Harvard Business School
Thomas H. Davenport and DJ Patil
July 15, 2022, HBR Staff/StudioM1/Moritz Otto/Getty Images
Executive Summary by:
Joaquim Cardoso MSc.
Transforming Health — Research and Advisory Institute
Data Driven Health Care Unit
July 18, 2022
Introduction:
- Ten years ago we published the article “ Data Scientist: Sexiest Job of the 21stCentury.”
- At the time, we defined the data scientist as “a high-ranking professional with the training and curiosity to make discoveries in the world of big data.”
- A decade later, the job is more in demand than ever with employers and recruiters.
- Many of the same headaches remain, too. Many organizations don’t have data-driven cultures and don’t take advantage of the insights provided by data scientists.
Even so, the job has changed — in both large and small ways.
- It’s become better institutionalized,
- its scope has been redefined,
- the technology it relies on has made huge strides, and
- the importance of non-technical expertise, such as ethics and change management, has grown.
However, it will also continue to change.
- We expect to see continued differentiation of responsibilities and roles that all once fell under the data scientist category.
- Companies will need detailed skill classification and certification processes for these diverse jobs, and must ensure that all of the needed roles are present on large-scale data science projects.
- Professional data scientists themselves will focus on algorithmic innovation, but will also need to be responsible for ensuring that amateurs don’t get in over their heads.
- Most importantly, data scientists must contribute towards appropriate collection of data, responsible analysis, fully-deployed models, and successful business outcomes.
ORIGINAL PUBLICATION (full version)
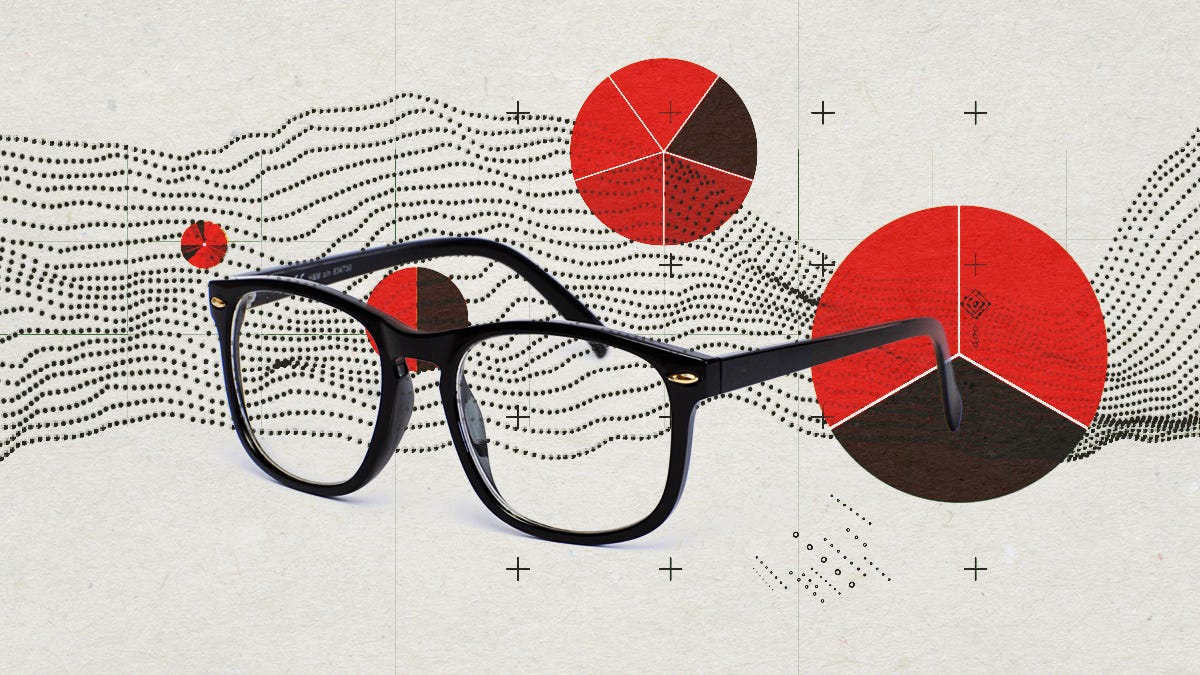
Is Data Scientist Still the Sexiest Job of the 21st Century?
Harvard Business School
Thomas H. Davenport and DJ Patil
July 15, 2022, HBR Staff/StudioM1/Moritz Otto/Getty Images
Ten years ago we published the article “ Data Scientist: Sexiest Job of the 21stCentury.”
Most casual readers probably remember only the “sexiest” modifier — a comment on their demand in the marketplace.
The role was relatively new at the time, but as more companies attempted to make sense of big data, they realized they needed people who could combine programming, analytics, and experimentation skills.
At the time, that demand was largely restricted to the San Francisco Bay Area and a few other coastal cities.
Startups and tech firms in those areas seemed to want all the data scientists they could hire.
We felt that the need would expand as mainstream companies embraced both business analytics and new forms and volumes of data.
At the time, we defined the data scientist as “a high-ranking professional with the training and curiosity to make discoveries in the world of big data.”
Companies were beginning to analyze voluminous and less-structured data like online clickstreams, social media, and images and speech.
Because there wasn’t yet a well-defined career path for people who could program with and analyze such data, data scientists had diverse educational backgrounds.
The most common qualification in our informal survey of 35 data scientists at the time was a PhD in experimental physics, but we also found astronomers, psychologists, and meteorologists.
Most had PhDs in some scientific field, were exceptional at math, and knew how to code.
Given the absence of tools and processes at the time to perform their roles, they were also good at experimentation and invention.
It’s not that a science PhD was really required to do the work, but rather that these individuals had the rare ability to unlock the potential of data, wading through complex, messy data sets and building recommendation algorithms.
A decade later, the job is more in demand than ever with employers and recruiters.
AI is increasingly popular in business, and companies of all sizes and locations feel they need data scientists to develop AI models.
By 2019, postings for data scientists on Indeed had risen by 256%, and the U.S. Bureau of Labor Statistics, predicts data science will see more growth than almost any other field between now and 2029.
The sought-after job is generally paid quite well; the median salary for an experienced data scientist in California is approaching $200,000.
Many of the same headaches remain, too.
In our research for the original article, many data scientists noted that they spend much of their time cleaning and wrangling data, and that is still the case despite a few advances in using AI itself for data management improvements.
In addition, many organizations don’t have data-driven cultures and don’t take advantage of the insights provided by data scientists.
Being hired and paid well doesn’t mean that data scientists will be able to make a difference for their employers. As a result, many are frustrated, leading to high turnover.
… many organizations don’t have data-driven cultures and don’t take advantage of the insights provided by data scientists.
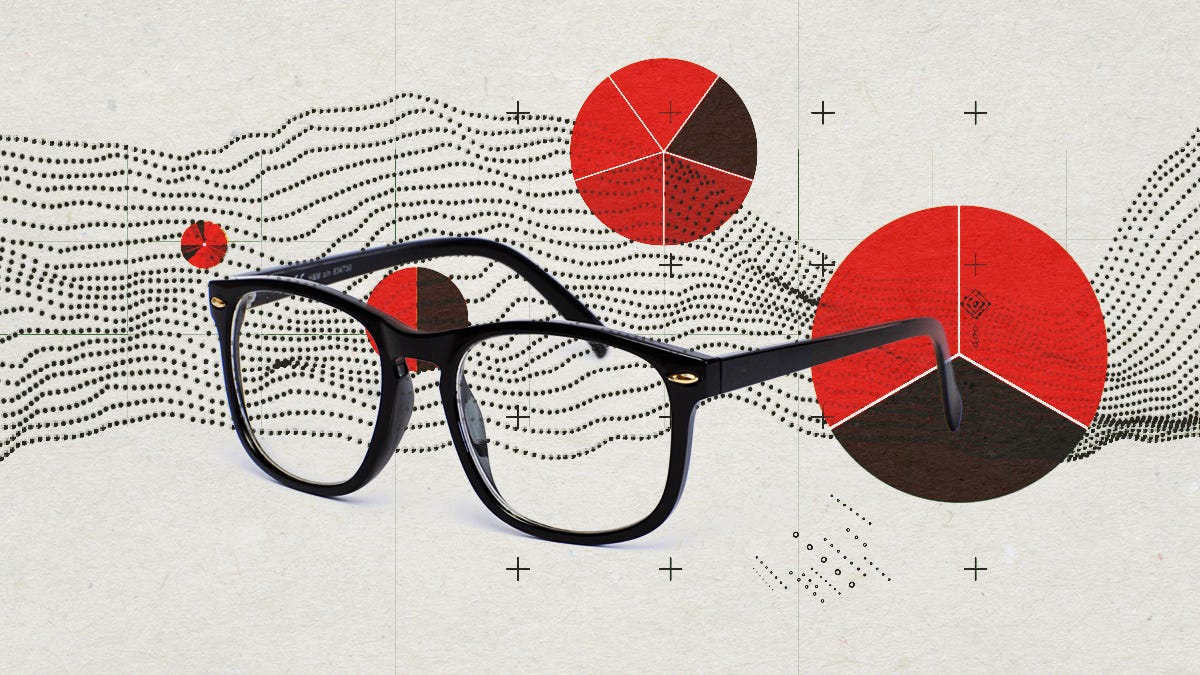
Even so, the job has changed — in both large and small ways.
It’s become better institutionalized, its scope has been redefined, the technology it relies on has made huge strides, and the importance of non-technical expertise, such as ethics and change management, has grown.
The many executives who recognize that data science is important to their businesses now need to create and oversee diverse data science teams rather than searching for data scientist unicorns. They can also begin to think about democratizing data science — still with the aid of data scientists, however.
Even so, the job has changed — in both large and small ways: (1) It’s become better institutionalized, (2) its scope has been redefined, (3) the technology it relies on has made huge strides, and the (4) importance of non-technical expertise, such as ethics and change management, has grown.
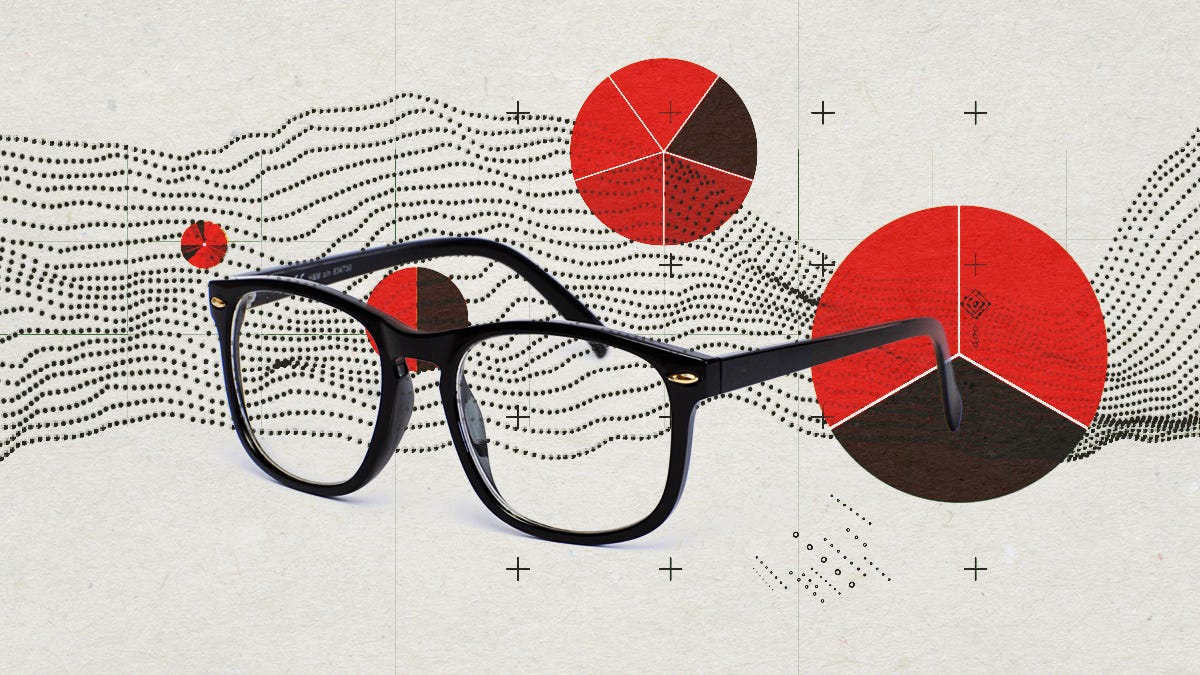
1.Better Institutionalized
In 2012, data science was a nascent function even in AI-oriented startups.
Today it is quite well-established, at least in firms with a major commitment to data and AI.
Banks, insurance companies, retailers, and even health care providers, and even government agencies have substantial data science groups; large financial services firms may have hundreds of data scientists.
Data science has also been effective in addressing societal crises, counting and predicting Covid-19 cases and deaths, helping to address weather disasters, and even fighting misinformation and cyber hacks related to the Ukraine invasion.
One important factor facilitating institutionalization has been the rise of data science-oriented educational offerings.
In 2012, there were effectively no degree programs in data science; data scientists were recruited from other quantitatively-oriented fields.
Now there are hundreds of degree programs in data science or the related fields of analytics and AI.
Most are masters degree programs, but there are also undergraduate majors and PhD programs in data science.
There are also enormous numbers of certificates, online course offerings, and boot camps in data science-related fields.
There are even high school data science courses and curricula.
It’s clear that anyone desiring to be trained in data science capabilities will have plenty of options for doing so.
However, it’s unlikely that any single program can inculcate all of the skills necessary to conceive, build, and deploy effective and ethical data science analysis, experiments, and models.
Indeed, making sense of the diverse educational choices even at a single institution is a challenge for prospective data scientists and for the companies that wish to employ them.
… making sense of the diverse educational choices even at a single institution is a challenge for prospective data scientists and for the companies that wish to employ them.
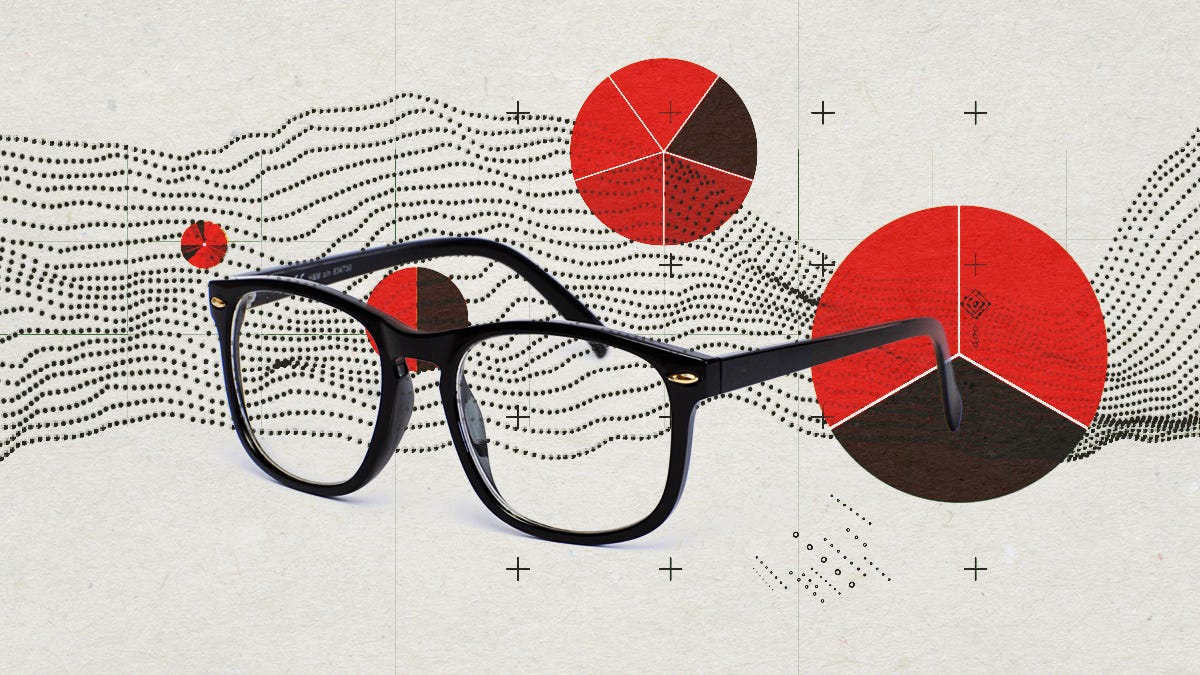
2.Data Scientists in Relation to Other Roles
The data science role is also now supplemented with a variety of other jobs.
The assumption in 2012 was that data scientists could do all required tasks in a data science application — from conceptualizing the use case, to interfacing with business and technology stakeholders, to developing the algorithm and deploying it into production.
Now, however, there has been a proliferation of related jobs to handle many of those tasks, including machine learning engineer, data engineer, AI specialist, analytics and AI translators, and data oriented product managers.
LinkedIn reported some of these jobs as being more popular than data scientists in its “ Jobs on the Rise “ reports for 2021 and 2022 for the U.S.
Part of the proliferation is due to the fact that no single job incumbent can possess all the skills needed to successfully deploy a complex AI or analytics system.
There is an increasing recognition that many algorithms are never deployed, which has led many organizations to try to improve deployment rates.
Additionally, the challenges of managing increased data systems and technologies have resulted in a more complex technical environment.
There have been some attempts at certification of data scientists and related jobs, but these are not yet widely sought or recognized.
Some companies, like TD Bank, have developed classification structures for the many data science-related careers and skills, but these are not common enough in organizations.
As a result of this proliferation of skills, companies need to identify all of the different roles required to effectively deploy data science models in their businesses, and ensure that they are present and collaborating on teams.
As a result of this proliferation of skills, companies need to identify all of the different roles required to effectively deploy data science models in their businesses, and ensure that they are present and collaborating on teams.
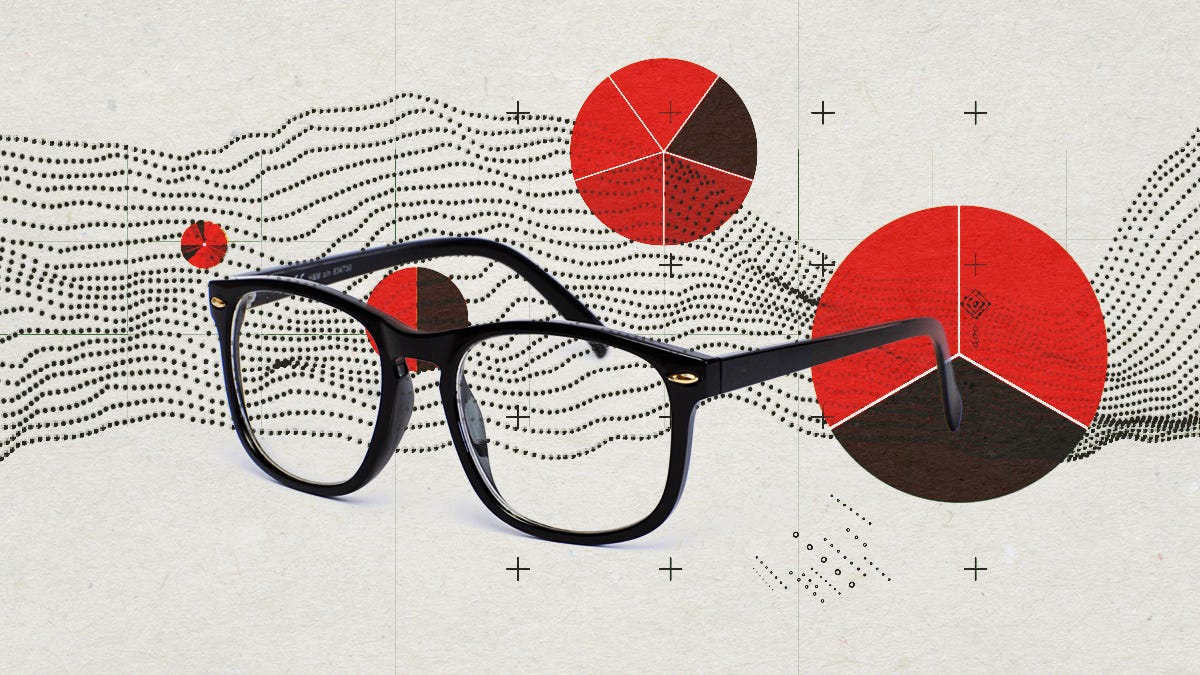
3.Changes in Technology
One reason why the data scientist job keeps changing is because the technologies data scientists use are changing.
Some technology trends are continuations of directions present in 2012, such as the use of open source tools and the move to cloud-based processing and data storage.
But some affect the core of data science work.
For example, some aspects of data science are increasingly automated (using automated machine learning or AutoML), which can both improve the productivity of data science professionals and open up the possibility of “citizen data scientists” with only some quantitative training.
These automated tools haven’t dimmed the appeal of professional data scientists yet, but they may in the future.
For example, some aspects of data science are increasingly automated (using automated machine learning or AutoML), which can both improve the productivity of data science professionals and open up the possibility of “citizen data scientists” with only some quantitative training.
These automated tools haven’t dimmed the appeal of professional data scientists yet, but they may in the future.
Companies should begin to democratize advanced analytics and AI within their organizations, relying on data scientists to ensure that citizen-developed models are accurate and that all relevant data is employed.
Companies should begin to democratize advanced analytics and AI within their organizations, relying on data scientists to ensure that citizen-developed models are accurate and that all relevant data is employed.
Data scientists have realized that their models can “drift” in turbulent business environments like the Covid-19 pandemic, so there is a new emphasis on monitoring their accuracy after deployment.
Machine learning operations, or “MLOps,” tools provide ongoing monitoring of models; automated retraining of drifted models is just beginning to be employed. Some AutoML and MLOps tools even test for algorithmic bias.
These developments mean that coding, which was perhaps the single most common job requirement when we wrote a decade ago, is somewhat less essential in data science.
It has migrated to other jobs or is being increasingly automated. (Data cleaning is a notable exception to this trend, however.)
These developments mean that coding, which was perhaps the single most common job requirement when we wrote a decade ago, is somewhat less essential in data science.
It has migrated to other jobs or is being increasingly automated. (Data cleaning is a notable exception to this trend, however.)
The key focus of the job continues to shift towards predictive modeling and the ability to translate business issues and requirements into models.
These are collaborative activities, but unfortunately there are as yet no great tools for structuring and supporting collaborative data science activities.
The key focus of the job continues to shift towards predictive modeling and the ability to translate business issues and requirements into models.
These are collaborative activities, but unfortunately there are as yet no great tools for structuring and supporting collaborative data science activities.
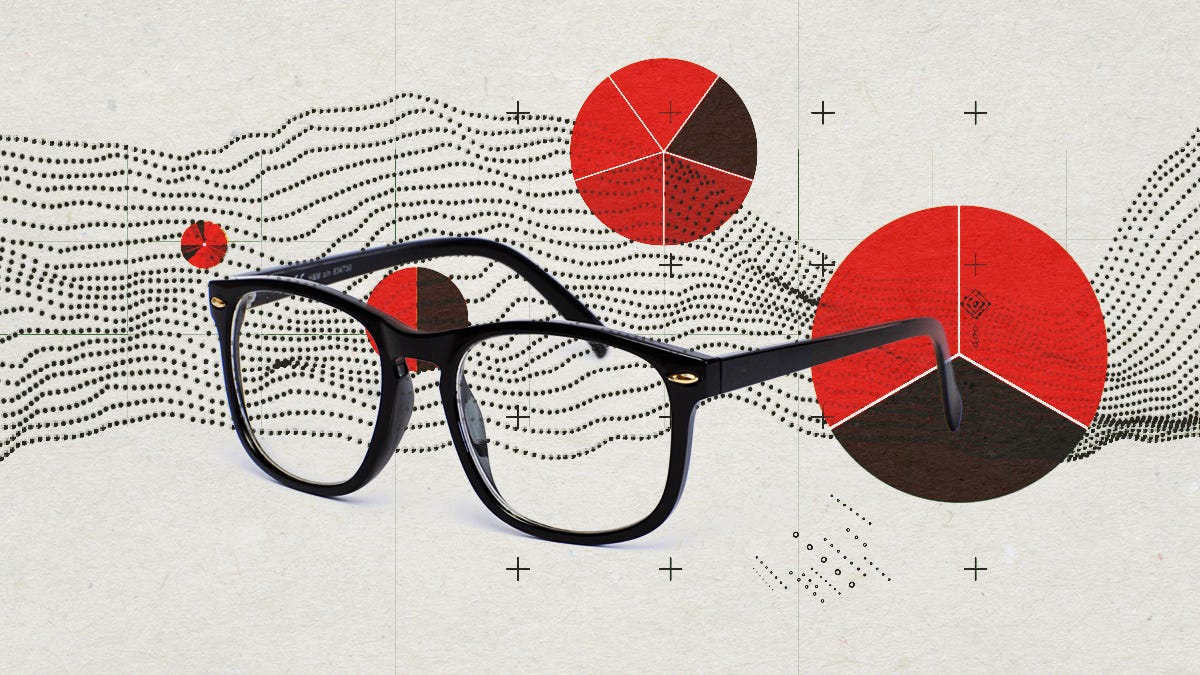
4.The Ethics of Data Science
A major change in data science over the past decade is that the need for an ethical dimension to the field is now widely acknowledged, though the topic was rarely mentioned in 2012.
The turning point for data science ethics was probably the 2016 U.S. presidential election, in which data scientists in social media ( Cambridge Analytica and Facebook in particular) attempted to influence voters and further polarized electoral politics.
Since that time, considerable attention has been devoted to issues of algorithmic bias, transparency, and responsible use of analytics and AI.
The turning point for data science ethics was probably the 2016 U.S. presidential election, in which data scientists in social media ( Cambridge Analytica and Facebook in particular) attempted to influence voters and further polarized electoral politics.
Since that time, considerable attention has been devoted to issues of algorithmic bias, transparency, and responsible use of analytics and AI.
Some companies have already established responsible AI groups and processes.
A key function of them is to educate data scientists about the issues involved in ethical AI. And there is an increased regulation that is being instituted in response to ethical lapses.
We have seen both continuity and change in the data science role.
It has been remarkably successful in many ways, and some of its challenges — proliferation of related roles, the need for an ethical perspective — result in part from the widespread adoption of data science.
The amount of data, analytics, and AI in business and society seem unlikely to decline, so the job of data scientist will only continue to grow in its importance in the business landscape.
The amount of data, analytics, and AI in business and society seem unlikely to decline, so the job of data scientist will only continue to grow in its importance in the business landscape.
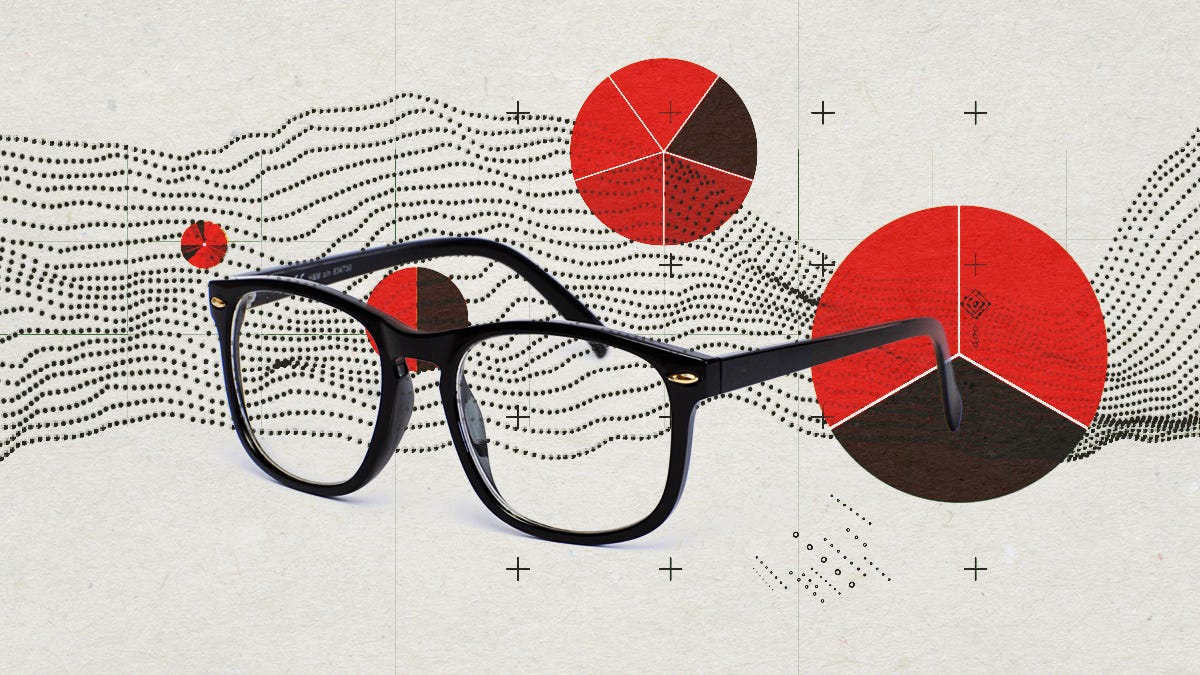
However, it will also continue to change.
We expect to see continued differentiation of responsibilities and roles that all once fell under the data scientist category.
Companies will need detailed skill classification and certification processes for these diverse jobs, and must ensure that all of the needed roles are present on large-scale data science projects.
Professional data scientists themselves will focus on algorithmic innovation, but will also need to be responsible for ensuring that amateurs don’t get in over their heads.
Most importantly, data scientists must contribute towards appropriate collection of data, responsible analysis, fully-deployed models, and successful business outcomes.
Originally published at https://hbr.org on July 15, 2022.
Editor’s note: This post has been updated.