New research identifies four ways bias can arise in AI, and how developers and regulators can mitigate it
Site editor:
Joaquim Cardoso MSc.
The Health Advisory — for continuous health transformation
September 22, 2022
Pew Trusts
Projects:Health Care Products
August 24, 2022
Synthesis:
Medical tools enabled with artificial intelligence (AI) can greatly improve health care by helping with problem-solving, automating tasks, and identifying patterns.
- AI can aid in diagnoses, predict patients’ risk of developing certain illnesses, and help determine the communities most in need of preventative care.
- But AI products can also replicate — and worse, exacerbate — existing biases and disparities in patients’ care and health outcomes. Alleviating these biases requires first understanding where they come from.
The authors identified four areas in which bias can be introduced:
- 1.Inequitable framing of the health care challenge or the user’s next steps
- 2.The use of unrepresentative training data
- 3.The use of biased training data
- 4.Insufficient care with choices in data selection, curation, preparation, and model development
Given these sources of biases and inequities, what can be done if a tool does show biased performance after development?
- The best option is to determine the cause of the bias and to go back to development and retrain the model.
- A second option is to make clear (within the product use instructions and during marketing and training) that the AI tool is only intended for use in certain populations.
Looking Forward
- While there is much work to be done to ensure AI tools in the health care setting don’t ingrain or exacerbate existing biases, we should also acknowledge that AI has the potential to help solve longstanding issues. For example, where there is detectable bias in patient records, AI might be able to flag that bias and ensure institutions are living up to their stated values.45
- There are also ample examples of the potential of AI to address other issues such as biased pain measurement and gaps in image datasets used to teach clinicians on how to identify dermatological conditions.
- It is important to recognize that AI is a tool developed by humans and can be fallible like any other. However, when used effectively for the intended purpose and with proper oversight, AI tools can provide important value.
- This paper focused mostly on how AI is used in health care settings under FDA authority.
- However, it is also worth considering the role of AI in other similar settings and purposes, such as promoting population health or identifying social determinants of health and how to best meet the needs of patients that have historically had and continue to have adverse interactions with the health care system.
Christina Silcox, Ph.D., research director for digital health at the Duke-Margolis Center for Health Policy, and her team identified four sources of AI bias and ways to mitigate them in a recent report funded by The Pew Charitable Trusts. She spoke with Pew about the findings.
This interview with Silcox has been edited for clarity and length.
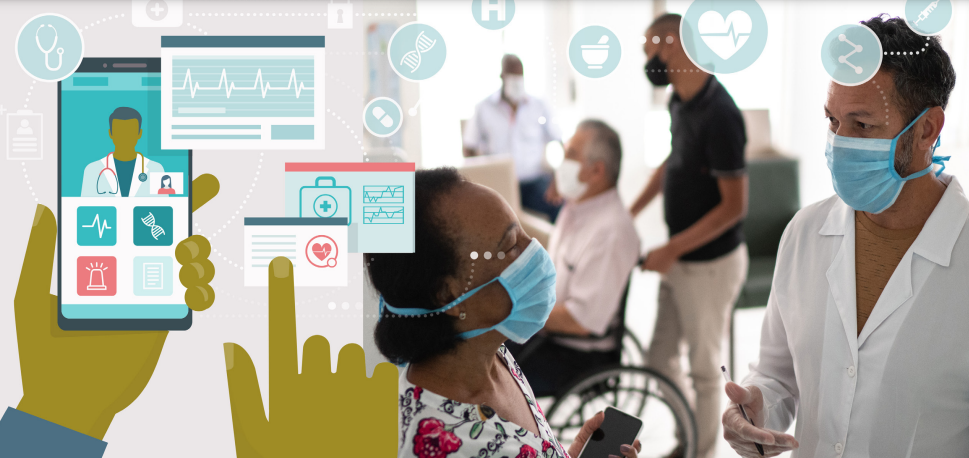
How does bias in AI affect health care?
AI tools do not have to be biased.
But if we’re not paying careful attention, we teach these tools to reflect existing biases in humans and health care, which leads to continued or worsened health inequities.
It’s particularly concerning if this happens in groups that have historically gotten poor care, such as those who have been affected by structural racism and institutionalized disparities like lack of access to affordable health insurance and care.
For example, when machine-learning-based tools are trained on real-world health data that reflects inequitable care, we run the risk of perpetuating and even scaling up those inequities.
Those tools may end up leading to slower diagnoses or incomplete treatments, decreased access, or reduced overall quality of care — and that’s the last thing we want to do.
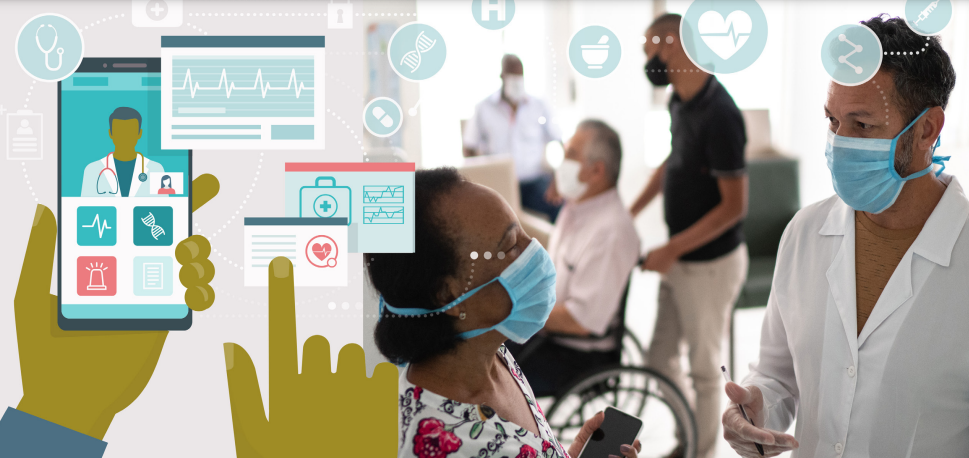
Your research identified four ways bias can arise in AI. One is inequitable framing — what is that and how does that lead to bias?
Inequitable framing means that a tool is programmed to solve for the wrong question and, as a result, ends up addressing the wrong issue.
An example is a “no-show” algorithm, which can predict which patients may not keep their appointments.
Based on those predictions, many clinics and hospitals double-book certain patients to try to minimize lost revenue.
The problem with that is Black, Latino, and American Indian or Alaskan Native patients disproportionately experience systemic barriers to care, such as lack of reliable transportation and limited access to paid sick leave or affordable health insurance, that may prevent them from getting to the doctor’s office.
And double-booking those appointments only exacerbates those problems, because when both patients do show up, they are either not seen promptly or are rushed through their appointments.
Asking an AI tool “who won’t show up?” and double-booking only solves a financial problem.
Asking an AI tool “who won’t show up?” and double-booking only solves a financial problem.
A better approach is to design tools that can predict “which supportive measure is most likely to help this patient attend their appointment?
A better approach is to design tools that can predict “which supportive measure is most likely to help this patient attend their appointment?
- Who needs transportation?
- Who would benefit from a reminder call?
- Who should be booked for a video consult rather than in person?”
That framing solves both the financial problem and the health problem.
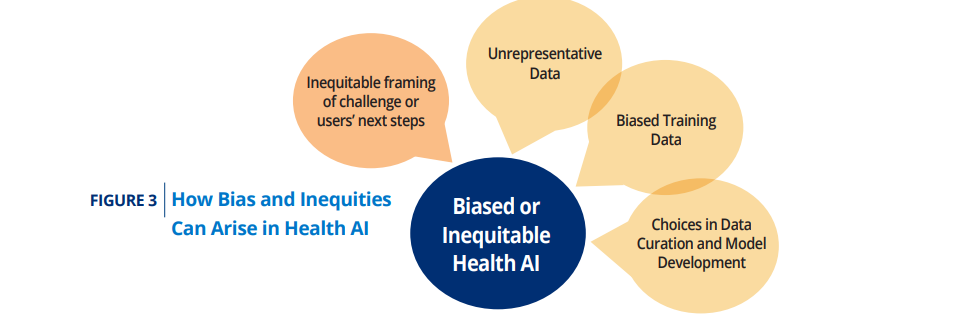
Other areas of bias you identified are unrepresentative training data and biased training data. What’s the difference between them and how do they lead to bias?
Unrepresentative data means that the data collected is inconsistent with the locations and populations on which the tool will be used.
For example, training a tool with data from Northeastern urban academic medical centers or large research institutes and then bringing it to a Southwestern rural community clinic and using it on the population there.
Workflows, access to certain tests and treatments, data collection, patient demographics, and the likelihood of certain diseases all vary between different places and hospital types, so a tool trained on one set of patient data may not perform well when the data or patient populations change significantly.
Workflows, access to certain tests and treatments, data collection, patient demographics, and the likelihood of certain diseases all vary between different places and hospital types, so a tool trained on one set of patient data may not perform well when the data or patient populations change significantly.
But even if you have data that is representative, there may be bias within the data.
We’ve seen this with AI tools that used data from pulse oximeters, the finger clip-like devices that measure the oxygen saturation level of your blood.
These tools were used to help guide triage and therapy decisions for COVID-19 patients during the pandemic.
But it turns out the pulse oximetry sensors don’t work as well for darker-skinned people, people who have smaller fingers, or people who have thicker skin.
That data is less accurate for certain groups of people than it is for others.
And it’s fed into an algorithm that potentially leads doctors to make inadequate treatment decisions for those patients.
We’ve seen this with AI tools that used data from pulse oximeters, the finger clip-like devices that measure the oxygen saturation level of your blood.
But it turns out the pulse oximetry sensors don’t work as well for darker-skinned people, people who have smaller fingers, or people who have thicker skin.
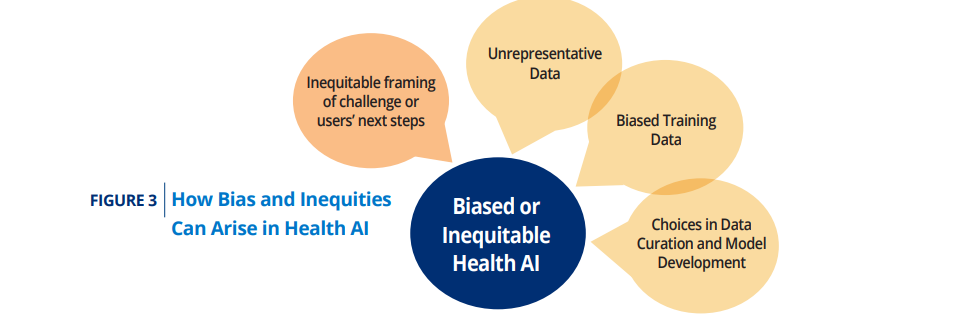
The final area of bias you identified was data selection and curation. How does that affect AI?
AI developers have to select the data used to train their programs, and their choices can have serious consequences.
For example, a few years ago, health systems used a tool to predict which patients were at risk of severe illness so they could better allocate services meant to prevent those illnesses.
Researchers found that when developing the tool, the AI developers had chosen to use data on patients’ health care costs as a proxy for the severity of their illnesses.
The problem with that is, Black patients historically have less access to health care — and therefore lower costs — even when they’re very ill.
Costs are a racially biased proxy for severity of illness. So the tool underestimated the likelihood of serious health conditions for Black patients.
For example, a few years ago, health systems used a tool to predict which patients were at risk of severe illness so they could better allocate services meant to prevent those illnesses.
… the AI developers had chosen to use data on patients’ health care costs as a proxy for the severity of their illnesses. The problem with that is, Black patients historically have less access to health care — and therefore lower costs — even when they’re very ill.
Decisions in data curation can also cause bias.
Health care data is complicated and messy.
There’s an urge to clean it up and use only the most complete data, or data that has little to no missing information.
But people who tend to have missing information in their medical records are those who have less access to care, those who have lower socioeconomic statuses, and those less likely to have insurance.
Removing these individuals from the training data set may reduce the performance of the tool for these populations.
Health care data is complicated and messy. There’s an urge to clean it up and use only the most complete data, or data that has little to no missing information.
But people who tend to have missing information in their medical records are those who have less access to care, those who have lower socioeconomic statuses, and those less likely to have insurance.
Removing these individuals from the training data set may reduce the performance of the tool for these populations.
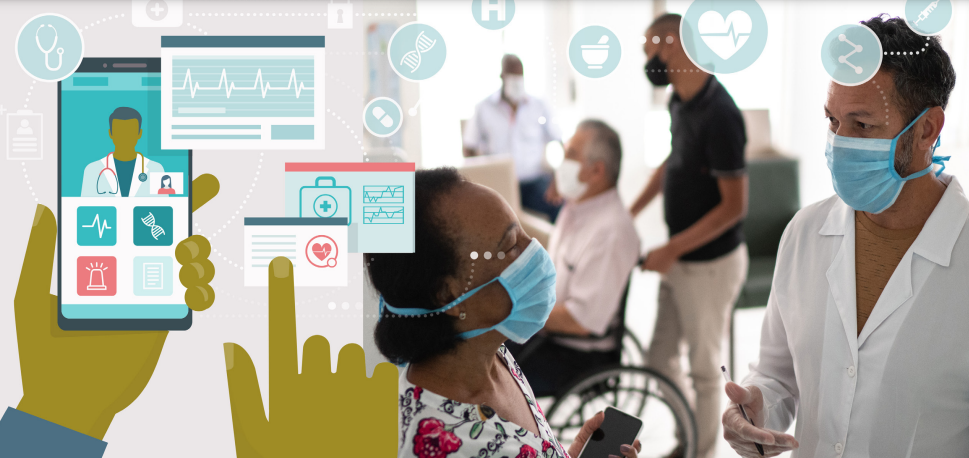
What can be done to help mitigate these biases?
AI developers have the responsibility to create teams with diverse expertise and with a deep understanding of the problem being solved, the data being used, and the differences that can occur across various subgroups.
Purchasers of these tools also have an enormous responsibility to test them within their own subpopulations and to demand developers use emerging good machine learning practices — standards and practices that help promote safety and effectiveness — in the creation of those products.
FDA has the authority to regulate some AI products, specifically software that is considered a medical device.
The agency has a responsibility to ensure those devices perform well across subgroups, should require clear and accessible labeling of the products indicating the populations they’re intended for, and work to build systems that can monitor for biased performance of medical products.
Finally, people who originate data … have a responsibility to make sure that data is as unbiased and equitable as possible,
Finally, people who originate data — people who put data into electronic health records, people who put data into claims, people who build wellness algorithms and other tools that collect consumer data — have a responsibility to make sure that data is as unbiased and equitable as possible, particularly if they plan to share it for AI tool development or for other purposes.
That means increasing standardization and common definitions in health data, reducing bias in subjective medical descriptions, and annotating where data may differ across populations.
… people who originate data … have a responsibility to make sure that data is as unbiased and equitable as possible,
That means increasing standardization and common definitions in health data, reducing bias in subjective medical descriptions, and annotating where data may differ across populations.
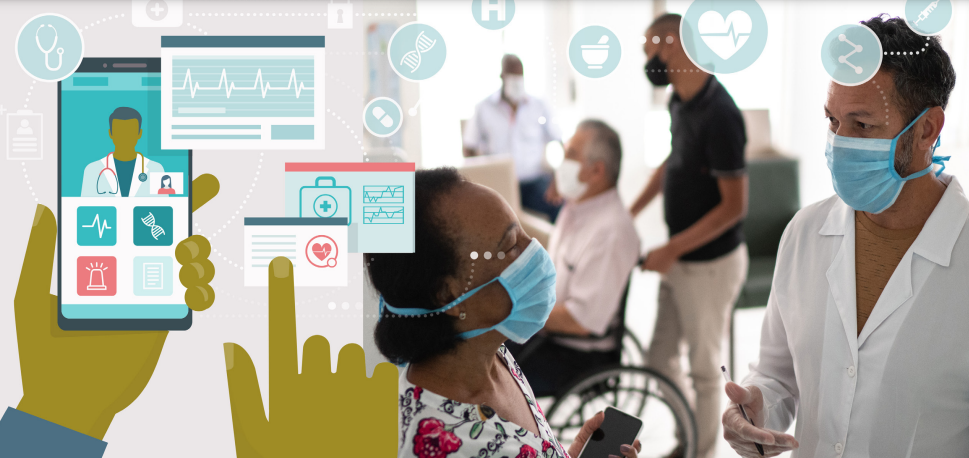
What can be done if a tool shows biased performance after development?
The best option here is to determine the cause of the bias, go back to development, and retrain the model.
The other option is to clarify within the product’s use instructions and in its marketing and training materials that the tool is only intended for use in certain populations.
Through these remedies, we can ensure that AI tools aren’t replicating or worsening the current state of health care, but rather helping us create a more just and equitable system.
What can be done if a tool shows biased performance after development? (1) The best option here is to determine the cause of the bias, go back to development, and retrain the model. (2) The other option is to clarify within the product’s use instructions and in its marketing and training materials that the tool is only intended for use in certain populations.
Names mentioned:
Christina Silcox, Ph.D., research director for digital health at the Duke-Margolis Center for Health Policy,
REFERENCE PUBLICATION (excerpts)
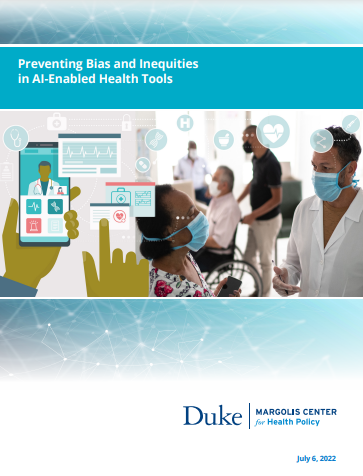
Preventing Bias and Inequities in AI-Enabled Health Tools
Duke Margolis Center for Health Policy
Trevan Locke,
Assistant Research Director, Duke-Margolis Center for Health Policy
Valerie J. Parker,
Senior Policy Analyst, Duke-Margolis Center for Health Policy
Andrea Thoumi,
Health Equity Policy Fellow, Duke-Margolis Center for Health Policy
Benjamin A. Goldstein,
Associate Professor of Biostatistics and Bioinformatics, Duke University
Christina Silcox,
Research Director, Digital Health, Duke-Margolis Center for Health Policy
July 6, 2022
Executive Summary
Artificial Intelligence (AI) has shown great potential across a variety of areas in our society, including within the health care system. AI is used to analyze images in radiology, assess patients to provide decision support to providers, and flag patients at a high risk of deterioration. While AI can be a useful tool, it is built by humans and with data collected by humans. As such, it is susceptible to reproducing and potentially scaling the effects of the biases and inequities which pervade our society. As such, it is unsurprising that there are many documented cases in which health AI has shown disparate performance amongst different patient subgroups and has otherwise been shown to worsen health inequities. But if carefully built and tested, AI has the potential to reduce biased care and improve health equity, for example through increased access, nudging health care professionals past subconscious bias, and more personalized care. This paper explores how bias enters into an AI-enabled health tool throughout various stages of the development and implementation process, identifies mitigation and testing practices that can reduce the likelihood of building a tool that is biased or inequitable, and describes gaps where more research is needed.
Building from insights from numerous stakeholder interviews conducted in Fall 2021 through Spring 2022, a public convening held during December 2021, and a literature review.
We identified four areas in which bias can be introduced:
- 1.Inequitable framing of the health care challenge or the user’s next steps
- 2.The use of unrepresentative training data
- 3.The use of biased training data
- 4.Insufficient care with choices in data selection, curation, preparation, and model development
From there, the paper provides recommendations for identifying and mitigating biased AI in health care focused on different stakeholder groups: developers, purchasers of the AI tools such as providers or payers, data originators such as health systems, and regulators, with a particular focus on the U.S. Food and Drug Administration (FDA).
Developers should be aware of how bias or inequitable outcomes can be caused by the development process. They need to follow consensus standards (where they exist) and help develop good machine learning practices (GMLP) to prevent tools from biased performance or contributing to inequitable outcomes. They need to work with teams with diverse expertise, including a deep understanding of the problem being solved, the data being used, the differences that can occur across subgroups within the population of interest, and how the AI tool output is likely to be used.
Purchasers and users need to test tools within their own subpopulations, both during implementation, but also over time to monitor any drift towards bias or inequity. This includes not just the accuracy of the tool itself, but also patient outcomes resulting from use of the tool.
Data originators have a responsibility to ensure that their data is recorded in just and equitable ways. Data originators include multiple groups: real-world data generators like health systems, payers, and tech companies making wearables and remote monitoring home devices, as well as private and public consortiums building health databases for health research and AI development purposes. All data originators should prioritize standardization, reductions of bias in subjective descriptions, and annotation of where their data may differ across populations. These differences can be due to access challenges, differing performance of data collection tools such as sensors, or other reasons. This is a responsibility that is not simply about building better AI but also ensuring the highest quality care decisions and improving the overall learning health care system.
The FDA, working in tandem with other federal agencies, should ensure that AI-enabled medical devices perform well across subgroups. They should also require clear and accessible labeling of the products regarding subgroup testing and populations intended for use and work to build systems to monitor for biased performance of AI-enabled devices.
As a developing field, some of the best practices, data, and tests needed to facilitate implementation of these recommendations are not yet built or are still in development. The health care ecosystem as a whole needs to work together to ensure that AI tools are purposefully built to create a more just and equitable health care system, rather than replicating or worsening the current state.
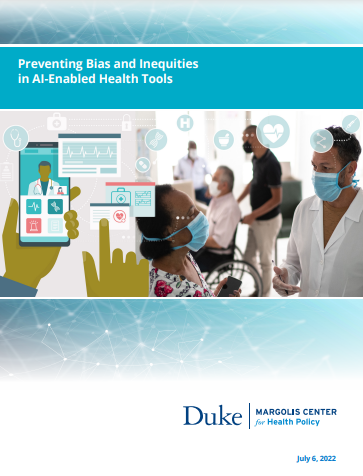
Introduction
Artificial Intelligence (AI) has shown considerable promise for improving health and medical treatments, but it can reflect and scale biases and inequities already prevalent throughout our society. This is particularly a concern for machine-learning (Figure 1) trained on real-world health data that reflects the biased and inequitable care given to certain subgroups within patient populations due to factors attributable to structural racism and institutionalized inequities such as lack of access to affordable health insurance and care, as well as prejudice and implicit biases.5–9
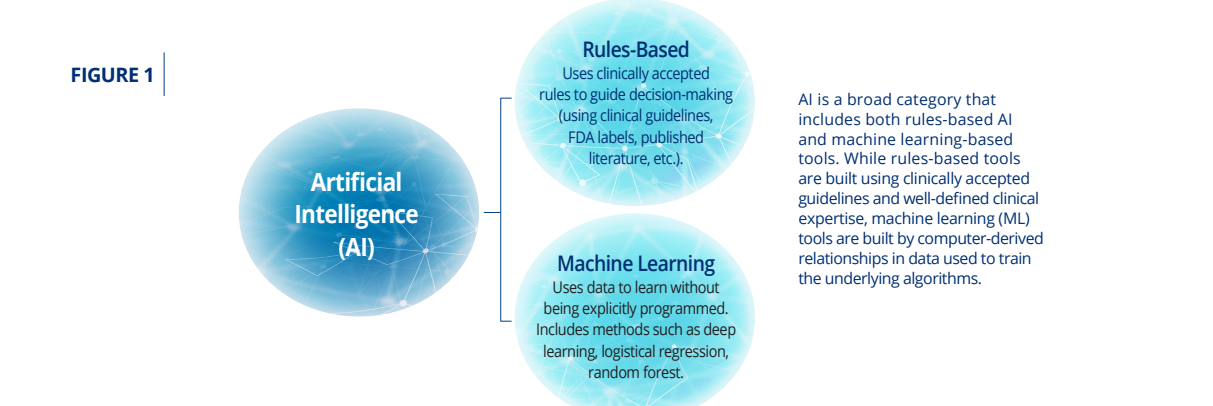
But rules-based algorithms have also been shown to have biased performance and outcomes.10 As the health care field works to leverage innovative technology into new approaches to delivering care, all stakeholders must commit to ensuring that current and past inequities and biases do not become more ingrained. These stakeholders include developers of AI health tools, but also purchasers, regulators, and other contributors to the development of AI health tools including health systems, payers, companies that collect health and wellness data such as wearables and health monitors, and regulators (Table 1).
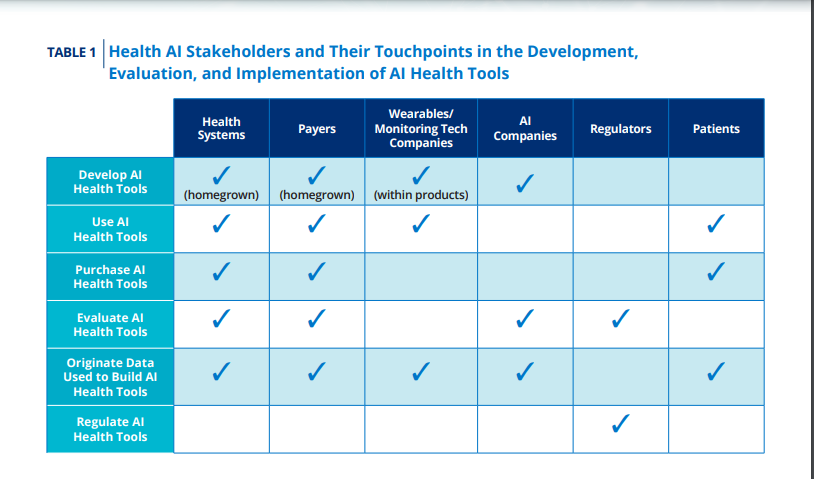
These stakeholders often have multiple roles regarding data origination, development, evaluation, and use of these AI tools. Patients that are impacted by these tools are also critical stakeholders that the rest of the community must work with to help ensure equitable and just health care.
This responsibility of ensuring that AI tools lead to more equitable health care is critical as more health tools are being built and implemented. According to the consulting firm Advisory Board, use of AI by provider organizations has increased in recent years with 18 percent for precision medicine, 16 percent of organizations using AI for protocol compliance, and 14 percent for risk and care gap identification.11 Provider systems, especially larger or academic-based health systems, may be using a combination of commercial products and “homegrown” tools developed by their own staff and researchers. AI is also being used in public health, in payer systems, and in the development and surveillance of other medical products; for example, to identify drug targets and for clinical trial data collection and management.
Figure 2, adapted from a United States Agency for International Development report, shows the diversity of AI uses within the health space.12
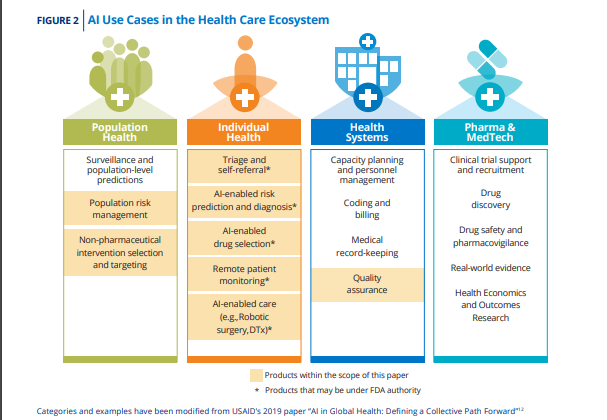
AI applications in the health care setting include clinical decision support (CDS), wearable remote monitoring and analyses, digital therapeutics, administrative software (such as scheduling software that can predict “no-shows”, software used to determine home nursing aid hours, and supply chain management), and population health management. Some of these tools and products are classified as medical devices, under U.S. Food and Drug Administration (FDA) authority, while others are used for administrative, wellness, or business purposes and are outside of FDA review. These AI-enabled tools have the potential to significantly improve outcomes as well as increase access and efficiency in health care by improving decision-making, increasing access, reducing costs, or hastening diagnosis and treatment. But, if developed without care to the prevention of bias, inequity, and injustice, they also can scale inequities and further entrench health care disparities.
In a recent blog post, the Office of the National Coordinator for Health Information Technology (ONC) defined an unbiased and equitable AI tool as one that “does not exhibit prejudice or favoritism toward an individual or group based on their inherent or acquired characteristics. The impact of using the [tool] is similar across same or different populations or groups.”13 We believe this definition includes protected classes around age, race, and gender, but also includes other subgroups where performance may differ due to geography, insurance status, or comorbidities. At the same time, developers, evaluators, purchasers, and, ultimately, users should be particularly focused on subgroups which have been subject to discrimination, as bias in AI tools could then systematically perpetuate these inequities and lead to unjust outcomes.
There is nuance in this topic. An AI tool can be biased in ways that are not necessarily inequitable as long as performance remains clinically acceptable for the full population of interest. For example, a mortality prediction tool may have much higher accuracy in individuals with conditions that have clearly defined disease courses, but still work acceptably well in the rest of the indicated population. On the other hand, AI tools can also be accurate across subpopulations, but the typical use of tool leads to inequitable outcomes because the problem has not been well defined or the actions taken with respect to the prediction cause injustice. It can also be difficult to measure if a tool has biased performance or causes inequities if the true patient outcome of interest is not standardly defined, not typically collected in health care systems, or takes an extended time to occur.
It is critically important for processes and tests to be put in place throughout the development cycle to allow developers and manufacturers to mitigate potential issues before they become a problem or, alternatively, “fail fast” and go back to drawing board. This includes involving other stakeholders and experts on the development team, including people knowledgeable about the data being used to train AI/machine learning (ML) tools, potential users, and patients. Additionally, it is important to test for bias after the tool is developed, both before deployment but also in regular intervals after deployment. Biased performance could result later in time from updates to the AI tool, but also changes in the data used by the tool to compute the results.14
Bias in AI tools is not an issue specific to health care and has been seen in applications from facial recognition to finance to criminal sentencing.15 However, there are unique challenges to addressing bias in health care settings. Health data is often complicated by a lack of common definitions and standardization, resulting in challenges with making a cohesive, interoperable data system. Because of historical and ongoing systemic racism and discrimination in health care and the subjective nature of much of health data, AI can be biased in many ways. While other sectors can fully leverage the “move fast and break things” mantra of Silicon Valley, doing so in the health care setting could have significant consequences for life and wellbeing. With the rise of utilization of AI tools in health care settings, it is crucial that there are processes in place to remove or minimize bias in existing and newly built systems, rigorous tests to detect bias when it does arise, and a shared commitment from all stakeholders, including developers, purchasers, data originators, and regulators to ensuring justice and equity in health care.
Bias in health care also long predates the advent of AI. For example, systemic racism and discrimination have resulted in racial and gender biases in how providers rate and interpret patients’ pain in real-world settings.16 Only in recent years have strides been made to ensure clinical trials are representative of real-world populations, but more work remains. There are also wide geographic gaps in access to tests, procedures, specialists, and treatments both within and across countries.17 With these historical and contemporary experiences in mind, it is clear that we need to examine how bias within AI can perpetuate human biases that already exist in health care, what steps need to be taken to ensure health care uses of AI do not ingrain the same biases in a newer system, and how AI might be leveraged to mitigate existing biases.
With these challenges in mind, this white paper explains how bias and inequities can be introduced and incorporated into AI-enabled health tools during the origination and development process, along with strategies for testing and detecting bias to inform regulators and other evaluators of AI, policymakers, and those responsible for creating, purchasing, implementing, or maintaining AI tools.
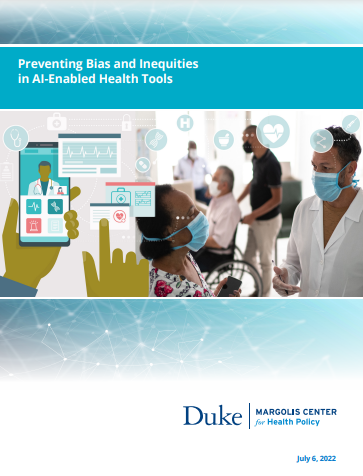
Intermediary chapters
See the original publication
Addressing Biased or Inequitable Performance in AI Tools After Development
Given these sources of biases and inequities, what can be done if a tool does show biased performance after development?
- The best option is to determine the cause of the bias and to go back to development and retrain the model.
- A second option is to make clear (within the product use instructions and during marketing and training) that the AI tool is only intended for use in certain populations.
In the context of FDA regulated devices, the device label would need to make this clear.
However, device labels may not be enough if users are unlikely to see or retain the information in the label. FDA would also need to consider if other steps were necessary to ensure the user knows when they may be using a device off-label. For example, a user may have to enter certain patient data that matches the population of use before the device will report results.
But there are also important ethical and legal considerations to taking this label-based approach, more so if the lower performance is seen in subgroups that are considered protected classes.
For instance, if an AI tool only accurately predicts the risk of heart attacks in non-Hispanic white men, is it equitable to allow marketing of that product if its use is restricted to only that population? Does that risk systematically perpetuate inequities for marginalized populations for which this tool is inaccessible? On the other hand, what if the tool is purposefully optimized to better diagnose conditions in historically marginalized or medically underserved populations, and therefore does not perform as well on populations that historically and currently have had better outcomes? This type of bias, as long as the user was properly trained on who to use it for, may be acceptable from a health equity standpoint, although there could be legal challenges if the subgroups involved protected classes. The Federal Trade Commission (FTC) has made clear that the “FTC Act prohibits unfair or deceptive practices. That would include the sale or use of — for example — racially biased algorithms.”56 However, it is unclear if that prohibition would also be true for nonprotected subpopulations that are based on geography or socioeconomic status. So, while proper documentation and labeling may help prevent biased outcomes from AI tools trained on limited data, additional health equity and health justice issues will arise if only some parts of the population have access to AI tools. Developers considering this approach would also need to be aware of the potential for reputational damage, even if the approach is legal. The complicated reception of BiDil, approved in 2005 for the treatment of heart failure in “self-identified” African Americans, may offer some insights into potential physician, patient, and media reactions.57,58
Regulators and policymakers will need to examine the potential for disparate outcomes when AI tools are not trained to work with the entire patient population.
There is an argument that we should allow tools, even if they don’t work on the entire population, that would have a significant positive impact as long as the use doesn’t change the risks for others. One could even argue that certain triage or screening tools used on a portion of the population could allow physicians to spend more time on complicated cases where the AI tool may not work as well, improving overall outcomes. But there are other considerations, such as whether provider skills are likely to degrade as they start to depend on the AI tool over time, leading to poorer patient outcomes when the tool can’t be used. For example, if an AI tool assists in proper imaging positioning from technicians for most of their patients, will they know how to correctly position patients for whom they don’t have AI assistance? Or will those patients suffer from having lower-quality images throughout the rest of their care journey? Longterm outcome-based research would be needed to fully understand the implications.
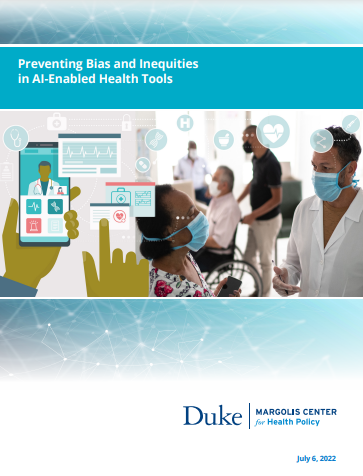
Looking Forward
While there is much work to be done to ensure AI tools in the health care setting don’t ingrain or exacerbate existing biases, we should also acknowledge that AI has the potential to help solve longstanding issues.
For example, where there is detectable bias in patient records, AI might be able to flag that bias and ensure institutions are living up to their stated values.45
There are also ample examples of the potential of AI to address other issues such as biased pain measurement and gaps in image datasets used to teach clinicians on how to identify dermatological conditions.
It is important to recognize that AI is a tool developed by humans and can be fallible like any other.
However, when used effectively for the intended purpose and with proper oversight, AI tools can provide important value.
This paper focused mostly on how AI is used in health care settings under FDA authority.
However, it is also worth considering the role of AI in other similar settings and purposes, such as promoting population health or identifying social determinants of health and how to best meet the needs of patients that have historically had and continue to have adverse interactions with the health care system.
We hope that the information and recommendations provided here will be useful for stakeholders across the health care AI space as they look for strategies and opportunities to mitigate or eliminate bias in health AI to ensure equitable access to quality care for all patients.
Infographic
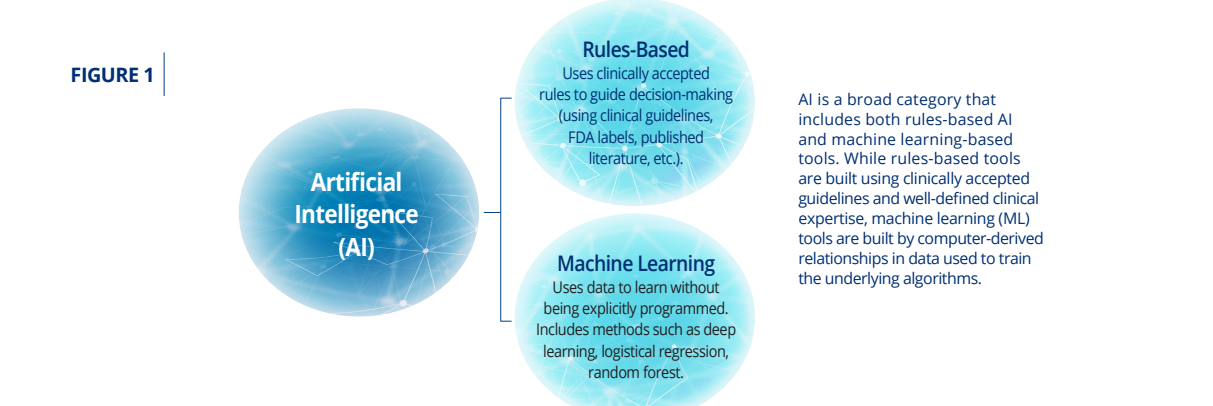
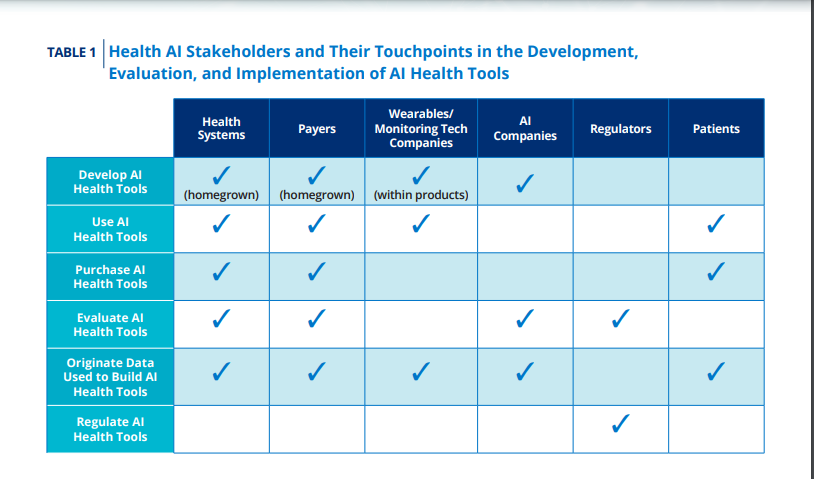
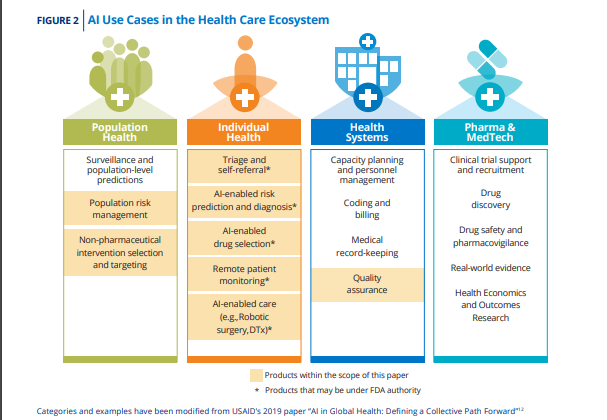
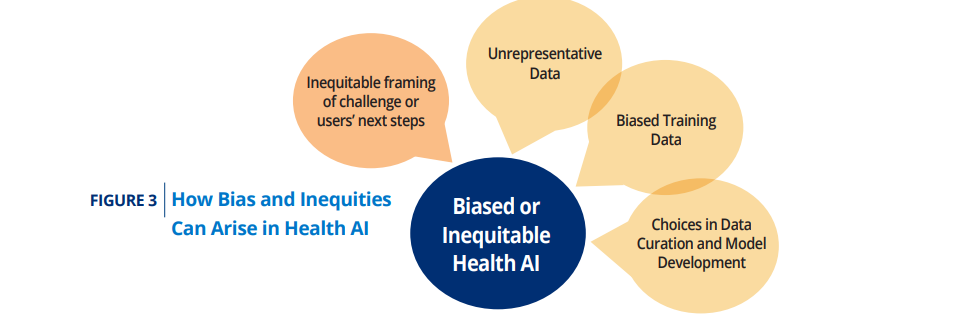
References:
See the original publication