invoices, claims and payments, monitor error messages and respond to routine requests from customers and suppliers.3
While RPA is not widespread in health care, a number of NHS trusts have successfully used it to manage scheduling and other processes, and NHSX is exploring how RPA can be scaled in the NHS through its Digital Productivity Programme.
A second approach to considering which kinds of task are amenable to automation is to analyse the skills and knowledge they require.
The Oxford study used the O*NET database’s ‘occupation features’ to analyse how predictions of a task’s automatability related to the different skills and knowledge required. O*NET is a database of hundreds of standardised descriptors of almost 1,000 occupations in the US economy, which includes information on the skills, knowledge, work activities and interests associated with these occupations. The researchers looked at which features were clearly predictive of automatability and also at where an increase in the presence of a particular feature led to an increase in predicted automatability.
Table 1 presents the five largest (positive) percentage differences in O*NET occupational features for tasks that were rated automatable, not automatable and partly automatable, when compared to the entire dataset of health care tasks.
Health care tasks predicted as ‘not-automatable’ require 26.0% higher personnel and human resources knowledge, 17.0% higher education and training knowledge and 18.7% higher management and financial resources skills. By contrast, ‘automatable’ tasks require 24.4% higher clerical skills.
Table 2 presents the occupational features with the largest positive influence on predicted task automatability, given an increase of the particular feature. Telecommunications and clerical knowledge have the largest gradient, meaning that if a task requires more of this, then its automatability score will increase to a greater extent than increasing other features.,
So these analyses suggest there might be a range of tasks in health care, especially clerical tasks, that could be promising candidates for automation.
Table 1: Largest feature differences relative to dataset by automatable category
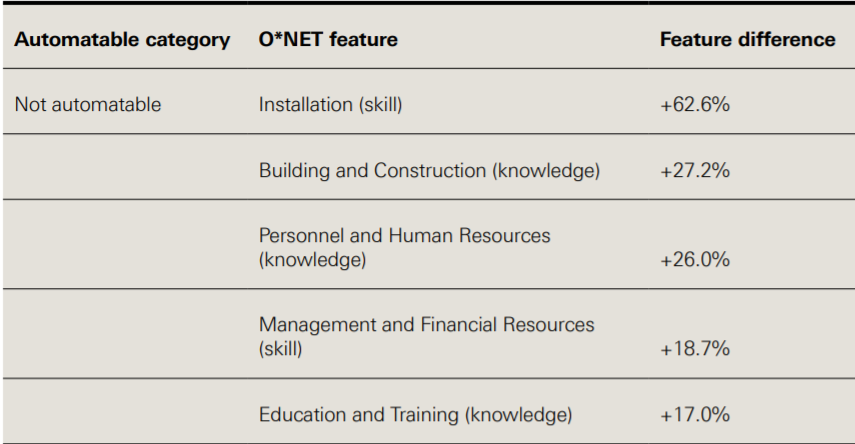
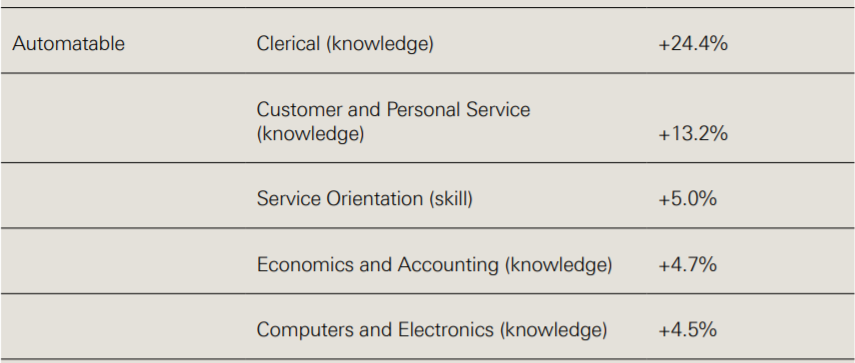
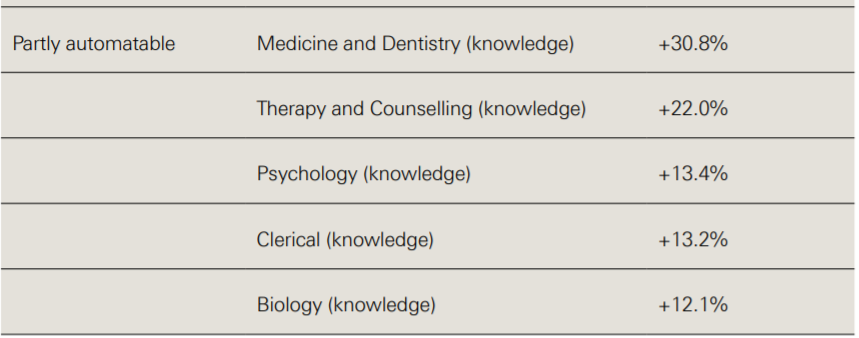
Source: Willis M, et al.3
Table 2: Ten largest O*NET occupational feature gradients
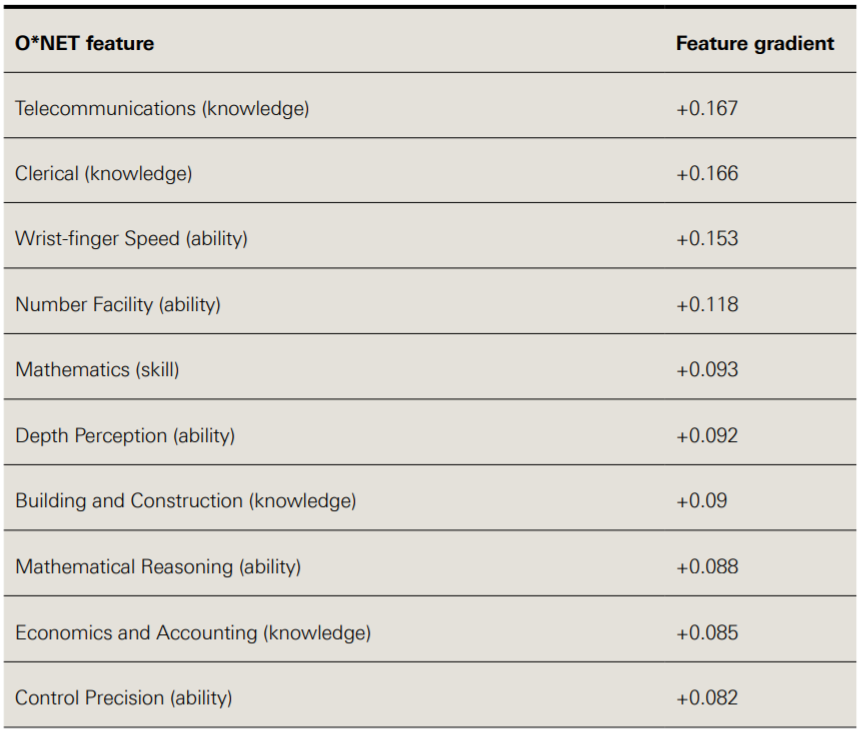
A third approach to thinking about the types of work that can be automated is to look at different task ‘functions’ and consider what level of automation might be appropriate in each case.
To take one example, drawing on the different stages of human information processing, Parasuraman and colleagues describe four system functions that can potentially be automated: information acquisition, information analysis, decision and action selection, and action implementation. For each type of function there are different possible levels of automation.
For example, with regard to decision making, a computer could suggest options to support human decision making, on the one hand, or it could actually make a decision and act autonomously, on the other; and there may be further levels in between, such as having a computer make the decision but still with the ability of a human operator to override it if necessary.
Parasuraman and colleagues argue that a variety of factors will be relevant to determining the appropriate level of automation in different cases, especially the reliability of the automation technology, the potential impact on human performance in the resulting system and, crucially, the risks associated with decisions. So, for example, looking at air traffic control systems, they suggest that in the case of information acquisition and analysis, high levels of automation could be appropriate, while in the case of decision selection and implementation high levels of automation would only be appropriate for low-risk situations.
Work in health care is often complex and carries particular risks, with different kinds of tasks and work environments compared to other industries, and different legal, governance and institutional requirements.
These factors might make it hard to translate some applications of automation from other industries to health care.
This is explored in more detail in Chapter 3. First, we take a look at some specific areas where automation can be, and is being, applied in health care.
2.2 Application to administrative tasks in health care
Here we use the term ‘administrative’ loosely to mean tasks involved in the management or organisation of health care services, rather than in the direct delivery of them. Some administrative tasks may sit separately from clinical work, such as managing finances and payroll, while others may be embedded within it, such as processing prescriptions and managing hospital bed capacity.
Given that much administrative work involves routine information processing, the discussion above suggests that there may be significant opportunities for the automation of administrative tasks in health care. And with a high volume of administrative work in the NHS, it is easy to see why there is such appetite to take advantage of these opportunities, with the NHS Long Term Plan and NHSX both highlighting the potential of technology for reducing the burden of administrative work. Some administrative processes in health care are already being automated in particular settings, such as appointment booking and scanning letters in GP practices. However, advances in data availability, computational power and machine learning are creating possibilities for further automation.
The Oxford study estimated that around 44% of administrative tasks in general practice are ‘mostly’ or ‘completely’ automatable using current technology, based on its model derived from the opinions of automation experts (though, notably, the study also found that no single full-time role in general practice could be entirely automated).
The research suggested that tasks such as managing finances and payroll, checking and sorting post, printing letters, texting patients, note-taking and letter scanning could all theoretically be automated.
Given that such tasks require a considerable amount of time in any GP practice (including the time of GPs themselves), automating them could have a significant, positive impact in reducing the administrative burden and freeing up staff to focus on other work, particularly in light of current financial and workforce pressures.
Automation could make a particular difference with information-processing tasks that are time consuming and which have an important bearing on resources and patient care, such as in the management, scheduling and planning of clinical services.
For example, in order to address high outpatient non-attendance rates, East Suffolk and North Essex NHS Foundation Trust has automated patient appointment reminders and cancellations using a simple text messaging process, reallocating free bookings when cancellations are made.
The Trust claims that in 2018 this prevented 1,356 appointments being missed over a period of eight weeks, equivalent to a value of £216,960.
Given the issue of non-attendance is common across health care services, this type of application could be useful in a range of other settings beyond secondary care, such as primary, community and mental health services.
Appointments and scheduling are also an area where AI and predictive analytics could add an extra dimension to automation.
For example, Beth Israel Deaconess Medical Center in Boston is using the automated analysis of historical data to predict how much time a patient might need in the operating theatre and then using this to inform scheduling.
AI can also help with the management of resources such as hospital beds.
For example, funded by the Health Foundation, Chelsea and Westminster NHS Foundation Trust has developed a model to predict rises in acute hospital bed occupancy (discussed in more detail in Chapter 3), which could be deployed to provide an early warning system that gives staff sufficient time to avert occupancy crises.
Advances in natural language processing (NLP), which can extract structured (machine analysable) data from unstructured narrative texts (such as clinical notes and letters), mean that automation technologies have increasing potential to support text-based administrative work, for example processing and producing letters — activities that require a significant amount of time across the NHS.
In general practice, the Oxford study found that letter work — such as opening letters, triaging, scanning and redirecting them to relevant staff members, and responding to them in different ways — entails a large amount of work, which is typically undertaken by receptionists and secretaries, but also often by GPs.
The study also found that a significant amount of time is spent trawling through letters to find small amounts of relevant information.
Automation technologies using NLP could therefore be used to scan for relevant information and present it to health care professionals, prioritising information by context and urgency, as well as to produce letters automatically as patients have their examinations.
In addition to helping make letter management in the NHS more efficient and less time consuming, such approaches could also open up opportunities for a wider range of communications options for patients, tailored to their needs and preferences, such as emails, texts, large print, braille, audio recordings and language translations.
Another way NLP can be of particular value is in analysing and categorising patient feedback, which can then be used to target quality improvement work.
Box 5: Automated analysis of patient feedback
___________________________________________________________________
Imperial College Healthcare NHS Trust (Innovating for Improvement, 2017–18)
Free-text patient experience feedback within the NHS Friends and Family Test (FFT) offers rich information for quality improvement. But many providers find it difficult to analyse and interpret because of the large volume of unstructured data and the challenge in linking the information to other quality indicators. Beyond missing out on opportunities to improve quality, asking for patient feedback without being able to analyse and act on it also raises ethical questions.
At Imperial College Healthcare NHS Trust (ICHNT), the patient experience team received around 20,000 comments a month and were only able to analyse a small fraction of them. To address this issue, a multidisciplinary team from ICHNT and Imperial College London, supported by the Health Foundation, developed a tool which uses NLP to analyse free text comments in the FFT. The resulting analysis is then used to create easily digestible reports of patient experience at ward or service level, providing front-line staff with the information needed to devise effective quality improvement interventions.
Free-text FFT comments were collected in outpatient, inpatient, maternity and A&E services, with a view to improving care across these four areas. The team validated and then implemented the tool, which can analyse 6,000 comments in 15 minutes, compared with four days if the same analysis was undertaken by a member of staff. The time saved allows the patient experience team to spend more time supporting staff to act on patient feedback and improve services. For example, staff in the outpatient department were quickly able to identify improvements such as better informing patients of their position in the clinic queue and making water fountains available. Elsewhere, improvements were made to discharge processes through developing checklists.
While the project has enjoyed early success, to have greater impact and ensure sustainability for the long term the team are leading a drive to embed the tool into the workflows of all services in the Trust — helping to create a culture of ‘measurement for improvement’. This includes a strong focus on quality improvement methodologies, to ensure the patient feedback leads to positive change.
The team are also working with NHS England and NHS Improvement to spread the tool to other NHS trusts in England. A further Health Foundation grant is supporting the team to test and evaluate the wider application of this NLP platform across NHS trusts, in combination with quality improvement methodology.
‘For successful implementation, use and sustainability, you have to think beyond the shiny tool. It’s also about quality improvement, which takes thought, time and effort to embed.’
Erik Mayer, project lead
___________________________________________________________________
Given AI-driven advances in speech recognition, there is also significant potential for the automation of speech-related administrative tasks.
For example, speech recognition could be used to streamline documentation tasks by using NLP to analyse patient–clinician conversations and create notes, turning a discussion into a text transcript, summarising and annotating it to provide clinically relevant information and categorising it to appropriate sections of the medical record.
After the consultation, the clinician could review a summary for editing and saving (though see Chapter 3 on potential challenges with the automation of note-taking).
An application such as this could also make connections with information already in the patient’s record, or with analysis of similar patients, to support clinical decision making.
For example, Nuance Communications and Microsoft have developed a system that records each patient interaction and uses AI to convert it to text, which is then saved into the electronic health record.
2.3 Application in clinical services
Beyond some of the established uses of automation in health care highlighted in Chapter 1, advances in computing, the codification of clinical knowledge and the availability of large datasets are creating scope for further automation of clinical tasks, or at least components of clinical tasks, especially those that relate to data analysis and decision selection.
There is particular interest in the potential of data analytics and AI to support clinical decision making around diagnosis and treatment, where — as discussed above — the spectrum of automation could range from clinical decision support systems that assist human decision making all the way to scenarios in which decisions could be delegated to machines.
In many cases, these technologies are still in the early stages of development, testing and adoption, and the excitement about their potential currently outstrips the reality on the front line.
Often, more real-world testing is required to investigate how technologies that have shown potential in the lab can be best deployed.
For example, recent systematic reviews of studies comparing the diagnostic accuracy of AI-driven medical image analysis with that of health care professionals found that while many AI models demonstrated comparable accuracy, few studies were prospective or randomised trials in live clinical settings, nor did many present externally validated results.
Nevertheless, there are a growing number of examples of automation and AI that have shown their potential, ranging along the whole patient pathway — from promotion and prevention, through diagnosis and treatment, all the way to rehabilitation and supporting people to live with long-term conditions. We highlight a few examples below.
- Diagnosis
- Risk assessment and prediction
- Triage
- Patient-facing symptom checkers
- Treatment decisions and planning
- Health promotion and self-management
- Quality improvement
Diagnosis: While AI has been applied to analyse a range of diagnostic data, some of the most impressive advances in recent years relate to diagnostic imaging. Machine learning potentially enables a high degree of accuracy in pattern recognition and the classification of images, with the ability to identify abnormalities, in some cases more acutely than the human eye. The application of machine learning in the analysis of medical scans and pathology slides therefore holds significant potential for supporting and improving the detection of diseases. Significant progress has been made in radiology, with AI being used in screening for conditions such as breast cancer. Studies show AI-powered screening systems can be a useful addition to the breast screening pathway; for example, one study by McKinney and colleagues showed that, while only tested using retrospective data, an AI system using deep learning improved specificity and sensitivity in predicting the development of malignancy when compared to a first clinical assessor and performed no worse in comparison to a second clinical assessor, including a reduction in the incidence of false positives and false negatives.
Risk assessment and prediction: AI can also assist clinical decisions by using data to assess risks and make predictions — for example, predicting if a patient’s condition is likely to deteriorate. For example, a project funded by the Health Foundation and led by King’s College London to improve outcomes in stroke care (described in more detail in Box 6) has developed a machine learning model that could be used to predict which patients are at highest risk of mortality after stroke. At a population level, AI could also be used for risk stratification and public health surveillance, such as for disease outbreak prediction and surveillance, something that could be particularly helpful in anticipating future waves of COVID-19 as well as the spread of other diseases.
Box 6: Machine learning analytics for quality improvement in stroke care
___________________________________________________________________
King’s College London (Insight, 2017–2021)
Variation in stroke outcomes is a complex phenomenon, meaning it can be difficult to predict the likelihood of disability after stroke.
While there is an increasingly large and detailed amount of health data available, it can be challenging to translate this into knowledge that can be used to improve care quality.
This project, led by King’s College London, funded as part of the Health Foundation’s Insight programme, sought to address these challenges by using more sophisticated methods to analyse clinical audit data contained in the Sentinel Stroke National Audit Programme (SSNAP).
The project developed machine learning algorithms to predict mortality after stroke, which were trained using SSNAP data from 488,497 patients. The performance of these machine learning methods was then compared to models using traditional statistical methods.
Compared to logistic regression, the machine learning model was slightly more accurate in predicting 30-day mortality after stroke.
The largest accuracy gains were demonstrated when a wider range of potential variables were available to make predictions from, which underlines the importance of high-quality data if the benefits of using more advanced forms of predictive analytics such as machine learning are to be realised in health care.
More accurate predictions of outcomes after stroke could potentially be used to aid patient management, such as identifying early which patients might benefit from more intensive monitoring and management.
They could also be used to analyse how services are performing, to support quality improvement and identify best practice where services are delivering better than expected care. The project team are also now looking at the potential of this technique to predict stroke-associated pneumonia.
‘Health services will need to invest resources in generating better quality data, otherwise the gains from using more advanced methods for predictive analytics will be limited.’
Ben Bray and Wenjuan Wang, project leads
___________________________________________________________________
Triage: There is also increasing interest in the potential of AI to help clinicians make triage decisions and to help patients assess their own symptoms. Several AI triage tools are currently being tested, although these are currently intended to support clinicians in decision making, rather than to make triage decisions themselves. For example, a Health Foundation-funded project led by Barking, Havering and Redbridge University Hospitals NHS Trust is developing a system to assist the emergency department triage process by quickly identifying high-risk patients needing urgent care, described in more detail in Box 13 (page 46).
Patient-facing symptom checkers: A number of companies have also developed patient-facing symptom checkers that are currently available in the UK. These are ‘chatbots’ that use AI to compare data gathered from the patient with medical knowledge, with the aim of helping people get a better understanding of when to seek medical attention and connecting them to the appropriate service. For example, Your.MD and Babylon Health have both developed symptom checkers that ask users a series of questions to build a picture of their symptoms, before suggesting the most appropriate course of action, such as recommending a visit to the GP or hospital or providing reassurance that the person can take steps to recover at home. Babylon claims its chatbot has been trained to recognise the ‘vast majority of health care issues seen in primary care’, although as of May 2021 it also says it is not suitable for people with mental health concerns, skin problems, and pregnancy or post-natal concerns, among others, recommending an appointment with a doctor., University Hospitals Birmingham NHS Foundation Trust is now working with Babylon to use this technology to develop a pre-hospital triage app for people considering using A&E services, as part of a drive to reduce unnecessary A&E attendances.
Treatment decisions and planning: As well as diagnosis and triage, data tools and AI can also be used to support better treatment decisions and planning, by suggesting treatment options or creating prompts and reminders to optimise the delivery of treatment. AI can be coupled with a range of patient data, including genomics data, as well as research and best practice, to help tailor treatments to individual patients. Electronic prescribing systems, for example, can incorporate decision support tools to check dosages and potential interactions with other drugs or conditions, and suggest safer or better-value alternatives. Used in this way, decision support systems — while stopping well short of complete automation — can be a useful tool in promoting adherence to best practice and helping reduce errors and unwarranted variation. In the case of electronic prescribing systems, for example, studies suggest that when used effectively these systems can reduce medication errors and adverse drug events, although there is more work required to ensure they are consistently safe and effective.,
Health promotion and self-management: Automation and AI are also increasingly supporting people to manage their own health. For example, a range of technologies, such as apps, wearables and medical devices, are enabling health monitoring and management in homes and residential care settings. One example is the latest generation of insulin pumps, which combine continuous glucose monitors with smart algorithms that automatically adjust dosage.
Quality improvement: These technologies can also play an important role in supporting quality improvement by helping to generate data and analysis about how services are performing and how they can be improved — critical components of a learning health system. The projects at Imperial College Healthcare NHS Trust and King’s College London described above, which are using machine learning to analyse patient feedback and clinical audit data, demonstrate how AI can be used to help identify where improvements can be made.
In many of the areas highlighted above, robotics can be combined with AI to automate tasks that require movement as well as information processing.
The example of surgery has already been highlighted. Another area where this has potential is medication dispensing and stock management in hospitals and community pharmacies; robotic dispensers can operate with greater speed and precision than humans, with a significantly lower risk of error., A further application of robotics in clinical settings is transportation. For example, Moxi is a robotic assistant with a mobile base, arm and gripper, reportedly being trialled in the US, designed to assist clinical staff by transporting supplies to patient rooms and delivering lab samples. Robotic technologies also present opportunities to provide therapy, whether in the home or in a variety of care settings. For example, Paro is a therapeutic AI robot, designed to look like a seal, to support people with dementia, which has shown signs of being able to reduce agitation and improve verbal and visual engagement among users.
While at present several of the technologies reviewed here currently require human supervision or input, and so are not examples of full automation, they could conceivably become more independent in future.
Either way, used effectively, they have the potential to improve outcomes, experience and efficiency, as well as to help alleviate workforce pressures.
Box 7: NHSX
___________________________________________________________________
NHSX, a joint venture between NHS England and NHS Improvement and the Department of Health and Social Care, provides leadership for digital transformation in health and social care, focused on five missions:
- reducing the burden on clinicians and staff, so they can focus on patients
- giving people the tools to access information and services directly
- ensuring clinical information can be safely accessed, wherever it is needed
- improving patient safety across the NHS
- improving NHS productivity with digital technology.
Through setting policy and targeting investment, NHSX supports the NHS to take advantage of the opportunities presented by technology, including AI and automation.
The NHSX report Artificial Intelligence: How to get it right set out some of the main opportunities where AI could be used to automate or augment medical or care-related tasks traditionally done by health care workers — illustrated below.
These include diagnostics, knowledge generation, public health, system efficiency and ‘P4 medicine’, which is another way of describing precision medicine, emphasising its predictive, preventative, personalised and participatory characteristics.
___________________________________________________________________
Figure 4: Range of potential uses for AI in health and care
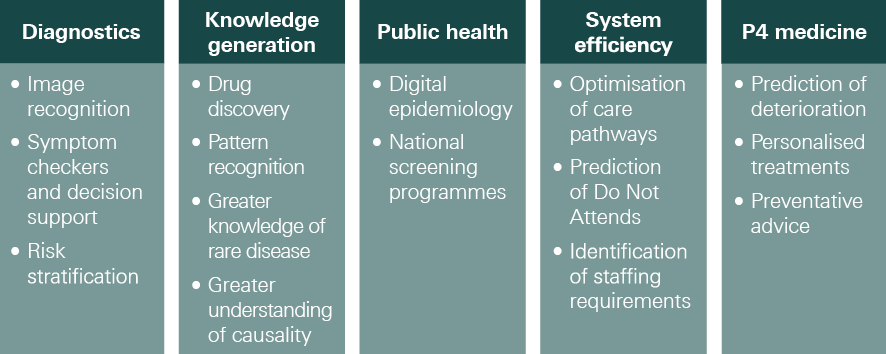
Source: NHSX, Artificial Intelligence: How to get it right, 2019
Box 8: NHS staff views on the biggest opportunities for automation and AI in health care
___________________________________________________________________
As part of our NHS staff survey, we asked which of the many ways in which automation and AI can be applied to health care represented the ‘biggest opportunities’ for improving health care.
Respondents were presented with a list of ten possible uses of these technologies in health care and asked to pick up to three of these.
- The application of automation and AI that clearly ranked highest was ‘analysis of images and test results’, picked by 40% of respondents.
- This was followed by three other applications, all chosen by 28%:
- ‘risk prediction and screening’, ‘use of robots in surgery’ and ‘demand and capacity management, scheduling and rostering’.
- Notably, only 2% of respondents thought ‘robotic carers and assistants’ represented one of the three biggest opportunities.
Figure 5: Views of NHS staff on the biggest opportunities for automation and AI in health care
Which, if any, of the following do you think represent the biggest opportunities for using automation and AI to improve health care?
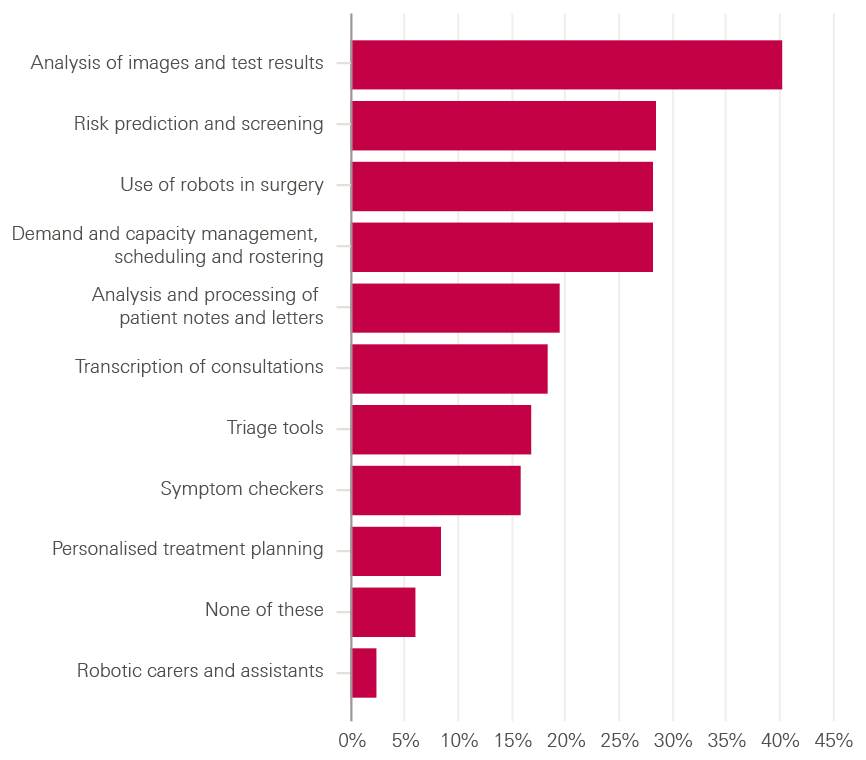
There were a few differences in the pattern of results among different occupational groups, mainly that groups tended to pick options that were particularly relevant to their work.
- For example, ambulance staff gave triage tools a higher rating than average (29% compared to 17% for all respondents)
- while public health professionals gave them a lower rating than average (13%).
- But the ranking of opportunities was broadly similar across occupational groups.
The list of opportunities provided was certainly not comprehensive, and it is worth noting in this respect that 6% of respondents thought that none of these listed opportunities were in their top three biggest opportunities (represented by the bar labelled ‘none of these’ in the figure above).
___________________________________________________________________